- 1School of Statistics and Data Science, Lanzhou University of Finance and Economics, Lanzhou, China
- 2Economic Research Institute of the Belt and Road Initiative, Lanzhou University of Finance and Economics, Lanzhou, China
Introduction: To achieve higher levels of development in China, building an environmentally friendly, low-carbon economic system is crucial, and policymaking plays a key role in this. Low-carbon and innovative cities pilot were launched in 2010 and 2008, respectively, with the range of pilot cities continuing to expand to date. This study aimed to calculating carbon emission efficiency and exploration the impact of dual pilot low carbon cities and innovative cities on carbon emission efficiency.
Methods: Using a multi-period DID model, this study analyses data from 284 prefecture-level cities in China between 2006 and 2020. The Super-SBM model with undesired output is applied to calculate urban carbon emission efficiency.
Results: The study performs a sequence of robustness tests; it is still found that the dual-pilot policy has a positive promotion effect on urban carbon emission efficiency. Heterogeneity analysis indicates that the effect of dual-pilot is more significant in the central and western regions, with notable differences observed regardless of city size. The impact mechanism analysis finds that the dual-pilot policy boosts the rate by enhancing green innovation in general. The synergy analysis found that the efficiency improvement effect of the dual-pilot on carbon emissions would be more significant than that of the single-pilot policy, indicating that the dual-pilot policy has a synergistic effect and is still significant two years after either the single-pilot or the dual-pilot.
Discussion: The findings indicate that the dual-pilot policy has a better capacity to reduce carbon emission and improve its efficiency, which can work to achieve green development. Nevertheless, the limitation is that it fails to reflect spatial differences, and the relevant research will be further strengthened.
1 Introduction
This study focuses on China’s commitment to green innovation and modern high-quality development, prioritizing a “people-oriented” approach and actively working toward establishing a global community with a shared destiny. China has taken proactive steps to propose and implement various targets as well as initiatives aimed at reducing its emissions. The 2022 Annual Report on China’s Policies and Actions to Respond Climate Change suggests a new deployment plan that includes top-level design, medium- and long-term greenhouse gas emission strategies, and establishing a comprehensive national strategy to adapt to climate change. Simultaneously, it is recommended that the industrial structure be adjusted, the growth of “two high, one low” projects be regulated, and clean energy be applied to improve quality and energy efficiency so that pilot demonstrations are advanced. The innovative pilot project began with the development of an innovative city in Shenzhen and later expanded to include 62 cities from the initial 16. The significance of low-carbon growth in China’s socioeconomic progress is growing.
The current literature mostly discusses low-carbon city pilots in relation to economic and environmental aspects, including innovative carbon reduction policy (Song Q. et al., 2021), enhancing energy efficiency (Chen and Wang, 2022), and stimulating economic growth (Liang et al., 2023). Green efficiency is assessed using various methods to create a “siphon effect” and energy efficiency generating a positive spatial spillover effect (Fang et al., 2024). The impact of carbon reduction is analyzed in terms of production, residents, and government administration to provide recommendations for enhancing economic growth at the micro, meso, and macro levels. It aims to connect people’s ways of making a living and local governments using low-carbon pilot policies. This involves considering available resources and the unique characteristics of those in charge of implementing policies in government (Zhao and Wang, 2021), as well as the level of environmental governance (Zeng J. et al., 2023). The focus is on factors such as employment (Chen et al., 2021) and individual consumption (Wang K. L. et al., 2022) in support of development. The impact of policy implementation is affected by personal characteristics known as the “rule of man.” Improvements in the quality of labor and employment and incentives for green consumption indicate that developing primary and tertiary industries through industrial transformation can help achieve a low-carbon effect. Eventually, the literature indicates that innovation is fundamental to reducing emissions and saving efficiency by applying technological innovation in enterprise production (Yang, 2023), establishing a conducive atmosphere for fostering creativity, and disclosing environmental information in a timely manner (Pan et al., 2022). This adheres to the innovation-led approach of green development.
Current research on innovative cities focuses on three main aspects. (1) Innovative cities focus on several industries to encompass both services and manufacturing. On the one hand, social network analysis and super-efficiency DEA are utilized to assess R&D outcomes and social change and to investigate the influence of industry innovation (Zeng S. et al., 2023). Research has also examined in-depth the spatial spillover effect, focusing on the “demonstration effect” of urban innovation—the radiation impact on surrounding cities (Gao and Yuan, 2022). (2) The mechanism study of innovative city formation, joint knowledge spillover effect, and government support for innovative policy will link market externalities and government activism (He et al., 2023) from green innovation technology (Jiang et al., 2023), enhance the aggregation of city formation factors (Li and Wang, 2020) and promote industrial upgrading (Zhou et al., 2023) to explore the construction and development of innovative cities. (3) The “Indicator System for Building Innovative Cities” of 2016 establishes key indicators for evaluating innovative cities in China. It emphasizes enhancement of the economy, boosting innovation capacity, and creating a favorable innovative environment. Xu (2024) contends that urban innovation serves as a catalyst for economic growth and may be utilized to address pollution issues and enhance public awareness of environmental conservation. Zhang et al. (2023) argue that the strategy has effectively reduced the financialization of tangible firms. They suggest that a negative management outlook, unfavorable regional business conditions, and decreasing company activity have intensified the shift to being “out of touch with reality and in favor of emptiness.”
There has been extensive research on low-carbon cities and innovative cities individually, but less attention has been given to the impact of the two policies combined. Their combination has a significant impact on improving the efficiency of reducing carbon emissions (Zhang and Fan, 2023). There has been little research on integrated low-carbon city experiments with innovative city experiments. Zhang and Zheng (2023) proposed that sustainable living can be advanced by improving energy structures rather than solely relying on eco-friendly production. Further research is needed on the merging of the two types of city pilot. Initially, there has been more research on single policies compared to dual or multiple policies, with less emphasis on the interactions between policies. In addition, research on factors that affect carbon emission efficiency has concentrated mainly on industrial structure, economic growth, available resources, and innovative development, with few studies considering policy factors.
Therefore, this paper examines the impact of a dual pilot program involving low-carbon and innovative cities on urban carbon-emission efficiency using a multi-period difference-in-difference model. Urban carbon emission efficiency is assessed through the super-SBM model to solve specific problems with undesired output. In low-carbon and innovative cities, do dual pilot projects effectively reduce emissions and increase efficiency? If the answer is affirmative, how does the existence of the two pilots affect carbon emission efficiency? Is there variation in the effect? Do dual pilots enhance cities’ carbon efficiency more than single pilots? Is there a synergistic impact? Possible contributions to such questions may include the following. Initially, the research topic focuses on how a dual pilot of low-carbon and innovative cities affects the efficiency of urban carbon emissions. At present, only a limited body of research has examined the impacts of the dual-pilot strategy. This study aims to enhance and expand evaluation of the policies regarding the development of green, low-carbon, creative Chinese cities. The estimation process also employed several robustness checks to guarantee the soundness of the conclusions, including a multi-period difference-in-differences model, a super-efficient SBM model of undesirable output, a PSM-DID model, a parallel trend test, a placebo test, and quantile regression and counterfactual tests. Eventually, after considering spatio-temporal evolution as a characteristic of efficiency, this paper adopts a multi-period DID estimation framework to analyze the evolution mechanism of efficiency. In order to explore the synergies of the pilots in more depth, it also incorporates regional heterogeneity and considers the process of green innovation and industrial structure analysis on efficiency.
2 Institutional background and research hypotheses
2.1 Institutional background
China’s economy is currently grappling with balancing carbon emissions and economic growth during a transitional phase. Developing a low-carbon economy is paramount for attaining green development by effectively utilizing ecological resources, promoting responsibility and protection in actions, and meeting energy-saving targets. China has already established the Three Rings Low Carbon City Pilot Zone, and as part of its low-carbon economy practice, it will follow the principle of policy innovation and diffusion gradually and progressively (Zhang et al., 2022). In 2009, the low-carbon initiative set the goal of minimizing greenhouse gas emissions by developing low-carbon industrial systems and consumption habits. In 2010, China selected a group of eight cities in five provinces to serve as low-carbon pilot cities with the aim of promoting the growth of low-carbon industries and urban areas while reducing reliance on fossil fuels. This initiative aimed to engage local governments in low-carbon initiatives. The second phase of the pilot program for low carbon cities began in late 2012. Currently, with the exception of Hunan, Ningxia, Tibet, and Qinghai, at least one city in every province, municipality, and autonomous region in the country is participating in the low-carbon city pilot.
Local governments respond positively to development goals, leading to the sequential pilot deployment of innovation-oriented cities. Clear criteria exist for identifying innovative cities based on factors such as innovation investment, advancements in science and technology, and dependence on external technology. In 2008, the regional innovation system was successfully established with the approval of Shenzhen as a national pilot innovative city. Immediately before 2010, 16 cities including Dalian and 20 cities (districts) including Haidian District in Beijing were chosen as pilot cities. The third batch of 61 pilot districts was released in a detailed list; in April 2018, the National Development and Reform Commission (NDRC) confirmed 17 cities, including Jilin Province, as a new type of city experimental zone. Taking the lead in creating low-carbon and innovative cities will have a significant impact on the country’s innovation strategy to promote economic growth and environmental protection.
2.2 Research hypothesis
Environmental regulation is very important to the growth of a green economy, as implementing suitable regulatory measures can provide a fresh boost to its development and enhance carbon emission efficiency. Its successful implementation depends on the establishment of a cooperative structure that links central and local governments. From the perspective of pilot-city construction, local governments promote the realization of this development through three kinds of environmental governance policy tools: command and control tools through mandatory intervention to set strict emission reduction targets and technical standards; front-loading environmental protection to transform traditional industries to promote innovation and upgrading; reducing environmental emissions to enhance emission efficiency (Xu and Cui, 2020). In contrast, market-incentivized policy tools are biased toward autonomous choice through fully mobilizing the role of the market to guide enterprises to use environmental taxes, carbon emissions trading, subsidized low-interest loans, and other forms of low-carbon technological innovation (Yang et al., 2020). When trying to achieve a city’s environmental goals, public participation policy tools primarily involve advocating for universal supervision and involvement and aggressively promoting the low-carbon ideal (Zhang et al., 2021). As the implementation of a growing set of environmental laws and regulations increases, the spread of low-carbon ideals will help reduce urban carbon emissions and ultimately achieve efficiency.
Dual-pilot cities have more significant emission reductions and efficiency gains than single-pilot cities. Low-carbon pilot cities will reduce their emissions by implementing policies that can help them become more innovative. On the one hand, in pursuit of enhanced financial returns and in accordance with the tenet of “cost effectiveness,” they will reallocate the expenses incurred in technological research and development toward managing emissions. However, this reallocation of funds has resulted in a decline in carbon emissions efficiency due to accumulated expenditure on human labor and material resources during their transitional (Guo and Liang, 2022). On the other hand, cities that are innovative serve as a catalyst for new ideas and advances. By implementing policies that foster innovation, cities can enhance their performance in reducing carbon emissions (Yu et al., 2022). Technological breakthroughs resulting from this will lead to more environmentally friendly production and reduced energy use. These two effects coupled with low carbon policies have a dampening effect on carbon emissions, indicating a synergy between the two, where dual-pilots are more effective than single pilots in increasing carbon emission efficiency. The following hypotheses are thus proposed.
Hypothesis 1. The dual-pilot construction of low carbon and innovative cities makes a significant contribution to improving urban carbon emissions efficiency.
Hypothesis 2. The dual pilot of low-carbon and innovative cities is more effective than the construction of single-pilot cities in improving urban carbon emission efficiency.
Dual-pilot low-carbon and innovative cities are designed to face the challenge of climate change and “…build a high-level science and technology innovation center to attract high-end talent and innovation resources.” In a context of political limitations, dual-pilot cities will attract more clean-energy enterprises to move in and invest while increasing incentives for local businesses to invent, adapt, and upgrade technology for use in both production and daily life (Shang et al., 2023). The level of green innovation will reduce carbon emissions by supporting more technological development (Dong and Wang, 2024). This is because reduced carbon emissions can reduce economic losses and increase efficiency through environmental supervision and management. In terms of scale and technology, direct carbon emission reductions are induced through scale effects, and technological innovations indirectly cause reductions in production costs and thus promote energy transformation, further achieving carbon reduction targets (Fang et al., 2023). Environmental control helps the technological capability of advanced innovation, where the constraints on carbon emissions considerably increase the cost of production for polluting enterprises, motivating them to further transform and upgrade. The new technology will then reduce production costs (Porter and van der Linde, 1995). In pursuit of these overarching objectives of energy conservation, economic expansion, and scientific, technological, and innovative (STI) advancement, the dual pilot program serves as a catalyst for fostering a dual development paradigm that simultaneously emphasizes low-carbon practices and innovative endeavor. This approach is designed to harmonize environmental sustainability with economic growth, leveraging technological advancements to mitigate carbon emissions while simultaneously driving economic expansion and technological innovation. Strong human capital in science and technology innovation provides favorable competitiveness for technological development, and the scientific research results and invention patents formed by its research all provide a strong impetus for urban development. Innovative technology thus inhibits urban carbon emissions from technological progress (Si et al., 2023).
Hypothesis 3. The dual pilot construction of low carbon and innovative cities improves the efficiency of urban carbon emissions by increasing levels of green innovation in cities.
The assessment of the industrial structure is the primary element that is impacting carbon emission efficiency. For the purposes of reducing emissions and boosting efficiency, the government has established a low-emissions system with macro-controlled taxes, subsidies, and other policies. However, because of many cities’ abundant natural resources and more advanced mining sectors, the accelerated use of non-renewable energy will contribute to increasingly severe urban environmental degradation (Fang et al., 2022) and pollution. In order to build a clean industrial system and develop new industries, as well as to encourage the development of conventional industrial technology and management concepts, it is necessary to create an advanced industrial structure by gradually raising the bar for research and development, expediting the practical conversion of scientific findings to realize the power-driven transformation to low carbon. Improving production efficiency and reducing the weight of high-carbon industries will help upgrade industrial infrastructure, thereby lowering carbon emissions (Zheng et al., 2021). It is secondary industries that are the principal application for energy consumption, with less demand from the primary and tertiary sectors. By comprehensively considering the double effect of policy constraints and industrial structure advancement, efficiency will be enhanced if, following the dual pilot’s implementation, the share of secondary industry in the advancement of industrial structure is optimized.
Hypothesis 4. The dual pilot construction of low carbon and innovative cities improves the efficiency of urban carbon emission by optimizing the industrial infrastructure.
3 Research design and sample explanation
3.1 Modeling setting
This study investigates the effects of the low-carbon and innovative cities pilot program on the carbon efficiency of carbon emissions. The analysis takes into account the varied pilot times, selection criteria for pilot cities, and potential influence of unobservable characteristics on policy implementation. This study carefully addressed the complex issues surrounding endogeneity and meticulously dissected the intricate relationships that can potentially confuse the analysis. By employing meticulous empirical techniques, the research effectively distinguished the influence of policy interventions on carbon emission efficiency, revealing a noteworthy correlation between the two. The model aims to isolate the impact of the dual pilot program by comparing carbon emission efficiency before and after its implementation while controlling for factors such as the non-random selection of pilot cities and unobservable variables. Thus, this study can accurately measure the influence of the dual testing policy on efficiency. The effect of having two pilots on efficiency is further analyzed. The multi-period difference-in-difference model (1) is defined as follows:
in which
3.2 Measurement of urban carbon emission efficiency
The primary driver of long-term economic growth is the increase in factor inputs and the enhancement of factor productivity. Therefore, a low-carbon footprint is seen as having fewer inputs and more desired outputs while also minimizing non-desired outputs. The radial efficiency measure aims to achieve target efficiency by increasing outputs and decreasing inputs equally. However, it has limitations such as overestimating actual efficiency, the inability to distinguish the efficiency of individual factors, and primarily emphasizing the quantification of green total-factor productivity. Consequently, the research incorporates efficiency measurement while considering carbon dioxide emissions. The SBM model is used to calculate carbon emission efficiency (Yang et al., 2021). Identifying the variables in Table 1 as the SBM model can yield efficiency values greater than 1, potentially impacting study accuracy. Tone (2002) proposed the highly efficient SBM model with undesirable output, which is used in this paper to boost its relevance. The calculation formula is shown below.
3.3 Variable settings
3.3.1 Variable being explained
3.3.2 Core explanatory variables
Whether or not a city serves as a pilot for both low carbon cities and creative cities with two pilots
3.3.3 Control variable
As seen in Table 2 below, five control variables were chosen.
3.3.4 Mechanism analysis
In accordance with Hypotheses 2 and 3, two mediator variables—the level of green innovation
3.4 Data
We analyzed panel data from 284 prefecture-level cities in China for 2006–2020. The sample excluded Hong Kong, Macao, and Taiwan. Additionally, cities in Tibet were excluded due to incomplete data, discrepancies in data from cities with changes in administrative levels, and difficulties in acquiring data for certain cities. Urban carbon emission figures are based on accounting by Wu and Guo (2016). The statistics were obtained from publications such as the China Urban Statistical Yearbook, China Urban Construction Statistical Yearbook, China Regional Statistical Yearbook, China Research Statistics Service Platform (CNRDS), China Energy Statistical Yearbook, and the China Economic Statistical Database. Interpolation was utilized to compensate for discrepancies in data from cities in Tibet, and to address localized missing data.
Low-carbon city pilots represented 52.15% in eastern cities1, 27.06% in western cities2, and 20.79% in central cities3. Innovative city pilots accounted for 56.35% in mid-eastern regions, 20.38% in western cities, and 23.27% in central cities. The proportion of dual-pilot cities in eastern, western, and central cities was 55.56%, 24.44%, and 20%, respectively. The proportion of low-carbon cities, innovative cities and cities in the east, west, and middle of the double pilot cities is about 2:1:1, which is flat, indicating that most of the policy implementation tends to be located in areas with better initial economic development. Although the pilot cities accounted for a small proportion, they might indirectly achieve carbon emission reduction through policy, rational resource allocation, and experienced sharing. The data’s statistical properties are presented in Table 3.
4 Empirical results and robustness test
4.1 Characterizing the time-series evolution of urban carbon emission efficiency
The fundamental focus of this study is to examine the impact of urban carbon emission efficiency as an explanatory variable in the dual pilot program. Additionally, understanding the temporal development patterns of the past 15 years is crucial.
Figure 1 shows carbon emission efficiencies at both sub-regional and total levels from 2006 to 202, as calculated by the model. Over the course of 15 years, its efficiency has shown a steady pattern of development over time. Cities in the eastern, central, and western regions have carbon emission efficiencies that are in line with general national trends. Efficiency is highest in the eastern region, steadily increasing since 2006. Western cities have the lowest efficiency with more fluctuations. Central regions have efficiency similar to the national average. In 2017, there was a notable decrease in efficiency in the central area, likely caused by increased energy demand. This led to a slight recovery in efficiency, although the effect was not substantial, showing a decrease of 4.16%. The disparity in efficiency ratings among areas in China decreased notably 2008–2017 but has progressively increased since 2017. The curve’s trend may be categorized into two stages. Urban carbon efficiency averaged roughly 0.5 during the steady development stage from 2006 to 2016. Having a mean yearly expansion rate of 7.03%, efficiency grew from 0.56 to 0.65 throughout the growth and development period from 2017 to 2020. This development was quick but exhibited changing patterns. China’s economy transitioned to high-quality development by optimizing its industrial and energy structure and deepening pilot demonstrations. Generally, there is an obvious upward trend in the overall efforts of regions to reduce carbon emissions, and the effectiveness of these efforts is consistently improving. However, cities in middle and western regions of the country still need to narrow the gap with cities in the eastern part.
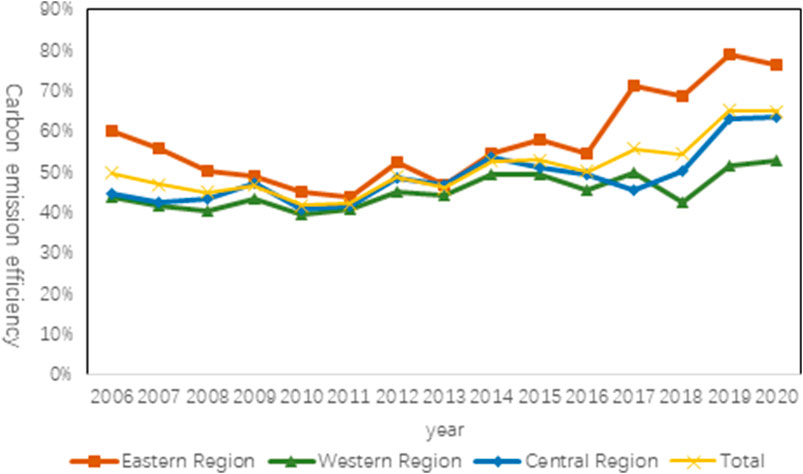
Figure 1. Characteristics of the time-series evolution of urban carbon emission efficiency in different regions.
4.2 Baseline regression
Table 4 displays the regression findings regarding the effect of the dual pilot strategy on efficiency. Model (1) is a double fixed-effect model without control variables, considering the impact of the year and the city. Model (2) includes control variables in a two-way fixed effect model. Models (3)–(5) are double fixed-effect models with a lag of one to three periods on the core explanatory variables. Model (6) is a regression model that assumes no dual-pilot policy implementation for dual-pilot cities starting from 2010, with the second group of pilot cities set as 2012, and it assumes no dual-pilot policy implementation for dual-pilot cities thereafter.
As the results indicate, when the model includes the double fixed effect of year and city, irrespective of whether the control variables are included or primary explanatory variables are lag-dependent, the impact of the double-pilot policy on urban carbon emission efficiency is consistently positive. This shows that the implementation of the dual-pilot program has a noticeable impact on efficiency, which further confirms the expected role of the city’s double pilot to reduce carbon emissions, verifying Hypothesis 1. In the meantime, the significant explanatory variables are those lagged for one to three periods, signifying a delay in the impact of the program. The coefficients of the models incorporating control factors exhibit a substantial decrease, thus affirming the effectiveness and legitimacy of the control variables. In controlling for variables, the regression coefficients for population density and human capital were significantly positively correlated. An increase in population density has a scale effect on the effective use of resources and pollution emission treatment. Similarly, an increase in human capital indicates an increase in talent and labor force. Therefore, increasing population size and attracting high-skilled workers from all aspects of the city will provide a labor-force base for achieving low-carbon and innovation. The government’s macro-control level is significantly negative, indicating that there is now excessive policy intervention, resulting in the local financial expenditure ratio increasing but with smaller impact on efficiency. In this study, evaluating the effects of economic progress, FDI share, and scientific and technological development on urban carbon emissions is hard to judge. From model (6), it can be seen that dual-pilot cities were implemented from 2010 to 2020, and assumption 1 remains valid for the cities in the first two pilot batches. This means that the policy is generalizable, and the consequences are positive and meaningful for both the initial two rounds of the pilot cities and the subsequent cities that adopt the dual-pilot approach.
4.3 Parallel trend test and dynamic analysis
To assess the carbon emission efficiency trends between pilot and non-pilot cities before the program’s implementation, a parallel trend test is required for the multi-period DID model used in the dual-pilot program. Referring to the framework of McGavock (2021) and Song et al. (2019) and considering that the sample data spans 15 years and that the duration frame prior to and subsequent to the policy’s implementation is extensive, it was decided to tail process the model-related time virtual variables and construct the following model using the interaction terms of the dual-pilot policy dummy variables with 10 years, corresponding to before and after policy implementation. Within this framework, the initial 4 years following the execution of the program, along with the subsequent approximate 5 years after its enactment, were identified as critical moments for analysis.
From Figure 2, the regression coefficients pertaining to the dual-pilot program, when comparing the 4 years preceding its implementation to the subsequent 2 years post-implementation, failed to achieve significance in the tests, indicating that the comparison between the pilot cities and non-pilot cities during this period was passed using the parallel trend test. The evidence demonstrates that there is no discernible distinction in the trend of efficiency change between trial and non-trial cities in each year prior to the operation of the dual pilot program. From the dynamic effect, the regression coefficients of the dual-pilot policy did not pass the significance test in the 2 years after its implementation, but there is an upward tendency in efficiency compared with before its implementation. The coefficients at 5% significance level turned out to be significantly positive after the operation of the dual-pilot program for 3 years, suggesting that the impact of the dual pilot on improving carbon efficiency was delayed until year 3, which means that the policy effects has time lags issuance, implementation until the effectiveness, and the performance of the effect It takes time to accumulate. The regression coefficients indicate that the dual-pilot program has a progressively increasing effect on the efficiency of the city, starting with the third year of policy implementation. Overall, those results prove Hypothesis 1.
4.4 Robustness test
4.4.1 PSM-DID test
The PSM-DID test addressed the visible factors that influence the dual-pilot policy’s deployment using propensity score matching (PSM). Matching samples are acquired by a year-by-year comparison of pilot and non-pilot cities in order to consider individual variations. The overall impact of the dual-pilot project is then made apparent by applying the DID model to exclude the influence of time-varying and unobservable elements. The procedure involves measuring matching variables with six control variables, using a Logit model for regression, applying the caliper nearest-neighbor matching method based on propensity scores to match pilot with non-pilot cities, identifying propensity score values and cities without the policy as the control group, and then conducting regression on the matched samples using the DID method. The regression findings are displayed in Table 5 below. Regression results after fitting were compared with the results of Table 4 before matching. It was observed that the average causal influence of the dual-pilot project is positive. The distributions of Figure 3 below exhibit a high degree of similarity, as confirmed by passing the parallel trend test. This suggests that the conclusions are strong and reliable.
4.4.2 Placebo test
The double difference model passes the placebo test, which examines whether the dual pilot strategy impacts carbon emission efficiency or if other variables are responsible. This study employs a randomized treatment group selection procedure due to the use of short panel data. The coefficients on the “dummy policy variables” of the interaction terms randomized to the new setup are assumed to remain significant in the dummy scenario. In this scenario, it is implied that the initial estimations could be skewed and that alterations following the creation of the dual pilot may have been impacted by other variables. Conversely, an insignificant coefficient indicates a robust result. The initial step involved removing the provided text referring to the sample data of the treatment group obtained from the original source dataset one by one. Next, participants are randomized into different treatment groups and these groups are combined with the rest of the raw data set. Thirdly, regression analysis is conducted utilizing the randomized treatment group. After doing this 1,000 times, the resulting distribution of estimated coefficients is obtained.
The “dummy policy variables” estimates are derived from 1,000 simulations. The Figure 4 displays the coefficient distributions and T-statistics. The graph shows that the estimated coefficients and p-values are mostly clustered around the horizontal axis at 0, to the left of the original values. This suggests that the coefficients of the variables are not significant, so we can consider the results of the model to be robust.
4.4.3 Quantile regression test
This investigation employs panel quantile regression to calculate the model and analyze the influence of data distribution on the robustness of the results, in addition to the marginal effect of applying the dual-pilot strategy at distinctive quartiles (10%, 30%, 50%, 70%, and 90%) (Wen et al., 2022). The results from Table 6 suggest that as the effect of the dual-pilot strategy on efficiency steadily increases, the quantile regression coefficients of the dual-pilot strategy increases as the quantile increases. Except for the 10% quantile, which does not effectively represent the impact of the dual-pilot program on urban efficiency, the remaining quantiles show relevance. The dual-pilot strategy has a more significant effect on municipalities with high as opposed to those with low efficiency.
4.4.4 Additional robustness tests
Three additional robustness tests were conducted by the study based on the insights of the above tests. ① Subsample test: deleting the data processing group’s sample of first-tier cities—Beijing, Shanghai, Guangzhou, and Shenzhen—to exclude the possibility of influencing results because of various other factors, such as the economy. ② Constructing a counterfactual test like that of Qiu et al. (2021): take the cities before the construction of the dual-pilot in 2006–2009 as the research samples, set the dual-pilot time as 2007, and compare the counterfactual experiment with the baseline regression model for evaluation. ③ Excluding the influence of other policies: in the same time span, both the smart city pilot and the carbon trading pilot may have the effect of reducing carbon, so enhancing efficiency through the dual-pilot project may include the influence of other policies. To eliminate interference with other measures, the interaction terms of the smart city pilot program and the pilot time and the carbon trading pilot and the pilot time are added to the group of control variables. If the dual-pilot project remains substantial, this indicates that the policy is unaffected by other policies, and vice versa.
Table 5 displays the results. Column (2) controls for double-fixed effects after removing some of the samples and finds that the policy effect is still markedly positive, showing that whether dealing with a first-tier city or not does not have much effect on the implementation of the dual-pilot policy. Column (3) regresses DID2017 with and without control variables and finds that the coefficients are insignificant, suggesting that the disturbances do not explain the observed increase in efficiency and that the increase in efficiency is largely due to the policy itself. Column (4) after excluding the smart city and carbon trading pilot strategy—the dual-pilot program—continues to show positive, suggesting that the dual-pilot policy is not affected by other policies to improve urban carbon emission efficiency.
5 Influencing mechanism analysis
5.1 Heterogeneity analysis
5.1.1 Location heterogeneity analysis
The overall cities are grouped, and heterogeneity is further examined by looking at the regions in which different cities are located, the sizes of different cities, and their administrative levels. This prevents the possibility of the overall analysis hiding the possible differences between the dual pilot on the carbon emission efficiency of various cities and the differences between the geographic locations, city sizes, and administrative levels of the cities.
Regarding the geographical positioning of cities, China varies in its economic development due to geographical differences across eastern, central, and western regions. This study’s findings indicate that the implementation of the dual-pilot program had a substantial impact on improving carbon emission efficiency in the central and western regions. The estimated coefficients for the eastern regions are not significant, probably due to the complexity of the factors affecting the region, such as the high degree of economic outward mobility, excellent geographic location, and the inflow of highly skilled workers, meaning that a large number of resources are already fully utilized, so that the effects of the dual-pilot policy are not yet fully apparent. The effect of the dual-pilot project has been weakened by the geographical constraints of cities in the western region, with their economic strength and industrial base weaker than cities in the central region, making innovation more difficult.
5.1.2 Analysis of urban scale heterogeneity
Larger and smaller cities differ in terms of industrial structure, scientific and technological trends, and economic development due to disparities in the extent of urban development. Referring to the city classification criteria published by the State Council, we divided cities with a permanent population of 3 million or less into one type of city and cities with over 3 million permanent inhabitants into two types. Table 7 shows that the implementation of dual-pilot cities can markedly improve their carbon emission efficiency. Type Ⅰ cities improved their carbon emission efficiency more than type Ⅱ cities, but type Ⅱ cities have greater significance. This could be because type Ⅰ cities have a smaller population than type Ⅱ cities and their economies have developed more slowly, their governments have less macro control, and their limited current scientific and technological potential. Therefore, cities with initially low carbon emission efficiency can increase their extraction rate by implementing the dual-pilot policy. This will allow them to then implement carbon reduction measures and improve innovation, resulting in an enhanced extraction rate.
5.2 Influencing mechanism analysis
To gain insight into dual-pilot city construction on carbon emission efficiency, two recursive models are added to the multi-period DID model (1) to establish a mediating effect model.
where
5.2.1 The influence mechanism of the green innovation level
This paper illustrates the influence of the level of green innovation on the direction of the project on efficiency and explores whether there is any difference in the influence mechanism in terms of the overall, city size, and geographic location.
As shown in Table 8 above, the establishment of dual-pilot cities in Columns (1) and (2) generally has a virtuous relationship with green innovation at the 1% significance level. There is a significantly positive effect of the green innovation level on the carbon emission efficiency of the city, but at this time, project construction may not have a substantial impact on efficiency. This shows that the level of green innovation has a complete mediating effect on urban carbon emission efficiency—that is, the establishment of dual pilot cities is through the green innovation level and thus has a positive impact on urban efficiency. At the same time, the Sobel test yielded a p-value of 0, the data demonstrating the existence of a mediation effect, with the fraction of this effect accounting for 92.2% of the overall impact. In terms of city size, the impact of the level of green innovation within rows (3) and (4) of category 1 cities on efficiency is less pronounced, but the Sobel and bootstrap tests are both passed, signaling the presence of an ongoing mediating influence. There is also a full mediation effect in columns (5) and (6) for Type II cities, indicating that large-scale cities are subject to a larger mediation effect than small-scale cities and further indicating that the effect of the level of green innovation level on carbon emission efficiency is also heterogeneous. Regarding geography, there is also a full mediating effect in the eastern region, some intermediation effects exist in the central region, and no intermediation effects exist in the western region. The reasoning behind the implementation of this plan in the eastern region appears to be sound. The city boasts a robust economy and innovative capabilities, and green innovation can be achieved through a mutually beneficial approach of reducing emissions, enhancing efficiency, and generating economic growth. In the central region, the strength is marginally lower than the eastern region, but there is still a good environmental and innovation foundation, and the implementation of environmental regulating policies can be efficiently executed. In the western region, the dual-pilot policy exhibits a noteworthy and favorable influence on green innovation. Nevertheless, despite the apparent boost to green innovation, its subsequent effect on enhancing efficiency remains relatively muted, and both tests show that there is no intermediary effect. The impact is not significant, while both tests were not yield passed. However, most secondary industry is concentrated in the western region with its rich resources; thus, the pollution caused by activities such as production and extraction is difficult to improve only through policy and innovation but needs the influence of the concerned parties. It is apparent that the dual-pilot program can not only directly reduce carbon emissions and improve efficiency, but it can also advance urban green development by raising the bar for urban green innovation.
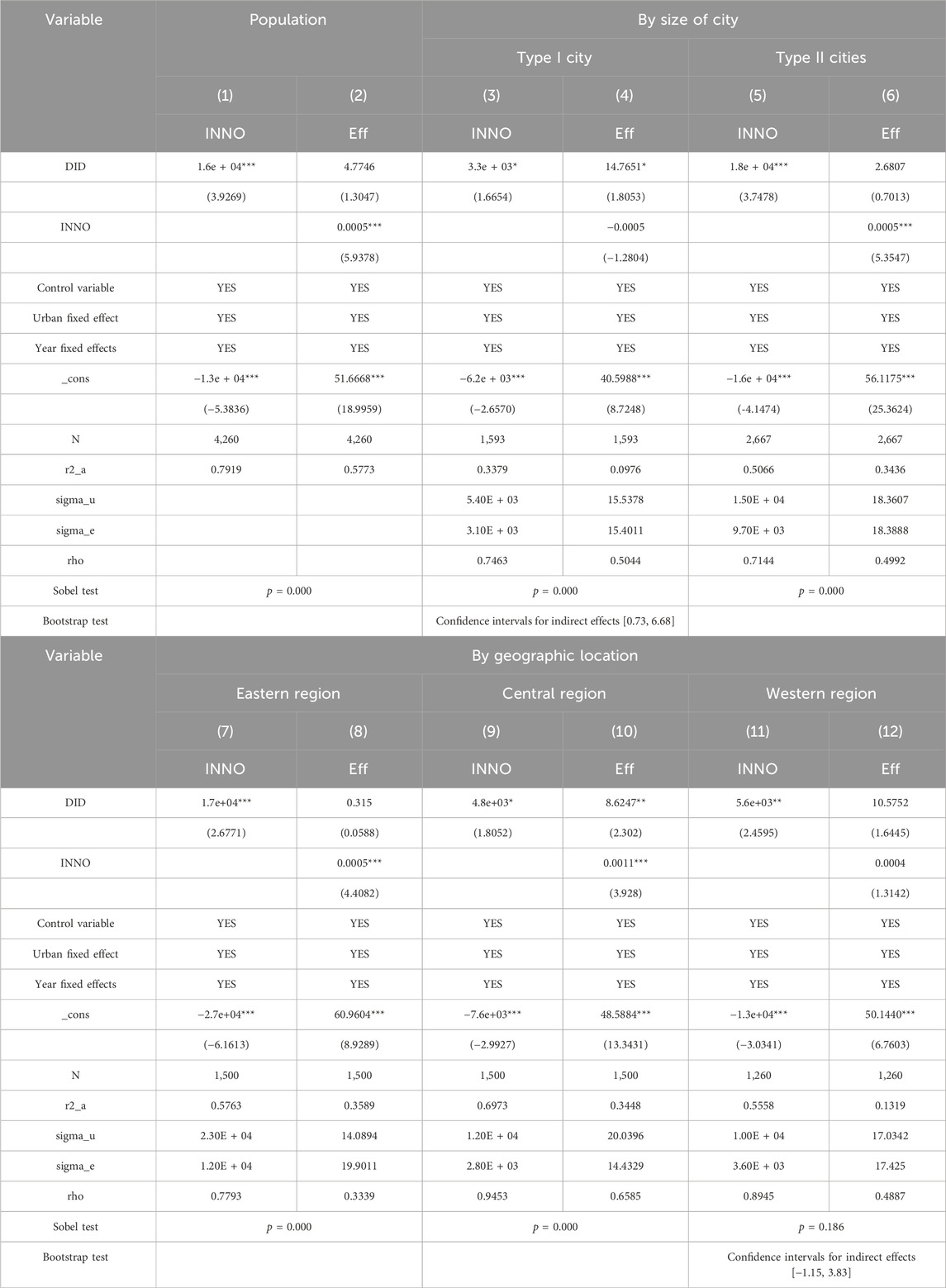
Table 8. Analysis results of the mechanisms influencing green innovation levels by general, city size, and geographic location.
In conclusion, this supports the effect on levels of green innovation by demonstrating the mediating role of green innovation and the differences that exist between city size in the eastern and central regions.
5.2.2 Analysis of the influence mechanism of industrial structure
From the data presented in columns (1) and (2) of Table 9 above, it can be observed that the implementation of the dual-pilot model in cities has a significantly negative impact on its industrial structure, but efficiency is not significantly influenced by the ratio from secondary industries. Meanwhile, the Sobel test p-value of 0.837 is not significant, and the bootstrap test found that the confidence interval for the indirect effect includes the value of 0. The statement may be interpreted as suggesting that there is no mediating effect. In consideration of the city scale, the influence of industrial structure on efficiency in columns (3) and (4) of type I cities is not significant, and both tests fail, indicating that there is no mediating effect. On the other hand, the coefficients in columns (5) and (6) of type II cities are all significant, and the optimization of industrial structure continues to play an intermediary role, suggesting the presence of a partial mediating effect—large-scale cities are more susceptible to the mediating effect than small-scale cities. It further indicates that secondary industry plays an indispensable role in type II cities, and the industrial scale effect on efficiency reveals heterogeneity at the city scale. With respect to geographic location, there is no mediating effect in all three regions, indicating that the mediating effect of industrial structure on efficiency does not have significant heterogeneity in terms of geography.
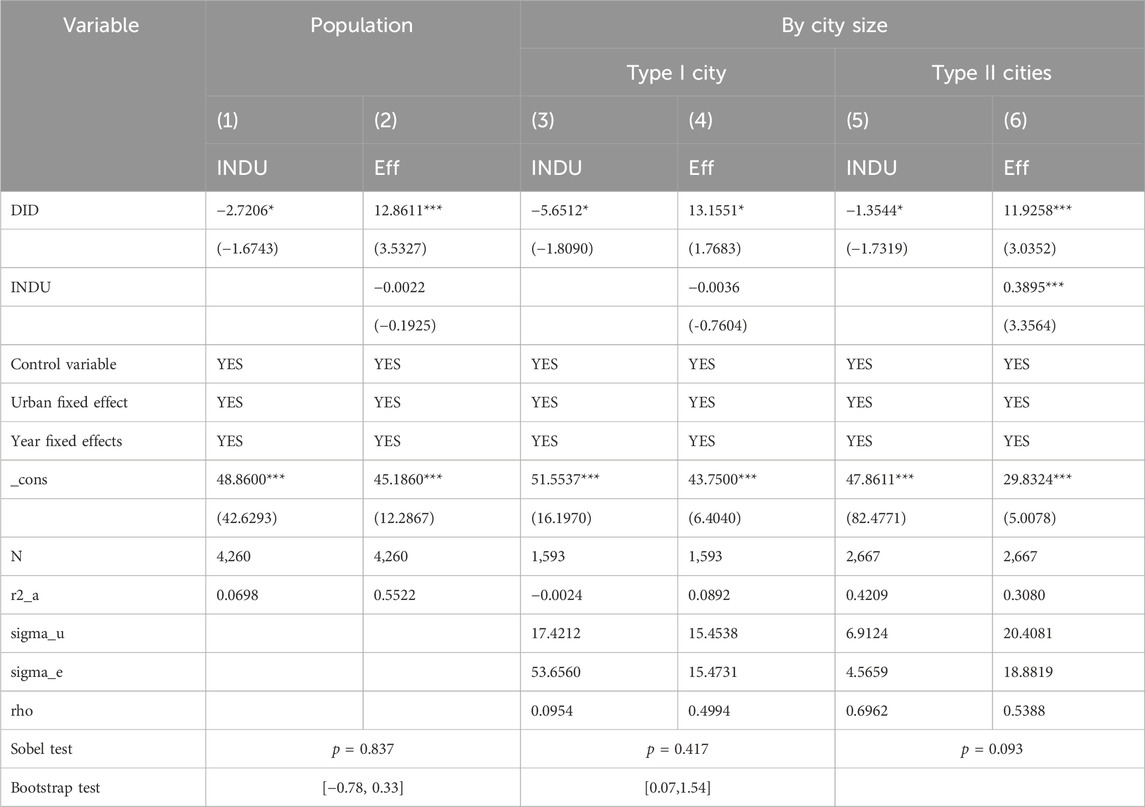
Table 9. Analysis of the mechanism of the impact of the industrial structure by general and urban scale analysis results.
In summary, this demonstrates that the industrial structure only has a mediating influence in type II cities, which in turn validates the mechanism of industrial structure effect.
5.3 Synergistic effect of dual pilot low carbon and innovative cities
Within the body of current literature, Guo and Liang (2022) and Yang and Shi (2023) have shown that the implementation of the pilot low-carbon city and pilot innovative city initiatives can enhance carbon emission efficiency and decrease carbon intensity, respectively. Thus, to promote a reduction in carbon emissions, this paper will further ascertain whether the implementation of the dual-pilot project is expected to yield greater effectiveness than the single-pilot approach, and thus determine whether the dual-pilot project will promote synergy.
We analyzed the influence of single-pilot (low-carbon) on the efficiency of carbon emissions in urban areas. The process is as follows. We excluded the sample of innovative cities but not the sample of dual-pilot cities, and we retained the single-pilot sample of low-carbon cities and the sample of non-pilot cities. Similarly, the influence of single-pilot (innovation) on efficiency was examined. The procedure can be outlined as follows. We omit the example of cities with minimal carbon emissions as well as the sample of dual-pilot cities, and we retain the single-pilot and non-pilot samples of innovative cities. As shown in Table 10, both cities with a single pilot can maximize efficiency, but the coefficients pertaining to its predictor variables of the low-carbon pilot project progressively diminish after the implementation of the policy, and cease to be statistically significant after a two-period lag, implying a limited durability in the effectiveness of the policy. Conversely, the individual pilot coefficients of the innovative cities are significant at the 1% level of significance in one and two lags, and the coefficients display an ascending magnitude, which indicates that the innovative cities’ single pilot has a certain degree of continuity in improving the efficiency of the city’s carbon emissions and lasts for a long time. Meanwhile, since the regression coefficients of innovative pilot cities are generally larger than those of low-carbon pilot cities, the efficiency improvement ability of innovative cities is higher than that of low-carbon cities. In comparison to the baseline regression, the regression coefficients of single-pilot cities are smaller than those of double-pilot cities, confirming Hypothesis 2, as it is evident that the double-pilot policy is superior to the single-pilot policy in enhancing carbon emission efficiency.
6 Conclusion and policy implications
This study analyzed data from 284 prefecture-level cities in China between 2006 and 2020 to study the impact of low-carbon and innovative dual-pilot cities on carbon emission efficiency. It utilized a multi-period DID model to investigate influence mechanisms and synergistic effects. (1) According to this study, the eastern region exhibits a higher level of carbon emission efficiency, and the central region exhibited parity with the national average, whereas the western region demonstrated the lowest values. (2) Additionally, implementing dual-pilot cities can effectively enhance carbon emission efficiency, with a time lag in policy effects. (3) Furthermore, dual-pilot cities show better and more enduring outcomes in enhancing carbon emission reduction than individual pilot policies. This suggests that innovation can facilitate environmentally friendly development and offer sustainable benefits, demonstrating its synergistic impact. (4) The analysis of heterogeneity demonstrates that the policy effect of the dual-pilot program is significantly more pronounced in the central and western regions, with differences in city scale. The carbon enhancement effect in eastern cities is insignificant. (5) Analysis of the impact mechanism reveals that the dual pilot policy largely improves carbon efficiency by fostering the development of green innovation. The mediating effect on large cities in the eastern and central regions is significant. Policy measures in Type II cities aim to enhance the efficiency of urban carbon emissions by optimizing industrial structure.
To encourage the development of dual-pilot cities and enhance urban carbon emission efficiency, the following policy proposals are offered.
(1) Enhance collaboration in building creative and low-carbon cities and progressively broaden the range of experimental initiatives. The combination of pilot policies creates a synergistic impact, and applying these policies together to improve carbon emission efficiency is more successful than executing a single pilot program. Promoting the dual-pilot policy tool is undeniably a beneficial step. In the future, successful experiences from western and central cities, as well as larger cities, will be collected and shared with other cities to enhance the policy impact of establishing dual-pilot cities.
(2) Adapting measures to local conditions and implementing strategies according to the situation, each with its own emphasis, strengthens the inclusiveness of policy implementation. Eastern cities have high carbon emission efficiency due to the implementation process. However, the policy effects in these cities are not as effective as in central and western cities, particularly in type I and II cities where urban transformation policies have been fully utilized. In the future, it is essential to enhance financial assistance for central and western cities while exploring low-carbon development strategies and carbon emission traits that are appropriate for the region in order to accelerate transition to a low-carbon city.
(3) Enhance funding for environmentally friendly technology development and consistently enhance innovation. Promote the recruitment of creative individuals and environmentally friendly businesses to ensure successful research and development as well as practical implementation of product technology. Local governments should boost their support for innovation and promote the active involvement of innovative entities, thereby achieving innovation-driven progress. Enhance the promotion and education of green innovation concepts to make green practices widespread and encourage individuals to adopt a green and low-carbon lifestyles. Enhance and strengthen the innovation market, enhance enthusiasm for innovation, and bolster faith in the ability to innovate.
(4) Encourage the improvement of industrial structure and leverage variations in city sizes. Develop low-carbon industries in large cities to improve energy utilization and resource efficiency. Develop complex strategies in research and technology, and build low-carbon industrial parks and clusters. Low-carbon and innovation-related policies should be applied to eliminate highly polluting and energy-intensive segments of industrial enterprises. In particular, it is recommended that government regulation be improved to optimize the production environment.
This paper has some limitations, as it only studied dual and single policies to achieve pollution reduction and efficiency improvement. It only considered policies under joint support, and whether there is still emissions reduction and efficiency improvement is not yet clear. In addition, it is still ambiguous whether the impact of relevant policies will geographically differ, and how big the difference is, which should be subject to further research.
Data availability statement
The raw data supporting the conclusions of this article will be made available by the authors, without undue reservation.
Author contributions
GD: funding acquisition, writing–original draft, and writing–review and editing. YW: data curation, writing–original draft, and writing–review and editing. JQ: writing–review and editing.
Funding
The authors declare that financial support was received for the research, authorship, and/or publication of this article. This work was supported by the Natural Science Foundation of China under the grant (number 72363021 and 12101279); Outstanding Youth Fund of Gansu Province (number 20JR5RA206); Longyuan Youth Talent Project (2022); Double First-Class Scientific Research Key Project of Gansu Provincial Department of Education (GSSYLXM-06); and the key scientific research project of the Silk Road Economic Research Institute of Lanzhou University of Finance and economics (JYYZ202102).
Conflict of interest
The authors declare that the research was conducted in the absence of any commercial or financial relationships that could be construed as a potential conflict of interest.
Publisher’s note
All claims expressed in this article are solely those of the authors and do not necessarily represent those of their affiliated organizations, or those of the publisher, the editors, and the reviewers. Any product that may be evaluated in this article, or claim that may be made by its manufacturer, is not guaranteed or endorsed by the publisher.
Footnotes
1Geographically, it includes the following provinces and municipalities where the prefecture-level cities are located. Eastern province cities include Beijing, Tianjin, Hebei, Liaoning, Shanghai, Jiangsu, Zhejiang, Fujian, Shandong, Guangdong, and Hainan.
2Western province cities include Inner Mongolia, Guangxi, Chongqing, Sichuan, Guizhou, Yunnan, Shaanxi, Gansu, Qinghai, Ningxia, and Xinjiang.
3Central province cities include Shanxi, Jilin, Heilongjiang, Anhui, Jiangxi, Henan, Hubei, and Hunan.
References
Chen, L., and Wang, K. (2022). The spatial spillover effect of low-carbon city pilot scheme on green efficiency in China’s cities: evidence from a quasi-natural experiment. Energy Econ. 110, 106018. doi:10.1016/j.eneco.2022.106018
Chen, H., Guo, W., Feng, X., Wei, W., Liu, H., Feng, Y., et al. (2021). The impact of low-carbon city pilot policy on the total factor productivity of listed enterprises in China. Resour. Conserv. Recy. 169, 105457. doi:10.1016/j.resconrec.2021.105457
Dong, D., and Wang, J. (2024). The undesirable impact of China’s “national sustainable development plan of resource-based cities” on CO2 intensity. Int. J. Sustain. Dev. and World Ecol. 31 (4), 395–410. doi:10.1080/13504509.2023.2294444
Fang, G., Yang, K., Tian, L., and Ma, Y. (2022). Can environmental tax promote renewable energy consumption? an empirical study from the typical countries along the Belt and Road. Energy 260, 125193. doi:10.1016/j.energy.2022.125193
Fang, G., Gao, Z., and Sun, C. (2023). How the new energy industry contributes to carbon reduction? evidence from China. J. Environ. Manag. 329, 117066. doi:10.1016/j.jenvman.2022.117066
Fang, G., Chen, G., Yang, K., Yin, W., and Tian, L. (2024). How does green fiscal expenditure promote green total factor energy efficiency? evidence from Chinese 254 cities. Appl. Energy 353, 122098. doi:10.1016/j.apenergy.2023.122098
Gao, K., and Yuan, Y. (2022). Government intervention, spillover effect and urban innovation performance: empirical evidence from national innovative city pilot policy in China. Technol. Soc. 70, 102035. doi:10.1016/j.techsoc.2022.102035
Guo, P., and Liang, D. (2022). Does the low-carbon pilot policy improve the efficiency of urban carbon emissions: quasi-natural experimental research based on low-carbon pilot cities. J. Nat. Resour. 37 (07), 1876–1892. doi:10.31497/zrzyxb.20220715
He, S., Liu, J., and Ying, Q. (2023). Externalities of government-oriented support for innovation: evidence from the national innovative city pilot policy in China. Econ. Model. 128, 106503. doi:10.1016/j.econmod.2023.106503
Jiang, N., Jiang, W., and Chen, H. (2023). Innovative urban design for low-carbon sustainable development: evidence from China's innovative city pilots. Sustain. Dev. 31 (2), 698–715. doi:10.1002/sd.2413
Li, H., and Wang, L. (2020). “Research on the impact of China’s national innovation city pilot policy on high-efficiency agglomeration—estimation and analysis based on DID models,” in Fifth International Conference on Economic and Business Management (FEBM 2020) (Atlantis Press), 37–41. doi:10.2991/aebmr.k.201211.007
Liang, X., Xu, Z., Wang, Z., and Wei, Z. (2023). Low-carbon economic growth in Chinese cities: a case study in Shenzhen city. Sci. Pollut. R. 30 (10), 25740–25754. doi:10.1007/s11356-022-24001-9
Liu, P., Zhao, Y., Zhu, J., and Yang, C. (2022). Technological industry agglomeration, green innovation efficiency, and development quality of city cluster. Green Finance 4 (4), 411–435. doi:10.3934/GF.2022020
Magazzino, C. (2017). The relationship among economic growth, CO2 emissions, and energy use in the APEC countries: a panel VAR approach. Environ. Syst. Decis. 37, 353–366. doi:10.1007/s10669-017-9626-9
McGavock, T. (2021). Here waits the bride? The effect of Ethiopia's child marriage law. J. Dev. Econ. 149, 102580. doi:10.1016/j.jdeveco.2020.102580
Pan, A., Zhang, W., Shi, X., and Dai, L. (2022). Climate policy and low-carbon innovation: evidence from low-carbon city pilots in China. Energy Econ. 112, 106129. doi:10.1016/j.eneco.2022.106129
Porter, M. E., and van der Linde, C. (1995). Toward a new conception of the environment-Competitiveness relationship. J. Econ. Perspect. 9, 97–118. doi:10.1257/jep.9.4.97
Qiu, S., Wang, Z., and Liu, S. (2021). The policy outcomes of low-carbon city construction on urban green development: evidence from a quasi-natural experiment conducted in China. Sustain. Cities Soc. 66, 102699. doi:10.1016/j.scs.2020.102699
Shang, Y., Raza, S. A., Huo, Z., Shahzad, U., and Zhao, X. (2023). Does enterprise digital transformation contribute to the carbon emission reduction? Micro-level evidence from China. Int. Rev. Econ. and Finance 86, 1–13. doi:10.1016/j.iref.2023.02.019
Si, H., Li, N., Duan, X., Cheng, L., and Bao, Z. (2023). Understanding the public's willingness to participate in the Carbon Generalized System of Preferences (CGSP): an innovative mechanism to drive low-carbon behavior in China. Sustain. Prod. Consum. 38, 1–12. doi:10.1016/j.spc.2023.03.016
Song, H., Sun, Y., and Chen, D. (2019). Evaluating the effectiveness of government air pollution control: an empirical study of China’s low carbon cities. Manag. World 35 (6), 95–108. doi:10.19744/j.cnki.11-1235/f.2019.0082
Song, Q., Liu, T., and Qi, Y. (2021). Policy innovation in low carbon pilot cities: lessons learned from China. Urban Clim. 39, 100936. doi:10.1016/j.uclim.2021.100936
Song, W., Mao, H., and Han, X. (2021). The two-sided effects of foreign direct investment on carbon emissions performance in China. Sci. Total Environ. 791, 148331. doi:10.1016/j.scitotenv.2021.148331
Tone, K. (2002). A slacks-based measure of super-efficiency in data envelopment analysis. Eur. J. Oper. Res. 143 (1), 32–41. doi:10.1016/S0377-2217(99)00407-5
Wang, Q., and Li, L. (2021). The effects of population aging, life expectancy, unemployment rate, population density, per capita GDP, urbanization on per capita carbon emissions. Sustain. Prod. Consum. 28, 760–774. doi:10.1016/J.SPC.2021.06.029
Wang, H., and Yi, Q. (2023). Effect of new urbanization on cities’ innovation in China: evidence from a quasi-natural experiment of a comprehensive pilot. Plos one 18 (4), e0284772. doi:10.1371/JOURNAL.PONE.0284772
Wang, K. L., Xu, R. Y., Zhang, F. Q., and Cheng, Y. H. (2022). Reinvestigating the spatiotemporal differences and driving factors of urban carbon emission in China. Front. Environ. Sci. 10, 880527. doi:10.3389/FENVS.2022.880527
Wang, T., Song, Z., Zhou, J., Sun, H., and Liu, F. (2022). Low-carbon transition and green innovation: evidence from pilot cities in China. Sustainability 14 (12), 7264. doi:10.3390/SU14127264
Wen, S., Jia, Z., and Chen, X. (2022). Can low-carbon city pilot policies significantly improve carbon emission efficiency? Empirical evidence from China. J. Clean. Prod. 346, 131131. doi:10.1016/J.JCLEPRO.2022.131131
Wu, J., and Guo, Z. (2016). Convergence analysis of China's carbon emissions based on the continuum dynamic distribution approach. Stat. Res. 33 (01), 54–60. doi:10.19343/j.cnki.11-1302/c.2016.01.008
Xu, J., and Cui, J. (2020). Low-carbon cities and firms’ green technological innovation. China Ind. Econ. 37, 178–196. doi:10.3969/j.issn.1006-480X.2020.12.016
Xu, Y. (2024). Urban innovation, heterogeneous environmental regulations and haze reduction effects–evidence from pilot policies of innovative cities. Front. Environ. Sci. 11. doi:10.3389/fenvs.2023.1310313
Yang, Z., and Shi, D. (2023). Towards carbon neutrality: the impact of innovative city pilot policy on corporate carbon intensity in China. Clim. Policy 23 (8), 975–988. doi:10.1080/14693062.2022.2124224
Yang, X., Jiang, P., and Pan, Y. (2020). Does China’s carbon emission trading policy have an employment double dividend and a Porter effect? Energy Policy 142, 111492. doi:10.1016/j.enpol.2020.111492
Yang, J., Hao, Y., and Feng, C. (2021). A race between economic growth and carbon emissions: what play important roles towards global low-carbon development? Energy Econ. 100, 105327. doi:10.1016/J.ENECO.2021.105327
Yang, G. (2023). Can the low-carbon city pilot policy promote firms’ low-carbon innovation: evidence from China. Plos one 18 (1), e0277879. doi:10.1371/JOURNAL.PONE.0277879
Yu, C., Long, H., Tu, C., Tan, Y., Zang, C., and Zhou, Y. (2022). Assessing the effectiveness of innovative city pilots in improving urban carbon emission performance: a spatial difference-in-difference approach. Front. Environ. Sci. 10, 983711. doi:10.3389/fenvs.2022.983711
Zeng, J., Ning, Z., Lassala, C., and Ribeiro-Navarrete, S. (2023). Effect of innovative-city pilot policy on industry–university–research collaborative innovation. J. Bus. Res. 162, 113867. doi:10.1016/J.JBUSRES.2023.113867
Zeng, S., Jin, G., Tan, K., and Liu, X. (2023). Can low-carbon city construction reduce carbon intensity? Empirical evidence from low-carbon city pilot policy in China. J. Environ. Manage. 332, 117363. doi:10.1016/J.JENVMAN.2023.117363
Zhang, X., and Fan, D. (2023). Collaborative emission reduction research on dual-pilot policies of the low-carbon city and smart city from the perspective of multiple innovations. Urban Clim. 47, 101364. doi:10.1016/j.uclim.2022.101364
Zhang, J., and Zheng, T. (2023). Can dual pilot policy of innovative city and low carbon city promote green lifestyle transformation of residents? J. Clean. Prod. 405, 136711. doi:10.1016/j.jclepro.2023.136711
Zhang, J., Wu, G., and Zhang, J. (2004). The Estimation of China’s provincial capital stock: 1952—2000. Econ. Res. (10), 35–44.
Zhang, B., Zhou, J., and Yan, Z. (2021). Low carbon city pilot policies and total factor energy efficiency improvement: quasi—natural experiment from the implementation of three batches of pilot policies. Econ. Rev. 42, 32–49. doi:10.19361/j.er.2021.05.03
Zhang, H., Feng, C., and Zhou, X. (2022). Going carbon-neutral in China: does the low-carbon city pilot policy improve carbon emission efficiency? Sustain. Prod. Consump. 33, 312–329. doi:10.1016/J.SPC.2022.07.002
Zhang, B., Xie, J., and Wu, J. (2023). Has the innovation-driven policy suppressed the “de-realization” of real enterprises--a quasi-natural experiment based on the pilot policy of national innovative cities? Sci. Technol. Prog. responses 40 (14), 114–122. doi:10.6049/kjjbydc.2023010026
Zhao, W., and Toh, Y. M. (2023). Impact of innovative city pilot policy on industrialstructure upgrading in China. Sustainability 15 (9), 7377. doi:10.3390/su15097377
Zhao, C., and Wang, B. (2021). Does China’s low-carbon pilot policy promote foreign direct investment? An empirical study based on city-level panel data of China. Sustainability 13 (19), 10848. doi:10.3390/su131910848
Zheng, J., Shao, X., Liu, W., Kong, J., and Zuo, G. (2021). The impact of the pilot program on industrial structure upgrading in low-carbon cities. J. Clean. Prod. 290, 125868. doi:10.1016/j.jclepro.2021.125868
Keywords: dual pilot of low-carbon and innovative cities, carbon emission efficiency, super-SBM model, multi-period DID model, China
Citation: Deng G, Wu Y and Qian J (2024) Research on the impact of dual pilot projects for low-carbon and innovative cities on carbon emission efficiency. Front. Environ. Sci. 12:1432400. doi: 10.3389/fenvs.2024.1432400
Received: 14 May 2024; Accepted: 25 October 2024;
Published: 22 November 2024.
Edited by:
Worradorn Phairuang, Chiang Mai University, ThailandReviewed by:
Mohamed R. Abonazel, Cairo University, EgyptGuochang Fang, Nanjing University of Finance and Economics, China
Copyright © 2024 Deng, Wu and Qian. This is an open-access article distributed under the terms of the Creative Commons Attribution License (CC BY). The use, distribution or reproduction in other forums is permitted, provided the original author(s) and the copyright owner(s) are credited and that the original publication in this journal is cited, in accordance with accepted academic practice. No use, distribution or reproduction is permitted which does not comply with these terms.
*Correspondence: Guangyao Deng, ZGd5MzE2MjAzQDE2My5jb20=