- 1Department of Animal Science, Iowa State University, Ames, IA, United States
- 2Veterinary Diagnostic & Production Animal Medicine, Iowa State University, Ames, IA, United States
Animal health and feed intake are closely interrelated, with the latter being an important indicator of an animal’s health status. Automated sensors for dairy cattle have been developed to detect changes in indicators of health, such as decreased rumination or activity. Previous studies have identified associations between sensor measurements and feed intake. Thus, the objective of this study was to determine if health disorders impact the associations identified between sensors and dry matter intake (DMI), and to measure the impact of health disorders on DMI. A total of 934 cows with health disorders (lameness, mastitis, and other), of which 57, 94, and 333 cows had observations for a rumen bolus and one of two ear tags, were analyzed to determine how health disorders impact the association of sensors with DMI. Eleven sensor measurements were collected across the three sensors, including total and point-in-time activity, rumination time, inner-ear temperature, rumen pH and rumen temperature. Associations of health disorders and sensor measures with DMI were evaluated when accounting for systematic effects (i.e., contemporary group, parity, and days in milk) and energy sinks accounted for in determination of feed efficiency (e.g., milk production, body weight and composition). In order to determine if inclusion of health disorders or sensor measures improved model fit, model AICs were assessed. Health disorders were significantly associated with all sensor measurements (P< 0.0001), with the direction of association dependent on sensor measure and health disorder. Moreover, DMI decreased with all health disorders, with larger impacts observed in animals in third and higher lactations. Numerous sensor measurements were associated with DMI, including when DMI was adjusted for energy sink variables and health. Inclusion of rumen bolus temperature, rumination or activity with health data reduced model AIC when evaluating DMI as the dependent variable. Some sensor measures, including measurements of activity, temperature and rumination, accounted for additional variation in feed intake when adjusted for health disorders. Results from the study indicate that feed intake and sensor measures are impacted by health disorders. These findings may have implications for use of sensors in genetic evaluations and precision feeding of dairy cattle.
1. Introduction
Automated sensor and high-throughput phenotyping traits, such as milk spectral data, have recently been identified as associated with or predictive of feed intake (Dórea et al., 2018; Olijhoek et al., 2020; Martin et al., 2021; Siberski-Cooper et al., 2022). The objective of studying the relationships of these new technologies with feed intake is to develop new, portable, lower cost proxies (i.e., indicator traits) that are accurate and reliable, and will increase the information sources available to monitor individual animal feed intake. The application of sensor data for proxies in dairy cattle is desirable because many sensors are already used on farms, allowing for sensor data to be collected from large numbers of commercial animals, across a variety of management systems. Adding more feed intake data to existing prediction models, even from correlated proxy traits, is expected to subsequently improve the accuracy of predicting feed intake and efficiency for use in precision feeding and genetic evaluations (Siberski-Cooper and Koltes, 2021).
Automated sensor information and feeding behaviors were impacted by health disorders in previous studies. For example, Weigele et al. (2018) reported that activity measured by neck and leg mounted accelerometers was lower in the hour after feed was delivered or pushed up in lame animals compared to healthy animals. Furthermore, lame animals exhibit a difference in feeding time compared to sound animals (Thorup et al., 2016; Barker et al., 2018). Prior to the diagnosis of mastitis, King et al. (2018) observed that animals exhibited a decrease in rumination time measured with a collar device. Animals with mastitis have also been reported to exhibit disruptions in the circadian pattern of activities, including time spent resting, feeding, and standing in the alley (Veissier et al., 2017). Despite the known differences in feeding behavior due to health disorders (Bareille et al., 2003; Palmer et al., 2012; Fogsgaard et al., 2015), limited research has quantified the change in daily feed intake and feed efficiency due to health disorders. Further, in the current era of precision technologies, there are no estimates of the impact of health disorders on the relationship between feed intake and sensors. Understanding the relationship between sensors, health disorders and feed intake if sensor measurements are planned to be used for genetic evaluations and precision feeding strategies.
Recently, the United States implemented feed saved (FS) as a measure of feed efficiency in their genetic evaluation of Holstein cattle (Gaddis et al., 2021). The FS trait incorporates predicted transmitting abilities (PTAs) of two traits related to energy use: 1) residual feed intake (RFI) which accounts for stage of lactation, milk energy, and weight and the fluctuation in weight of an animal (Koch et al., 1963; Kennedy et al., 1993), and 2) maintenance requirements of the animal, measured as body weight composite (Gaddis et al., 2021). These variables account for a proportion of variation in feed efficiency (i.e., 14% of genetic variation based on heritability estimates; Gaddis et al., 2021). However, feed intake data collection is expensive and laborious, which limits the amount of data collected today. Additional feed intake, correlated traits (i.e., indicator traits) or information about other factors impacting feed intake (i.e., environment, management and health) could increase the amount of variation in feed intake explained or increase the accuracy of predicting the genetic merit. Therefore, due to the relationship between health and feed intake, consideration should be given to if health disorders should be accounted for in genetic evaluations of feed efficiency if sensors are used as proxies of feed intake.
The objectives of this study were to determine (1) the impact of health disorders on feed intake; (2) the association of health disorders with automated sensor measures, including data recorded via rumen bolus and two different types of commercially used ear tags; and (3) the impact of health disorders on the association of sensor measures with feed intake.
2. Materials and methods
2.1. Animal husbandry
The data used in this study were generated by projects approved by the Iowa State University Institutional Animal Care and Use Committee (IACUC), including the protocol 18-174 and those described in Hardie et al. (2017). A total of 934 Holstein cows, ranging in parity from first to sixth and 24 to 278 days in milk (DIM) at the start of data collection, were selected for use. Data were collected from 2013 to 2016 on 476 cows, and from 2018 to 2021 on 458 cows. Data were collected in 39 contemporary groups (CG), with cows grouped by barn location and study period. The CG defines a group of animals exposed to the same diet, feeding regimen, length of data collection, season (weather and climate), location in the barn (pen) and management conditions. The distribution of cows by parity and initial DIM can be found in Supplementary Figures 1 and 2.
Animals were housed at the Iowa State University (ISU) Dairy Farm in a free stall facility and received a total mixed ration (TMR) diet. The TMR consisted of corn silage, alfalfa hay, whole cottonseed, molasses, ground corn, soybean meal and hulls, dried distiller grains, and a mineral and protein mix. The majority of animals were milked three times a day (initial 30 CG), while others were milked twice daily (9 CG). The number of milkings per day was changed due to management decisions and to better reflect industry standards. Data were collected for 36 to 88 days, with length of the data collection period depending on CG.
2.2. Phenotype collection
For details regarding the collection of feed intake, milk production and composition, body weight and condition score traits, see Siberski-Cooper et al. (2022). Briefly, individual feed intake data were collected using the Calan Broadbent Feeding System (American Calan®, Northwood, NH). Animals were required to have a minimum of 10 days of feed intake data to be included in data analyses. Weekly feed samples were collected and analyzed for dry matter to determine the dry matter intake (DMI) of animals. Monthly composites of weekly samples were analyzed for nutrient content (i.e., protein, fiber, starch, fat, etc.) to ensure diet consistency. All feed samples were analyzed by Dairyland Laboratories, Inc. using near infrared technology (Arcadia, WI).
2.3. Health information
Animal health was monitored daily by farm and research workers, with cows determined to be needing care by ISU veterinary personnel. All health disorders were diagnosed on a daily basis by ISU veterinary staff following complete physical exams and results were entered into an electronic record keeping system (i.e., PCDart), from which data were obtained for analyses. In the absence of a diagnosed health disorder, animals were considered to be healthy. Health disorders included primarily mild mastitis (mastitis) and lameness (lame; diagnosed as a binary category), while limited observations of various injuries or respiratory illnesses occurred (grouped together and labeled as “other” illnesses within this study). Additionally, animals occasionally had multiple health disorders occur simultaneously, which were also classified as “other”. Health disorders were therefore classified as either mastitis, lameness or other. Mastitis was diagnosed as mild when an animal presented with minimal clinical symptoms (i.e., slight changes in milk appearance) but did not show signs of systemic infections (e.g., fever, inflammation of the udder, etc.), therefore they did not require treatment. Cows with mild mastitis remained in the research pen and were monitored for worsening symptoms. Clinical cases of lameness and other illnesses were observed, but treatments were such that the majority of cows remained in the research pen. When animals were diagnosed with severe clinical health disorders, we were unable to obtain feed intake data as cows were moved to another pen for treatment. Due to the fact that most disorders have effects on animals prior to clinical diagnosis and following diagnosis or final treatment (i.e., subclinical events; King et al., 2017; Aghamohammadi et al., 2018), a 7-day buffer period pre- and post-disorder was included surrounding all health disorders (i.e., the health disorder was considered to have started 7 days prior to clinical diagnosis and ended 7 days following diagnosis or final treatment).
2.4. Data collection, cleaning and summaries
Two ear tag technologies (ear tag 1: CowSense by Quantified Ag® [now SenseHub® Feedlot by Merck], https://sensehubfeedlot.com/; ear tag 2: Allflex Heatime® Pro+ by Merck [previously SCR], https://www.allflex.global/na/product/heatime-pro/) and a rumen bolus (smaXtec Premium Bolus, https://smaxtec.com/en/) were used to record activity, inner-ear temperature, rumen temperature, rumen pH, and rumination at varying frequencies. Specifically, ear tag 1 measured activity and inner-ear temperature using an infrared beam directed down the ear canal. Ear tag 2 measured activity and rumination via an accelerometer. Lastly, the rumen bolus measured cow activity, rumen pH and rumen temperature. All sensors utilized an accelerometer to determine activity, measuring movement in the X, Y and Z planes. Activity was analyzed as the average in a one-hour (ear tag 1), two-hour (ear tag 2) or ten-minute (rumen bolus) interval within a day, or as the daily total activity. Similarly, rumination was analyzed as a within day two-hour interval average or daily total. Finally, temperature was analyzed as a one-hour (ear tag 1) or ten-minute (rumen bolus) within day average, and rumen pH was analyzed as the ten-minute average within a day. Prior to placement of a rumen bolus in a cow, pH was calibrated per manufacturer guidelines. All other sensors and rumen bolus measures were standardized by the manufacturer prior to receiving the product. Studies beginning in 2018 (CG 27 and later) utilized at least one of the aforementioned sensors during the study period. Production traits collected include DIM, milk yield (kg/d), fat, protein and lactose yields (kg/d), metabolic body weight (body weight0.75; MBW; kg), body condition score (BCS; Elanco Animal Health, 1997), and DMI (kg/d).
Sensor data were cleaned to remove faulty sensor measurements. To do so, missing observations within a day were identified and removed, ensuring each interval of measurement had a non-missing reading. Then sensors with a calculated daily interval average or daily total of zero were removed, as this is biologically unrealistic. Next, the range for each sensor measure within a CG was determined for healthy animals. This was done to identify sensor failure, determined to have occurred when a daily sensor reading was outside three standard deviations from the mean for more than five consecutive days. Data were removed beginning three days prior to the start of sensor failure. Following data cleaning, cows were required to have at minimum ten days of sensor data to be included in statistical analyses.
2.5. Statistical analyses
Average daily values (by measurement interval) or daily total values were used for statistical analyses. Averages of daily ear tag 1 activity (CS1ACT) and temperature (CS1TEMP), rumen bolus activity (RBACT), rumen pH (RBPH) and temperature (RBTEMP), and ear tag 2 activity (SCRACT) and rumination (SCRRUM) were calculated in RStudio (RStudio Inc., Boston, MA). Total daily activity (all sensors) and rumination (SCRRUM) were also calculated by summing all measures recorded in a single day. The following models were fit using PROC GLIMMIX in SAS 9.4 (SAS Inst. Inc., Cary, NC).
2.5.1. Adjusted sensor measures (ASM)
Sensor measures were adjusted for the systematic effects of CG, parity and DIM. The model used was:
where the response variable, SMijk, is the daily average sensor measure; μ is the overall mean; CGi and Parj are the class effects of the ith contemporary group and jth parity and DIMk is the fixed effect of days in milk. The residual of the model, εijk, was considered to be the ASM.
2.5.2. Association of health and sensor measures
To determine if sensor measures significantly differed when animals where undergoing health disorders, the following model was used.
where the Healthi is health category (healthy, lame, mastitis or other); μ is the overall mean; ASMi is the fixed effect of the ith adjusted sensor measure; and εij is the random residual associated with Healthi. The model was applied a total of eleven times with one sensor measure included at a time. Least squares means (LSM) of sensor measures for each of the four categories of health disorders were also estimated.
2.5.3. Association of sensor, health, and feed intake data
Differences in DMI between animals when healthy and when diagnosed with a health disorder were assessed using model [3]. Building on this model, ASM was included to determine if sensors account for variation in feed intake previously accounted for by animal health (model [4]). Model [5] was used to determine the impact of adding health and ASM to the model, above the variables typically used to evaluate residual feed intake,
where dry matter intake is the response variable; μ is the overall mean; Healthi is the class effect of health classification (healthy, lame, mastitis or other); CG and Par are the class effects of contemporary group and parity (classified as 1, 2 or 3+); ASMj is the fixed effect of adjusted sensor measure; MYj, Fatj, Protj and Lactj are the fixed effects of milk yield, fat, protein and lactose in kg, respectively; MBWj, BCSj and DIMj are the fixed effects of metabolic body weight, body condition score and days in milk; and ε is the random residual associated with DMI. As in previous models, a single sensor measure was included at a time, thus eleven total models were fit. Based on previous results in which activity was significantly associated with feed intake only when rumination was also included (Siberski-Cooper et al., 2022), models [3] and [4] were run including both SCR measures (i.e., activity and rumination) simultaneously. This finding was consistent with the fact these sensors use accelerometer data to report both activity and rumination. With each model, the LSM of dry matter intake were determined for each of the four health classifications to determine the impact of health disorders on daily DMI. Finally, to examine if health disorders or sensor measures impacted the modeling of feed intake, the AIC of models [3], [4], and [5] were compared.
3. Results
A summary of the descriptive statistics for the traits and model covariates recorded during the study are presented in Supplementary Table 1. Included are summary statistics of DIM, milk yield, fat, protein and lactose yields, BW, BCS, and DMI. Tables 1, 2 report frequencies of each class of health disorder, number of cows documented with each type of health disorder, and the number of days of DMI data that were classified within a health disorder for the complete dataset and the dataset with automated sensor measures, respectively.
3.1. Association of health disorders with sensor measures
Health disorders were significantly associated with all adjusted sensor measures (P< 0.0001). The LSM of adjusted sensor measures for each health disorder are presented in Table 3. Adjusted activity, whether measured as the sensor interval daily average or as a daily total, significantly differed between healthy animals and those categorized as lame or other (P< 0.05). Regardless of the sensor used, adjusted activity was lower in ‘other’ animals than in healthy animals. When the activity was measured with either of the two ear tag technologies, lame animals had reduced activity compared to healthy animals; however, when measured by the rumen bolus, lame animals had increased activity. Adjusted activity of animals with mastitis was not different from activity of healthy animals, with the exception of total CS1ACT, for which mastitis animals had higher overall activity (healthy: 2875.28 ± 1267.85 activity units vs. mastitis: 5108.54 ± 1267.85 activity units; P< 0.0001). Adjusted ear temperature (i.e., CS1TEMP) was lower in lame (-0.670 ± 0.093°C) and ‘other’ (-1.048 ± 0.072°C) animals compared to healthy animals (0.033 ± 0.014°C). Adjusted temperature of the rumen (i.e., RBTEMP) was not different between healthy and lame animals but was higher in animals with mastitis (healthy: 0.00 ± 0.01°C vs. mastitis: 0.12 ± 0.02°C) and lower in animals categorized as other (-0.06 ± 0.02°C). The adjusted rumen pH of animals in the lame or mastitis categories was higher than healthy animals, whereas the ‘other’ animals had lower RBPH. For all health categories, adjusted rumination time was lower than that of healthy animals, irrespective of if rumination was the sensor interval daily average or the daily total. The LSM estimate of adjusted rumination time was most different for the animals classified with ‘other’ illnesses, whereas the LSM for lame animals was the closest to that for the healthy animals.
3.2. Association of health disorders with feed intake
Health disorders were significantly associated with DMI (P< 0.0001) when simultaneously accounting for CG, parity, and DIM. Using model [3], the decrease from healthy in LSM estimates of DMI for each health categories are provided in Figure 1. The DMI of each non-healthy category was significantly lower than the healthy DMI. The lame category had an estimated DMI 0.78 kg/d lower than healthy, followed by mastitis (1.48 kg/d) and other (1.98 kg/d). The addition of health in model [3] resulted in a slight reduction of AIC (Figure 2).
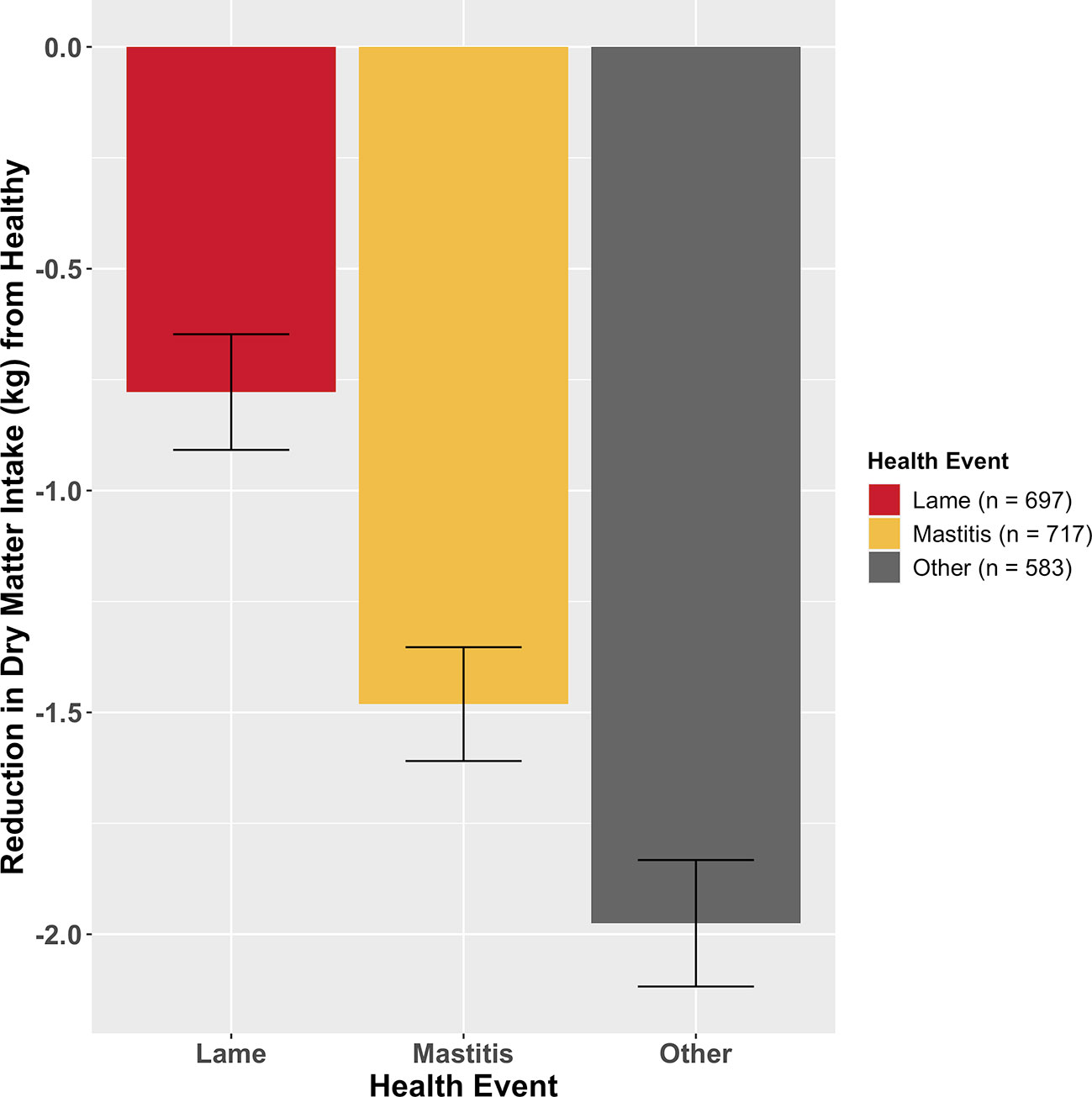
Figure 1 Reduction in least squares means of dry matter intake (DMI) for health disorders compared to cows considered healthy when accounting for systematic effects (i.e., contemporary group, days in milk, and parity; Model [3]). All reductions in DMI from healthy were significant at P< 0.0001. Importantly, the mastitis category only includes mild cases. The other category included disorders with minimal observations, including respiratory illness, injury, and multiple concurrent health disorders.
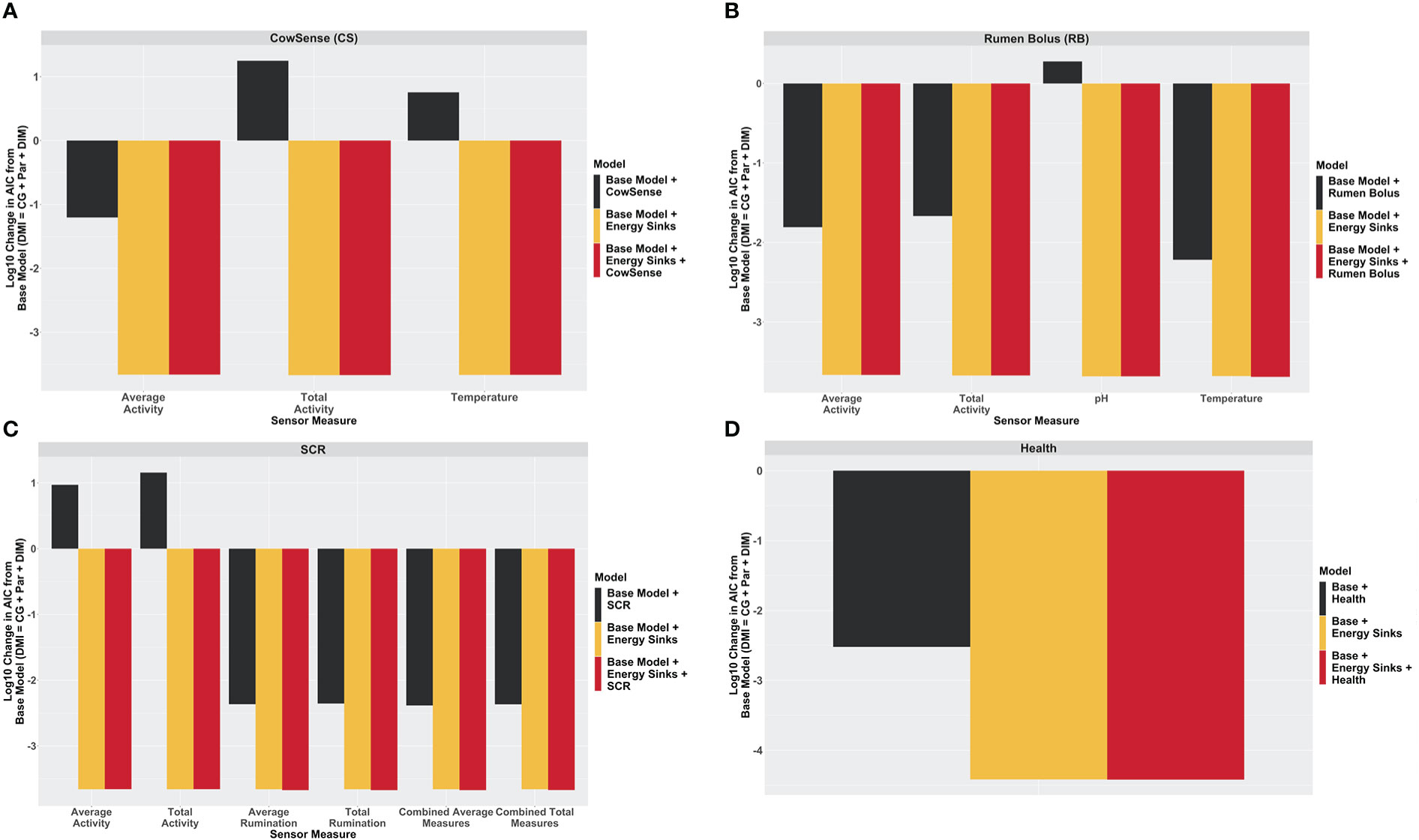
Figure 2 Evaluation of the impact of health disorders and sensor measures on model fit when estimating dry matter intake (DMI). Changes in Log10 AIC were compared to a “base model” (dry matter intake = contemporary group + parity + days in milk). Lower (more negative) values indicate a larger improvement in model fit as a result of the additional variables in the model. (A–C) Evaluate the impact of sensor measurements on model fit, while panel (D) evaluates the impact of health disorder information model fit. (A) Models estimating DMI including CowSense (CS) ear tag measures. A total of 93 cows had CS data, for a total of 4242 – 4331 days of data, depending on the measure. (B) Models estimating DMI including SmaXtec rumen bolus (RB) measures. A total of 56 cows had RB data, for a total of 2745 – 2885 days of data, depending on the measure. (C) Models estimating DMI including SCR ear tag measures. A total of 330 cows had SCR data, for a total of 10,284 days of data for all measures. (D) Models estimating DMI including health disorders. A total of 930 cows had health data, for a total of 45,023 days of data.
3.3. Association of health disorders, sensor measures and feed intake
Regardless of the ASM included, health was always significant (P< 0.0001) in models [4] and [5]. The majority of sensor measures were also significantly associated with feed intake (Supplementary Tables 2 and 3). Ear temperature (CSTEMP) was not associated with feed intake in either model, while SCRACT (daily total or interval average) was only associated with feed intake when SCRRUM was also included in the model. The estimated effect sizes for adjusted sensor measures in model [4] ranged from 2.02E-06 ± 0.00 kg/activity unit to -3.38 ± 0.26 kg/°C, with the smallest effect being for CSACT and the largest for daily total RBACT, respectively. Effect sizes of adjusted sensor measures were typically slightly smaller when energy sink variables (i.e., variables related to the energy demands of a lactating cow; milk weight, components, MBW and BCS) were included in the model (i.e., model [5]). The range of estimated effect sizes in model [5] were 1.37E-06 ± 0.00 kg/activity unit to -3.47 ± 0.32 kg/°C for daily total CSACT and RBTEMP, respectively (Supplementary Table 3).
Changes in AIC from a base model (i.e., model [3]) due to the inclusion of sensor measures and/or energy sinks are presented in Figure 2. The addition of SCRRUM to models resulted in the largest improvement in AIC, with a considerable reduction regardless of whether only systematic effects were included (i.e., model [4]) or energy sinks were also included (i.e., model [5]). The addition of RBACT or RBTEMP also resulted in a slight improvement in AIC. All other sensor measures had little effect on the model AIC.
4. Discussion
Sensors and milk spectral data have previously been associated with feed intake, which indicates they could be used as indicators of feed intake in commercial dairies given their widespread use (Dórea et al., 2018; Martin et al., 2021; Siberski-Cooper et al., 2022). The objectives of this study were designed to determine how sensor measures may be influenced by health disorders, as this could impact how the sensors are used in genetic prediction or precision feeding models. Differences in automated sensor data from animals undergoing lameness or mastitis have been reported in the days leading up to diagnosis of or during diagnosed health disorders, compared to healthy animals (De Mol et al., 2013; Thorup et al., 2016; Veissier et al., 2017; Barker et al., 2018; King et al., 2018; Gusterer et al., 2020). For example, lame cows were found to decrease in average daily feeding duration (Barker et al., 2018) and cows with mastitis had higher activity throughout the day (Veissier et al., 2017). Thus, when considering sensor measurements as potential indicator traits of feed intake, accounting for health is an important consideration. In this study, sensor measures significantly differed as a function of health disorders, different health disorders impacted the association of sensors with DMI, and model fit was improved when health disorders were included in models of DMI. Notably, after accounting for energy sinks and health disorders in the current study (i.e., model [5]), nearly all sensor measures were still significantly associated with DMI, which indicates that sensors account for variability in DMI other than that related to health (i.e., beyond health disorders).
4.1. Health disorders were associated with differences in sensor measurements
Sensor measurements were found to significantly differ between health disorders (P< 0.0001) and could record physiological and behavioral changes associated with health disorders. Activity was reduced in lame animals when measured via the ear tags but was higher when recorded via the rumen bolus. It may be possible that this reduction in activity from the ear tag is more drastic than results show, as increased head motion is associated with lameness (i.e., head bobbing; Schlageter-Tello et al., 2015). Reduction in activity under lameness conditions has been observed in previous studies (O’Callghan et al., 2003) and would align with common industry perceptions. The increase in rumen bolus activity may be due to the fact that data are preprocessed by the company for unknown factors through proprietary algorithms. Additionally, future research should examine the impact that increased cow activity related to estrus and how this may impact sensor associations. As expected, compared to animals characterized as healthy, rumen pH was significantly higher in the lame and mastitis groups and lower in the ‘other’ group. This may reflect differences in feed intake or feeding behaviors in the lame and mastitis groups, while the ‘other’ category may have included cows with acidosis. Importantly, rumen pH is likely influenced more by metabolic diseases, and therefore larger effects may be seen when metabolic diseases are observed and at greater number of instances. Temperature via the rumen bolus was only increased in the mastitis group, which agrees with previous research in which cows with mastitis exhibited increased temperature when measured via a rumen bolus (Kim et al., 2019) or rectally (Siivonen et al., 2011). Due to sensor location, bolus temperature may be more similar to core body temperature, thus potentially detecting temperature changes due to inflammation or immune system activation undetectable by ear tags. Ear temperature was lower in the lame and ‘other’ groups compared to healthy. This could in part be due to changes in blood flow related to diversion of blood to the animal’s core and therefore a decrease in temperature in the extremities. Importantly, rumination time was reduced in all non-healthy categories, with the lowest rumination occurring for the ‘other’ category. This may reflect reduced feed intake when animals are undergoing health disorders. It is important to recognize that the sample sizes for the rumen bolus and ear tag 1 data were limited and results for these should be given some caution, as associations may differ in other populations and breeds of dairy cattle.
4.2. Health disorders were associated with reduced feed intake
Significant reductions in feed intake were observed for all health disorders after adjusting for CG, DIM, and parity. The decrease in DMI for health disorders ranged from 0.78 (lame) to 1.98 (other) kg DMI/day compared to the healthy group (Figure 1). The average length of health disorders observed in this study were 13 days for lameness, 14 days for mastitis, and 16 days for other disorders. Considering the length of the health disorder is important when assessing the magnitude of impact the disorders have on DMI, as the estimated effects on DMI are an average over the period of the disorder. Given the estimated effects and average health disorder durations, resulting reductions in feed intake would be 10.14 kg, 20.72 kg, and 31.68 kg of DMI per bout of lameness, mastitis, and other illnesses, respectively. These results are supported by findings in research across species, with reductions in feed intake occurring during periods of immune activation and inflammation (Horst et al., 2021). Moreover, Bareille et al. (2003) and Sepúlveda-Varas et al. (2016) found a similar reduction in DMI when cows had mastitis. González et al. (2008) reported a slightly higher decrease in intake when cows suffered from locomotion disorders; however, such cases included more disorders (e.g., abnormal gate due to spinal abscess) than observed in the current study. Importantly, previous studies have had fewer observations of health disorders in comparison to the current study.
Due to the significant effect of parity in this model (i.e., model [3]; result not shown), we fit the same model with an additional variable for the interaction between parity and health. Results from this model (model [3] + parity×health) indicate that regardless of the health disorder, first lactation cows decrease just over 1 kg DMI/d when sick, with much more drastic impacts on feed intake in third and later lactations (Figure 3). Third and greater lactation cows decreased in DMI between 0.99 (lame) and 4.09 (other) kg/d. Due to the relationship between feed intake and milk production, it is expected that the 3+ lactation cows that have large decreases in feed intake would subsequently have large decreases in milk production, with a potential to impact their production efficiency depending on the severity of milk production loss. As older cows are thought to have different energy demands than younger cows which are still growing, these findings corroborate the differences between first and third lactation cows observed in a previous study (Hurley et al., 2018).
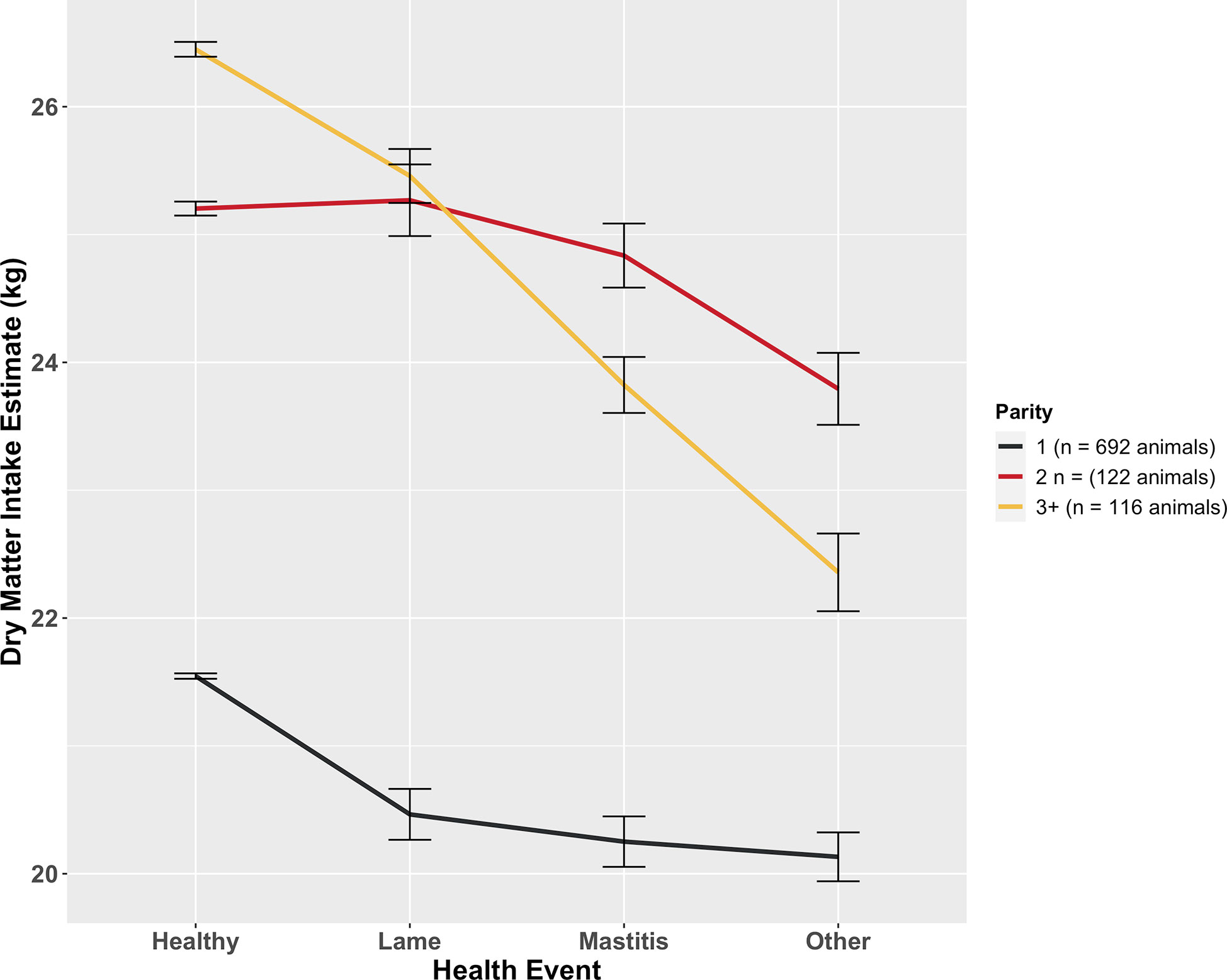
Figure 3 Impact of parity on dry matter intake (DMI) under different health disorders. Least squares means estimates of DMI for the interaction of health disorders and parity. The number of observations for each health category for parities 1, 2, and 3+ (P1, P2, P3+) are as follows: Healthy: 33,761 P1, 5,165 P2, 4,100 P3+; Lame: 289 P1, 146 P2, 262 P3+; Mastitis: 295 P1, 182 P2, 240 P3+; Other: 314 P1, 145 P2, 124 P3+. The other category included disorders with minimal observations, including respiratory illness, injury, and multiple concurrent health disorders.
Sensor and health associations with feed intake could be applied in precision feeding or genetics applications to help adjust for the impact of health disorders on feed intake. The reported estimates of reduced feed intake in response to health disorders provide an initial estimate of potential industry production and revenue loses that producers could recoup through the reduction of health disorders. The reported associations could aid in the development of more accurate prediction of health disorders prior to clinical signs. These adjustments to feed intake used in genetic evaluations would help remove the impact of temporary health disorders, which are not indicative of the animal’s genetic potential related to feed intake. A limitation of this study is that only mild health disorders were included. Estimates of reduced feed intake with illness should therefore be considered conservative since only mild cases of mastitis (cows not requiring treatment) are observed and cows receive rapid interventions with vet staff present nearly every day at the ISU research dairy.
4.3. The association of sensor and health variables with feed intake
The addition of health disorders to models including systematic effects (i.e., CG, parity, DIM) and energy sinks improves the model fit as measured by AIC (Figure 2). The impact of adding a variable for health disorders on DMI changes with the addition of energy sink data in the model, as it appears energy sinks may capture a portion of the variability in feed intake related to health of the animal.
It is important to consider that cows used in this study are monitored more frequently than commercial cows since the ISU Veterinary School has nearly constant staffing at the ISU dairy farm. Thus, cows with illnesses are often identified early on in the disorder. Cows that do experience more severe illness are moved to a hospital pen, where feed intake is not collected; thus, we tend not to capture feed intake data on cows with more severe cases of health disorders. Therefore, it is likely the effect of health events on feed intake captured at the ISU dairy are conservative. It is also important to keep in mind that the DMI estimates provided are an average across the window of time classified as a health disorder. Therefore, more severe decreases in DMI may be seen in the days closest to and during clinical diagnosis.
4.3.1. Sensor measures capture extra variation in feed intake beyond health effects
Results from the current study indicate that data recorded by sensors account for some variation in feed intake beyond that related to energy sinks and health of the animal based on the improvement in model fit and significant associations of sensor measures with feed intake after adjustment for energy sink variables and health disorders. Nearly all sensor measures were significantly associated (P< 0.05) with DMI in model [5] (i.e., accounting for systematic effects, energy sinks, and health), with the exception of CSTEMP and SCRACT as a daily average or total. Importantly, SCRACT was only significantly associated with DMI when SCRRUM was also included in the model, as observed in previous studies not evaluating the influence of animal health on feed intake (Siberski-Cooper et al., 2022). The sensor measure with the largest estimated effect was RBTEMP (-3.47 ± 0.32 kg/°C), whereas daily total CSACT had the smallest estimated effect (1.37E-06 ± 0.0 kg/activity unit). The interpretation of these estimates on DMI would be: 1) a 3.47 decease in DMI for every one degree increase in RBTEMP, and 2) a 1.37E-06 increase in DMI for every one unit increase in CSACT. Importantly, the observed range of recorded measurements for RBTEMP was very narrow (-1.57 to 2.08°C) and the range for CSACT was quite large (-23185.08 to 30021.54 activity units). Therefore, despite the small size of the CSACT effect, the difference in associated DMI across cows could be substantial. A potential benefit of utilization of sensor measurements in relation to feed intake and health may be that the length of impact of diseases on feed intake, and therefore likely production, could be derived. Future research should examine how sensors could aid in determining the duration of impact on intake from health disorders, which could potentially be done utilizing sliding windows.
4.4. Potential impact of the relationship of health and intake on genetic evaluations
The impact of health disorders on feed intake could impact genetic evaluations for feed efficiency. Results from the current study indicate that even mild illnesses have significant, measurable impacts on daily feed intake, especially in third and higher parities. However, this study also indicated it is possible to model the effects of mild health disorders such that feed intake data could be included within a genetic evaluation for feed efficiency. In some cases, the use of energy sink data may account for health disorders. However, more severe health disorders may not be captured by energy sink data, and in these cases, health disorders could be modeled or animals with severe health disorders may need to be removed from genetic evaluations. Notably, we identified sizable CG effects (model [3]: 6.82 kg DMI/day differences across CG), underscoring the impact of environment, management and potentially an effect of genetic selection over time due to the range in years data were collected. This study indicated addition of sensor data as an indicator trait for DMI could provide information useful to improve genetic evaluations. This is especially true when health data were unavailable, since sensor data accounts for some of the variation due to illness, as well as variability in feed intake beyond that of health, energy sinks, and environmental effects. The source of the added variability in feed intake detected by sensors is currently unknown. Future studies will be needed to tease apart the effects on feed intake that sensor measures detect.
5. Conclusions
Health disorders impacted feed intake and the statistical relationship between sensor measures and feed intake. All health disorders were statistically associated with reduced feed intake (P< 0.0001). Eleven different sensor measurements were significantly associated with lameness, mild mastitis and other health disorders (Model [2]; P< 0.0001). Health disorders had the greatest impact in parity 3+ cows. Several sensor measures, including CSACT (daily interval average and total), RBACT (daily interval average and total), RBTEMP, RBPH, and SCRRUM (daily interval average and total) were associated with DMI, even after adjusting for all variables included in RFI and health disorders, indicating that sensors account for health effects as well as additional unknown variation in feed intake. For five sensor measurements (RBTEMP, daily average and total SCRRUM, and combined SCR daily average and total measures), sensor and health data improved model fit (based on reduced AIC) when added into models including all variables included in RFI estimation. Results from this study indicate that health disorders can be detected by sensors and that even mild health disorders impact DMI. Further, these results indicate that health disorders may impact the relationship between sensor data and DMI. Thus, the impact of health on DMI and sensor measures should be evaluated in downstream uses of feed intake such as genetic evaluations. The study also indicates that most mild health disorders could be easily modeled to remove their effect, though it appears mild health disorders do not impact feed intake greatly compared to other sources of variation. The interaction of health disorders and parity suggests that it may be more difficult to model the impact of health disorders in older cows (i.e., parity 3+) and may require exclusion of data used for genetic evaluations.
Data availability statement
The datasets presented in this article are not readily available because of confidentiality and data sharing agreements for this project. Requests to access the datasets should be directed to JK, jekoltes@iastate.edu.
Ethics statement
The animal study was reviewed and approved by Iowa State University Institutional Animal Care and Use Committee.
Author contributions
JK developed the study, obtained funding and oversaw all aspects of the study. CS-C collected data, conducted statistical analyses, and wrote the initial draft of the manuscript. JK wrote sections of the manuscript. LB, BG, DK, BS-M, LH, MH, and MM collected and/or managed data for the study. PG and KH provided health incident data and oversaw care of animals. All authors contributed to writing and revision of the manuscript, and read and approved the submitted version of the manuscript.
Funding
Funding was provided the Foundation for Food and Agricultural Research (FFAR) and the Council for Dairy Cattle Breeding (CDCB) under project #CA18-SS-0000000236 Improving dairy feed efficiency, sustainability and profitability by impacting farmers’ breeding and culling decisions, the United States Department of Agriculture National Institute of Food and Agriculture (USDA-NIFA) under Project #2011-68004-30340, the Iowa Agriculture and Home Economics Experiment Station, Ames, Iowa (Project No. NRSP-8), the Hatch Act and the State of Iowa. CJSC was also funded in part by a dairy graduate fellowship from Land O’ Lakes.
Conflict of interest
The authors declare that the research was conducted in the absence of any commercial or financial relationships that could be construed as a potential conflict of interest.
Publisher’s note
All claims expressed in this article are solely those of the authors and do not necessarily represent those of their affiliated organizations, or those of the publisher, the editors and the reviewers. Any product that may be evaluated in this article, or claim that may be made by its manufacturer, is not guaranteed or endorsed by the publisher.
Supplementary material
The Supplementary Material for this article can be found online at: https://www.frontiersin.org/articles/10.3389/fanim.2022.1064205/full#supplementary-material
References
Aghamohammadi M., Haine D., Kelton D. F., Barkema H. W., Hogeveen H., Keefe G. P., et al. (2018). Herd-level mastitis-associated costs on Canadian dairy farms. Front. Vet. Sci. 5. doi: 10.3389/fvets.2018.00100
Bareille N., Beaudeau S., Billion R. A., Faverdin P. (2003). Effects of health disorders on feed intake and milk production in dairy cows. Livest. Prod. Sci. 83 (1), 53–62. doi: 10.1016/S0301-6226(03)0040-X
Barker Z. E., Vázquez Diosdado J. A., Codling E. A., Bell N. J., Hodges H. R., Croft D. P., et al. (2018). Use of novel sensors combining local positioning and acceleration to measure feeding behavior differences associated with lameness in dairy cattle. J. Dairy Sci. 101 (7), 6310–6321. doi: 10.3168/jds.2016-12172
De Mol R. M., André G., Bleumer E. J. B., van der Werf J. T. N., de Haas Y., van Reenen C. G. (2013). Applicability of day-to-day variation in behavior for the automated detection of lameness in dairy cows. J. Dairy Sci. 96 (6), 3703–3712. doi: 10.3168/jds.2012-6305
Dórea J. R. R., Rosa G. J. M., Weld K. A., Armentano L. E. (2018). Mining data from milk infrared spectroscopy to improve feed intake predictions in lactating dairy cows. J. Dairy Sci. 101 (7), 5878–5889. doi: 10.3168/jds.2017-13997
Fogsgaard K. K., Bennedsgaard T. W., Herskin M. S. (2015). Behavioral changes in freestall-housed dairy cows with naturally occuring clinical mastitis. J. Dairy Sci. 98 (3), 1730–1738. doi: 10.3168/jds.2014-8347
Gaddis K. L., VanRaden P. M., Tempelman R. J., Weigel K. A., White H. M., Peñagaricano F., et al. (2021). Implementation of feed saved evaluations in the U.S. Interbull Bull. 56, 147–152.
González L. A., Tolkamp B. J., Coffey M. P., Ferret A., Kyriazakis I. (2008). Changes in feeding behavior as possible indicators for the automatic monitoring of health disorders in diary cows. J. Dairy Sci. 91 (3), 1017–1028. doi: 10.3168/jds.2007-0530
Gusterer E., Kanz P., Krieger S., Schweinzer V., Süss D., Lidauer L., et al. (2020). Sensor technology to support herd health monitoring: Using rumination duration and activity measures as unspecific variables for the early detection of dairy cows with health deviations. Theriogenology 157, 61–69. doi: 10.1016/j.theriogenology.2020.07.028
Hardie L. C., VandeHaar M. J., Tempelman R. J., Weigel K. A., Armentano L. E., Wiggans G. R., et al. (2017). The genetic and biological basis of feed efficiency in mid-lactation Holstein dairy cows. J. Dairy Sci. 100 (11), 9061–9075. doi: 10.3168/jds.2017-12604
Horst E. A., Kvidera S. K., Baumgard L. H. (2021). Invited review: The influence of immune activation on transition cow health and performance – a critical evaluation of traditional dogmas. J. Dairy Sci. 104 (8), 83808410. doi: 10.3168/jds.2021-20330
Hurley A. M., Lopez-Villalobos N., McParland S., Lewis E., Kennedy E., O’Donnovan M., et al. (2018). Characteristics of feed efficiency within and across lactation in dairy cows and the effect of genetic selection. J. Dairy Sci. 101 (2), 1267–1280. doi: 10.3168/jds.2017-12841
Kennedy B. W., van der Werf J. H., Meuwissen T. H. (1993). Genetic and statistical properties of residual feed intake. J. Anim. Sci. 71, 12, 3239–3250. doi: 10.2527/1993.71123239x
Kim H., Min Y., Choi B. (2019). Real-time monitoring for the early detection of mastitis in dairy cattle: Methods and case researches. Comput. Electron. Agric. 162, 119–125. doi: 10.1016/j.compag.2019.04.004
King M. T. M., Dancy K. M., LeBlanc S. J., Pajor E. A., DeVries T. J. (2017). Deviations in behavior and productivity data before diagnosis of health disorders in cows milked with an automated system. J. Dairy Sci. 100 (10), 83588371. doi: 10.3168/jds.2017-12723
King M. T. M., LeBlanc S. J., Pajor E. A., Wright T. C., DeVries T. J. (2018). Behavior and productivity of cows milked in automated systems before diagnosis of health disorders in early lactation. J. Dairy Sci. 101 (5), 4343–4356. doi: 10.3168/jds.2017-13686
Koch R. M., Swiger L. A., Chambers D., Gregory K. E. (1963). Efficiency of feed use in beef cattle. J. Anim. Sci. 22 (2), 486–494. doi: 10.2527/jas1963.222486x
Martin M. J., Dórea J. R. R., Borchers M. R., Wallace R. L., Bertics S. J., DeNise S. K., et al. (2021). Comparison of methods to predict feed intake and residual feed intake using behavioral and metabolite data in addition to classical performance variables. J. Dairy Sci. 104 (8), 8765–9782. doi: 10.3168/jds.2020-20051
O’Callghan K. A., Cripps P. J., Downham D. Y., Murray R. D. (2003). Subjective and objective assessment of pain ad discomfort due to lameness in dairy cattle. Anim. Welf. 12 (4), 605610.
Olijhoek D. W., Difford G. F., Lund P., Løvendahl P. (2020). Phenotypic modeling of residual feed intake using physical activity and methane production as energy sinks. J. Dairy Sci. 103 (8), 6967–6981. doi: 10.3168/jds.2019-17489
Palmer M. A., Law R., O’Connell N. E. (2012). Relationships between lameness and feeding behaviour in cubicle-housed Holstein-fresian dairy cows. Appl. Anim. Behav. Sci. 140 (3-4), 121–127. doi: 10.1016/j.applanim.2012.06.005
Schlageter-Tello A., Bokkers E. A. M., Groot Koerkamp P. W. G., Van Hertem T., Viazzi S., Romanini C. E. B., et al. (2015). Relation between observed locomotion traits and locomotion score in dairy cows. J. Dairy Sci. 98 (12), 8623–8633. doi: 10.3168/jds.2014-9059
Sepúlveda-Varas P., Proudfoot K. L., Weary D. M., von Keyserlingk M. A. G. (2016). Changes in behaviour of dairy cows with clinical mastitis. Appl. Anim. Behav. Sci. 175, 8–13. doi: 10.1016/j.applanim.2014.09.022
Siberski-Cooper C. J., Koltes J. E. (2021). Opportunities to harness high-throughput and novel sensing phenotypes to improve feed efficiency in dairy cattle. Animals 12, 1, 1–15. doi: 10.3390/ani12010015
Siberski-Cooper C. J., Mayes M. S., Healey M., Goetz B. M., Baumgard L. H., Koltes J. E. (2022). Associations of wearable sensore measures with feed intake, production traits, and environmental parameters impacting feed efficiency in dairy cattle. Front. Anim. Sci. 3. doi: 10.3389/fanim.2022.841797
Siivonen J., Taponen S., Hovinen M., Pastell M., Lensink B. J., Pyörälä S., et al. (2011). Impact of acute clinical mastitis on cow behaviour. Appl. Anim. Behav. Sci. 132 (3-4), 101–106. doi: 10.1016/j.applanim.2011.04.005
Thorup V. M., Nielsen B. L., Robert P. E., Giger-Reverdin S., Konka J., Michie C., et al. (2016). Lameness affects cow feeding but not rumination behavior as characterized from sensor data. Front. Vet. Sci. 3 (37). doi: 10.3389/fvets.2016.00037
Veissier I., Mialon M. M., Sloth K. H. (2017). Short communication: Early modification of the circadian organization of cow activity in relation to disease or estrus. J. Dairy Sci. 100 (5), 3969–3974. doi: 10.3168/jds.2016-11853
Keywords: dairy cattle, feed intake, feed efficiency, precision livestock technologies, sensor data, health
Citation: Siberski-Cooper CJ, Mayes MS, Gorden PJ, Hayman K, Hardie L, Shonka-Martin BN, Koltes DA, Healey M, Goetz BM, Baumgard LH and Koltes JE (2023) The impact of health disorders on automated sensor measures and feed intake in lactating Holstein dairy cattle. Front. Anim. Sci. 3:1064205. doi: 10.3389/fanim.2022.1064205
Received: 07 October 2022; Accepted: 22 December 2022;
Published: 19 January 2023.
Edited by:
Luiz Brito, Purdue University, United StatesReviewed by:
Meagan King, University of Manitoba, CanadaBradley Heins, University of Minnesota Twin Cities, United States
Christa Egger-Danner, ZuchtData EDV-Dienstleistungen GmbH, Austria
Copyright © 2023 Siberski-Cooper, Mayes, Gorden, Hayman, Hardie, Shonka-Martin, Koltes, Healey, Goetz, Baumgard and Koltes. This is an open-access article distributed under the terms of the Creative Commons Attribution License (CC BY). The use, distribution or reproduction in other forums is permitted, provided the original author(s) and the copyright owner(s) are credited and that the original publication in this journal is cited, in accordance with accepted academic practice. No use, distribution or reproduction is permitted which does not comply with these terms.
*Correspondence: James E. Koltes, jekoltes@iastate.edu