- 1 School of Economics and Management, Weifang University of Science and Technology, Weifang, Shandong, China
- 2 School of Accounting, Anhui University of Finance and Economics, Bengbu, China
Introduction: With the acceleration of the allocation of agricultural resource elements in agricultural development, the relationship between digital rural construction and rural land use efficiency has become increasingly close.
Methods: In order to explore the impact and underlying mechanism of digital rural construction on rural land use efficiency, this paper constructs an evaluation system index for China's digital rural construction and uses the SBM-GML model to measure rural land use efficiency. Based on this, data from 30 provinces in China from 2010 to 2022 are used to test it using fixed effects and mediation effects models.
Results: (1) The construction of digital rural areas can directly promote the improvement of rural land use efficiency. This conclusion still holds true after endogeneity and robustness tests. (2) Mechanism analysis shows that digital rural construction can alleviate the mismatch of land resources, capital resources, and labor resources, thereby indirectly promoting the improvement of rural land use efficiency. (3) Heterogeneity analysis shows that the construction of digital rural areas has a more significant driving effect on the efficiency of rural land use in eastern and southern regions of China, as well as in major grain producing and selling areas.
Discussion: This article suggests continuing to promote the development strategy of digital rural construction, improving the problem of resource mismatch, and paying attention to the regional imbalance of digital rural construction. It is necessary to maintain the leading position of “first mover advantage” areas and also pay attention to filling the gaps in “later mover advantage” areas, in order to comprehensively promote the further improvement of rural land use efficiency.
1 Introduction
China’s agricultural development has achieved remarkable success in the global economic landscape, not only becoming a new engine driving China’s economic growth, but also demonstrating strong competitiveness and resilience on the international stage. However, the achievement of this result has long relied mainly on intensive investment in traditional factor resources. With the increasing depletion of these traditional resources, Chinese agriculture is entering a critical period of urgent transformation. Guo and Liu (2023) pointed out that solving the problem of resource mismatch and exploring and utilizing modern production factors have become the key to promoting the sustainable and healthy development of Chinese agriculture. Against the backdrop of China’s economy entering a new stage of development, the rapid rise of the tertiary industry has brought profound changes to the rural economic structure. However, this change has also brought many challenges, such as the massive loss of rural labor, the continuous decline in the quality of arable land, the decreasing efficiency of land use, and the generally low return on agricultural capital. These issues not only severely constrain the efficiency and benefits of agricultural production, but also pose a serious threat to the livelihoods of farmers and the sustainable use of rural land. In the face of this severe situation, we must take prompt action to explore new sources of driving force for improving rural land use efficiency and promoting high-quality agricultural development. Land, as the most fundamental and important resource element in agricultural production, its effective utilization and rational allocation are of great significance for improving agricultural production efficiency, safeguarding farmers’ land rights, alleviating human land conflicts, and promoting sustainable agricultural development. However, under the existing non market mechanism land transaction model in China, there is a serious waste of land resources, and the problem of low efficiency in rural land use is particularly prominent. This not only leads to the damage of farmers’ land and property rights, but also exacerbates the tension of the human land conflict. Therefore, further deepening the market-oriented reform of rural land factors in China and establishing a more fair, transparent, and efficient land trading market has become an urgent task to ensure the sustainable development of agriculture. In this process, we also need to pay close attention to the impact of agricultural resource allocation efficiency on rural land use efficiency. The mismatch of resources, especially the mismatch of capital factors, has become an important bottleneck restricting the development of agricultural economy. Hsieh and Klenow (2009) found that compared to optimal resource allocation, misallocation of resources can lead to reverse flow of resources, severely constraining the development of agricultural economy. Sun et al. (2021) further pointed out that the mismatch of capital factors has a significant negative impact on China’s economic growth, and the income gap in regions where there is no mismatch of capital factors is smaller. This discovery reveals the intrinsic connection between resource misallocation and income inequality, and provides important reference for us to formulate more scientific and reasonable agricultural policies. Therefore, in order to promote the high-quality development of Chinese agriculture, we must start from multiple levels, including deepening the market-oriented reform of rural land factors and improving land use efficiency; We need to optimize the allocation of agricultural resources and reduce the mismatch of resource elements; At the same time, it is necessary to strengthen agricultural technological innovation and talent cultivation, and improve the total factor productivity of agriculture. Only in this way can we ensure that China’s agriculture maintains a leading position in global economic competition, create better living conditions for farmers, and inject stronger impetus into China’s economic and social development.
The construction of digital villages, as an inevitable product of the informatization of economic and social development in agriculture and rural areas and the enhancement of farmers’ information skills, marks an important process of modernization and transformation of agriculture and rural areas (Silijuan, 2024). The construction of digital rural areas, as an important component of the rural revitalization strategy in the new era, is leading China’s rural areas towards an unprecedented new stage of intelligent and information-based development. In 2019, the Outline of the Strategy for the Development of Digital Villages, jointly issued by the General Office of the Communist Party of China (CPC) Central Committee and the State Council, explicitly puts the “construction of a smart and green countryside” as one of the key tasks, requiring the deployment of the promotion of green production methods in agriculture, promoting the reduction of the use of chemical fertilizers and pesticides, and the development of green agriculture. Since then, the Ministry of Agriculture and Rural Affairs and other relevant departments have issued the “Digital Agricultural and Rural Development Plan (2019–2025),” the “Key Points of the Work on the Development of Digital Countryside for the Year 2020,” as well as the “Notice on the Implementation of the National Digital Countryside Notice on Pilot Work” and other policy documents, which provide an important guide for further in-depth implementation of the digital rural development action and promote the greening and modernization of agriculture and rural areas. Through the construction of digital rural areas, we hope to create a smart, green, and harmonious new rural landscape, allowing farmers to enjoy more convenient, efficient, and intelligent production and living services, and making rural areas a beautiful home for living and working. This is not only a positive response to the aspirations of farmers for a better life, but also a powerful support for the country’s modernization process, and a positive contribution to the global sustainable development goals. Therefore, in the era of digital economy, in-depth exploration of the potential relationship between digital village construction and rural land use efficiency from both theoretical and empirical perspectives can provide certain empirical evidence to promote the transformation of China’s farmers’ production, and accelerate the realization of comprehensive transformation and upgrading of agriculture. Specifically, this paper focuses on the following questions: first, does digital village construction have an impact on rural land use efficiency? Does it produce heterogeneous impacts under different locational conditions? Second, from the perspective of mismatch of resource, i.e., mismatch of land resource factors, mismatch of capital resource factors, and mismatch of labor resource factors, what is the intermediary role played by the mismatch of resource in the relationship between digital countryside construction and agricultural land use efficiency? Answering the above questions will help to further promote the real-time digital village construction policy, improve the efficiency of rural land utilization and put forward corresponding measures to solve the problem, thus empowering the synergistic and high-quality development of China’s agriculture.
The existing literature has built a basic framework for the study of digital village construction as well as rural land use efficiency, but there are still the following shortcomings. First, the evaluation system of digital village construction is not perfect enough and is in the exploratory stage. Second, the existing literature on the study of digital village construction on rural land use efficiency is relatively lacking, and the causal relationship between the two is not yet clear. Third, the transmission mechanism of digital village construction on rural land use efficiency is not yet clear, ignoring the impact of resource mismatch. Compared with the existing studies, the possible marginal contributions of this paper are: first, constructing indicators of digital village construction. On the basis of existing research, this paper constructs digital village construction indicators from three major dimensions: digital infrastructure, agricultural digitization, and rural digitization, to further improve the evaluation system of China’s digital village construction, and to provide a more scientific and standard evaluation support for China’s digital village construction evaluation system. Second, from the existing literature, there is a lack of research on the utilization efficiency of rural land by digital rural construction, which leads to a biased assessment of the accuracy of rural land utilization efficiency. In order to explore the possible impact of digital village construction on rural land use efficiency, this paper uses data from 30 Chinese provinces in China for the period 2020–2022 to empirically test and confirm the reliability of this paper’s conclusions through a variety of methods, including instrumental variables and robustness tests, to provide important references for China’s agricultural development as well as the improvement of land use efficiency. Thirdly, due to the relatively short period of time that digital village construction is proposed, the transmission mechanism on rural land use efficiency is still unclear, and from the existing studies, no literature has been conducted from the channel of resource mismatch, which may lead to an overestimation of the positive effect of digital village construction on rural land use efficiency. This paper clarifies the transmission mechanism of digital village construction on rural land use efficiency from the three dimensions of land resource factor mismatch, capital resource factor mismatch and labor resource factor mismatch.
The remainder of the paper is organized as follows: the second part is a literature review and theoretical hypotheses; the third part is the research design, which introduces the data sources, variables, and models; the fourth part is the benchmark regression, endogeneity test, robustness test, heterogeneity analysis, and the role of renewable energy sources, and the fifth part is a further study to test the roles of government environmental protection expenditures and government environmental protection taxation; and lastly, the research conclusions and policy recommendations.
2 Literature review
2.1 Research on the measurement and influencing factors of rural land use efficiency
In previous studies, some scholars have used principal component analysis and analytic hierarchy process to measure land use efficiency. However, the drawback of these two methods is that they have subjectivity in the selection of indicator weights and assignment values. More scholars will use data envelopment analysis to measure land use efficiency, thereby eliminating the interference of subjective factors and ensuring the objectivity of evaluation results (Zhang et al., 2019). For example, Lin and Ma (2004) used data envelopment analysis to measure the land use efficiency of urban agglomerations in China in the years 2000, 2006, and 2011. The study found that the land use efficiency of urban agglomerations in China was relatively low and exhibited significant heterogeneity. In terms of indicator selection, scholars pay more attention to the economic benefits brought by land use efficiency. For example, Rongzeng et al. (2021) used the SBM model to measure the efficiency of urban and rural land use in China from land input, capital input, labor input, and other aspects. However, Wu et al. (2011) found that the efficiency of urban land use in China is the result of multiple factors working together, and the evaluation system needs to be based on natural, economic, and social perspectives. In addition to considering the economic benefits of land use, social and environmental benefits should also be taken into account.
Long et al. (2007) studied the impact of socio-economic factors on land use efficiency in the Yangtze River Delta region of China. Liao et al. (2010) empirically analyzed the impact of urbanization rate on land use efficiency in the Changsha Zhuzhou Xiangtan region of China. The study found that in the short term, an increase in urbanization level would reduce land use efficiency, but in the long run, urbanization level would improve land use efficiency. Yang et al. (2010) found that rural population, urbanization rate, proportion of primary industry, Engel coefficient, and urban-rural per capita income ratio are all influencing factors of rural land use.
2.2 Research on the economic effects of digital rural construction
The research closely related to this article includes the economic and social effects of digital rural construction. Firstly, the scientific construction of an evaluation index system for digital rural areas is a prerequisite for studying their economic and social effects. Existing scholars often combine digital rural policy documents and select indicators such as digital infrastructure conditions, digital rural governance, digital capital investment, digital industry development, and digital service level to evaluate the construction of digital rural areas (Lin et al., 2023; Zhu and Chen, 2022). Other scholars use the Digital Rural County Development Index to refine digital rural construction into aspects such as infrastructure digitization, rural economic digitization, rural governance digitization, and rural life digitization (Jiajia et al., 2023). Specifically, in terms of the economic and social effects of digital rural construction, at the macro level, digital rural construction can significantly promote the improvement of industrial prosperity (Benqing et al., 2022), reduce the proportion of the primary industry in the county economy, optimize the non-agricultural industry structure, increase the proportion of the service industry, promote the transformation of the county economy to the tertiary industry (Tao et al., 2022), and help enhance the resilience of the food system (Aimin and Tan, 2022), and promote the improvement of agricultural green total factor productivity (Du et al., 2022); At the micro level, the development of digital rural areas can achieve the upgrading of rural household consumption by promoting the increase of total consumption and optimizing consumption structure (Wan and Wang, 2024), and significantly increase the probability of non-agricultural employment and entrepreneurship in rural households (Yin et al., 2024), and improve the income level of farmers (Qi et al., 2021). The above-mentioned experts and scholars have conducted rich research on digital rural areas, laying the research foundation for this article. However, it is not difficult to find that existing studies have mostly constructed indicator systems from a macro level when evaluating the level of digital rural construction, with little refinement or quantification to a specific administrative village, and little research has focused on the potential relationship between digital rural construction and land use efficiency.
2.3 Related research on resource mismatch problem
Classical economic theory holds that in a state of perfect competition, the required resource factors are regulated by the market, and the factors can flow freely between regions, achieving effective allocation of factors. The academic community has conducted in-depth research and discussion on the issue of element configuration. Based on existing literature, the discussion mainly focuses on the reasons, impacts, and improvement behaviors.
The first reason is the mismatch of elements. There are two main explanations for the formation reasons. One reason is the “endogenous allocation distortion” caused by imperfect market competition, mainly due to market segmentation leading to inefficient allocation of resource factors (Kong and Zhou, 2020). Chen and Wang (2020) found that due to the “siphon effect” in cities, they compete with rural markets, leading to a decrease in the ability of rural markets to attract resource elements and further scarcity of agricultural resources.
Secondly, external factors outside the factor market, such as unreasonable institutional arrangements and excessive government intervention, can lead to problems in the allocation ratio of resource factors, resulting in misallocation of factor resources (Hengzhou et al., 2011). Zhou et al. (2022) found that although the implementation of the “urban bias” development policy has brought rapid development to cities and industrial sectors, it also leads to urban-rural segmentation and further widening of the economic gap, which is the key reason for the mismatch. Secondly, the impact of mismatched elements. Yuan and Dongdong (2011) found that labor mismatch in China leads to a 20% loss in economic output efficiency. Peiwen and Yang (2019) found that labor mismatch further widens income inequality, leading to uneven economic development and forming a vicious cycle. Some scholars have also found that the rural market in China has poor circulation of factors and insufficient activation of resources. If the flow of labor and capital in the rural market can be facilitated, the mismatch of factors between regions can be improved, and China’s total productivity level can be increased by up to 51% (Jing et al., 2012). The above research conclusions indicate that the reasons for factor mismatch are diverse, and the impact of factor mismatch on the economy is negative, which will greatly affect the development of the Chinese economy.
Thirdly, improve the mismatch of elements. If it is in a closed market environment, Restuccia and Rogerson (2012) found that optimizing the flow of labor and capital factors can improve factor allocation. In the current situation of rapid development of digital technology, it is necessary to use the products of the new era to improve the current factor allocation (Wang and Zhang, 2021). Zhou et al. (2022) found from the perspective of resource mismatch that the digital economy has narrowed the urban-rural gap. However, Han and Zhang (2019) found that digital technology represented by the Internet has a threshold effect on the improvement of factor mismatch, and only when the Internet penetration reaches a certain level can the labor force mismatch be reduced.
2.4 Research on the impact of digital rural construction on agricultural land use efficiency
Firstly, the construction of digital rural areas is achieved through the superposition, diffusion, and penetration of rural infrastructure, data elements, and resource allocation, as well as digital technology. This results in the formation of superposition, diffusion, and penetration effects on rural agricultural production, thereby reducing resource misallocation and production costs, and improving agricultural scale production (Wang and Yongxiu, 2022). Wen and Chen (2020) believe that the integration of digital economy development with rural agricultural production can effectively alleviate the information asymmetry in traditional agricultural production, optimize agricultural resource allocation, and achieve goals such as reducing transaction costs and increasing agricultural scale production. In this context, studying the relationship between digital rural construction and rural land use efficiency has become a hot topic of academic concern. Currently, the academic community has a relatively consistent view on the impact of digital economy and digital rural areas on rural development. Firstly, the digital economy can provide platform technology for agriculture, improve agricultural production and land use efficiency, transform traditional extensive production methods, and influence agricultural development (Brody et al., 2006; Camarinha-Matos et al., 2009; Attour and Barbaroux, 2021).
Secondly, the construction of digital rural areas empowers agricultural production through multidimensional digital technology, becoming a new type of productive force for the development of the agricultural industry (Shen and Ye, 2021). Digital technology is conducive to optimizing resource allocation, creating disruptive innovation and creative destruction to traditional agricultural production models (Yin et al., 2020), and contributing to the construction of green, efficient, and intensive modern agriculture. Thirdly, the construction of digital rural areas can generate strong resource spillover effects on agricultural entities (Chen, 2021), help optimize factor allocation, reduce production costs, alleviate information asymmetry, further optimize labor structure, land use efficiency, transform agricultural production models (Shen and Ye, 2021), enhance agricultural production enthusiasm, stimulate internal motivation, and thus generate a multiplier effect on agricultural modernization. Fourthly, the construction of digital countryside can also reduce the cost of farmers obtaining information, including commodity and market information (Mary George et al., 2016), improve farmers’ ability to directly connect with the market and human capital level, and promote the transformation and upgrading of the agricultural industry (Song et al., 2020).
In summary, in the new era of digital rural construction, digital rural construction plays an important role in the development of China’s rural economy and also has a significant impact on improving the efficiency of rural land use. However, existing literature on this topic is relatively scarce. Therefore, this article first analyzes the mechanism of digital rural construction on rural land use efficiency from a theoretical perspective, and uses panel data from 30 provinces in China from 2010 to 2022 to measure the evaluation system of China’s land use efficiency and digital rural construction. Further, an empirical model is constructed to test the impact and mechanism of digital rural construction on rural land use efficiency.
3 Theoretical analysis and research hypothesis
The Outline of the Digital Rural Development Strategy states that digital rural construction is the application of networking, informatization and digitization to the economic and social development of rural agriculture, and the improvement of farmers’ modern information skills, as well as the scaling up of agricultural production. Therefore, digital rural construction to improve agricultural economic efficiency, to a large extent, need to improve the efficiency of resource allocation, land as an important resource endowment, the utilization of efficiency can help agriculture to achieve transformation and upgrading. The connotation of digital rural construction is to further extend agricultural informatization by relying on the development of the digital economy, using modern information technology such as artificial intelligence as a driving force, and using platform technology as a carrier to reshape rural economic development as a means and process. The main feature of digital rural construction is to utilize the concept of digital economic development, drive the improvement of agricultural land use efficiency through technological innovation, realize efficiency change, power change and quality change, promote the precision, intelligence and greening of rural construction, and promote the comprehensive upgrading of agriculture and the comprehensive progress of rural areas (Wang et al., 2021). The following will comprehensively explain the impact of digital rural construction on rural land use efficiency and its mechanism of action.
3.1 Digital village construction and rural land use efficiency
First, agricultural technology transformation and upgrading and the optimal allocation of agricultural resources are key factors in the improvement of land use efficiency. Based on the theory of induced technological change, the key source of motivation for agricultural technology transformation and upgrading is the endowment of technological resource factors, and the application of technology and the relative price of factors determine the way of resource factor allocation. With the policy advancement of digital village construction, the pace of digital application in agriculture has accelerated, and the improvement of information collection, transmission and processing capacity is conducive to the flow of land resources, agricultural costs continue to decline, and land use efficiency has been improved. Digital village construction can provide channels for agricultural business entities to apply digital technology, and provide a meritocratic path for optimizing resource allocation, improving land use efficiency, and reducing agricultural pollution and carbon emissions. Therefore, digital village construction has changed the original traditional mode of rough operation, improved the development of agricultural industrialization, and land use efficiency is bound to be improved under large-scale operation.
Second, from the perspective of the land transfer side, the development of rural e-commerce and Taobao villages improves employment opportunities for the rural population, which leads to a decrease in the opportunity cost of land transfer and increases the probability of transferring the right to use land (Zhang and Zhang, 2020), which is conducive to the formation of large-scale development of agriculture and the improvement of the efficiency of land use. Therefore, if farmers originally engaged in agricultural production receive higher income from non-farm industries, farmers will be more willing to transfer land and thus invest in the non-farm sector (Shen et al., 2017). From the perspective of both sides of land transfer, digital village construction can provide an information platform for agricultural development, break the obstacles of time and space, provide timely information for both sides of land transfer (Coelli and Rao, 2004), and improve the efficiency of land transfer. Therefore, digital village construction can accelerate land transfer and improve land utilization efficiency. Since individual farmers lack access to information, they need to complete the collection of transfer information, contract negotiation and signing by themselves, which requires high costs. However, the information platform provided by digital village construction can help farmers obtain efficient land supply and demand information and reduce transaction costs. In short, agricultural production and management activities rely on digital village construction to increase land transfer, improve land allocation efficiency, and meet the large-scale operation of agricultural production.
Finally, the construction of digital villages can accelerate the flow of agricultural information and break down information barriers. First, Pereira et al. (2019) found that the application of digital technology can obtain timely information on production technology, market demand, government transmission, etc., which is beneficial to the rational allocation of resources, timely adjustment of industrial structure, and increased land utilization efficiency. Relying on digital technology can further break through the market information barriers between urban and rural areas and regions, reduce the cost of obtaining information, and increase the probability of successful transactions. Secondly, digital rural construction helps to improve the agricultural industry chain, and through the realization of the data sharing system between farmers and research institutes, it can accurately match the situation needed for scientific research and agriculture, thus improving the efficiency of the use of chemical fertilizers and pesticides, and promoting the effectiveness of the use of agricultural production resources. Thirdly, the construction of digital countryside helps to build a system to promote agricultural science and technology, form scale development, reduce the cost of agricultural learning, and drive the improvement of land use efficiency. Based on this, the following hypotheses are proposed.
Hypothesis 1. The construction of digital villages can contribute to the efficiency of rural land use.
3.2 Digital village construction, resource mismatch and rural land use efficiency
Resource mismatch will lead to a decline in agricultural production efficiency, and the improvement of the allocation efficiency of resource is an important source of improving land utilization efficiency. Digital village construction, as an organic combination of digital economy and traditional agriculture and rural areas, can largely improve the allocation efficiency of resource elements according to the penetration effect, substitution effect and synergistic effect of digital technology, inhibit the degree of mismatch of resource to a certain extent, and promote the improvement of the efficiency of rural land use. In the process of digital village construction, the penetration effect refers to the initial stage of data elements used in rural areas, driving the flow of resource elements between urban and rural areas. The substitution effect refers to the substitution of data elements for the original traditional elements and the improvement of the utilization efficiency of traditional elements. Synergistic effect refers to the synergistic evolution of industrial digitization and digitized industries, which jointly promote the efficiency of factor allocation. Based on the ternary theory of agricultural production factors, this paper will analyze the role played by resource mismatch between digital village construction and rural land use efficiency from the three dimensions of land resource mismatch, capital resource mismatch, and labor mismatch.
First, in terms of land resource mismatch. All along, land elements have been evenly distributed based on the size of the family, so the differences in land utilization efficiency between different families have been ignored, resulting in the mismatch of land resources caused by the mismatch between scale and efficiency. In addition, the lack of digital technology leads to greater information asymmetry, increasing the opportunity cost of land scale transfer, and the existence of the above factors leads to a greater degree of land resource mismatch. The construction of digital villages can help realize the digitization of the whole process of land management, promote the new business model of land transfer, accelerate the process of land transfer, and to a certain extent can inhibit the degree of land mismatch. Firstly, the construction of digital rural areas can promote the establishment of land resource information platforms, achieve digitalization and visualization of land resources, and further improve the transparency of land resource information. Secondly, the construction of digital rural areas utilizes technologies such as artificial intelligence and big data to accurately analyze land resources.
Hypothesis 2. Digital rural construction can alleviate the mismatch of land elements and improve the efficiency of rural land use.
Second, in terms of capital resource mismatch. Due to the development of industrialization, the proportion of secondary and tertiary industries has gradually increased, and at the same time, the development of the urban-rural dual structure has led to a high degree of concentration of capital in the cities, resulting in a greater flow of capital to the cities and the emergence of a mismatch of capital in rural areas. According to Soto capitalization theory, capital can only be capitalized in the market. Digital village construction through digital technology in agriculture and rural areas continue to penetrate, gradually break the market space constraints, promote the integration of digital technology and rural economic development, stimulate the potential of rural idle assets, to the direction of capitalization, to a certain extent, can inhibit the degree of capital mismatch. At the same time, relying on digital finance and other forms of technology can further make capital flow back to rural agriculture, and further improve the capital mismatch situation.
Hypothesis 3. The construction of digital villages can alleviate capital factor mismatch and improve the efficiency of rural land use.
Finally, in terms of labor resource mismatch. Digital village construction can break the barrier of two-way flow of factors between urban and rural areas and reduce the degree of labor mismatch. Compared with cities, rural areas are more backward in terms of infrastructure, transportation facilities, etc., and have a single form of industry, mainly based on agricultural production, which makes the labor force with a higher level of skills unable to return to the rural areas for development, and one-way flow to the towns. At the same time, rural laborers with single skills and low educational level will encounter problems such as market system segmentation and structural employment in the urban market, which makes it impossible for rural laborers to integrate well into the urban market, and the efficiency of agricultural production is also not high. Through the transformation of rural infrastructure, the integration of factor endowments, and industrial digitalization, digital village construction can improve the quality of the rural workforce, improve the structure of the agricultural industry chain, and promote the development of the overall agricultural industry, which is conducive to the improvement of the efficiency of land use. At the same time, the construction of digital villages can drive mass entrepreneurship and innovation, provide more laborers with entrepreneurial and employment positions, attract the return of laborers, and further improve the efficiency of rural land use. Based on this, the following hypotheses are proposed.
Hypothesis 4. The construction of digital villages can alleviate labor mismatch and improve the efficiency of rural land use.
4 Research design
4.1 Initiative variable definition
4.1.1 Explained variable: rural land use efficiency (lue)
In 1978, Charnes and other scholars firstly proposed the Data Envelopment Analysis (DEA) method for measuring multiple-input multiple-output decision units, and this method has been widely used in efficiency evaluation and other aspects. However, the traditional DEA model cannot realize the efficiency evaluation of non-expected outputs, and the model also suffers from the problem of input-output variable slackness, in this regard, Tone (2002) proposed the SBM-Undesirable model and the super-efficient SBM-Undesirable model, thus effectively solved the problem of input-output variable slack. The scientific validity of using SBM Undesirable model and super efficiency SBM Undesirable model to measure rural land use efficiency mainly lies in the following aspects: firstly, considering the influence of slack variables. It considers the slack variables of input and output, namely, the situation of input surplus and output deficit. This consideration enables the SBM model to more comprehensively evaluate the efficiency of decision units (DMUs), avoiding result bias caused by radial and angular selection issues. Secondly, it can effectively distinguish decision-making units. In the evaluation of rural land use efficiency, there may be situations where the efficiency values of multiple regions or farmers are high and close to 1. The use of the super efficient SBM model can more finely distinguish the efficiency differences between these efficient regions or farmers, providing a basis for formulating more accurate improvement strategies. Thirdly, consider cross period dynamic changes. The SBM-GML model can clearly demonstrate the changes in rural land use efficiency over different periods, as well as the driving factors behind efficiency changes. This helps policymakers understand the development trends of land use efficiency and formulate more forward-looking policies. To this end, this paper uses the super-efficient SBM-Undesirable model that considers non-desired outputs under the input perspective and constant fixed scale payoffs to measure the rural land use efficiency, and also uses the agricultural land use efficiency measured by the SBM-Undesirable model as a replacement of the explanatory variables for robustness Test.
Assuming that there are n production decision-making units (DMUs, n = 1, 2, 3, N), and each DMU contains cast desired outputs, non-desired outputs, the number of which are m, l, and h, respectively, the formula for measuring the efficiency of agricultural land use is as follows (Equation 1):
Among them,
Table 1 shows the inputs and outputs of rural land use efficiency.
4.1.2 Explanatory variables: digital village construction
In this paper, digital village construction is based on the Digital Village Development Strategy Outline issued by China as the policy basis, referring to the research of Zhu and Chen (2022), following the principles of data availability, scientificity and comprehensiveness, and constructing evaluation indicators for digital village construction from three dimensions: digital basic environment, agricultural digitization, and rural digitization.
First, the digital infrastructure environment provides the preconstruction foundation for digital village construction. This dimension is mainly measured by the construction of rural circulation facilities, the number of 5G base stations, rural electricity consumption, and the level of digital base construction, in which the number of 5G base stations indicates the current digital construction infrastructure situation, which is the core element of digital village construction. The construction of rural distribution facilities is mainly the number of rural express stations set up and delivery routes, which can reflect the level of digital technology application. Rural electricity consumption at a certain level can indicate the development of rural digital economy, the higher the level of digital economy, the higher the electricity consumption, the level of rural digital economy construction is an important supporting force for the construction of digital villages. The level of digital base construction is measured by the ratio of rural Taobao villages to administrative villages, and the use of e-commerce data can well reflect the level of digital base construction.
Second, the digitization of agriculture is the core content of digital village construction. This dimension is mainly measured by the degree of digitization used in the four links of agricultural production, distribution, sales, and service, thus measuring the penetration of digitization in the agricultural industry chain from different links. In particular, with reference to the study of Wang et al. (2023), the degree of digitization of agricultural production is measured by the number of enterprises engaged in production activities using Internet technology and by the ratio of the number of people employed in agriculture to the total number of people employed. The agricultural distribution, sales, and service segments are represented using the digitalized transactions of agricultural products, the scale of agricultural digitization, and the level of agricultural digitization services, respectively. The level of agricultural digitalization services is measured using the Digital Financial Inclusion Index compiled by Peking University.
Finally, rural digitization is a direct reflection of digital village construction. This dimension is mainly reflected through the degree of digitization of rural life, which is measured by the use of smartphones by the rural population, the rural Internet penetration rate, and the number of rural meteorological observation stations, respectively, from which the degree of digital upgrading of the countryside in terms of mobile communications, networks, and meteorological services is indicated. In addition, meteorological information such as wind speed, light, temperature, humidity, etc., can also be obtained through rural meteorological observation stations, thus providing first-hand meteorological information for agricultural digital production. The level of rural digital application is measured from the per capita transportation and communication expenditure of rural residents. Since the metrics of the indicators selected in this paper are not uniform, the entropy weight method is used to standardize the indicators. Specific indicators are shown in Table 2.
4.1.3 Mediating variable: resource mismatch
The research of this paper is resource mismatch, according to the characteristics of agricultural production, this paper divides resource mismatch into three dimensions: land resource mismatch (lan), capital resource mismatch (kap) and labor resource mismatch (lab). The resource factor mismatch index refers to the calculation method of Chen and Zhang (2022). The specific calculation formula is shown below (Equations 2–4):
Among them,
4.1.4 Control variable
Gross domestic product (gdp) per capita, measured by the proportion of provincial gdp to total population; (2) rural population (rpo), measured by the proportion of rural population to year-end resident population; (3) water use in agriculture (wag), measured by the proportion of total water use in agriculture to total water use; (4) degree of agrarianization (dag), measured by the proportion of value added in agriculture to regional gdp; (5) machinery density (med), measured by the proportion of total power of agricultural machinery to the value added of agriculture; (6) the proportion of primary industry (psi), measured by the proportion of the value added of primary industry to the value added of secondary and tertiary industries. Descriptive statistics of the variables are shown in Table 3.
4.2 Model building
4.2.1 Baseline regression model
In order to test the impact of digital village construction on rural land use efficiency, this paper uses the “time-individual” two-way fixed-effects model for empirical testing. The use of a two-way fixed effects model is to simultaneously control for individual specific effects that do not change over time and the common utility that changes over time but affects all individuals. It can also avoid missing variables, reduce estimation bias, provide accurate estimation results, and avoid spurious regression. The model is shown below (Equation 5):
Where
4.2.2 Mediation effects model
In order to test the mechanism of the role of digital village construction on the efficiency of rural land use, we use the mediating effect model to test. Referring to the research of Wen et al. (2004), it can be seen that there are many defects in a single test method, and on the basis of Baron, Sobel and other test methods, Use a new mediation effect test method. This method has obvious advantages, firstly, it has high statistical efficiency, and secondly, it can control the probability of the first and second types of errors. Secondly, better elucidate the intrinsic mechanisms among variables. The mediation effect model can help researchers reveal how independent variables affect the dependent variable through mediation variables, thereby gaining a deeper understanding of the complex pathways and underlying mechanisms between variables. Thirdly, enhance the explanatory power of the model. By introducing mediator variables, the model can better explain the mechanism of the independent variable’s influence on the dependent variable. The mediation effect model usually has higher explanatory power than models without mediation variables, and can provide more information to support research hypotheses. In this paper, we use the following model for the mechanism test (Equations 6, 7).
where
4.3 Data sources
Based on data availability, this paper selects the panel data of 30 provinces in China except Hong Kong, Macao, Taiwan and Tibet from 2010 to 2022. Among them, the data on the output value of agriculture, forestry, animal husbandry and fishery come from China Tertiary Industry Statistical Yearbook; the data on input-output variables and control variables come from China Statistical Yearbook and China Rural Statistical Yearbook, and the missing data are made up through the statistical yearbooks of each province and relevant statistical bulletins. In addition, the relevant data were deflated with 2010 as the base period and transformed into comparable variables in 2010.
5 Analysis of empirical results
5.1 Benchmark regression results
In order to test the impact of digital village construction on rural land use efficiency, the analysis is based on the baseline regression model constructed in this paper. Table 4, column (1) shows the regression results of digital village construction on rural land use efficiency when no control variables are added, and from the results, it can be seen that the coefficients of digital village construction are positive at 1% significance level, indicating that the digital village construction improves the efficiency of rural land use. After adding control variables in column (2), the coefficient of digital countryside construction is significantly positive at 5% significance level, and also digital countryside construction improves the efficiency of rural land use, and Hypothesis 1 of this paper can be verified.
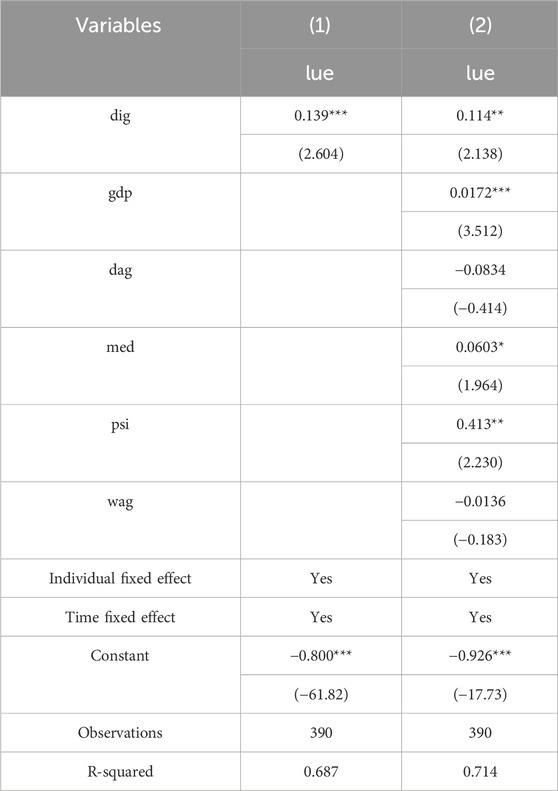
Table 4. Regression results of the impact of digital village construction on rural land use efficiency.
The construction of digital countryside realizes the large-scale operation of agriculture by improving the rural land transfer, while the main body of large-scale operation prefers to use digital technology to obtain new agricultural technology and promote the improvement of agricultural production efficiency. In addition, the information platform established by the digital countryside can provide timely market information, which is conducive to the optimization of the agricultural industrial structure, so that the agricultural production division of labor refinement and specialization, which is conducive to the improvement of rural land efficiency. At the same time, the construction of digital villages can optimize the allocation of agricultural resources and factors and promote the improvement of land efficiency. In addition, farmers can also accurately control the process of agricultural breeding, irrigation, fertilization and other processes through the network information platform of digital rural construction, saving capital investment and reducing pollution and carbon emissions. Therefore, digital rural construction has improved rural land utilization efficiency in multiple dimensions.
5.2 Endogeneity test
In order to mitigate the possible endogeneity problem of digital village construction and rural land use efficiency, this paper includes control variables as much as possible to prevent the endogeneity problem caused by omitted variables. The results of the benchmark regression show that digital village construction can improve rural land use efficiency, and in turn, an increase in rural land use efficiency may lead to an increase in the level of digital village construction, thus leading to bidirectional causality. Therefore, this paper deals with the possible endogeneity problem by lagging the core explanatory variables by one period as well as the instrumental variables approach.
5.2.1 Lagging phase I digital village construction
Considering that the construction of digital rural areas requires a certain amount of time and may have a certain time delay, the construction of digital rural areas will be lagged by one period as an instrumental variable, and the system GMM method will be used for testing,and the results are shown in column (1) of Table 5, the AR (2) test results show that there is no second-order autocorrelation problem, the Sargan test results show that there is no over-identification problem, and the estimation results using the GMM model are reliable. From the empirical results, the coefficients are significantly positive at the 5% level after one period of lagging digital village construction, indicating that digital village construction with one period of lagging still has a positive promotion effect on rural land use efficiency.
5.2.2 Instrumental variables method
Digital village construction needs the support of Internet technology. To alleviate the endogeneity problem of the model, referring to Daleng and Peng (2023), the Internet penetration rate in each province is selected as an instrumental variable for digital village construction. On the one hand, digital village construction relies heavily on regional Internet penetration rates, and regions with better Internet penetration rates are more likely to carry out digital village construction; on the other hand, Internet penetration rates have a negligible effect on rural land use efficiency. Therefore, the selection of Internet penetration rate as an instrumental variable for digital village construction satisfies the requirements of relevance and exogeneity. Table 5 columns (2) (3) show the regression results of instrumental variables. The first-stage regression results indicate that the coefficient of Internet penetration is significantly positive at the 1% level, indicating that Internet penetration is correlated with digital village construction, the value of Cragg-Donald Wald F-statistic is 67.74, which is much larger than 10, and the value of Kleibergen Paaprk LM-statistic is 75.18, with a p-value of 0.000, rejecting the weakly instrumental variables and the original hypothesis of unidentifiable variables, proving that the selected instrumental variables are valid. The results of the second stage regression indicate that the coefficient of digital village construction is significantly positive at the 1% level, which is consistent with the results of the benchmark regression.
5.3 Robustness tests
5.3.1 Replacement of explanatory variables
Considering that the evaluation system of digital village construction has different standards and there is no recognized and common evaluation system of digital village construction, in order to ensure the robustness of the digital village construction indexes constructed in this paper, this paper refers to the methodology of Han and Sun (2024), and reconstructs the evaluation indexes of digital village construction from the four dimensions of digital village environment, rural digitization, agricultural digitization, and farmers’ wisdom, and uses entropy weight method to carry out the construction. The evaluation indexes are shown in Table 6. The constructed digital village construction index replaces the index in the benchmark regression, and the empirical results are shown in column (1) of Table 7. The coefficient of the digital village construction index is significantly positive at the 1% level, which is consistent with the results of the benchmark regression and proves that the conclusions of this paper are robust.
5.3.2 Replacement of explanatory variables
In this section, rural land use efficiency is measured using the results of the SBM-Undesirable model (xleu) instead of the results of the Ultra-Efficient BM-Undesirable model, and the effect of digital village construction on rural land use efficiency is re-examined, and the results are shown in column (2) of Table 7, the digital village construction coefficient remains significantly positive at the 1% level, indicating that the results of this paper are robust.
5.3.3 Excluding municipalities
Since the four municipalities of Beijing, Tianjin, Shanghai, and Chongqing are not clearly delineated between urban and rural boundaries, and the situation is quite different from that of other provinces, and the agricultural function is not focused on production, and the digital infrastructure is more complete and strong, the four municipalities are excluded from the test and re-tested, and the results of the test are as shown in column (3) of Table 8, and the coefficient of the digital countryside construction is still significantly positive at the 5% level, which suggests that the results of this paper are robust.
5.3.4 Mechanism analysis
The theoretical analysis shows that the digital village construction can alleviate the resource mismatch problem, but what role the resource mismatch problem can play between the digital village construction and the rural land use efficiency needs to be further tested. For this reason, the mediation effect model is used to test it, and the empirical results are shown in Table 8. Columns (1), (3) and (5) show the empirical results of digital village construction on land resource mismatch (lan), capital resource mismatch (kap) and labor resource mismatch (lab), respectively. The empirical results show that column (1) shows that the coefficient of the impact of digital village construction on land resource mismatch is significantly negative at the 5% level, and the regression coefficient of land resource mismatch on rural land use efficiency is also significantly negative in column (2), thus proving that the land resource mismatch has a mediating effect between the digital village construction and rural land use efficiency. Similarly, column (3) shows that the coefficient of the impact of digital village construction on capital resource mismatch is significantly negative at the 1% level and the regression coefficient of capital resource mismatch on rural land use efficiency is also significantly negative in column (4), thus proving that capital resource mismatch has a mediating effect between digital village construction and rural land use efficiency. Column (5) shows that the coefficient of the impact of digital village construction on labor resource mismatch is significantly negative at the 10% level, and the regression coefficient of labor resource mismatch on rural land use efficiency is also significantly negative in column (6), thus proving that labor resource mismatch has a mediating effect between digital village construction and rural land use efficiency. Meanwhile, the coefficients of digital village construction on rural land use efficiency are positive in columns (2) (4) (6).
Further, this paper conducts relevant tests on the mediation model, using Bootstrap method and Sobel method to test the robustness of the model. In the Bootstrap method test, the land resource mismatch, capital resource mismatch and labor resource mismatch do not cover 0 under the 95% confidence interval. Meanwhile, in the Sobel test, the land resource mismatch, capital resource mismatch and labor resource mismatch all pass the significance test. Tracing back the root cause, from the perspective of land resource mismatch, digital village construction with the help of digital technology can carry out real-time monitoring in the whole process of land planning, use, management, monitoring, etc., which reduces the degree of land resource mismatch, promotes the improvement of total factor productivity in agriculture, and then promotes the improvement of rural land utilization efficiency. From the perspective of capital resource mismatch, the construction of digital villages is conducive to the construction of rural digital infrastructure, thus breaking through the limitations of time and space, which can better break the boundaries between urban and rural areas, promote the integration of the digital economy and the real economy, activate the original idle assets in the countryside, and promote the transformation of rural capital into digital capital, for example, the immersive experience of the online digital rural tourism experience. From the perspective of labor resource mismatch, digital rural construction makes the digital literacy of farmers engaged in the main body of agricultural production improve, which in turn leads to the improvement of the level of human capitalization of the rural labor force. Due to the penetration and substitution effects of digital rural construction, mechanized production is brought into agricultural production, increasing labor productivity and forming a gravitational demand for highly skilled personnel, which in turn reduces the degree of labor resource mismatch and improves the efficiency of rural land use. To sum up, digital rural construction forms a “booster” effect on rural land use efficiency by reducing the degree of land resource mismatch, capital resource mismatch and labor resource mismatch, and Hypothesis 2, Hypothesis 3, and Hypothesis 4 can be verified.
5.3.5 Heterogeneity analysis
In the baseline regression, we empirically test the average effect of digital village construction on rural land use efficiency, but China is a vast country, with large differences in economic development between the east, middle and west, and large differences between the north and south in terms of resource endowment, agricultural infrastructure and development level. Based on this, this section analyzes heterogeneity based on location differences, geographic location differences, food functional areas, and mismatch degree differences.
5.3.5.1 Heterogeneity analysis of the eastern, central and western regions
Based on China’s division into eastern, central, and western regions, we empirically test whether the construction of digital villages has a differential impact on rural land use efficiency in different regions. The eastern region includes Beijing, Tianjin, Hebei, Liaoning, Shanghai, Jiangsu, Zhejiang, Fujian, Shandong, Guangdong, and Hainan. The central region includes Shanxi, Jilin, Heilongjiang, Anhui, Jiangxi, Henan, Hubei, and Hunan provinces. The western region includes Inner Mongolia Autonomous Region, Guangxi Zhuang Autonomous Region, Chongqing Municipality, Sichuan Province, Guizhou Province, Yunnan Province, Shaanxi Province, Gansu Province, Qinghai Province, Ningxia Hui Autonomous Region, and Xinjiang Uygur Autonomous Region. The empirical results are shown in Table 9. Column (1) shows the regression results in the east, and the coefficient of digital village construction is significantly positive at the 5% level, while the coefficients of digital village construction in columns (2) and (3) are positive but non-significant, suggesting that digital village construction has a stronger role in promoting the efficiency of rural land use in the eastern region of China.
The construction of digital rural areas has had varying impacts on the efficiency of rural land use in different regions. We believe that there are three main reasons for the impact of regional differences. Firstly, there are differences in digital infrastructure. In the eastern region, the construction of digital infrastructure such as the Internet of Things and big data platforms is relatively complete. The improvement of these facilities provides efficient information processing capabilities for rural land use efficiency, which is conducive to the development of precision agriculture and smart agriculture. In the central and western regions, the construction of digital infrastructure is relatively lagging behind, which limits the speed of information transmission and processing, thereby affecting the intelligence level of rural land use efficiency. Secondly, there are differences in the degree of application of digital technology. In the eastern region, the use of digital technology in agricultural production is becoming increasingly widespread, such as intelligent irrigation, drone detection, precision fertilization, and so on. The application of these digital technologies has improved agricultural production efficiency, reduced resource misallocation, further lowered costs, and increased the sustainability of rural land use. In the central and western regions, the application of digital technology in agricultural production is relatively limited, and traditional agricultural farming methods still dominate. This will result in lower land use efficiency in rural areas compared to the eastern region, making it difficult to meet the needs of modern agricultural development. Thirdly, there are differences in economic development level and policy support. In the eastern region, the level of economic development is relatively high, and there is also strong government policy support, which leads to significant investment and support for the construction of digital rural areas. This is conducive to the innovative development and further improvement of rural land use efficiency in the eastern region. In the central and western regions, the level of economic development is relatively low, and the government’s financial pressure is high. The investment and support for digital rural construction are limited, which limits the promotion and application of digital technology in rural land use and affects the improvement of rural land use efficiency.
The construction of digital villages requires a good economic foundation and digital infrastructure environment, in comparison, the eastern region has obvious advantages in this regard, and the digital literacy of agricultural business farmers in the eastern region is higher, and the spillover effect is more obvious, and more colleges and universities and scientific research institutions are concentrated in the east, and the scientific research capacity is stronger, these conditions are favorable to the construction of the digital villages. Therefore, the construction of digital villages has a stronger effect on the eastern region and a relatively weaker effect on the central and western regions. However, on the other hand, the central and western regions can take advantage of the spillover and penetration effects of digital technology to obtain the “latecomer’s advantage,” gradually narrow the gap with the eastern region, and improve the efficiency of rural land use with the help of digital rural construction.
5.3.5.2 Heterogeneity between the south and the north
The previous empirical test shows that digital village construction has a heterogeneous impact on rural land use efficiency in east, central and west China, showing a certain degree of convergence. Then, the difference between the south and the north of China has become a new issue of regional unbalanced development, and the difference of regional economic development has become an important basic condition for the construction of digital villages. Therefore, this paper divides the south and the north into the south and the north according to the demarcation line of the south and the north “Qinling-Huaihe River.” The southern region includes 15 provinces, including Jiangsu, Anhui, Hubei, Hunan, Sichuan, Chongqing, Yunnan, Guizhou, Guangdong, Guangxi Zhuang Autonomous Region, Fujian, Zhejiang, Shanghai, Jiangxi, and Hainan. The northern region includes Beijing, Tianjin, Hebei, Shaanxi, Shanxi, Inner Mongolia Autonomous Region, Heilongjiang, Inner Mongolia Autonomous Region, Jilin, Liaoning, Ningxia Hui Autonomous Region, Henan, Shandong, Gansu, Qinghai, and Xinjiang Uygur Autonomous Region, totaling 15 provinces.
The regression results are shown in Table 10. Columns (1) and (2) show the regression results of digital village construction on rural land use efficiency in the southern and northern regions, respectively. From the results, the promotion effect of digital village construction on the efficiency of rural land use in the southern region has already appeared, while the promotion effect on the efficiency of rural land use in the northern region has not yet appeared.
The construction of digital rural areas has had differential impacts on the efficiency of rural land use in the south and north. We believe that there are three main reasons for the impact of regional differences. Firstly, the level of economic development in the south is stronger than that in the north. The construction of digital rural areas predates that of the north, and in terms of speed, the development of digital rural areas in the south is also faster than that in the north. According to the “Research Report on Taobao Villages in China (2009–2010),” in 2013, only seven provinces in China, namely, Zhejiang, Guangdong, Jiangsu, Shandong, Hebei, Fujian, and Jiangxi, had Taobao villages. In terms of proportion, the number of Taobao villages in the southern region reached 70%. By 2014, this proportion had reached 80.2%. From 2015 to 2016, the proportion of Taobao villages in the southern region had reached 82.9%. Although this proportion has decreased since 2016, it still remained at 75.1% in 2020, indicating that the construction of digital villages in the southern region has a first mover advantage and has a significant promoting effect on rural land use efficiency. For the northern region, it is necessary to continue to work hard to tap into the potential of digital rural construction and help improve the efficiency of rural land use. Secondly, there are differences in natural resources and resource endowments. In the southern region, the climate is warm and humid, which is more suitable for the growth of crops. Abundant water resources are conducive to irrigation and agricultural water use, and can improve the efficiency of rural land use. In the northern region, the climate is cold and dry, the agricultural production cycle is long, and it is greatly affected by seasonality. The relative scarcity of water resources limits irrigation conditions, which may affect the improvement of land use efficiency. Thirdly, there are differences in talent reserves and training. In the southern region, the talent reserve is more abundant, and the ability in digital literacy and innovation is stronger. There are numerous universities and research institutions, which are conducive to the research and promotion of digital agricultural technology. The government and enterprises attach great importance to talent cultivation and introduction, providing strong talent guarantee for the construction of digital countryside. In the northern region, talent reserves are relatively scarce, and digital literacy and innovation capabilities need to be improved. The number of universities and research institutions is relatively small, and the research and promotion capabilities of digital agriculture technology are limited. The government and enterprises do not attach enough importance to talent cultivation and introduction, which has affected the process of digital rural construction.
5.3.5.3 Heterogeneity of functional food areas
Guaranteeing food security is the primary task of the current modernization of agriculture and rural areas. Therefore, food functional zones have a pivotal position for China’s agriculture. Therefore, whether digital village construction has a heterogeneous effect on rural land use efficiency in food functional areas deserves further study. According to the division criteria of the Ministry of Agriculture and Rural Affairs, the sample is divided into main grain producing areas, main marketing areas, and balance areas. The main grain producing areas include Hebei Province, Shandong Province, Jiangsu Province, Anhui Province, Liaoning Province, Jilin Province, Heilongjiang Province, Inner Mongolia Autonomous Region, Jiangxi Province, Henan Province, Hubei Province, Hunan Province, and Sichuan Province. The main grain marketing area includes Beijing, Tianjin, Shanghai, Zhejiang, Fujian, Guangdong and Hainan provinces. The Grain Balancing Area includes Shanxi Province, Guangxi Zhuang Autonomous Region, Chongqing Municipality, Guizhou Province, Yunnan Province, Shaanxi Province, Gansu Province, Qinghai Province, Ningxia Hui Autonomous Region, and Xinjiang Uygur Autonomous Region.
The regression results are shown in Table 11. From the empirical results, the digital village construction has a significant promotion effect on the rural land use efficiency in the main grain production area and the main grain marketing area, while it has no significant effect on the rural land efficiency in the grain balance area. This shows that digital village construction has an unbalanced impact on grain functional areas, which also reflects the phenomenon of “digital divide” in China’s grain functional areas, especially the obvious difference between grain balanced areas and grain main production areas and grain main marketing areas.
The construction of digital rural areas has had a differential impact on the efficiency of rural land use in food functional zones. We believe that the reasons for the impact of regional differences are: firstly, the division of the three major functional zones for grain is the result of the adjustment of the relationship between people and land in each region (Guo and Liu, 2023), which has led to practical problems such as “transporting grain from the north to the south.” In this actual situation, it not only increases the environmental carrying capacity of the main grain producing areas in the north, but also poses certain challenges to the circulation efficiency and grain loss costs of the main grain selling areas. Due to the development of digital technology, the construction of digital rural areas can provide technical support for precise production, intelligent operation, and high-precision services of agricultural products, while improving the efficiency of rural land use, expanding production, and reducing unnecessary costs. Secondly, there are differences in cooperation and sharing mechanisms between regions. The cooperation and sharing mechanisms between different food functional zones can also affect land use efficiency. A sound regional cooperation mechanism has been established in the main grain producing and main grain selling areas, realizing the sharing and promotion of digital agricultural technology. Areas lacking cooperation mechanisms in the grain balance zone may face problems such as technological isolation and resource waste. The construction of digital rural areas requires information sharing and collaborative management. A unified spatial information platform has been established in the main grain producing and main grain selling areas, achieving information sharing and collaborative management among multiple departments. Areas with lower levels of information sharing in the grain balance zone may face problems such as information silos and low management efficiency. Therefore, Hypothesis 1 in this article is once again confirmed.
6 Conclusion and policy recommendations
China’s agricultural development has made rapid progress, and the total output of agricultural products as well as exports have been rising, but the food self-sufficiency rate has not been significantly increased, reflecting from the side that China’s rural land-use efficiency has not been effectively utilized, and that the growth of output of cultivated land area needs to be urgently improved. Therefore, how to empower rural land use efficiency through digital village construction is an important issue to realize steady growth of agricultural economy and improvement of land use efficiency. Different from what previous scholars have studied, this paper provides a feasible interpretation for promoting rural utilization efficiency from the perspective of digital village construction. In order to explain the core logic, firstly, the impact of digital village construction on rural land use efficiency is empirically examined; secondly, the mediating effect model is used to examine the mechanism played by the mismatch of agricultural resource factors; and lastly, the heterogeneous impact of digital village construction on rural land use efficiency is systematically analyzed from the perspectives of regional differences, south-north differences, and food functional areas.
This paper selects data from 30 provinces in China from 2010 to 2022, and after empirical analysis using fixed-effects model and mediated-effects model, it is found that, firstly, digital countryside construction improves rural land use efficiency, and the results still hold after endogeneity and robustness test; secondly, mechanism analysis finds that digital countryside construction can alleviate land resource factor mismatch, capital resource factor mismatch, and labor factor mismatch, which in turn promotes the improvement of rural land use efficiency; third, heterogeneity analysis finds that at the zoning level, digital rural construction promotes rural land use efficiency more strongly in the eastern region. At the geographic location level, digital village construction has a stronger role in promoting rural land use efficiency in the southern region. At the level of food functional areas, digital village construction has a stronger effect on the promotion of rural land use efficiency in the main food production areas and the main food marketing areas.
Based on the above findings, this paper has the following policy implications:
First, the empirical results indicate that digital rural construction is beneficial for improving the efficiency of rural land use. Therefore, regions need to continue to promote the construction of digital villages and deeply consolidate digital village infrastructure. In future development, it is necessary to build new infrastructure, attract more investment to the rural market, expand the rural coverage of 5G networks, promote the coverage and upgrading of rural power grids through the government, accelerate the “information into villages and households,” and improve the infrastructure of the digital countryside through a variety of ways. At the same time, according to the resource endowment of each region, we will create special agricultural products and establish a digital platform for production, processing and sales. Improving the efficiency of rural land use through digital technology can transform and upgrade agricultural development, improve total factor productivity, encourage the transfer of land resources in a variety of ways, promote the improvement of the efficiency of the scale of rural land, create a new form of agriculture, and improve the capacity of sustainable development for the construction of digital villages.
Second, the empirical results indicate that digital rural construction can further improve the efficiency of rural land use by alleviating resource misallocation. Therefore, in future development, it is necessary to deeply explore the mitigating effect of digital rural construction on the mismatch of resource and promote the improvement of rural land use efficiency. In terms of land elements, through digital technology to build up strength, actively improve the total amount of rural land resources, the proportion of agricultural cultivated area, the efficiency of rural land use and other deficiencies, to further improve the mechanism of rural land transfer, reduce the cost of land transactions, and make full use of rural idle land resources. In terms of capital elements, accelerate the integration and development of digital technology and idle rural resources, turn them to capitalization development, expand the coverage of digital rural construction in the agricultural industry chain, and rationally allocate agricultural resources. Open up the information channel between agricultural products and the agricultural market, shorten the realization cycle of agricultural capital stock as much as possible, introduce more capital into the rural market, alleviate the constraints of financing constraints on agricultural products, and optimize the efficiency of rural capital allocation. In terms of labor factors, through the digital information platform to increase the training of the rural labor force, efforts to improve the digital literacy of the rural labor force, with the help of digital technology to improve the ability of the labor force to use the land.
Third, the empirical results indicate that the construction of digital rural areas has heterogeneous effects on the efficiency of rural land use improve the non-equilibrium characteristics of digital rural construction caused by regions and differentiate the development of digital rural construction. In regions with first-mover advantages, such as eastern China, southern China, and the main grain-producing and main marketing areas, the dividends brought by digital technology should be steadily pushed forward, the layout of digital agricultural scenarios should be accelerated, the penetration effect of digital elements into the construction of the countryside should be improved, and the digital virtual economy should be deeply integrated with the real economy of agriculture, so as to create a digital information center of agriculture centered on land resources, and to play a leading role in the digital countryside construction. Role due to the non-equilibrium capacity of digital rural construction, in the future development, it also focuses on strengthening regional cooperation and exchanges, and rapidly promotes the synergistic development of digital rural construction. The central and western regions, northern regions and food balance areas make full use of the “east counts and west counts,” grasp the advantage of latecomers, and steadily promote and catch up with the regions with first-mover advantages, so as to make the digital village construction break the technological divide, promote the balanced development of various regions, build a cooperation platform between the regions with first-mover advantages and the regions with late-mover advantages, and narrow the digital divide between different regions. The digital divide between different regions will be narrowed. On the road of future agricultural construction, agriculture, processing industry and service industry will be connected, the cost of applying digital technology in villages will be reduced, and a new model of Chinese agriculture will be formed.
Finally, improve the regulatory system of digital village construction to reduce risks. A perfect regulatory system can reduce the unexpected risks in the construction of digital countryside, which is crucial for agricultural development and land use efficiency. The application of digital technology in accelerating the speed of information exchange and reducing information costs while distinguishing new features from traditional agricultural information, the need for targeted supervision based on the characteristics of digital rural construction, to ensure the efficiency of the application of digital technology and technical safety, and the establishment of data and information sharing mechanisms for digital rural technology. Rural land transactions have their own characteristics, and risks are very likely to occur when carrying out land transfers; therefore, it is necessary to establish an all-round supervision procedure for transactions before, during and after the event, in order to effectively improve the utilization efficiency of rural land.
Data availability statement
The original contributions presented in the study are included in the article/supplementary material, further inquiries can be directed to the corresponding author.
Author contributions
ZF: Conceptualization, Data curation, Formal analysis, Investigation, Software, Writing–original draft, Writing–review and editing. FG: Formal analysis, Funding acquisition, Methodology, Project administration, Validation, Writing–original draft, Writing–review and editing. CB: Data curation, Formal analysis, Supervision, Validation, Conceptualization, Writing–review and editing.
Funding
The author(s) declare that financial support was received for the research, authorship, and/or publication of this article. 1. Shandong Province Philosophy and Social Sciences Young Talents Team, Federation for the Humanities and Social Sciences in Shandong Province (Key project of think tank) (2023-zkzd-035) (Results of phased research). 2. Weifang Science and Technology Development Plan (Soft Science) Project “Mechanisms of the Empowerment of the Manufacturing Industry Supply Chain Resilience by the Digital Economy and Research on Enhancement Paths (2023RKX080).”
Conflict of interest
The authors declare that the research was conducted in the absence of any commercial or financial relationships that could be construed as a potential conflict of interest.
Generative AI statement
The author(s) declare that no Generative AI was used in the creation of this manuscript.
Publisher’s note
All claims expressed in this article are solely those of the authors and do not necessarily represent those of their affiliated organizations, or those of the publisher, the editors and the reviewers. Any product that may be evaluated in this article, or claim that may be made by its manufacturer, is not guaranteed or endorsed by the publisher.
References
Aimin, H., and Tan, J. (2022). The impact of digital village construction on the resilience of China's food system. J. South China Agric. Univ. Soc. Sci. Ed. 21 (03), 10–24.
Attour, A., and Barbaroux, P. (2021). The role of knowledge processes in a business ecosystem's lifecycle. J. Knowl. Econ. 12, 238–255. doi:10.1007/s13132-016-0395-3
Benqing, L. I., Qingxiang, ZHOU, and Yue, H. (2022). An empirical test of the impact of digital village construction on industrial prosperity. Statistics Decis. Mak. 38 (17), 5–10. doi:10.13546/j.cnki.tjyjc.2022.17.001
Brody, S. D., Carrasco, V., and Highfield, W. E. (2006). Measuring the adoption of local sprawl: reduction planning policies in Florida. J. Plan. Educ. Res. 25 (3), 294–310. doi:10.1177/0739456X05280546
Camarinha-Matos, L. M., Afsarmanesh, H., Galeano, N., and Molina, A. (2009). Collaborative networked organizations-Concepts and practice in manufacturing enterprises. Comput. & industrial Eng. 57 (1), 46–60. doi:10.1016/j.cie.2008.11.024
Chen, G., and Wang, G. (2020). A preliminary study on the economic model of “Box Village” under the environment of new retail. Agric. Econ. Issues 07, 14–24. doi:10.13246/j.cnki.iae.2020.07.002
Chen, Y. (2021). Mechanism innovation of digital economy and rural industry integration development. Agric. Econ. Issues 12, 81–91. Available at: https://kns.cnki.net/kcms2/article/abstract?v.
Chen, Z., and Zhang, X. (2022). Can digital rural construction alleviate agricultural resource factor mismatch? J. Agric. For. Econ. Manag. 21 (06), 736–743. doi:10.16195/j.cnki.cn36-1328/f.2022.06.79
Coelli, T. J., and Rao, D. S. P. (2004). Total factor productivity growth in agriculture: a Malmquist index analysis of 93 countries, 1980-2000. Agric. Econ. 32, 115–134. doi:10.1111/j.0169-5150.2004.00018.x
Daleng, X., and Peng, Z. (2023). Impact of the pilot policy of “Broadband China” strategy on urban green innovation. China Population-Resources Environ. 33 (09), 159–170. doi:10.12062/cpre.20230338
Du, J., Zhang, Y., Liu, B., and Dong, R. (2022). Impact of digital countryside on green total factor productivity in agriculture and its mechanism of action. China Population-Resources Environ. 33 (02), 165–175. doi:10.12062/cpre.20221013
Guo, H., and Liu, X. (2023). Can digital village construction enhance green total factor productivity in agriculture by alleviating the degree of resource factor mismatch. Ningxia Soc. Sci. (05), 107–117.
Han, C., and Zhang, Li (2019). Whether the Internet improves resource mismatch in China - a test based on dynamic spatial Durbin model and threshold model. Explor. Econ. Issues 12, 43–55.
Han, Z., and Sun, Xu (2024). Whether digital village construction can realize urban-rural basic public service equalization: mechanism analysis and empirical test. Mod. Finance Econ. Tianjin Univ. Finance Econ. 44 (09), 3–19. doi:10.19559/j.cnki.12-1387.2024.09.001
Hengzhou, Xu, Futian, Qu, and Guo, Z. (2011). Market failure, non-market value and excessive losses from de-farming of agricultural land: an empirical study based on different regions in China. Yangtze River Basin Resour. Environ. 20 (01), 68–72.
Hsieh, C., and Klenow, P. (2009). Misallocation and manufacturing TFP in China and India. Q. J. Econ. 124 (4), 1403–1448. doi:10.1162/qjec.2009.124.4.1403
Jiajia, Z., Juan, W., and Liu, T. (2023). Research on the impact and mechanism of digital village development on farmers' entrepreneurship. China Rural. Econ. 05, 61–80. doi:10.20077/j.cnki.11-1262/f.2023.05.004
Jing, Li, Fei, P., and Mao, D. (2012). Resource mismatch and total factor productivity of Chinese industrial enterprises. Finance Trade Res. 23 (05), 46–53. doi:10.19337/j.cnki.34-1093/f.2012.05.007
Kong, X., and Zhou, Z. (2020). China's rural factor market allocation reform history, basic experience and deepening path. Reform 07, 27–38.
Liao, J., Han, F., Zhang, W., and Xu, D. (2010). Impact of urbanization on land use efficiency in ChangZhuTan area. China Population-Resources Environ. 20 (02), 30–36. Available at: https://kns.cnki.net/kcms2/article/abstract?v.
Lin, H., Lu Ben, Z., and Hu, Y. (2023). Whether digital village construction can promote common prosperity in old revolutionary areas. China Rural. Econ. 05, 81–102. doi:10.20077/j.cnki.11-1262/f.2023.05.005
Lin, J., and Ma, X. (2004). Measurement of land use efficiency in Chinese urban agglomerations. Urban issues. 2014 (05), 9–14+60. doi:10.13239/j.bjsshkxy.cswt.140502
Long, H., Tang, G., Li, X., and Heilig, G. K. (2007). Socio-economic driving forces of land-use change in Kunshan, the Yangtze River Delta economic area of China. J. Environ. Manag. 83 (3), 351–364. doi:10.1016/j.jenvman.2006.04.003
Mary George, N., Parida, V., Lahti, T., and Wincent, J. (2016). A systematic literature review of entrepreneurial opportunity recognition: insights on influencing factors. Int. Entrepreneursh. Manag. J. 12, 309–350. doi:10.1007/s11365-014-0347-y
Peiwen, Bo, and Yang, Z. (2019). Factor mismatch and income distribution pattern in China's dual economy. Econ. 18 (02), 639–660. doi:10.13821/j.cnki.ceq.2019.01.11
Pereira, J., Tavalaei, M. M., and Ozalp, H. (2019). Blockchain-based platforms: decentralized infrastructures and its boundary conditions. Technol. Forecast. Soc. Change 146, 94–102. doi:10.1016/j.techfore.2019.04.030
Qi, W., Mingjie, L. I., and Jingbo, L. I. (2021). Digital rural empowerment and farmers' income growth:role mechanism and empirical test--A study on the moderating effect based on farmers' entrepreneurial activity. J. Southeast Univ. Philosophy Soc. Sci. Ed. 23 (02), 116–125+148. doi:10.13916/j.cnki.issn1671-511x.2021.02.014
Restuccia, D., and Rogerson, R. (2012). Misallocation and productivity. Rev. Econ. Dyn. 16 (1), 1–10. doi:10.1016/j.red.2012.11.003
Rongzeng, L., Yuexia, H., and Chun, He (2021). Role mechanism and empirical test of urban-rural high quality integrated development affecting land utilization efficiency. Urban Dev. Res. 28 (12), 128–136.
Shen, F., and Ye, X. (2021). Digital village construction: a strategic choice to achieve high-quality rural revitalization. J. Nanjing Agric. Univ. Soc. Sci. Ed. 21 (5), 41–53. doi:10.19714/j.cnki.1671-7465.2021.0071
Shen, Z., Boussemart, J. P., and Leleu, H. (2017). Aggregate green productivity growth in OECD's countries. Int. J. Prod. Econ. 189, 30–39. doi:10.1016/j.ijpe.2017.04.007
Silijuan, K. S. (2024). How does digital village construction affect rural residents' consumption? --An empirical analysis based on county matched data. Shanghai Econ. Res. 06, 62–82. doi:10.19626/j.cnki.cn31-1163/f.2024.06.004
Song, Z., Wang, C., and Bergmann, L. (2020). China's prefectural digital divide: spatial analysis and multivariate determinants of ICT diffusion. Int. J. Inf. Manag. 52, 102072. doi:10.1016/j.ijinfomgt.2020.102072
Sun, G., Yongfang, A. I., and Miao, L. I. (2021). Capital mismatch and the quality of China's economic growth: an empirical study based on the mediating effects of financial efficiency and capacity utilization. J. Manag. 34 (05), 57–73. doi:10.19808/j.cnki.41-1408/F.2021.0037
Tao, T., Fan, K., and Zhu, Z. (2022). Digital village construction and county industrial structure upgrading--a quasi-natural experiment based on the comprehensive demonstration policy of e-commerce into rural areas. China Circ. Econ. 36 (05), 3–13. doi:10.14089/j.cnki.cn11-3664/f.2022.05.001
Tone, K. (2022). A strange case of the cost and allocative efficiencies in DEA. J. Oper. Res. Soc. 53 (11), 1225–1231. doi:10.1057/palgrave.jors.2
Wan, J., and Wang, L. (2024). The impact of digital village development on rural household consumption upgrade. Consum. Econ. 40 (04), 87–102.
Wang, D., Peng, Z., and Lingyi, Li (2023). Measuring and evaluating the level of integrated development of digital economy and agriculture in China. China Rural. Econ. 06, 48–71doi. doi:10.20077/j.cnki.11-1262/f.2023.06.004
Wang, S. (2023). Research on the role mechanism, challenges and realization path of digital rural construction to promote the common wealth of farmers and rural areas. Nankai Econ. Res. 11, 21–36. doi:10.14116/j.nkes.2023.11.002
Wang, S., Na, Yu, and Fu, R. (2021). Digital village construction: role mechanism, realistic challenges and implementation strategies. Reform 04, 45–59.
Wang, Yu, and Zhang, Z. (2021). Digital economy, factor allocation and regional integration level, 05. Southeast Academic, 129–138. doi:10.13658/j.cnki.sar.2021.05.014
Wang, Z., and Yongxiu, B. (2022). Theoretical construction, empirical analysis and optimization path of coupling digital economy and rural revitalization. China Soft Sci. 01, 132–146.
Wen, T., and Chen, Y. (2020). Integration and development of digital economy and agricultural and rural economy:practice mode, realistic obstacles and breakthrough path. Agric. Econ. Issues 07, 118–129. doi:10.13246/j.cnki.iae.2020.07.011
Wen, Z., Zhang, L., Hou, J., and Liu, H. (2004). The mediation effect test program and its application. J. Psychol. 05, 614–620. Available at: https://kns.cnki.net/kcms2/article/abstract?v.
Wu, D., Mao, H., Zhang, X., and Huang, J. (2011). Evaluation of land use efficiency in Chinese cities. J. Geogr. 66 (08), 1111–1121. Available at: https://kns.cnki.net/kcms2/article/abstract?v.
Yang, K., Zhang, Yu, Xiaofeng, Z., and Zhong, T. (2010). Spatio-temporal characteristics and influencing factors of rural land use structural efficiency. Prog. Geoscience 38 (09), 1393–1402. doi:10.3969/j.issn.1002—2104.2010.02.006
Yin, H., Huo, P., and Wang, S. (2020). Digital transformation of agriculture and rural areas: reality characterization, influence mechanism and promotion strategy. Reform 12, 48–56.
Yin, Z., Wu, Z., Wang, R., and Guo, J. (2024). Has digital village construction promoted non-farm employment--an empirical analysis based on tracking data from fixed observation points in rural areas across China. Economist 06, 25–34. doi:10.16158/j.cnki.51-1312/f.2024.06.011
Yuan, Z., and Dongdong, X. (2011). Analysis of the impact of labor mismatch on TFP in China. Econ. Res. 46 (07), 4–17. Available at: https://kns.cnki.net/kcms2/article/abstract?v.
Zhang, J., and Zhang, X. (2020). A study on the impact and mechanism of Internet use on the decision to transfer agricultural land--Micro evidence from CFPS. China Rural. Econ. 03, 57–77. Available at: https://kns.cnki.net/kcms2/article/abstract?v.
Zhang, Y., Chen, J., Gao, J., and Jiang, W. x. (2019). The impact mechanism of urban land use efficiency in the Yangtze River Delta from the perspective of economic transition. J. Nat. Resour. 34 (06), 1157–1170. doi:10.31497/zrzyxb.20190603
Zhou, H., Sun, G., and Zhou, J. L. (2022). Can the digital economy narrow the multidimensional gap between urban and rural areas? --A resource mismatch perspective. Mod. Finance Econ. J. Tianjin Univ. Finance Econ. 42 (01), 50–65. doi:10.19559/j.cnki.12-1387.2022.01.004
Keywords: digital village construction, rural land use efficiency, land resource mismatch, capital resource mismatch, labor resource mismatch
Citation: Fan Z, Guo F and Bian C (2025) Digital rural construction, resource mismatch, and rural land use efficiency. Front. Environ. Sci. 13:1546082. doi: 10.3389/fenvs.2025.1546082
Received: 16 December 2024; Accepted: 13 January 2025;
Published: 03 February 2025.
Edited by:
Shikuan Zhao, Chongqing University, ChinaCopyright © 2025 Fan, Guo and Bian. This is an open-access article distributed under the terms of the Creative Commons Attribution License (CC BY). The use, distribution or reproduction in other forums is permitted, provided the original author(s) and the copyright owner(s) are credited and that the original publication in this journal is cited, in accordance with accepted academic practice. No use, distribution or reproduction is permitted which does not comply with these terms.
*Correspondence: Fenghua Guo, MTIwMTIwMDE3QGF1ZmUuZWR1LmNu