- 1Guiyang Institute of Humanities and Technology, Guiyang, China
- 2Binary University of Management and Entrepreneurship, Selangor, Malaysia
- 3Business School, Nanjing Normal University, Nanjing, China
- 4College of Economics and Management, Nanjing University of Aeronautics and Astronautics, Nanjing, China
- 5School of Politics and Economic Administration, Guizhou Minzu University, Guiyang, China
Digital economy is being closely integrated with agricultural development and tapping into its unique potential to alleviate agriculture’s carbon emissions To explore the mechanism of how digital economy reduce the agricultural carbon emissions, this paper constructs a systematic evaluation method with extend STIRPAT model and panel data drawn from 29 provinces (or municipalities and autonomous regions) in the Chinese mainland from 2013–2020. The results show that the development of the rural digital economy has a significant negative influence on agricultural CEs, and this result is still valid given robustness tests. Second, the alleviation of CEs based on the rural digital economy is more significant in the higher technological investment zones than that in the lower technological investment zones, and the central and eastern regions also have more significant CEs reduction effect. Third, the influence mechanism analysis shows that agricultural green technology change is an effective means to promote the rural digital economy’s CEs reduction effect. This paper not only provide new empirical evidence for understanding nexus between digital economy and agricultural carbon reduction, but also give constructive policy implication to improve agricultural green development.
1 Introduction
Alleviating carbon emission is receiving more and more attention globally (Ma S. et al., 2022). To maintain harmonious coexistence between humans and natures and realize the United Nations’ Sustainable Development Goals, Chinese central government pledged the global stakeholders that the Chinese people will try their best to have CEs peak before 2030 and achieve carbon neutrality before 2060, which demonstrates a strong determination to solve the problem of climate change. Activities of agricultural sector not only release CO2 but also hold carbon sequestration function, and the CEs and sequestration function make agricultural production activities have function of maintaining the carbon balance in the atmospheric. However, agricultural CEs have obvious spatial heterogeneity (Charkovska et al., 2019). Faced with issues such as global economic instability, rising energy demand, frequent adverse weather conditions, and expanding food demand (Fahad et al., 2022), the Chinese government should attach importance to cutting agricultural CEs. China is a large and longstanding agricultural country with widespread and extensive agricultural production activities. In the traditional agricultural production mode, the overuse of pesticides and chemical fertilizers, land ploughing and irrigation, as well as the problems of low production efficiency and unreasonable resource allocation in the agricultural production process, will directly or indirectly lead to more agricultural CEs and their higher intensity, thereby seriously restricting the development of low-carbon and high-quality agriculture. The 14th Five-Year Plan for National Agricultural Green Development emphasizes building an agricultural industry system with characteristic of green, low-carbon, and circular, while the 2023 Government Work Report further emphasizes the need to continuously improve the ecological environment and achieve low-carbon and sustainable development.
The digital economy plays an important role in promoting the full and balanced development between urban and rural areas, and its development has driven the economic development of agricultural and rural areas (Zhao et al., 2023). In China, the digital transformation of agriculture sector has shown initial results. According to the Information Center of the Ministry of Agriculture and Rural Affairs, the informatization level of national agricultural production in 2020 was 22.5% and the national level of agricultural product quality and safety traceability informatization was 22.1%. In 2021, the online retail sales agricultural production nationwide has reached 2.05 trillion Yuan, with growth rate of 11.3% compared to the level of the previous year. The construction of digital rural areas has been promoted extensively, with 117 digital rural pilot projects established nationwide, nine agricultural IoT demonstration provinces delineated, and 100 digital agriculture pilot projects established. Alongside these tremendous achievements, the digital economy has a positive impact on carbon emissions from agricultural production (Zhao et al., 2023). Thus, the problem is how to realize the coordinated relationship between them. Would the rural digital economy development bring fresh momentum to reducing agricultural CEs? Meanwhile, how can the rural digital economy empower the reduction of agricultural CEs? Exploring these issues has important practical value for the development of the rural digital economy and improving the reduction of agricultural CEs while also contributing to policy enlightenment in terms of achieving the great mission of China’s “Carbon Peak and Carbon Neutrality”.
The main contribution of this paper comparing to the existing literature are as following. First, we use the extend STIRPAT model to explore the influence mechanism of agricultural digital economy on the agricultural carbon emission. Second, the agricultural green production efficiency is used as a proxy for agricultural green technology change, which not only considering the quantity of the agricultural green development, but also capture the quality of agricultural green development. Third, this paper use three dimensions to measure the agricultural digital economy. Digital infrastructure in rural areas, digitalization of agriculture, and rural digital finance).
The rest of this paper is organized as follows. Section 2 is the literature review. The theory base and research hypnosis are showed in Section 3. Section 4 describes the models and data used in this paper. Section 5 analysis the estimation results. Section 6 gave the conclusion and presents the policy implications.
2 Literature review
So far, the relevant studies relating to CEs focus on the challenges faced by China in realizing its CEs reduction strategy and corresponding countermeasures. Hu (2021), OuYang (2021) and others have analyzed the severe challenges faced by China in realizing the goals of dual carbon strategy in terms of international and domestic perspectives, respectively. Liu et al. (2021) and others have analyzed the problems that exist in China in the context of carbon neutrality from on the viewpoint of energy structure, and have put forward countermeasures such as energy conservation and efficiency improvement, while accelerating the transformation and further promotion of energy structures. Adopting another approach, some scholars have conducted empirical analysis on the CEs reduction effect of the carbon trading pilot policy implemented by the Chinese government through the synthetic control method (Li et al., 2021; Yang et al., 2021), and have argued that China’s carbon trading pilot policy has played a significant role in the reduction of CEs, but there are problems such as insufficient market driving force for low-carbon innovation, poor pilot policy incentives, and regional heterogeneity. At the same time, Chen et al. (2016) have emphasized that increasing CEs reduces green total factor productivity (GTFP) based on studying the relationship between CEs and GTFP and economic development, and Wang et al. (2019) have also reached the same conclusion in relation to GTFP in agriculture economy development.
In addition, many researchers have devoted attention to agricultural CEs and carried out relevant research on the characteristics and calculation of agricultural CEs, agricultural CEs reduction policies, and influencing factors. Jin and other authors (2021) have explored the structural characteristics of China’s agricultural CEs, and drawn the conclusion that agricultural CEs in China have a phased upward trend alongside regional and provincial heterogeneity. In terms of policy research, Zhang et al. (2001) compared different environmental and economic instruments and argued that the environmental tax system has been more advantageous; Zheng et al. (2011) elaborated on a number of low-carbon special plans and proposed relevant recommendations, such as the establishment of a Chinese low-carbon agricultural model. Based on evolutionary game theory, Fan et al. (2011) suggested that government support and intervention can guide agricultural source farmers to choose CEs reduction strategies. In terms of influencing factors, the empirical studies of Xu et al. (2022a) and Xu et al. (2022b) have suggested that agricultural mechanization and the rural finance service have significant preventative effects on agricultural CEs. Furthermore, He et al. (2020) have discussed the status and role of green production efficiency in agriculture in various provinces.
The digital economy, a new engine of high-quality economic growth, has also attracted extensive attention and discussion in the academic community in recent years. On the one hand, there is research on the definition of the digital economy. Li et al. (2021a) characterize the digital economy on macro, meso- and micro-levels, asserting it includes four levels, namely, broad, middle, narrow and narrowest, and explored the mechanism and evolution process involved in data becoming a production factor (Li et al., 2021b). On the other hand, researches about digital economy are mainly about the comprehensive effect of digital economy, and they have put forward the argument that the digital economy can reduce environmental pollution (Deng, 2022), while driving high-quality urban development and promoting a specific economic pattern, which aim to coordinate development between regions (Zhao et al., 2020).
Especially since the strategy “Carbon Peak and carbon neutrality” was put forward, the relationship between the digital economy and carbon emission has become an important topic, and academia has also carried out extensive research (Yu et al., 2022). While researchers hold different conclusion on the nexus between digital economy and carbon emissions. Most studies show that the digital economy has improved the environmental situation, and provided impetus for emission reduction, Wang (2022) point out the digital economy is helpful to reducing the carbon emissions. Zhang (2022a) find that the digital economy plays a significant role in carbon emission reduction. They all conduct their research based on China’s urban data. However, some studies hold that the digital economy has a heterogeneous influence on CEs. Some scholars (Salahuddin et al., 2015; Avom et al., 2020) believe that, as the core foundation of the digital economy, the development of digital technology will lead to a large amount of power consumption and energy consumption, thereby increasing carbon emissions.
Furthermore, there are many researches focusing on the development of the digital economy in rural areas. According to theoretical analysis, the existing literature mainly pays attention to the mechanisms or development paths of the rural digital economy. Wang et al. (2021), Yin and others (2020) and others have explored the significance, practice mode and mechanism of the digital economy development in agriculture production and rural regions, and believe that it should be promoted by, respectively, accelerating the construction of rural digital infrastructure, promoting agricultural digitalization, and developing rural e-commerce. Some researches on digital inclusive finance (DIF) have argued that DIF can push the regional convergence of green economic growth while less developed regions experience a more significant convergence effect (Wang et al., 2022).
Many studies have also been carried out focusing on the influence of digital economy on CEs, mainly adopting the empirical analysis method with panel data based on province- or city-level contexts in China, and have found that digital economy growth can significantly alleviate the intensity of CEs (Xu et al., 2022; Guo et al., 2023), however, there exist certain regional differences (Miao et al., 2022; Xie, 2022).
A few researches have focused on the correlation between digital economy growth and agricultural CEs in China or foreign countries, and these literature mainly concentrate on the introduction of information and communications technology (ICT) into the field of smart agriculture, the promotion of sustainable agriculture, and the reduction of chemical use on the basis of embedding artificial intelligence (Patrício and Rieder, 2018), sensors (Basnet and Bang, 2018), robotics, and remote sensing technologies (Huang et al., 2018) into agricultural modernization processes. ICT, as a main focus of advanced technology trends, can promote comprehensive productivity efficiency, total factor efficiency (TFP) and agricultural sustainability (Dlodlo and Kalezhi, 2015). The prevalence of ICT not only promotes agricultural productivity and TFP, but also improves the progress of sustainable agricultural development. Ma S. Z. et al. (2022) focus on the nexus between the development of the agricultural digital economy and agricultural CEs; their conclusions emphasize that digital economy development inhibits agricultural CEs. In addition, advances in agricultural technologies, the optimization of agricultural industrial structure, and improvements in rural education all significantly inhibit the agricultural CEs in the research area. Adding to the influence factors outlined above, Zhang J. et al. (2022) emphasize that the development of DIF has significantly reduced agricultural CEs. Unlike other countries or regions, China’s agricultural digital economy mostly stresses the digital transformation of rural industrial models (Wu, 2021), agricultural industries (Zhao MJ. et al., 2022; Zhao YL. et al., 2022) and the effectiveness of the digital economy (Xie, 2020). These studies all pay attention to the innovative developments in digital agriculture (Wang et al., 2020). Through the systematic review of the literature outlined above, three main shortcomings can be found in the existing research: First, although many researchers have devoted attention to the correlation between the digital economy and CEs, more of them have studied this on urban level, and rarely extended this correlation to the rural development context, hence there is a lack of research that directly and empirically tests the correlations between the rural digital economy and agricultural CEs. Second, when analyzing heterogeneity, most existing studies only conduct sub-sample studies by region, and consider to a lesser extent the role of R&D in leading the high-quality development of the digital economy. Third, the path or mechanisms of the digital economy in rural areas in relation to the reduction of agricultural CEs is unclear, hence this requires further research. Considering the three points mentioned above, this article measures the intensity and amount of agricultural CEs, the progress in agricultural green technology and the development level of rural digital economy at a provincial level in China and tests empirically the nexus between rural digital economy and agricultural CEs. Meanwhile, this study not only examines the regional heterogeneity of the rural digital economy on agricultural CEs, it also analyzes the heterogeneity of this in relation to the science and technology investment level.
3 The mechanism and research hypotheses
The digital economy is an advanced economic mode with data as the important production factor and its development depends on the ability to obtain data. The establishment of a digital infrastructure not only realizes the utilization and transmission of data information, but also improves the efficiency of data circulation, thereby accelerating the process of digital infrastructure construction, the latter having become an indispensable foundation for the promotion of the growth of the digital economy. China has ascribed importance to the construction of digital infrastructure, and since 2018, the Politburo of the Central Committee has repeatedly stressed the need to accelerate the roll out and promotion of new digital infrastructure and its construction. At the same time, the construction of digital infrastructure is an important prerequisite for the integration of the digital and rural economies; whether it is agricultural informatization, agricultural product trading e-commerce, or the rural digital finance development, the prerequisite is it must be a complete rural digital infrastructure construction.
The reports of the China Academy of Information and Communications Technology believe that the definition of the digital economy can be divided into industrial digitization and digital industrialization, whereby industrial digitalization means the output and efficiency improvement brought about by the introduction of ICT into traditional industries. With the empowerment of digital technology, an environmental monitoring system for agricultural pre-production and production can be established, while new formats such as rural e-commerce goods can be formed after production, thereby realizing the transformation of traditional agriculture into a scientifically based and efficient modern model.
The integration of the digital and rural economies has improved the practice model of digital financial services in China’s “San Nong” field. The development of the digital economy has spawned updated financial models while the innovative development of digital finance has continuously added new momentum to the digital economy. The integration of ICT and traditional finance provides the possibility of opening up the farmers’ “last mile”. Furthermore, digital finance enables rural areas to address difficulties in accessing affordable financing at a low cost, fully leveraging the inclusive and the sharing advantages of digital finance, thereby contributing to the rural revitalization strategy while promoting the in-depth and comprehensive growth of the digital economy in today’s China.
Based on these insights, this article mainly explores the effect and mechanism of the rural digital economy growth level (explained from three aspects: rural digital infrastructure construction, agricultural digitalization, and development of the rural digital finance development) on agricultural CEs while also examining the intermediary effect of green technologies progress, which was measured by the agricultural green technological efficiency (see Figure 1).
3.1 Digital infrastructure in rural areas
Digital infrastructure as a foundation for the development of the digital economy plays an important role in realizing agricultural digitalization and rural digital finance. It contributes to promoting the deep development of the digital economy while limiting the digital economy’s CEs. The agricultural CEs reduction effect of rural digital infrastructure construction is mainly manifested in the following two aspects: First, rural digital infrastructure construction can guide residents in rural areas to form green environmental protection concepts. The development of ICT enables rural residents to accelerate their access to the online environment, understand news and public opinion related to environmental pollution, and develop green and environmental protection concepts, thereby promoting the formation of informal environmental regulations on the Internet (Xu, 2014) while helping to alleviate agricultural CEs and reshaping patterns of rural environmental governance. Second, the establishment of perfect rural digital infrastructures can reduce the limitations of geographical space, promote information interconnections and sharing, and help achieve a rational allocation of resources, thereby reducing the energy consumption caused by spatial and time factors in production and life, improving energy efficiency while unleashing CEs reduction effects.
3.2 Digitalization of agriculture
In terms of agricultural production management, the technology of big data analysis can promote the establishment of large-scale and standardized agricultural production bases, realize scientific analysis and reasonable predictions of crop sowing, output and demand, while reducing the imbalance between supply and demand and the waste of resources caused by insufficient and asymmetric information. In addition, through modern information processing technologies such as remote sensing satellites, real-time data collection, monitoring and analysis of agricultural production can be realized, and a scientific environmental monitoring system can be established so as to improve the allocation efficiency of production factor, grasp changes in the ecological environment, accurately measure CEs and trace them in time, thereby promoting effective governance and green development.
Digital technology can also continuously enrich the marketing methods of agricultural products, forming new sales models, i.e., rural e-commerce and live streaming. The continuous popularization of the rural Internet has connected farmers to online consumption cyberspace, realized “point-to-point” transactions, and reduced resource waste and CEs caused by the problems of information asymmetry and high transaction costs in traditional agricultural sales models. In terms of logistics and distribution, low-carbon logistics has become an important future development direction. The Vision 2035 Plan points out that green and low-carbon development should be promoted in the transportation industry while low-carbon freight logistics should also be realized. Aim to achieve development of the low-carbon logistics, relying on digital technology, the logistics and distribution industry is gradually replacing traditional fuel vehicles with clean energy electric vehicles, and accelerating the application of drones in rural areas for logistics distribution to reduce CEs. Regarding the latter, Jingdong drones have been used in some rural areas of Suqian City, Jiangsu Province, and this has already achieved normalized delivery (Lin et al., 2020). Relying on artificial intelligence technology can also promote the intelligence of agricultural product logistics systems, while the establishment of rural smart logistics information platform can optimize distribution routes, achieve resource intensification, continuously save costs, improve efficiency, and deepen the digital economy’s Carbon reduction effect.
3.3 Rural digital finance
The development of rural digital finance has promoted the establishment of rural environmental protection service platforms. Participation in environmental governance and other activities has effectively increased farmers’ enthusiasm for engaging in environmental protection and has helped to improve their sense of social responsibility (Meng et al., 2022; Dong et al., 2023). Taking the “Ant Forest” in Alipay’s personal carbon account platform as an example, users collect online energy and plant virtual trees to achieve real afforestation projects in reality, which attracts lots of subscribers to participate in environmental protection actions. In addition, it not only provides a sense of gain for the masses, but also promotes agricultural green development and reduces CEs. Furthermore, the rural environmental protection service platform built by relying on the digital finance development can also analyze the information of platform users through big data technology while rationally allocating resources, thereby reducing agricultural CEs. For example, Alipay’s “garbage sorting and recycling platform” is specially set up for problems such as the low recycling rate of domestic waste, supporting door-to-door collection of waste items so that the resource recycling rate is improved. Digital finance promotes green growth and green technological significantly (Wu et al., 2022; Razzaq and Yang, 2023). Mobile payment and online financial services can continuously reduce farmers’ dependence on financial institutions, not only reducing the transaction costs of paper money but also promoting the rational layout of financial business outlets, lowering resource consumption, while uniting both economic and environmental benefits.
In addition, digital finance can effectively compensate for the neglect of traditional finance in rural areas. In the traditional financial environment, farmers have difficulty in financing and own single source of funds, which is not conducive to introduce new agricultural technologies and form the extensive production methods, resulting in more agricultural CEs, hence more serious agricultural pollution problems. The promotion and application of digital finance has broadened the channels of farmers’ capital sources, assisted them to introduce efficient and low-carbon new agricultural technologies, and formed a green agricultural business model, thereby continuously reducing agricultural CEs’ intensity and promoting green agricultural development. Besides, digital finance can also alleviate the misallocation of financial resources and provide more career options for rural residents.
3.4 The progress of agricultural green technology
Generally speaking, a valuable way to achieve high-quality agricultural development is via green agricultural technological change (Deng et al., 2022).
In the existing agricultural economics research, more studies focus on green technological change or environmental technological change using different methods to assess agricultural green technology’s efficiency or that of environmentally friendly technology’s efficiency. According to the existing study on agricultural green technology change (AGTC) of China, the improvement of China’s agricultural productivity is overestimated due to ignoring the influence of environmental factors. Considering the regional heterogeneity of environmental conditions, agricultural technological change in rural China shows an increase trend, while there is a descending trend in the eastern, western, and central regions respectively. The northeast region has experienced an obvious decline in levels of technological change, while technological change without environmental constraints has exhibited a descending trend from eastern to western China (Jiang et al., 2022). He et al. (2021) have identified some important factors affecting agricultural green innovation efficiency, such as the level of agricultural technologies’ diffusion, absorption, implementation, and informatization, the amounts of agricultural extension workers, the average schooling of households, and levels of agricultural mechanization.
To estimate the green efficiency of agricultural production, Korhonen and Luptacik (2004) developed and extended the DEA considering environmental aspects. Existing literature usually through two ways to calculate the green efficiency, one is choosing the environmental factors as the inputs, the other is taking the environmental factors, especially the bad environmental results as bad outputs. The SBM-DEA taking account undesirable outputs is a widely used model to deal with economic and ecological issues (Liu et al., 2022). In this paper, we also chose the SBM-DEA model to estimate the agricultural green production efficiency, taking the carbon emission as the bad output in the DEA model.
In view of the above analysis regarding how the rural digital economy influences agricultural CEs, this article puts forward two research hypothesizes.
Hypothesis 1:. The rural digital economy may reduce the level and intensity of agricultural CEs significantly.
Hypothesis 2:. The rural digital economy may reduce CEs through green technological innovation efficiencies.
4 Research design
4.1 Constructing the modelling
The STIRPAT (Stochastic Impacts by Regression on Population, Affluence, and Technology) model initially proposed by Dietz and Rosa (1994) explores the factors influencing atmospheric emissions, such as socioeconomic, demographic, and technological issues. In the existing literature, the STIRPAT model mainly has been introduced to explore the causes of CEs in different industries, countries or cross-government economic organizations. These researches have concluded that certain factors such as rising population and affluence levels, the growth of urbanization, the structure of economic development and energy consumption as well as the energy mix and related technological issues are all responsible for increasing emissions. The STIRPAT model is in introduced in our study and is extended from a base IPAT model, which was initially proposed by Ehrlich and Holdren (1971). The advantage of this is that it allows for appropriate decomposition of population, technology, and wealth, while also adding other issues when analyzing environmental impact factors. The expression is:
where
The fixed-effects model can be used to control regional invisible differentiation, so the endogeneity issue generated by invisible or unchanging is addressed (Liu et al., 2024). Because of the advantages of fixed-effects, here we choose the fixed-effects model.
To effectively avoid the heteroscedasticity of the model, this article converts the terms in Equation 1 into their logarithms as follows:
where i indicates province; t indicates time;
Thus in our paper we choose certain control variables, including urbanization rate (
Digital agriculture is conducive to the green transformation of agricultural industry, meanwhile, the progress of green technologies can decrease the CEs level of agricultural production. Thus, the influence path of digital agricultural economy on CEs can be expressed as the following models, as shown in (3) to (5).
Here, Eq. 5 is the total effect model, Eq. 4 is the estimated model of the agricultural digital economy on agricultural green production efficiency, and Eq. 3 is the estimated model that considers both the agricultural digital economy and the mediating mechanism. Where, the mediator variable is the variable GTFP, the green agricultural production efficiency. The coefficient
4.2 Variable selection
1. Variable to be explained: Agricultural carbon intensity (AE). In this study, agricultural CEs intensity is chosen to measure the level of agricultural CEs in provinces. Agricultural CEs intensity is expressed by the ratio of total agricultural CEs to agricultural added value. The total amounts of agricultural CEs of each province were calculated from six dimensions: agricultural fertilizer, pesticide, farm PE film, agricultural diesel, tilling and irrigation (Li et al., 2011).
The CEs estimation formula is:
where variable
2. Core explanatory variable: Rural Digital Economy Development Index (ADIG). Based on the existing research, this paper selects 10 indicators such as rural Internet penetration rate and agricultural meteorological observation stations from the three aspects of rural digital economy infrastructure construction, agricultural digitalization, and rural digital services, and constructs an evaluation index system for the growth level of the digital economy in rural areas, as shown in Table 1. The Internet penetration rate in rural areas is assessed using the proportion of rural Internet broadband access users to the rural population in an area, while the number of Taobao villages is taken from the Ali Research Institute’s China Taobao Village Research Report,1 the DIF coverage breadth index is obtained from the digital inclusive financial index data of Peking University (Guo et al., 2020) measured by account coverage status, including the number of Alipay accounts per 10,000 people, the ratio of Alipay card users, and the average amounts of bank cards bound to an Alipay account. Other metric data is available directly. Among these, the average population served by postal outlets is a negative indicator while the others are positive indicators. In this research, the entropy method is introduced to measure 10 indicators of rural digital economy growth at three dimensions in order to get the rural digital economy development index of each province (city).
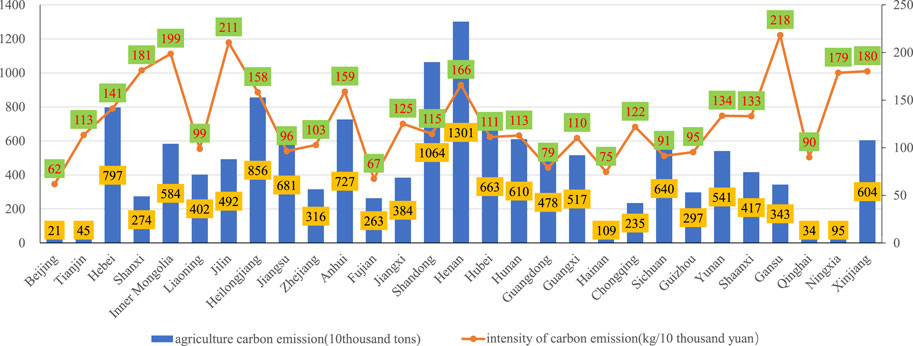
Figure 2. Average level of total agricultural CEs amounts and intensity in each province (city), 2013–2020.
The growth level of the rural digital economy in every province (city) in 2013 and 2020 are shown in Figure 3. It is found that there is significant heterogeneity in the growth level of the rural digital economy between different regions and different years.
3. Mediated variables: Green efficiency agricultural development (GE). In the existing literature, the total factor productivity (TFP) calculated by DEA-Malmquist index is always used to measure the technological change, while using the Malmquist index will sacrifice time information. Thus, this paper uses agricultural green technological efficiency with environmental constraints. In the DEA model of this paper, agricultural added value was defined as the good output, agricultural CEs constitute the bad output, meanwhile the sown area of crops, fixed capital investment and the agricultural workers were set as the input variables.
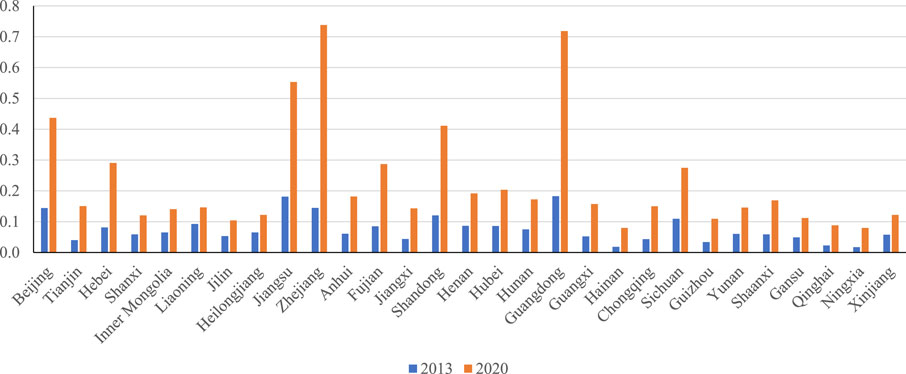
Figure 3. Comparison of comprehensive scores of rural digital economy development in 29 provinces (municipalities and districts) in China, 2013–2020.
From Figure 4, it is obvious that the green agricultural technological efficiency of less than half province is more than 1, which means that more than half of provinces have less efficient green agricultural technologies. Thus, for China, there is still more space to improve the green technologies. In this paper, we use GE to stand for green technological efficiency.
4. Control variables. Due to the complexity of factors influencing the agricultural carbon emission, considering only the impact of the agricultural digital economy on agricultural CEs might lead to bias, and even serious endogeneity issues. Therefore, the following variables are selected to ensure the comprehensiveness and accuracy of empirical analysis. Is complexity and variables: 1) Urbanization rate (URBAN), measured by the proportion of urban population in a region to total population in the same area; 2) The level of agricultural mechanization (MECH), expressed as the total power of agricultural machinery; 3) Planting structure (STRU), expressed as the ratio of the grain sown area to the crop sown area; 4) Agricultural chemical input intensity (CHEM), expressed as the ratio of fertilizer use to the crop sown area; 5) Traffic conditions (TRAN), expressed as the sum of railway operating mileage and highway mileage; 6) Rural electricity consumption (ELEC), expressed in terms of agricultural power generation. The above variables are logarithmic.
4.3 Data
Considering the availability of data, the Institute uses all data for 29 provinces (cities) in China from 2013–2020 (excluding Shanghai, Tibet, Taiwan, Hong Kong and Macao), which are derived from the China Statistical Yearbook (2014–2021)2 and China Rural Statistical Yearbook (2014–2021), the EPS data platform, the Ali Research Institute Report, and the Peking University Digital Inclusive Finance Index (2011–2020). The descriptive results for all variables chosen are shown in Table 2.
As shown in Table 2, except for lnELEC, all other variables have very small fluctuation trends, namely, less than 1.
5 Empirical results and analysis
5.1 Estimates of basic regression model
Firstly, only the core explanatory variable, namely, rural digital economy development composite score (ADIG) is considered, while the mixed-, fixed- and random-effects model is selected, and the F-test is 25.04 and the p-value is 0.0000, and the fixed-effect model should be selected. The Hausmann test shows that χ2 is 4.77 and the p-value is 0.029, choosing a fixed-effect model. The other control variables were then added, and mixed-, fixed-, and random-effects models were selected, and the F-test was 42.79 and the p-value was 0.0000, and the fixed-effect model should be selected. The Hausmann test showed that χ2 was 17.29 and the p-value was 0.0156, choosing a fixed-effect model.
Table 3 reports the baseline estimation of the influence effect of the rural digital economy development on the intensity of agricultural CEs. 1) considers only the core explanatory variable, and finds that the rural digital economy growth significantly reduces agricultural CEs intensity at the 1% level. Adding control variables to column 2), it is found that for every 1 unit increase in the growth level of rural digital economy, agricultural CEs intensity decreases by 40.01%, and this negative impact is still significant at the 1% level, thus validating the research hypothesis. For one thing, the development of the rural digital economy accelerates rural residents’ access to the network environment, not only promoting information interconnection and sharing while realizing the rational allocation of resources, but also helps rural residents establish the concept of green consumption and to develop informal network environment regulations, thereby reducing agricultural CEs intensity. And for another, the close combination of digital technology and agriculture helps farmers to, respectively, grasp agricultural production data accurately, improve production efficiency, and effectively reduce agricultural pollution caused by waste of resources. In addition, in an environment marked by the continuous development of rural digital finance, rural residents can broaden financing channels, introduce efficient and low-carbon new agricultural technologies, form a green business model, and promote the transformation of traditional extensive agricultural production methods to intensive ones, thereby realizing the agricultural CEs reduction effect of the rural digital economy.
5.2 Endogeneity test
To alleviate the impact of endogeneity on empirical results, this article also verifies the relationship between agricultural digital economy with a lag of one period and agricultural CEs, the results are in the column 3) in Table 3. The results of Table 3 have verified the negative impact of agricultural digital economy on agricultural carbon emissions. If the digital economy is an endogenous variable, then the estimation results in this paper are biased. This paper will test the core explanatory variable and each control variable with a lag of one period to overcome the possible reverse causal relationship between contemporaneous variables. The corresponding empirical results are shown in column 4) of Table 3. The regression results show that the coefficient of the core explanatory variable is −0.4564, with a p-value of 0.047, excluding the possibility that agricultural digital economy is an endogenous variable.
5.3 Robustness test
1. Replace the explanatory variable. In the baseline regression, the logarithmic form of agricultural CEs intensity was used as the explanatory variable. In order to further enhance the robustness of the conclusion, the dependent variable was replaced with the total amounts of agricultural CEs (logarithmic value) for robustness testing, and the results are shown in columns 1) and 2), Table 4. With the variables to be replaced, the growth of the rural digital economy still has a significant negative impact on agricultural CEs.
2. Exclude part of sampling. Considering substantial heterogeneity in the levels digital economy growth among Chinese provinces, in order to further strength the robustness of the conclusions, the data of two provinces with a digital economy scale of more than 15 trillion yuan and 12 provinces (cities) with a digital economy scale of more than one trillion yuan of 2020 are excluded. The results in column 3) and column 4) of Table 4 show that the development of rural digital economy still has a significant negative impact on agricultural CEs, and this negative impact has become stronger, which may be due to the fact that the digital economy in these provinces is on the rise, with accelerated development speed and greater development potential, so it is easier to reduce agricultural CEs intensity.
5.4 Heterogeneity analysis
1. Regional heterogeneity. This study categorizes the samples into four parts: eastern, central, western and northeastern regions for sub-sample regression, and discusses the regional heterogeneous impact of rural digital economy development on agricultural CEs intensity in the four parts. The estimations of regional heterogeneity analysis are shown in Table 5; for the eastern and central China, the development of rural digital economy still has a significant negative impact on agricultural CEs intensity and the central China have greater influence than their eastern counterparts while the western China is not significant. Possible explanations are: the eastern region has a good economic development foundation; the digital economy came early; it has a relatively complete rural digital economy infrastructure; and the integration and development of digital technology and agriculture is higher. Meanwhile, the central region is China’s most important agricultural production zone, the central government places greater focus on agricultural input, especially its green agricultural policy and finance support, which may lead to a larger and more significant negative impact on the intensity of agricultural CEs. The development and application of digital technology in the western region started late, that is might the reason why the impact is not significant. But it is not rational to deny its rapid upward phase and the low-carbon development potential of agriculture. The results also show that the coefficient of the rural digital economy development in the northeast region is positive, indicating that the development of the rural digital economy may increase the intensity of agricultural CEs. The development of the digital economy in northeast China is relatively backward, its digital infrastructure is not yet perfect, the coverage of rural digital finance is small, the proportion of secondary industry is large, while the integration of digital technology and agriculture is not complete.
2. Heterogeneity of scientific investment. As the primary productive and innovative force, the increased science and technology investment plays an important supporting role in the reduction of CEs and the growth of the digital economy. On the one hand, advances in science and technology have a direct impact on CEs’ reduction. At present, technological progress is an important driving force for the reduction of CEs and green development, while investment in science and technology helps to promote green technology innovations (Yang et al., 2019; Gu et al., 2022), saving production costs, promoting the professional division of labor in various fields, and improving productivity, thereby directly reducing CEs. On the other hand, the progress of science and technology will also promote the progress of digital technologies such as AI and big data, accelerating the development process of industrial digitalization and digital industrialization, thereby promoting the high-quality development of the digital economy, thus further reducing CEs.
To examine the impact of rural digital economy development on agricultural CEs’ intensity against the background of different scientific and technological inputs, this paper divides 29 provinces (municipalities) into high and low sample groups for heterogeneity analysis based on the average science and technology expenditures in each province (municipality) over 2013–2020, and the results are shown in Table 6. For the high-tech input group, the development of the rural digital economy still had a significant negative impact on the intensity of agricultural CEs, while the low-tech input group was not significantly negative. This shows that high scientific and technological investment can help promote the green development of agriculture while reducing the intensity of agricultural CEs. The development of the rural digital economy is premised on the completion and improvement of rural digital infrastructure as well as the production, transportation, sales of agricultural products, as well as the supervision, measurement, and traceability of CEs in the whole process of agricultural digitalization, which depends on sound digital infrastructure. High levels of investment in science and technology is conducive to promoting scientific and technological innovation and building a higher quality digital economy infrastructure, thereby providing the realization method and technical guarantee required for the close integration of digital technology and agriculture while promoting the reduction of agricultural CEs. At the same time, the continuous inflow of high-tech labor as a result of government investment in science and technology in the form of subsidies can enhance the level of local innovation, thereby promoting the sustainable and high-quality development of the digital economy and realizing the digital economy’s capacity to reduce CEs. Therefore, local governments should vigorously promote innovation-driven development strategies, increase financial support for science and technology, establish a sound incentive system, and encourage applied research and technological innovation in key fields. In addition, local governments can also increase the weight and proportion of indicators such as scientific and technological investment and their application in the government assessment index system, design a sound talent introduction system, and pay attention to cultivating high-quality talent (Bian et al., 2020), so as to achieve high-quality development and deepen the digital economy’s CEs reduction effects.
5.5 Mediated effect analysis
From above analysis, it is obvious that the digital economy development has ability to decrease the agriculture CEs intensity and amounts. Further to explore the influence mechanism of the digital economy development on the agriculture CEs, the model 3) and model 4) mentioned in Section 4.1 is run using Stata software. To directly and conveniently compare the mediating effects with the estimates of the basic model of digital economy influence on agricultural CEs’ intensity, the baseline regression results in Table 3 were listed again in column 1), Table 7. The dependent variable in column 2) is the mediator variable agriculture green efficiency (GE), while the explanatory variable focused on in this paper, agricultural digital economy (ADIG), is significantly positive, consistent with expectations. The dependent variable in column 3) is the agricultural CEs intensity (lnAE). After adding the mediating variable GE, the explanatory variable agricultural digital economy (ADIG) remained significantly negative at the 1% level, while the mediating variable agricultural green efficiency (GE) was significantly negative.
Comparing the results of Table 3 and Table 7, the coefficient
6 Discussion
6.1 The construction of agricultural digital economy indicators
Based on the existing researches, this paper mainly focuses on the three aspects of rural digital economy infrastructure, digitalization of agriculture and rural digital services to construct the indicator of agricultural digital economy. This indicator not only consider the hardware and software agricultural digital economy level, but also digital service level. In Zhao et al. (2023) study, the indicators of digitalization level mainly focus on two aspects of digital economy infrastructure and digital economy service level, while they choose the digitization levels to substitute the rural digitalization index. In our study, we use the agricultural digital economy, which is closely related to the development agriculture and rural areas, and can better reflect the digitization level of agriculture.
6.2 The main effect of agricultural digital economy on agricultural carbon emission
In the existing studies, the level of digitalization can significantly reduce the agricultural carbon emission (Zhao et al., 2023), although their research chose the carbon emission intensity of different agricultural sector, cropping and livestock sector respectively. Even in the city level or other sector of China, most studies also hold the same conclusion as our study, such as Wang et al. (2022), Zhang W. et al. (2022). And our study also support the carbon emission reduction effect of digital economy.
6.3 The mediating effect of agricultural digital economy on agricultural carbon emission intensity
Through the mediating effect analysis, it is obvious that the agricultural green production technology is an important mechanism for the development of the digital economy’s capacity to alleviate agricultural CEs. The same results are also evident in the research of Rong et al. (2023). They emphasize that green technology can effectively suppress agricultural CEs directly, which has significantly negative spatial spillover effects on agricultural CEs in both the short and long term. Except for the influence mechanism, Guo et al. (2023) underline that the role of agricultural green technology in reducing agricultural CEs is particularly dominant in the main grain-producing areas. Zhao et al. (2023) emphasis digitalization can reduce China’s carbon intensity by promoting the agricultural technological input. This can support our influence mechanism of agricultural digital economy on the agricultural carbon emission. Except for the agricultural technology inputs, Zhao et al. (2023) also emphasis the role of human capital level and urbanization rate. In our research we use the agricultural green production efficiency as the mediating variable, which both considering the input and output of agricultural technology, and considering the agricultural green transformation.
6.4 Discussion of heterogeneity in the impact of agricultural digital economy on the agricultural carbon emissions
In Zhao et al. (2023) study, the carbon reduction effect is slightly greater in the central and western regions than that in the eastern regions, which is slightly different with our results, one reason is the different research period, the former chose the 2006–2018, while we chose the 2013–2020, considering the fact China’s digital economy has entered a mature period since the year 2013, thus we choose the 2013 is more rational for agricultural digital economy. Other reasons such as the region and province chosen difference also would lead to the less reduction effect of west region.
7 Conclusion and policy implications
This study uses the data of 29 provinces (cities) in China from 2013–2020 in order to measure the intensity of agricultural CEs as well as the development level of rural digital economy in each province. On this basis, the influence of the development of the rural digital economy on agricultural CEs is empirically estimated. The results show that: 1) the development of the rural digital economy could significantly reduce the intensity of agricultural CEs, a conclusion which is still valid after robustness test such as replacing the explanatory variables and removing some samples. The overall environmental effect is 40.01%, which means the agricultural CEs would decrease 40.01% when the agricultural digital economy increase one unit, the direct effect of digital economy on the agricultural CEs reduction is 33.75%; 2) The alleviation of CEs based on the rural digital economy is more significant in the higher technological investment zones than that in the lower technological investment zones, and the central and eastern regions also have more significant CEs reduction effect. 3) The influence mechanism analysis shows that agricultural green technology change is an effective means to promote the rural digital economy’s CEs reduction effect, and the mediating effect is −6.26%, which means the agricultural CEs would decrease 6.26% for one unit agricultural digital economy increase, through mediating effect of the agricultural green technology. Based on the above conclusions, this article puts forward the policy recommendations as follows.
Firstly, continuously improve the level of agricultural digital economy. Including build a complete rural digital economy infrastructure, strength the agricultural digitalization and promote the agricultural finance service. Further promote the full coverage of rural Internet, accelerate the construction of rural 5G networks, realize the in-depth application of agricultural Internet, and establish a smart agricultural technology system. Accelerate information interconnection and sharing, build a unified Big Data platform for agricultural and rural development, and provide solid information infrastructure support for the rural digital economy and agricultural digitalization, so as to accelerate the agricultural CEs reduction effect of the rural digital economy. Besides, increase the accessibility and coverage of agricultural finance is crucial for the green transformation of agricultural industry. The agricultural green development balances the agricultural industry growth and the sustainability of the rural environment.
Secondly, focus on achieving the balanced the rural digital economy development in various regions and better effect of agricultural CEs reduction. On the one hand, it is necessary to strengthen the interconnection and information sharing of various regions while deepening cooperation to promote the establishment of data sharing platforms. On the other hand, it is necessary to raise financial investment in the central, western and northeast regions, implement coordinated and sustainable digital economy development policies in accordance with local conditions, strive to eliminate the digital divide between regions, and bring into play the CEs reduction effect of digital economy. Meanwhile, the central China and western China can also take the initiative to expand foreign cooperation, such as introducing information technology to empower agriculture through free trade zone cooperation, thereby giving full scope to local comparative advantages, hence accelerating the digitization transformation of agriculture (Guo, 2021) while realizing the coordinated the digital economy development between regions.
Thirdly, the government should pay attention to agricultural green development, because the agricultural carbon reduction effect of digital economy needs to be achieved through the mediating variable of agricultural green technology change. Considering the peculiarity of agricultural development, there is a need to increase financial support and incentives for science and technology, set up special funds to encourage agricultural green technology R&D and innovation levels, continuously strengthen the scientific and technological research and technology research capacity of low-carbon technologies, while promoting agriculture’s turn to low-carbon and green development.
8 Limitations
This paper has some shortcomings and can be further analyzed. The assessment of agricultural digital economy has consistently constituted an important issue and challenge in related research. Although this paper assesses the agricultural digital economy by establishing a novel evaluation framework, because of the availability and measurability of data, some regions and some indicators cannot be included in the evaluation system in this paper. Thus, there is still space to further improve the evaluation methodology in the future, to enhance the comprehensiveness and scientific rigor of the research. Furthermore, since the agricultural digitalization and CEs are highly influence by the grassroots government, the role of township-level government played in the agricultural green development and agricultural digital economy is very direct and important. While the related data on the grassroots government is relatively incomplete, which would not provide sufficient evidence for our study. If we would get enough data of township level government, we would conduct more comprehensive research in this area.
Data availability statement
The raw data supporting the conclusions of this article will be made available by the authors, without undue reservation.
Author contributions
HZ: Writing–original draft, Conceptualization, Funding acquisition, Investigation, Resources. KG: Conceptualization, Data curation, Formal Analysis, Methodology, Writing–original draft, Resources. ZL: Conceptualization, Funding acquisition, Investigation, Writing–original draft, Data curation, Formal Analysis, Methodology, Validation. ZJ: Data curation, Formal Analysis, Methodology, Project administration, Resources, Visualization, Writing–original draft. JY: Data curation, Formal Analysis, Software, Writing–review and editing.
Funding
The author(s) declare that financial support was received for the research, authorship, and/or publication of this article. This work was supported by Guizhou Planning Office of Philosophy and Social Science grant numbers 22GZQN28.
Conflict of interest
The authors declare that the research was conducted in the absence of any commercial or financial relationships that could be construed as a potential conflict of interest.
Publisher’s note
All claims expressed in this article are solely those of the authors and do not necessarily represent those of their affiliated organizations, or those of the publisher, the editors and the reviewers. Any product that may be evaluated in this article, or claim that may be made by its manufacturer, is not guaranteed or endorsed by the publisher.
Footnotes
1Taobao Village: The Alibaba Research Institute’s recognition criteria for “Taobao Village” mainly includes: 1) business premises (in rural areas, administrative villages are the unit); 2) sales scale (the annual sales volume of e-commerce reaches 10 million yuan); 3) scale of online merchants (the number of active online stores in this village reaches 100, or the number of active online stores reaches 10% of the local household size).
2The China Statistical Yearbook (2014) shows the development of whole economic and social status in the year 2013, the rest can be done in the same manner.
References
Avom, D., Nkengfack, H., Fotio, H. K., and Totouom, A. (2020). ICT and environmental quality in Sub-Saharan Africa: effects and transmission channels. Technol. Forecast. Soc. Change 155, 120028. doi:10.1016/j.techfore.2020.120028
Basnet, B., and Bang, J. (2018). The state-of-the-art of knowledge-intensive agriculture: a review on applied sensing systems and data analytics. J. Sensors 2018, 1–13. doi:10.1155/2018/3528296
Bian, Y., Wu, L., and Bai, J. (2020). Does the competition of fiscal S&T expenditure improve the regional innovation performance? ——based on the perspective of R&D factor flow. Public Finance Res. (1), 45–58. [In Chinese]. doi:10.19477/j.cnki.11-1077/f.2020.01.004
Charkovska, N., Horabik-Pyzel, J., Bun, R., Danylo, O., Nahorski, Z., Jonas, M., et al. (2019). High-resolution spatial distribution and associated uncertainties of greenhouse gas emissions from the agricultural sector. Mitig. Adapt. Strategies Glob. Change 24 (6), 881–905. doi:10.1007/s11027-017-9779-3
Chen, C. (2016). China’s industrial green total factor productivity and its determinants—an empirical study based on ML index and dynamic panel data model. Stat. Res. 33 (3), 53–62. [in Chinese]. doi:10.19343/j.cnki.11-1302/c.2016.03.007
Deng, R., and Zhang, A. (2022). Research on the impact of urban digital economy development on environmental pollution and its mechanism. South China J. Econ. (2), 18–37. [In Chinese]. doi:10.19592/j.cnki.scje.390724
Deng, Y., Cui, Y., Khan, S. U., Zhao, M., and Lu, Q. (2022). The spatiotemporal dynamic and spatial spillover effect of agricultural green technological progress in China. Environ. Sci. Pollut. Res. 29 (19), 27909–27923. doi:10.1007/s11356-021-18424-z
Dietz, T., and Rosa, E. A. (1994). Rethinking the environmental impacts of population, affluence and technology. Hum. Ecol. Rev. 1 (2), 277–300.
Dlodlo, N., and Kalezhi, J. (2015). “The internet of things in agriculture for sustainable rural development,” in 2015 International Conference on Emerging Trends in Networks and Computer Communications (ETNCC), Windhoek, Namibia, 17-20 May 2015, 13–18.
Dong, H., Zhang, Y., and Chen, T. (2023). A study on farmers' participation in environmental protection in the context of rural revitalization: the moderating role of policy environment. Int. J. Environ. Res. public health 20 (3), 1768. doi:10.3390/ijerph20031768
Dubey, A., and Lal, R. (2009). Carbon footprint and sustainability of agricultural production systems in Punjab, India, and Ohio, USA. J. Crop Improv. 23, 332–350. doi:10.1080/15427520902969906
Ehrlich, P. R., and Holdren, J. P. (1971). Impact of population growth. Science 171 (3977), 1212–1217. doi:10.1126/science.171.3977.1212
Fahad, S., Bai, D., Liu, L., and Baloch, Z. A. (2022). Heterogeneous impacts of environmental regulation on foreign direct investment: do environmental regulation affect FDI decisions? Environ. Sci. Pollut. Res. 29 (4), 5092–5104. doi:10.1007/s11356-021-15277-4
Fan, D., and Liao, J. (2011). Evolutionary game analysis of agricultural carbon emission reduction. Statistics Decis. 4 (1), 40–42. [In Chinese]. doi:10.13546/j.cnki.tjyjc.2011.01.013
Gu, H., Yang, W., and Chen, W. (2022). Effect of green technology innovation on urban carbon emission reduction. Acad. Explor. (3), 120–132. [In Chinese]. doi:10.3969/j.issn.1006-723X.2022.03.014
Guo, F., Wang, J., Wang, F., Kong, T., Zhang, X., and Cheng, Z. (2020). Measuring China’s digital financial inclusion: index compilation and spatial characteristics. China Econ. Q. 19 (4), 1401–1418. [In Chinese]. doi:10.13821/j.cnki.ceq.2020.03.12
Guo, K. (2021). A new path for solving regional differences in digital economy development from the perspective of opening to the outside world—taking free trade area as an example. Serv. Sci. Manag. 10 (6), 151–156. [In Chinese]. doi:10.12677/ssem.2021.106021
Guo, Z., and Zhang, X. (2023). Carbon reduction effect of agricultural green production technology: a new evidence from China. Sci. Total Environ. 874, 162483. doi:10.1016/j.scitotenv.2023.162483
He, P., Zhang, J., He, K., and Chen, Z. (2020). Why there is a low-carbon efficiency illusion in agricultural production: evidence from Chinese provincial panel data in 1997-2016. J. Nat. Resour. 35 (9), 2205–2217. [In Chinese]. doi:10.31497/zrzyxb.20200913
He, W., Li, E., and Cui, Z. (2021). Evaluation and influence factor of green efficiency of China’s agricultural innovation from the perspective of technical transformation. Chin. Geogr. Sci. 31 (2), 313–328. doi:10.1007/s11769-021-1192-x
Hong, M., Tian, M., and Wang, J. (2023). The impact of digital economy on green development of agriculture and its spatial spillover effect. China Agric. Econ. Rev. 15 (4), 708–726. doi:10.1108/caer-01-2023-0004
Hu, A. (2021). China’s goal of achieving Carbon Peak by 2030 and its main approaches. J. Beijing Univ. Technol. Sci. Ed. 21 (3), 1–15. [in Chinese]. doi:10.12120/bjutskxb202103001
Huang, Y., Chen, Z.-x., Yu, T., Huang, X.-z., and Gu, X.-f. (2018). Agricultural remote sensing big data: management and applications. J. Integr. Agric. 17 (9), 1915–1931. doi:10.1016/s2095-3119(17)61859-8
Jiang, Q., Li, J., Si, H., and Su, Y. (2022). The impact of the digital economy on agricultural green development: evidence from China. Agriculture 12, 1107. doi:10.3390/agriculture12081107
Jin, S., Lin, Y., and Niu, K. (2021). Driving green transformation of agriculture with low carbon: characteristics of agricultural carbon emissions and its emission reduction path in China. Reform 5, 29–37. [in Chinese].
Korhonen, P. J., and Luptacik, M. (2004). Eco-efficiency analysis of power plants: an extension of data envelopment analysis. Eur. J. Operational Res. 154 (2), 437–446. doi:10.1016/s0377-2217(03)00180-2
Li, B., Zhang, J., and Li, H. (2011). Research on spatial-temporal characteristics and affecting factors decomposition of agricultural carbon emission in China. China Popul. Resour. Environ. 21 (8), 80–86. [In Chinese]. doi:10.3969/j.issn.1002-2104.2011.08.013
Li, H., and Zhang, J. (2021a). Some understanding on definition of digital economy. Enterp. Econ. 40 (7), 13–22. [In Chinese]. doi:10.13529/j.cnki.enterprise.economy.2021.07.002
Li, H., and Zhao, L. (2021b). Data becomes a factor of production: characteristics, mechanisms, and the evolution of value form. Shanghai J. Econ. (8), 48–59. [In Chinese]. doi:10.19626/j.cnki.cn31-1163/f.2021.08.005
Li, Z., and Wang, J. (2021). Spatial emission reduction effects of China’s carbon emission trading: quasi-natural experiments and policy spillovers. China Population,Resources Environ. 31 (1), 26–36. [in Chinese]. doi:10.12062/cpre.20200907
Lin, Y., Lyu, J., and Jiang, Y. (2020). Research on optimization of drone delivery based on urban-rural transportation considering time-varying characteristics of traffic. Appl. Res. Comput. 37 (10), 2984–2989. [In Chineses]. doi:10.19734/j.issn.1001-3695.2019.07.0210
Liu, L., Zhang, L., Li, B., Wang, Y., and Wang, M. (2024). Can financial agglomeration curb carbon emissions reduction from agricultural sector in China? Analyzing the role of industrial structure and digital finance. J. Clean. Prod. 440, 140862. doi:10.1016/j.jclepro.2024.140862
Liu, S., Lei, P., Li, X., and Li, Y. (2022). A nonseparable undesirable output modified three-stage data envelopment analysis application for evaluation of agricultural green total factor productivity in China. Sci. Total Environ. 838, 155947. doi:10.1016/j.scitotenv.2022.155947
Liu, X., Cui, L., Li, B., and Du, X. (2021). Research on the high-quality development path of China’s Energy Industry under the target of Carbon Neutralization. J. Beijing Inst. Technol. Sci. Ed. 23 (3), 1–8. [in Chinese]. doi:10.15918/j.jbitss1009-3370.2021.7522
Ma, S., Li, J., and Wei, W. (2022a). The carbon emission reduction effect of digital agriculture in China. Environ. Sci. Pollut. Res. doi:10.1007/s11356-022-24404-8
Ma, S. Q., Dai, J., and Wen, H. D. (2019). Trade openness, environmental regulation and green technology progress: spatial Econometric analysis based on Provincial data in China. J. Int. Trade (10), 132–145. [In Chinese]. doi:10.13510/j.cnki.jit.2019.10.009
Ma, S. Z., He, G., and Guo, J. W. (2022b). Welfare effects of digital agriculture: deconstruction from the perspective of value re-creation and redistribution. Issues Agric. Econ. (5), 10–26. [In Chinese]. doi:10.13246/j.cnki.iae.2022.05.006
Meng, F., Chen, H., Yu, Z., Xiao, W., and Tan, Y. (2022). What drives farmers to participate in rural environmental governance? Evidence from villages in sandu town, eastern China. Sustainability 14, 3394. doi:10.3390/su14063394
Miao, L., Chen, J., Fan, T., and Lv, Y. (2022). The impact of Digital economy development on carbon emission: a panel data analysis of 278 prefecture-level cities. South China Finance 2, 45–57. [In Chinese]. doi:10.3969/j.issn.1007-9041.2022.02.004
Ouyang, Z., Shi, Z., Shi, M., Yang, D., Long, R., Zhou, H., et al. (2021). Challenges and countermeasures of “carbon peak and carbon neutrality”. J. Hebei Univ. Econ. Bus. 42 (5), 1–11. [In Chinese]. doi:10.14178/j.cnki.issn1007-2101.20210826.001
Patrício, D. I., and Rieder, R. (2018). Computer vision and artificial intelligence in precision agriculture for grain crops: a systematic review. Comput. Electron. Agric. 153, 69–81. doi:10.1016/j.compag.2018.08.001
Razzaq, A., and Yang, X. (2023). Digital finance and green growth in China: appraising inclusive digital finance using web crawler technology and big data. Technol. Forecast. Soc. Change 188, 122262. doi:10.1016/j.techfore.2022.122262
Rong, J., Hong, J., Guo, Q., Fang, Z., and Chen, S. (2023). Path mechanism and spatial spillover effect of green technology innovation on agricultural CO2 emission intensity: a case study in Jiangsu Province, China. Ecol. Indic. 157, 111147. doi:10.1016/j.ecolind.2023.111147
Salahuddin, M., and Alam, K. (2015). Internet usage, electricity consumption and economic growth in Australia: a time series evidence. Telematics Inf. 32 (4), 862–878. doi:10.1016/j.tele.2015.04.011
Wang, J., Luo, X., and Zhu, J. (2022). Does the digital economy contribute to carbon emissions reduction? A city-level spatial analysis in China. Chin. J. Popul. Resour. Environ. 20 (2), 105–114. doi:10.1016/j.cjpre.2022.06.001
Wang, L., Yao, H., and Niu, K. (2019). Carbon emission, green total factor productivity and agricultural economic Growth. Inq. into Econ. Issues (2), 142–149. [In Chinese].
Wang, S., Yu, N., and Fu, R. (2021). Digital rural construction: action mechanism, realistic challenge and implementation strategy. Reform 4, 45–59. [In Chinese].
Wang, X., Zhu, Y., Ren, X., and Gozgor, G. (2023). The impact of digital inclusive finance on the spatial convergence of the green total factor productivity in the Chinese cities. Appl. Econ. 55 (42), 4871–4889. doi:10.1080/00036846.2022.2131721
Wang, X. H., Zhao, B., and Wang, X. (2020). Research on digital agriculture model innovation based on the case of Net Ease Weiyang Pig. Issues Agric. Econ. (8), 115–130. [In Chinese]. doi:10.13246/j.cnki.iae.2020.08.009
Wu, M., Guo, J., Tian, H., and Hong, Y. (2022). Can digital finance promote peak carbon dioxide emissions? Evidence from China. Int. J. Environ. Res. public health 19, 14276. doi:10.3390/ijerph192114276
Wu, X. X. (2021). Research on the integration of digital economy and rural industry. Southwest Finance 10, 78–88. [In Chinese].
Xie, L. (2020). Rural digital inclusive finance innovation model analysis under the development of digital agriculture and rural areas. Agric. Econ. 11, 12–14. [In Chinese].
Xie, Y. (2022). The effect and mechanism of digital economy on regional carbon emission intensity. Contemp. Econ. Manag. 44 (2), 68–78. [In Chinese]. doi:10.13253/j.cnki.ddjjgl.2022.02.008
Xu, Q., and Zhang, G. (2022a). Spatial spillover effect of agricultural mechanization on agricultural carbon emission intensity: an empirical analysis of panel data from 282 cities. China Population,Resources Environ. 32 (4), 23–33. [In Chinese]. doi:10.12062/cpre.20220411
Xu, W., Mao, Y., and Qu, X. (2022b). Research on the impact of rural financial development on agricultural carbon emissions -- a case study of 17 provincial cities in Henan Province. Credit. Ref. 40 (7), 86–92. [In Chinese]. doi:10.3969/j.issn.1674-747X.2022.07.013
Xu, W., Zhou, J., and Liu, C. (2022). The impact of digital economy on urban carbon emissions: based on the analysis of spatial effects. Geogr. Res. 41 (1), 111–129. [In Chinese]. doi:10.11821/dlyj020210459
Xu, Y. (2014). Whether informal environmental regulation from social pressure constraints on China’s industrial pollution? Finance Trade Res. 25 (2), 7–15. [In Chinese]. doi:10.19337/j.cnki.34-1093/f.2014.02.002
Yang, L., Zhu, J., and Jia, Z. (2019). Influencing factors and current challenges of CO2 emission reduction in China: a perspective based on technological progress. Econ. Res. J. 54 (11), 118–132. [In Chinese].
Yang, X., Li, J., and Guo, X. (2021). The impact of carbon trading pilots on emission mitigation in China: empirical evidence from synthetic control method. J. Xi'an Jiaot. Univ. Sci. 41 (3), 93–104. [in Chinese]. doi:10.15896/j.xjtuskxb.202103010
Yin, H., Hou, P., and Wang, S. (2020). Agricultural and rural digital transformation: realistic representation, impact mechanism and promotion strategy. Reform 12, 48–56. [In Chinese].
York, R., Rosa, E. A., and Dietz, T. (2003). STIRPAT, IPAT and ImPACT: analytic tools for unpacking the driving forces of environmental impacts. Ecol. Econ. 46 (3), 351–365. doi:10.1016/s0921-8009(03)00188-5
Yu, Z. X., Liu, S., and Zhu, Z. C. (2022). Has the digital economy reduced carbon emissions? analysis based on panel data of 278 cities in China. Int. J. Environ. Res. public health 19 (18), 11814. doi:10.3390/ijerph191811814
Zhang, B. Y., Liu, J. Y., and Zhu, R. B. (2022a). Digital agriculture development: international experience, emission reduction effects and financial support: based on the case study on Chengdu. Southwest Finance (1), 28–39. [In Chinese].
Zhang, J., Lyu, Y., Li, Y., and Geng, Y. (2022b). Digital economy: an innovation driving factor for low-carbon development. Environ. Impact Assess. Rev. 96, 106821. doi:10.1016/j.eiar.2022.106821
Zhang, S., He, H., and Cao, J. (2001). Environmental policy innovation: discussion on implementing environmental tax in China. Acta Sci. Nat. Univ. Pekin. 37 (4), 550–556. [In Chinese]. doi:10.13209/j.0479-8023.2001.100
Zhang, W., Liu, X., Wang, D., and Zhou, J. (2022c). Digital economy and carbon emission performance: evidence at China's city level. Energy Policy 165, 112927. doi:10.1016/j.enpol.2022.112927
Zhao, L., Rao, X., and Lin, Q. (2023). Study of the impact of digitization on the carbon emission intensity of agricultural production in China. Sci. Total Environ. 903, 166544. doi:10.1016/j.scitotenv.2023.166544
Zhao, M. J., Shi, R., and Yao, L. Y. (2022a). Analysis on the goals and paths of carbon neutral agriculture in China. Issues Agric. Econ. 9, 1–11. [In Chinese]. doi:10.13246/j.cnki.iae.20220913.002
Zhao, T., Zhang, Z., and Liang, S. (2020). Digital economy, entrepreneurship, and high-quality economic development: empirical evidence from urban China. J. Manag. World 36 (10), 65–76. [in Chinese]. doi:10.19744/j.cnki.11-1235/f.2020.0154
Zhao, Y. L., Zhang, Z. W., Wei, L. H., and Luo, S. (2022b). Digital agriculture in the whole industry chain promotes industrial digital transformation and upgrading. Yunnan Agric. 3, 17–19. [in Chinese]. doi:10.3969/j.issn.1005-1627.2022.3.ynny202203007
Keywords: agricultural carbon emissions, agricultural green technology efficiency, rural digital economy, rural digital finance, digitalization of agriculture
Citation: Zhang H, Guo K, Liu Z, Ji Z and Yu J (2024) How has the rural digital economy influenced agricultural carbon emissions? Agricultural green technology change as a mediated variable. Front. Environ. Sci. 12:1372500. doi: 10.3389/fenvs.2024.1372500
Received: 18 January 2024; Accepted: 20 March 2024;
Published: 08 April 2024.
Edited by:
Haitao Wu, Beijing Institute of Technology, ChinaReviewed by:
Nawab Khan, Sichuan Agricultural University, ChinaPeng Jiquan, Jiangxi University of Finance and Economics, China
Copyright © 2024 Zhang, Guo, Liu, Ji and Yu. This is an open-access article distributed under the terms of the Creative Commons Attribution License (CC BY). The use, distribution or reproduction in other forums is permitted, provided the original author(s) and the copyright owner(s) are credited and that the original publication in this journal is cited, in accordance with accepted academic practice. No use, distribution or reproduction is permitted which does not comply with these terms.
*Correspondence: Jinna Yu, eXVqaW5uYTIwMDRAMTYzLmNvbQ==