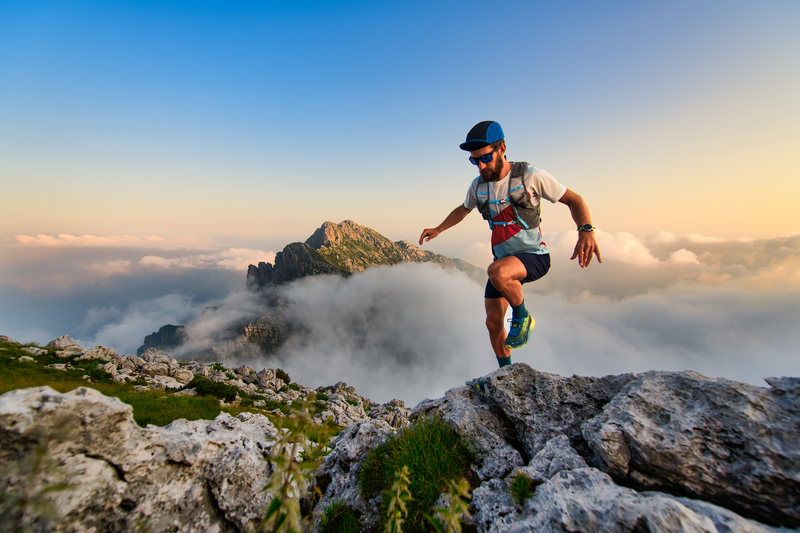
95% of researchers rate our articles as excellent or good
Learn more about the work of our research integrity team to safeguard the quality of each article we publish.
Find out more
ORIGINAL RESEARCH article
Front. Environ. Sci. , 22 November 2024
Sec. Environmental Economics and Management
Volume 12 - 2024 | https://doi.org/10.3389/fenvs.2024.1492454
This article is part of the Research Topic Advancing Carbon Reduction and Pollution Control Policies Management: Theoretical, Application, and Future Impacts View all 32 articles
Reducing agricultural carbon emissions is critical to achieving green agricultural development and the “dual carbon” goals. The present study conducts empirical analysis using provincial panel data from 29 provinces in China from 2011 to 2022 combined with econometric models based on the mechanism of the impact of digital rural construction on agricultural carbon emission intensity. The entropy method and carbon emission factor method are used to determine the level of digital rural construction and agricultural carbon emission intensity. The fixed effect and intermediary effect models are used to empirically analyze the impact of digital rural construction on agricultural carbon emission intensity. The results indicate that (1) digital rural construction significantly inhibits agricultural carbon emission intensity, and there are differences in different regions and dimensions of digital rural construction; (2) the construction of digital rural areas can indirectly reduce the intensity of agricultural carbon emissions by promoting the level of rural human capital; (3) financial support for agriculture played significant positive regulatory effect. The policy recommendations are proposed to provide a reference for promoting agricultural carbon reduction and digital rural construction in other countries.
Food production and total agricultural output have grown in recent years, but the accompanying problem of agricultural carbon emissions is becoming increasingly serious. According to the “Global Agriculture Outlook 2022–2031” report, greenhouse gas emissions directly generated by agriculture are projected to increase by 6% over the next decade. As a major agricultural country, China actively undertakes the significant responsibility of reducing agricultural carbon emissions and has introduced various plans to strengthen carbon reduction in agriculture. For instance, in September 2020, China set the goals of “peaking carbon emissions by 2030” and achieving “carbon neutrality by 2060”. In 2022, China issued the “Collaborative Implementation Plan for Pollution Reduction and Carbon Reduction”, which emphasized deepening the implementation of actions to reduce the quantity and improve the efficiency of fertilizers and pesticides to meet carbon reduction requirements. Reducing agricultural carbon emissions while ensuring food security has become a common concern for China and the world.
Agricultural carbon emission refers to the greenhouse gas emissions directly or indirectly generated by various activities in the process of agricultural production. Research on agricultural carbon emissions mainly focuses on carbon sources, carbon emission measurement, influencing factors, and carbon reduction measures. There are six primary sources of carbon emissions (Zhang et al., 2022). The calculation methods for agricultural carbon emissions primarily include the default coefficient of the IPCC (Sperow, 2020) and the life cycle assessment method (Jana and De, 2016). Agricultural carbon emissions influence economic development, urbanization, and energy use (Qing and Yuhang, 2022; Xu and Lin, 2017; Wei Z. et al., 2023). Land use patterns, farmland ecosystems, and soil erosion can affect the carbon balance of agricultural systems (Woomer et al., 2004; Lal, 2003; Kindler et al., 2011). Measures for agricultural carbon reduction mainly involve technological innovation, technological progress, industrial structure optimization (Li G. et al., 2024; Li J. et al., 2024; Wang et al., 2023), collecting environmental taxes (Iyke-Ofoedu et al., 2024), encouraging farmers to join rural cooperatives and adopt socialized services (Wang and Qiu, 2024; Chen et al., 2022), adjusting fertilizer use (Ji et al., 2024), promoting large-scale agricultural land management (Bai et al., 2023), and implementing crop rotation and fallow practices (Zhang et al., 2024). Financial support for agriculture, industrial upgrading and clean agricultural production technologies are effective for reducing agricultural carbon emissions (Wei S. et al., 2023; Du et al., 2023; Guo et al., 2022).
“The Outline of the Strategy for the Development of Digital Countryside” points out that the construction of digital rural countryside refers to the application of networking, informatization and digitalization in agricultural and rural development and the process of promoting the modernization and transformation of agriculture and rural areas, which is not only the strategic direction of rural revitalization but also an important content of the construction of digital China. “The Action Plan for Digital Rural Development (2022–2025)” emphasizes the application of digitalization in rural areas, positioning data as an essential input factor in modern agricultural production. In this context, exploring the impact of digital rural construction on agricultural carbon emission intensity can offer new insights into agricultural carbon reduction and assess the role of digital rural strategies in agricultural development. The Internet can facilitate land circulation among farmers and help them adopt agricultural productive services, thereby improving agricultural green total factor productivity (Liu et al., 2022). Digital inclusive finance can optimize resource allocation and reduce agricultural carbon emissions (Hong et al., 2024; Liu et al., 2024). The higher the level of digital finance development, the stronger its role in financial agglomeration and reducing agricultural carbon emissions (Li, 2023; Zhao et al., 2023). Digitalization can lower carbon intensity by enhancing agricultural technology, human capital levels, and urbanization rates, with regional variations (Wang et al., 2024). The relationship between digital agriculture growth and agricultural green total factor productivity exhibits an inverted U-shaped curve (Zhou et al., 2023).
As a predominantly agricultural country, China serves as a typical representative of global digital rural construction (Zhang et al., 2023). With the promotion of relevant national policies, several critical questions arise: How does the construction of digital rural areas affect the intensity of agricultural carbon emissions? Is there heterogeneity in the effects between different regions? What are the intermediate transmission mechanisms? What factors can regulate the carbon emission reduction effect of digital rural construction on agriculture? Clarifying these issues is essential for evaluating the impacts of rural digital construction, seizing the opportunities it presents, and exploring new strategies for agricultural carbon reduction.
Previous studies on agricultural carbon emissions mainly focused on the measurement of carbon emissions and the decomposition of influencing factors. Compared with previous studies, this paper makes several contributions. First, it innovates in research by exploring the impact of digital rural construction on agricultural carbon emission intensity, aligning with the “dual carbon” strategy, “digital countryside” strategy, thus providing new insights into reducing global agricultural carbon emissions,The construction of digital countryside brings new opportunities for agricultural carbon emission reduction. Second, it innovates in research content by constructing the index system, calculating the levels of digital rural construction and agricultural carbon emission intensity through an index system and clarifying the logical mechanisms between these systems using a combination of literature review and relevant theories. The study empirically analyzes the impact of digital rural construction on agricultural carbon emission intensity. It examines the intermediary effect of rural human capital, the regulatory implications of financial support for agriculture. This is conducive to understanding the logical mechanism behind the construction of digital rural construction to reduce agricultural carbon emission and the direction of policy regulation in various countries. The research provides suggestions for leveraging digital rural construction to achieve low-carbon agricultural development so as to promote the achievement of global carbon reduction targets.
The rest of chapters are arranged as follows: Section 2 offers literature review and research hypotheses. Section 3 introduces the methods, variable selection and description and data source. The results of the study are presented in the Section 4. Section 5 presents the discussion and several policy recommendations. The last section summarizes the conclusions.
Foreign scholars have explored the concept of the digital countryside primarily from the perspectives of digital agriculture or technology (Rotz et al., 2019; Engås et al., 2023). In 2019, China issued the “Digital Rural Development Strategy Outline”, providing a specific definition: digital rural construction refers to the application of networking, informatization, and digitization in agricultural and rural development, aiming to promote the modernization and transformation of agriculture and rural areas. There is yet to be a unified standard for evaluating digital rural construction. The calculation method is mainly based on the comprehensive evaluation model using the entropy method. Indicators typically involve the construction of digital rural information infrastructure, digital rural financial infrastructure, and digital rural service platforms (Ping et al., 2024; Hao and Tan, 2022). Some approaches replace the level of digital rural construction with a single indicator, such as the county-level digital rural index (Linghui and Yongxin, 2022).
With the implementation of the “Broadband China” and “Digital Rural” strategies, digital technology has become a significant input factor in agricultural production in the new era. Based on the theory of the digital economy, the construction of a digital rural construction can leverage the substitution and integration effects of the digital economy to alleviate the mismatch of agricultural resource factors and improve agriculture’s total green factor productivity (Honghai and Xinmin, 2023). Digital rural construction can reduce farmers’ transaction costs and alleviate market information asymmetry, effectively lowering agricultural production costs and environmental pollution (Rolandi et al., 2021). This construction has accelerated the widespread dissemination of advanced technologies in rural areas (Popescu et al., 2020), and technological innovation is a crucial measure to promote agricultural carbon reduction. It facilitates the recombination and efficient allocation of various production factors, thereby improving production efficiency (Acemoglu and Restrepo, 2018). Due to specific differences in resource endowments among different regions, the impact of digital rural construction on agricultural carbon emission intensity varies across areas.
Hypothesis 1:. Digital rural construction is beneficial for reducing agricultural carbon emission intensity.
Hypothesis 2:. The impact of digital rural construction on agricultural carbon emission intensity has regional differences.
The government can disseminate information on agricultural low-carbon production technologies to farmers through rural digital platforms. Farmers can utilize the Internet and social media to access high-quality educational resources, enabling them to acquire advanced green production technology quickly to enhance their labor skills, knowledge level, and environmental awareness. This can influence rural labor behavior, encouraging farmers to adopt green production practices beneficial for agricultural carbon emission reduction (Ma et al., 2022). The construction of digital rural areas can overcome the limitations of traditional rural areas in terms of talent, resources, time, and space, thereby improving resource allocation efficiency, promoting the enhancement of green total factor productivity in agriculture, and exerting a substitution effect on the rural labor force, alleviating issues related to insufficient rural labor. Digital technology, as a fundamental driver in digital rural area construction, can catalyze changes in agricultural workers’ skills and other elements, forming digital agrarian productivity (Junge and Qinmei, 2023). In promoting digital rural construction, digital technology can lower the cost of farmers’ access to market information (Mary George et al., 2016; Song et al., 2020), enhance human capital by focusing on farmers’ digital literacy, reinforce environmental awareness, and encourage green production practices (Aldieri et al., 2019). The proliferation of rural digitization has paved new pathways for developing low-carbon agriculture.
Hypothesis 3:. The construction of digital agriculture can reduce the intensity of agricultural carbon emissions by promoting the level of rural human capital.
Currently, agricultural management entities in China predominantly consist of small-scale operations, and the construction of digital rural areas constitutes projects with significant externalities and essential public welfare attributes. It is a comprehensive undertaking involving various components, such as network infrastructure construction and the enhancement of rural human capital, which necessitate financial backing. However, farmers typically need more financial resources to undertake such endeavors. The level of economic development and the availability of financial support are critical factors influencing the effectiveness of digital rural construction (Xing et al., 2023). Moreover, financial support for agriculture can facilitate the aggregation of information, technology, and talent, thereby aiding in the high-level development of digital rural areas (Chunlin et al., 2024).
Hypothesis 4:. financial support for agriculture can positively influence the impact of digital rural construction on agricultural carbon emission intensity. Based on the above theoretical analysis, the theoretical framework diagram of the impact of digital rural construction on agricultural carbon emission intensity was constructed, as shown in Figure 1.
AHP and Delphi methods are commonly employed to determine indicator weights, yet they entail significant subjectivity. Therefore, the entropy method is utilized to ascertain the weights of each indicator, enhancing the scientific validity of the evaluation results, which boast high credibility and accuracy. The specific application methods of the entropy method can be found in some literature (Chen and Zhang, 2023).
①Dimensionless treatment of indicatorsconverting the indicator values of each indicator to 0–1, which can avoid the impact of different indicator dimensions.
②Standardized processing of raw indicators
The dimensionless processed indicators are standardized and translated to obtain the standardized indicator values:
③Calculate the proportion of indicator value
④Calculate the entropy value of the indicator
⑤Calculate the differentiation coefficient of the indicator
⑥Calculate the weight of the indicator
⑦Calculate the comprehensive evaluation value in each province
The carbon emission measurement method based on IPCC (Intergovernmental Panel on Climate Change) carbon emission coefficient is the common method to estimate carbon emission at present with the advantages of simple calculation process, easy popularization and less data demand. In china there are six primary sources of agricultural carbon emissions (Li et al., 2011), including pesticides, chemical fertilizers, agricultural fuel, agricultural plastic film, agricultural sowing areas, and agricultural irrigation areas. The corresponding carbon emission coefficients are as follows (Li et al., 2011; West and Marland, 2002; Zhi and Gao, 2009; Wu et al., 2007; Dubey and Lal, 2009): 4.9341 kg/kg,0.8956 kg/kg, 0.5927 kg/kg, 5.180 kg/kg, 312.60 kg/km2, 25 kg/hm2. The total agricultural carbon emissions are calculated as follows:
Where ac represents the total agricultural carbon emissions, aci represents the carbon emissions of various carbon sources, Ti and δi respectively represent the actual consumption of multiple carbon sources and their corresponding carbon emission coefficients.
To test the impact of digital rural construction on agricultural carbon emission intensity, the benchmark regression model constructed is as follows:
Where acintensityit is the agricultural carbon emission intensity of Province i in year t, digcountyit is the digital rural construction level of Province i in year t, and controls is a series of control variables. μi and λt refer to the fixed province effect and time effect, respectively, and εit refers to the random disturbance term.
Utilizing the stepwise regression method (Wen and Ye, 2014) for testing, the model is constructed as follows:
Where rural human capital is the intermediary variable, c is the total effect coefficient of digital rural construction affecting agricultural carbon emission intensity; a is the effect of the core explanatory variable of digital rural construction on the intermediary variable of rural human capital; c' is the direct effect of digital rural construction on agricultural carbon emission intensity after controlling the influence of rural human capital; b is the indirect effect of the intermediary variable rural human capital on agricultural carbon emission intensity after controlling the impact of the core explanatory variable digital rural construction.
To further analyze the regulatory effect of financial support for agriculture on the impact of digital rural construction on agricultural carbon emission intensity, the regulatory effect model is developed as follows:
(1) Explained Variable. The explained variable in this paper is agricultural carbon emission intensity (variable: acintensity). Agricultural carbon emission is calculated by carbon emission coefficient method (see Equation 1). Agricultural carbon emission intensity is the total agricultural carbon emission ratio to total agricultural output value (Xueqiang et al., 2023).
(2) Explanatory Variable: The primary explanatory variable in this study is digital rural construction (variable:digcounty). As the establishment of “Taobao Villages” has been prevalent in numerous provinces for several years, it is not considered in this paper. The construction of digital rural information infrastructure primarily indicates the transformation of information dissemination and the proliferation of modern information technology in rural areas. Financial infrastructure mainly represents the digital economic infrastructure. Establishing a digital service platform predominantly reflects the state of digital logistics. The specific index system is outlined in Table 1.
(3) Intermediary Variables: The intermediary variable in this paper is rural human capital (variable: lnhuman), with per capita years of education in rural areas chosen to represent the development level of rural human capital in each province.
(4) Regulatory Variables: The regulatory variable in this paper is financial support for agriculture (variable:financialsupport), measured by the proportion of local financial expenditures on agriculture, forestry, and water affairs, local general budget expenditures.
(5) Control Variables: To account for the influence of other factors on agricultural carbon emission intensity and avoid interference, the following control variables are selected:Urbanization (variable:urbanization): Measured by the proportion of the urban population in different regions at the end of the year. Disaster rate (variable:disasterrate): Measured by the proportion of the affected area as a percentage of the planted crop area. Technological innovation development (variable:lntechnology): Measured by the number of patents granted. Agricultural industry structure (variable:agrstructure): Reflects the development of various industries in the region, measured by the proportion of the primary industry in the regional economy. Agricultural mechanization strength (variable:lnmachstrength): Measured by dividing the total power of agricultural machinery by the cultivated land area. Intensity of science and technology expenditure (variable:tcf): Measured by the proportion of science and technology expenditure to local general public budget expenditure.
The research sample comprises 29 provinces in China. Due to a significant number of missing values for specific indicators of digital countryside in Xizang and Shanghai, these provinces are temporarily excluded from the calculation to ensure the accuracy of the research. The study period spans from 2011 to 2022. Data sources include the China Statistical Yearbook, China Rural Statistical Yearbook, and the Peking University Digital Financial Inclusion Index. For individual missing data, the interpolation method is employed for completion. The statistical characteristics of essential variables are presented in Table 2.
A scatter plot and fitting line illustrating the relationship between digital rural construction and agricultural carbon emission intensity were generated using the sample data, as depicted in Figure 2. It is evident that as the level of digital rural construction increases, agricultural carbon emission intensity decreases, indicating a negative correlation between the two variables. This preliminary observation suggests that digital rural construction may reduce agricultural carbon emission intensity, aligning with the theoretical derivation presented earlier in this paper. Further analysis will involve the inclusion of control variables and the adoption of multiple models for verification.
Figure 2. Scatter plot and fitting line of digital rural construction and agricultural carbon emission intensity.
Prior to conducting the baseline regression, the variance inflation factor (vif) was utilized to test for collinearity among variables. The testing revealed that the minimum vif value for each variable was 1.43, the maximum was 4.06, and the average was 2.71,which is significantly less than 10. Therefore, there was no collinearity issue among the variables. Table 3 presents the benchmark regression results of “Digital rural construction-agricultural carbon emission intensity”. The calculation results are obtained by referring to Equation 2. Columns (1) and (2) display the outcomes of the fixed effects model, indicating that digital rural construction significantly reduces agricultural carbon emission intensity. Without control variables, the coefficient of digital rural construction is −0.090, exhibiting a substantial correlation at the 1% significance level. Even after incorporating a series of control variables, the coefficient remains −0.087, still significantly correlated at the 1% level. This suggests that digital rural construction exerts a significant carbon reduction effect on agriculture. Columns (3) and (4) present the results of the random effects model. The Hausman test indicates that chi2 (6) = 25.85, Prob > chi2 = 0.0002, thereby rejecting the null hypothesis and affirming that the fixed effects model is more suitable. The baseline regression results unequivocally demonstrate that digital rural construction significantly diminishes the intensity of agricultural carbon emissions, thereby confirming hypothesis 1.
(1) Replace Core Explanatory Variables. Carbon emissions from food production are a significant component of agricultural carbon emissions. To test robustness, the intensity of agricultural carbon emissions is replaced with the carbon emission intensity from food production, and the baseline regression is performed again. The results are presented in Table 4 (1).
(2) Reduce Sample Size. Municipalities like Beijing, Chongqing, and Tianjin differ markedly from other provinces regarding government resource support and agricultural development. Therefore, these municipalities are excluded from the sample, and the regression analysis is conducted again with the remaining samples. The results, shown in Table 4 (2), indicate that the estimated coefficient of digital rural construction is significantly negative at the 5% level.
(3) Indentation of Sample Variables. To avoid the interference of extreme values on regression results, all variables are winsorized at the 1% level. The suffix _w is used to denote variables after winsorization. The results are shown in Table 4. After this data adjustment, the estimated coefficient of digital rural construction remains significantly negative at the 5% level, further demonstrating the robustness of the baseline regression results.
The findings from these three robustness tests consistently show a negative and significant coefficient for digital rural construction, affirming the robustness of the baseline regression conclusion: the construction of digital countryside can significantly reduce agricultural carbon emission intensity.
Using the second lag of digital rural construction (L2. digcounty) as an instrumental variable helps avoid endogenous estimation bias caused by two-way causality. The initial phase of digital rural construction creates favorable conditions for subsequent stages, satisfying the relevance requirement. Early-stage digital countryside construction reduces agricultural carbon emission intensity in the current period, meeting the homogeneity requirement. The two-stage least squares method (IV_2SLS) was employed to re-examine the impact of digital rural construction on agricultural carbon emission intensity (see Table 5). In the first-stage regression results, the instrumental variable L2. digcounty is positively correlated with the endogenous variable (discount) at the 1% level, indicating that the chosen instrumental variable is sufficiently associated with the endogenous variable. In the under identification test, the LM value is 112.230, passing the 1% significance test, which confirms the absence of under identification issues. The Cragg-Donald Wald F-value is 4,276.309, exceeding the critical value of 16.38 at the 10% significance level for the Stock-Yogo weak instrumental variable test, indicating no weak instrumental variable issues. The Hansen J statistic indicates no over identification problem, affirming the appropriateness of the selected instrumental variable. After addressing the endogeneity problem using the instrumental variable method, digital rural construction continues to show a significant negative correlation with agricultural carbon emission intensity at the 1% level, confirming the robustness of the result.
Divide the country into two categories based on whether it is a major grain-producing area. The regression results are shown in Table 6. The regression coefficients for digital rural construction are negative and pass the significance tests at 1% and 5%. However, the degree of influence varies across different regions. Digital rural construction significantly inhibits agricultural carbon emission intensity in major grain-producing areas. This effect is due to the country’s favorable resource allocation towards these grain-producing areas. On the one hand, the major grain-producing areas bear the heavy responsibility of maintaining national food security, the state attaches great importance to the development of major grain-producing areas, and has introduced a series of targeted policies to promote the construction of digital countryside, such as providing a number of financial funds for the construction of digital countryside in major grain-producing areas for infrastructure construction, digital technology research and development and application promotion. The main grain-producing areas have relatively good network coverage and perfect infrastructure, which provides the necessary basic conditions for the construction of digital countryside. On the other hand, major grain-producing areas usually have large areas of farmland which facilitate the large-scale application of digital technology in agricultural production. The observed regional differences, attributed to varying resource endowments, confirm hypothesis 2.
This part analyzes the impact of the sub-dimension of digital rural construction on agricultural carbon emission intensity (see Table 7). Rural broadband access users and the average mobile telephone ownership per 100 rural households significantly reduce agricultural carbon emission intensity, each passing the 1% significance test. The total index of digital inclusive finance within the construction of digital rural finance and the length of rural delivery routes within the construction of digital rural service platforms show no significant effect on carbon emissions. But when the level of digital inclusive finance is above the 0.25 quartile value (lndigfi ≥ 5.106) and the length of rural delivery route is above the 0.75 quartile value (lndelroute ≥ 12.154), the agricultural carbon emission intensity is also significantly reduced, and both pass the significance test of 10%. In other words, with the continuous improvement of digital inclusive finance and the length of rural delivery routes, the effect on agricultural carbon emission reduction will gradually increase, and there is a nonlinear relationship between them. The sub-dimensional construction level is related to the overall level of digital rural construction. The mobile phones and the Internet enables farmers to obtain weather information and information of agricultural materials products earlier, so they can implement scientific and reasonable irrigation and fertilization, and avoid environmental problems caused by excessive use of agricultural materials. The popularization of digital inclusive finance and the construction of express routes require a large amount of investment in the early stage, then the carbon reduction effect will gradually appear in the later stage, so as to achieve the purpose of reducing agricultural carbon emission intensity.
The theoretical analysis indicates that digital rural construction can directly reduce agricultural carbon emission intensity and indirectly do so by enhancing the knowledge and skills of laborers, thereby leveraging the “human capital effect”. This paper employs a three-step method to build an intermediary effect model, with the measurement test results shown in Table 8, the calculation results refer to Equations 3–5. The coefficients in columns (1), (2), and (3) are all significantly correlated, suggesting that rural human capital plays a partial mediating role in the carbon reduction effect of digital rural construction on agriculture. The Sobel test was used to verify the robustness of the results of the intermediary effect further. The total utility coefficient of digital rural construction on agricultural carbon emission intensity was −0.087, the effect coefficient of digital rural construction on the intermediary variable rural human capital was 0.113, and the total utility coefficient of rural human capital on agricultural carbon emission intensity was −0.126. These correlations were significant at the 1%, 1%, and 5%, respectively. The direct effect coefficient is −0.073, significantly correlated at 5%, while the indirect effect coefficient is −0.014, accounting for 16.3% of the total effect. The direct effect accounts for 83.7%. The intermediary effect passed both the stepwise test of the three-step method and the Sobel test, demonstrating the robustness of the intermediary effect and confirming hypothesis 3.
Theoretical analysis indicates that financial support for agriculture influences the effect of digital rural construction on agricultural carbon emission intensity. An interaction term between financial support for agriculture and digital rural construction was introduced to test this hypothesis, avoiding collinearity between variables. After decentralizing the data, the variables of financial support for agriculture and digital rural construction were combined to form the interaction term c_digcounty *c_financialsupport. Columns (1) and (2) in Table 9 compare the results before and after introducing the interaction term, the calculation results refer to Equation 6. The coefficients of the main effect of digital rural construction are negative in both cases. After introducing the interaction term, its coefficient is −1.676. The original coefficient of digital rural construction changes from −0.082 to −0.118, showing a more significant effect. This indicates that the negative impact of digital rural construction on agricultural carbon emission intensity is amplified with increased financial support for agriculture, thereby verifying hypothesis 4.
This study not only elucidated the logical mechanism between digital rural construction and agricultural carbon emission intensity through a literature review and related theories but also comprehensively analyzed the impact path and degree from intermediary effect and regulatory effect. Future development suggestions were proposed to enhance the study’s applicability. The primary regression results indicate that digital rural construction significantly and negatively affects agricultural carbon emission intensity, with regional differences, aligning with previous scholarly findings. Digital rural development can reduce agricultural carbon emissions and improve total factor productivity in agriculture. The higher the level of economic growth, the stronger the carbon emission reduction effect of digital rural construction.
Compared to previous studies, this research has made the following improvements:
(1) It combines theoretical mechanism analysis with scientific demonstration, verifying digital rural construction’s overall carbon reduction effect and further analyzing its dimensions.
(2) It emphasizes the critical role of digital rural construction in enhancing rural human capital and underscores the importance of financial support from government.
This study uses China as a case study to analyze the impact of digital rural construction on agricultural carbon emission intensity, providing a valuable reference for other countries aiming for agricultural low-carbon emission reduction. While emphasizing the importance of rural human capital, further analysis is needed on how to promote these aspects in different regions better. Enhancing rural human capital to bolster the role of digital rural construction in reducing agricultural carbon emissions. With the intensification of global climate change, reducing carbon emissions has become a global goal, which requires joint efforts of all countries. Carbon emissions come mainly from human activities and natural processes, such as the burning of fossil fuels (Baoguo et al., 2022), development of industrialization (Zhenshuang et al., 2022), urban land use (Xueqiang et al., 2023) and development of agriculture. About one-fifth of the world’s greenhouse gases come from agriculture according to a report by the United Nations Food and Agriculture Organization. Agriculture must do something active to fight climate change because it is closely related to global sustainable development. Agriculture is an important industry in many countries, especially in developing countries. Digital rural construction can improve agricultural production efficiency by improving the human capital level of farmers, and reduce agricultural carbon emission intensity. Financial support for agriculture played significant positive regulatory effect. Promote the international exchange of China’s digital countryside construction and agricultural carbon emission reduction experience can contribute to the low-carbon development and sustainable development of global agriculture.
Some policy recommendations are proposed according to empirical results:
(1) Pay more attention to the carbon reduction impact of digital rural construction in agricultural development and accelerate the pace of digital rural construction. Enhancing internet accessibility in rural areas is foundational for the digital rural construction. Cornwall region is the representative of the implementation of the comprehensive strategy of rural digitalization in the United Kingdom, mainly implementing broadband access and digital training to strengthen the construction of digital countryside. The application of digital technology in agriculture can be optimized by the network infrastructure. For example, in the United States, farmers can accurately fertilize, irrigate according to precise data such as soil conditions and crop growth, avoiding the waste of resources and reducing carbon emissions in agricultural production.
(2) Focus on enhancing rural human capital, recognizing its long-term significance in agricultural productivity. Empowering rural labor with skills enhances productivity. Given the pivotal role of the “human capital effect” in carbon reduction, efforts should promote low-carbon concepts among small and medium-sized farmers. Initiatives like “technology to the countryside” should encourage farmers to adopt new technologies and agricultural methods, ensuring a smooth transmission path for the human capital effect of the digital countryside.
(3) Increase central financial support for digital agriculture development. Such support plays a vital role in regulating digital countryside construction. Recognizing the infancy of digital agriculture in certain areas, especially those with weak financial resources and infrastructure, the central government should provide targeted subsidies and investments to bolster development. Securing financial investment is paramount for the ambitious project of digital rural construction.
(4) Formulating differentiated countermeasures to heterogeneity results. In view of regional heterogeneity, major grain-producing areas should play an exemplary role for other regions. For example, integrate agricultural production data, market information and technical services to provide digital services for farmers. From the sub-dimension of digital rural construction, the mobile phones and the Internet played a significant role in promoting agricultural carbon emission reduction which need supportting. But the governments also need to actively promote digital financial inclusion in rural areas. Digital inclusive finance is the product of the combination of digital technology and inclusive finance, which has an important impact on farmers’ employment and production.
Drawing on provincial panel data from 2011 to 2022, this study employed the entropy and carbon emission factor methods to gauge China’s digital rural construction and agricultural carbon emission intensity. Through panel fixed effect, intermediary effect, regulatory effect, the study explored the impact of digital rural construction on agricultural carbon emission intensity, yielding the following key conclusions:
(1) Digital rural construction significantly reduces agricultural carbon emission intensity, indicating its potential to agricultural carbon emission reduction. This conclusion remains robust even after accounting for endogeneity and robustness testing.
(2) The impact of digital rural construction on agricultural carbon emission intensity varies across regions, with a more pronounced effect observed in major grain-producing areas. Among digital rural construction subdimensions, digital infrastructure construction notably curbs agricultural carbon emissions.
(3) Intermediary effect analysis reveals that digital rural construction indirectly reduces agricultural carbon emissions by elevating rural human capital, harnessing the “human capital effect” to achieve emission reduction. The direct and indirect effects account for 83.7% and 16.3% of the total impact, respectively. Enhanced rural human capital influences planting decisions and behaviors, affecting agricultural carbon emissions. Digital rural construction transcends temporal and spatial constraints, facilitating farmer access to new technologies and bolstering environmental awareness.
(4) Regulatory effect analysis demonstrates that government financial support for agriculture positively adjusts the impact of digital rural construction, with varying effects across regions based on different levels of financial support.
The original contributions presented in the study are included in the article/supplementary material, further inquiries can be directed to the corresponding author.
WL: Methodology, Writing–original draft, Writing–review and editing. JG: Conceptualization, Methodology, Writing–review and editing. YT: Software, Writing–review and editing. PZ: Methodology, Writing–review and editing.
The author(s) declare that financial support was received for the research, authorship, and/or publication of this article. This study was supported by the following project: Dongying Science Development Fund Project (DJB2023014).
The authors thank the institutions that provided funding.
The authors declare that the research was conducted in the absence of any commercial or financial relationships that could be construed as a potential conflict of interest.
All claims expressed in this article are solely those of the authors and do not necessarily represent those of their affiliated organizations, or those of the publisher, the editors and the reviewers. Any product that may be evaluated in this article, or claim that may be made by its manufacturer, is not guaranteed or endorsed by the publisher.
Acemoglu, D., and Restrepo, P. (2018). The race between man and machine: implications of technology for growth, factor shares, and employment. Am. Econ. Rev. 108 (6), 1488–1542. doi:10.1257/aer.20160696
Aldieri, L., Barra, C., and Vinci, C. P. (2019). The role of human capital in identifying the drivers of product and process innovation: empirical investigation from Italy. Qual. and Quantity 53, 1209–1238. doi:10.1007/s11135-018-0812-8
Bai, J., Liu, J., Ma, L., and Zhang, W. (2023). The impact of farmland management scale on carbon emissions. Land 12 (9), 1760. doi:10.3390/land12091760
Baoguo, S., Wenjing, X., Xiaodan, B., Wang, Y., Geng, G., and Zheng, J. (2022). District level decoupling analysis of energy-related carbon dioxide emissions from economic growth in Beijing, China. Energy Rep. 8, 2045–2051. doi:10.1016/j.egyr.2022.01.124
Chen, C., and Zhang, H. (2023). Evaluation of green development level of Mianyang agriculture, based on the entropy weight method. Sustainability 15 (9), 7589. doi:10.3390/su15097589
Chen, Z., Tang, C., Liu, B., Liu, P., and Zhang, X. (2022). Can socialized services reduce agricultural carbon emissions in the context of appropriate scale land management? Front. Environ. Sci. 10, 1039760. doi:10.3389/fenvs.2022.1039760
Chunlin, X., Shijie, Z., Fen, L., et al. (2024). Research on influencing factors and configuration path of high-quality construction of digital countryside - qualitative comparative analysis based on clear set of 37 cases. World Agric. 1, 66–78. doi:10.13856/j.cn11-1097/s.2024.01.006
Du, Y., Liu, H., Huang, H., and Li, X. (2023). The carbon emission reduction effect of agricultural policy——evidence from China. J. Clean. Prod. 406, 137005. doi:10.1016/j.jclepro.2023.137005
Dubey, A., and Lal, R. (2009). Carbon footprint and sustainability of agricultural production systems in Punjab, India, and Ohio, USA. J. Crop Improv 23, 332–350. doi:10.1080/15427520902969906
Engås, K. G., Raja, J. Z., and Neufang, I. F. (2023). Decoding technological frames: an exploratory study of access to and meaningful engagement with digital technologies in agriculture. Technol. Forecast. Soc. Change 190, 122405. doi:10.1016/j.techfore.2023.122405
Guo, L., Guo, S., Tang, M., Su, M., and Li, H. (2022). Financial support for agriculture, chemical fertilizer use, and carbon emissions from agricultural production in China. Int. J. Environ. Res. Public Health 19 (12), 7155. doi:10.3390/ijerph19127155
Hao, A., and Tan, J. (2022). Impact of digital rural construction on food system resilience. J. South China Agric. Univ.(Soc. Sci. Ed.) 21, 10–24. doi:10.7671/j.issn.1672-0202.2022.03.002
Hong, H., Sun, L., and Zhao, L. (2024). Exploring the impact of digital inclusive finance on agricultural carbon emissions: evidence from the mediation effect of capital deepening. Sustainability 16 (7), 3071. doi:10.3390/su16073071
Honghai, G., and Xinmin, L. (2023). Whether digital rural construction can improve agricultural green total factor productivity by alleviating the mismatch of resource factors. Ningxia Soc. Sci. 5, 107–117. doi:10.3969/j.issn.1002-0292.2023.05.013
Iyke-Ofoedu, M. I., Takon, S. M., Ugwunta, D. O., Ezeaku, H. C., Nsofor, E. S., and Egbo, O. P. (2024). Impact of CO2 emissions embodied in the agricultural sector on carbon sequestration in South Africa: the role of environmental taxes and technological innovation. J. Clean. Prod. 444, 141210. doi:10.1016/j.jclepro.2024.141210
Jana, K., and De, S. (2016). Environmental impact of an agro-waste based polygeneration without and with CO2 storage: life cycle assessment approach. Bioresour. Technol. 216, 931–940. doi:10.1016/j.biortech.2016.06.039
Ji, M., Li, J., and Zhang, M. (2024). What drives the agricultural carbon emissions for low-carbon transition? Evidence from China. Environ. Impact Assess. Rev. 105, 107440. doi:10.1016/j.eiar.2024.107440
Junge, Y., and Qinmei, W. (2023). Digital technology and Quality development in agriculture-a digital productivity perspective. J. Shanxi Univ. Finance Econ. 45 (04), 47–63. doi:10.13781/j.cnki.1007-9556.2023.04.004
Kindler, R., Siemens, J. A. N., Kaiser, K., Walmsley, D. C., Bernhofer, C., Buchmann, N., et al. (2011). Dissolved carbon leaching from soil is a crucial component of the net ecosystem carbon balance. Glob. Change Biol. 17 (2), 1167–1185. doi:10.1111/j.1365-2486.2010.02282.x
Lal, R. (2003). Soil erosion and the global carbon budget. Environ. Int. 29 (4), 437–450. doi:10.1016/s0160-4120(02)00192-7
Li, B., Zhang, J., and Li, H. (2011). Research on spatial-temporal characteristics and affecting factors decomposition of agricultural carbon emission in China. China Pollut. Resour. Environ. 8, 80–86. doi:10.3969/j.issn.1002-2104.2011.08.013
Li, G., Huang, Y., Peng, L., You, J., and Meng, A. (2024). Agricultural carbon reduction in China: the synergy effect of trade and technology on sustainable development. Environ. Res. 252, 119025. doi:10.1016/j.envres.2024.119025
Li, H. (2023). Digital inclusive finance, agricultural green technology innovation and agricultural carbon emissions: impact mechanism and empirical test. Plos one 18 (10), e0288072. doi:10.1371/journal.pone.0288072
Li, J., Ning, J., Song, J., and Chen, X. (2024). Analysis of the spatial mismatch pattern of net carbon in agriculture and its influencing factors. Environ. Impact Assess. Rev. 106, 107522. doi:10.1016/j.eiar.2024.107522
Linghui, L., and Yongxin, Z. (2022). Digital villages help rural revitalization: internal mechanism and empirical test. World Agric. 8, 51–65. doi:10.13856/j.cn11-1097/s.2022.08.005
Liu, L., Wang, G., and Song, K. (2022). Exploring the role of digital inclusive finance in agricultural carbon emissions reduction in China: insights from a two-way fixed-effects model. Front. Environ. Econ. 1, 1012346. doi:10.3389/frevc.2022.1012346
Liu, L., Zhang, L., Li, B., Wang, Y., and Wang, M. (2024). Can financial agglomeration curb carbon emissions reduction from agricultural sector in China? Analyzing the role of industrial structure and digital finance. J. Clean. Prod. 440, 140862. doi:10.1016/j.jclepro.2024.140862
Ma, S., Li, J., and Wei, W. (2022). The carbon emission reduction effect of digital agriculture in China. Environ. Sci. Pollut. Res., 1–18. doi:10.1007/s11356-022-24404-8
Mary George, N., Parida, V., Lahti, T., and Wincent, J. (2016). A systematic literature review of entrepreneurial opportunity recognition: insights on influencing factors. Int. Entrepreneursh. Manag. J. 12, 309–350. doi:10.1007/s11365-014-0347-y
Ping, L., Ruishi, H., and Chang, L. (2024). The influence mechanism and effect of digital rural construction to empower agricultural economic resilience. Statistics Decis. 40 (02), 11–17. doi:10.13546/j.cnki.tjyjc.2024.02.002
Popescu, M. F., Chiripuci, B. C., Orindaru, A., Constantin, M., and Scrieciu, A. (2020). Fostering sustainable development through shifting toward rural areas and digitalization—the case of Romanian universities. Sustainability 12 (10), 4020. doi:10.3390/su12104020
Qing, W., and Yuhang, X. (2022). Has urban construction land achieved low-carbon sustainable development? A case study of north China plain, China. Sustainability 14 (15), 9434. doi:10.3390/su14159434
Rolandi, S., Brunori, G., Bacco, M., and Scotti, I. (2021). The digitalization of agriculture and rural areas: towards a taxonomy of the impacts. Sustainability 13 (9), 5172. doi:10.3390/su13095172
Rotz, S., Gravely, E., Mosby, I., Duncan, E., Finnis, E., Horgan, M., et al. (2019). Automated pastures and the digital divide: how agricultural technologies are shaping labour and rural communities. J. Rural Stud. 68, 112–122. doi:10.1016/j.jrurstud.2019.01.023
Song, Z., Wang, C., and Bergmann, L. (2020). China’s prefectural digital divide: spatial analysis and multivariate determinants of ICT diffusion. Int. J. Inf. Manag. 52, 102072. doi:10.1016/j.ijinfomgt.2020.102072
Sperow, M. (2020). Updated potential soil carbon sequestration rates on US agricultural land based on the 2019 IPCC guidelines. Soil Tillage Res. 204, 104719. doi:10.1016/j.still.2020.104719
Wang, H., and Qiu, T. (2024). The effects of farmer cooperatives on agricultural carbon emissions reduction: evidence from rural China. J. Clean. Prod. 450, 141881. doi:10.1016/j.jclepro.2024.141881
Wang, J., Yang, G., and Zhou, C. (2024). Does internet use promote agricultural green development? Evidence from China. Int. Rev. Econ. and Finance 93, 98–111. doi:10.1016/j.iref.2024.03.009
Wang, R., Chen, J., Li, Z., Bai, W., and Deng, X. (2023). Factors analysis for the decoupling of grain production and carbon emissions from crop planting in China: a discussion on the regulating effects of planting scale and technological progress. Environ. Impact Assess. Rev. 103, 107249. doi:10.1016/j.eiar.2023.107249
Wei, S., Yang, Y., and Xu, Y. (2023). Regional development, agricultural industrial upgrading and carbon emissions: what is the role of fiscal expenditure? Evidence from Northeast China. Econ. Analysis Policy 80, 1858–1871. doi:10.1016/j.eap.2023.11.016
Wei, Z., Wei, K., Liu, J., and Zhou, Y. (2023). The relationship between agricultural and animal husbandry economic development and carbon emissions in Henan Province, the analysis of factors affecting carbon emissions, and carbon emissions prediction. Mar. Pollut. Bull. 193, 115134. doi:10.1016/j.marpolbul.2023.115134
Wen, Z., and Ye, B. (2014). Analyses of mediating effects: the development of methods and models. Adv. Psychol. Sci. 22 (5), 731. doi:10.3724/sp.j.1042.2014.00731
West, T. O., and Marland, G. (2002). A synthesis of carbon sequestration, carbon emissions, and net carbon flux in agriculture: comparing tillage practices in the United States. Agric. Ecosyst. Environ. 91, 217–232. doi:10.1016/s0167-8809(01)00233-x
Woomer, P. L., Tieszen, L. L., Tappan, G., Touré, A., and Sall, M. (2004). Land use change and terrestrial carbon stocks in Senegal. J. Arid Environ. 59 (3), 625–642. doi:10.1016/j.jaridenv.2004.03.025
Wu, F., Li, L., Zhang, H., and Chen, F. (2007). Effects of conservation tillage on net carbon flux from farmland ecosystems. Chin. J. Ecol. 12, 2035–2039.
Xing, Z., Zhao, S., and Wang, D. (2023). Performance and sustainability evaluation of rural digitalization and its driving mechanism: evidence from Hunan province of China. Front. Environ. Sci. 11. doi:10.3389/fenvs.2023.1326592
Xu, B., and Lin, B. (2017). Factors affecting CO2 emissions in China’s agriculture sector: evidence from geographically weighted regression model. Energy Policy 104, 404–414. doi:10.1016/j.enpol.2017.02.011
Xueqiang, J., Huimin, L., and Yuesong, Z. (2023). Study on the influence of rural land transfer on agricultural carbon emission intensity and its mechanism in China. China Land Sci. 37 (2), 51–61. doi:10.11994/zgtdkx.20230208.093642
Zhang, L., Chen, J., Dinis, F., Wei, S., and Cai, C. (2022). Decoupling effect, driving factors and prediction analysis of agricultural carbon emission reduction and product supply guarantee in China. Sustainability 14 (24), 16725. doi:10.3390/su142416725
Zhang, P., Li, W., Zhao, K., Zhao, Y., Chen, H., and Zhao, S. (2023). The impact factors and management policy of digital village development: a case study of gansu province, China. Land 12 (3), 616. doi:10.3390/land12030616
Zhang, X., Sun, H., Xia, X., Yang, Z., and Zhu, S. (2024). Can a crop rotation and fallow system reduce the carbon emission intensity of agriculture? Land 13 (3), 293. doi:10.3390/land13030293
Zhao, L., Rao, X., and Lin, Q. (2023). Study of the impact of digitization on the carbon emission intensity of agricultural production in China. Sci. Total Environ. 903, 166544. doi:10.1016/j.scitotenv.2023.166544
Zhenshuang, W., Yanxin, Z., Ning, Z., Wang, T., and Zhang, Z. (2022). Spatial correlation network and driving effect of carbon emission intensity in China’s construction industry. Buildings 12 (2), 201. doi:10.3390/buildings12020201
Zhi, J., and Gao, J. (2009). Analysis of carbon emission caused by food consumption in urban and rural inhabitants in China. Prog. Geogr. 3, 429–434. doi:10.11820/dlkxjz.2009.03.016
Keywords: digital rural construction, agricultural carbon emission intensity, human capital, financial support for agriculture, impact
Citation: Li W, Guo J, Tang Y and Zhang P (2024) The impact of digital rural construction on agricultural carbon emission intensity. Front. Environ. Sci. 12:1492454. doi: 10.3389/fenvs.2024.1492454
Received: 06 September 2024; Accepted: 11 November 2024;
Published: 22 November 2024.
Edited by:
Jiachao Peng, Wuhan Institute of Technology, ChinaReviewed by:
Dehua Shen, Nankai University, ChinaCopyright © 2024 Li, Guo, Tang and Zhang. This is an open-access article distributed under the terms of the Creative Commons Attribution License (CC BY). The use, distribution or reproduction in other forums is permitted, provided the original author(s) and the copyright owner(s) are credited and that the original publication in this journal is cited, in accordance with accepted academic practice. No use, distribution or reproduction is permitted which does not comply with these terms.
*Correspondence: Jinyong Guo, MTM0NzkxNzUwOTBAMTYzLmNvbQ==
Disclaimer: All claims expressed in this article are solely those of the authors and do not necessarily represent those of their affiliated organizations, or those of the publisher, the editors and the reviewers. Any product that may be evaluated in this article or claim that may be made by its manufacturer is not guaranteed or endorsed by the publisher.
Research integrity at Frontiers
Learn more about the work of our research integrity team to safeguard the quality of each article we publish.