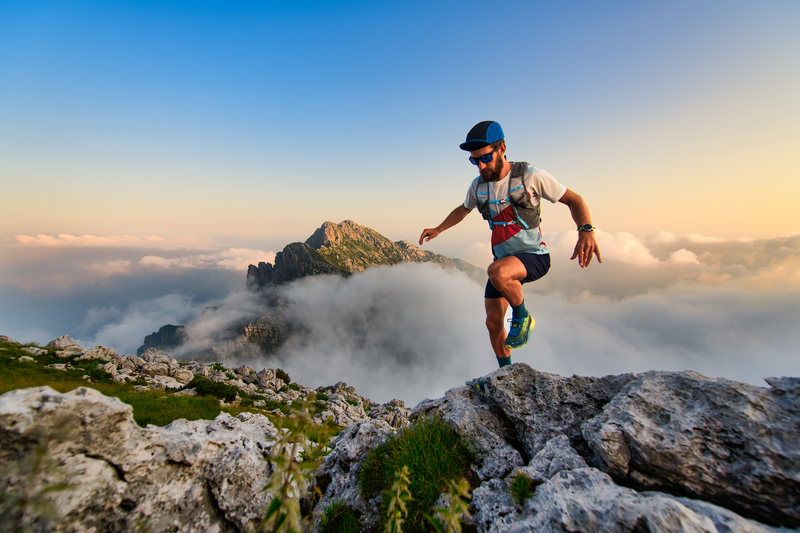
95% of researchers rate our articles as excellent or good
Learn more about the work of our research integrity team to safeguard the quality of each article we publish.
Find out more
ORIGINAL RESEARCH article
Front. Sustain. Food Syst. , 19 December 2024
Sec. Agricultural and Food Economics
Volume 8 - 2024 | https://doi.org/10.3389/fsufs.2024.1489448
Global climate change has intensified pressure on China's agricultural carbon emissions reduction. This study investigates the impact of agricultural technological progress on carbon emissions using panel data from 30 Chinese provinces (2002–2021). The research employs the DEA-Malmquist index to measure agricultural total factor productivity, the emission factor method to calculate carbon emissions, and two-tier stochastic frontier models for analysis. Results reveal that agricultural technological progress exhibits a significant double-edged effect on carbon emission intensity, with a positive effect increasing intensity by 9.01% and a negative effect reducing it by 12.18%, resulting in a net reduction of 3.17%. Different technological types show varying impacts: mechanical progress increases emission intensity, while resource-saving and biochemical technologies reduce it. Notable regional heterogeneity exists, with Southwest, South, Central, Northeast, and Northwest regions showing negative net effects, while North and East China exhibit positive effects. The findings suggest the need for differentiated regional policies and targeted agricultural emission reduction strategies to achieve China's agricultural “dual carbon” goals.
Global climate change has emerged as one of the most critical challenges facing humanity in the 21st century. According to research data from the Intergovernmental Panel on Climate Change (IPCC), global average temperatures continue to rise, extreme weather events are becoming more frequent, and ecosystems face severe threats. The reduction of greenhouse gas emissions has become an international consensus (Jha et al., 2023; Stetter and Sauer, 2022). Among various greenhouse gas emission sources, agricultural activities contribute a significant proportion to global total emissions (Zhao et al., 2018). Agricultural carbon emissions not only influence climate change progression but are also intricately linked to crucial issues such as food security and environmental protection. Agricultural technological progress serves as a key pathway for reducing agricultural carbon emissions and plays a vital role in achieving sustainable agricultural development. Recent years have witnessed significant advancement in agricultural technology as the industry faces unprecedented challenges in food security and sustainability (Smith et al., 2014). The integration of Internet of Things (IoT) and smart monitoring systems has transformed traditional farming practices into precision agriculture (Lavanya et al., 2019). Mobile technologies and smartphone applications have emerged as crucial tools for agricultural management and decision-making (Pongnumkul et al., 2015), while advanced sensing technologies and unmanned aerial vehicles (UAVs) enable precise monitoring of crop health and environmental conditions (Wortmann and Flüchter, 2015). Smart farming technologies are expected to play an increasingly vital role in achieving sustainable agriculture and food security (Gorli, 2017). However, the impact mechanism of agricultural technological progress on agricultural carbon emissions is complex, involving the interaction of multiple factors including technological innovation, economic benefits, and environmental effects. This study conducts empirical analysis to thoroughly examine the relationship between agricultural technological progress and agricultural carbon emissions. The findings hold significant theoretical value and practical implications for formulating precise emission reduction policies and promoting agricultural green transformation. Currently, most scholars believe that agricultural technological progress has had a positive effect on reducing agricultural carbon emissions (Cillis et al., 2018; Koondhar et al., 2021; Shan et al., 2022; Huang and Zhu, 2022; Liu et al., 2023; Wang et al., 2024). However, some researchers have reached opposite conclusions, arguing that agricultural technological advancements have led to increased total agricultural carbon emissions (Ismael et al., 2018; Xie et al., 2021; Anser et al., 2021; Li and Wang, 2023). These divergent views stem from the different types of agricultural technological progress examined in these studies. The classification of agricultural technological progress has evolved significantly in theoretical research. Early studies primarily adopted a binary classification approach, distinguishing between embodied and disembodied technological progress (Shih and Chang, 2009; Dosi et al., 2021). As research deepened, scholars proposed various classification frameworks based on different criteria. Some researchers categorized agricultural technology according to its application domains, such as cultivation technology, irrigation technology, and fertilization technology (Lu et al., 2022). Others classified it based on innovation characteristics, including radical innovation and incremental innovation in agricultural production (Acemoglu et al., 2022). Recent studies have increasingly focused on the environmental impacts of agricultural technological progress, leading to more sophisticated classification systems. Liu et al. (2023) analyzed agricultural technical efficiency and technical progress from the perspective of spatial spillovers and threshold effects. Wang et al. (2024) developed a classification system based on carbon emission effects, examining the impact of technical progress and fiscal support on agricultural carbon emissions. These classification approaches have provided valuable insights but often overlook the complex interactions between different types of technological progress and their comprehensive environmental impacts, as evidenced by the contrasting findings of Xie et al. (2021) and Li and Wang (2023). This study adopts a four-category classification framework (mechanical, structural, resource-saving, and biochemical technological progress) based on three theoretical considerations. First, this classification comprehensively covers the main technological pathways in modern agriculture, reflecting both traditional mechanization and emerging green technologies. Second, it allows for clear differentiation of carbon emission effects, as each category has distinct mechanisms influencing agricultural carbon emissions. Third, this classification aligns with China's agricultural development strategy and policy framework, making it particularly relevant for policy analysis and recommendations. This framework builds upon previous research while addressing the need for a more nuanced understanding of how different types of agricultural technological progress affect carbon emissions.
These different types of agricultural technological progress can be classified into two categories based on their impact mechanisms on agricultural carbon emissions: carbon-promoting and carbon-inhibiting effects. Mechanical technological progress falls under the category of carbon-promoting technologies. Agricultural mechanization effectively replaces traditional manual labor by introducing various mechanical equipment. While this substitution reduces labor dependency and costs, it also significantly increases production efficiency (Gallardo and Sauer, 2018). However, the production and use of agricultural machinery typically consume substantial energy and materials, directly leading to increased carbon emissions (Mantoam et al., 2020). Moreover, the carbon-promoting effect of agricultural mechanization on agricultural emissions is persistent over time (Guan et al., 2023). Structural technological progress refers to changes in production structures and methods through the introduction of new technologies, varieties, or management practices to improve agricultural production efficiency. This type of progress may lead to a shift toward monoculture, resulting in excessive utilization of certain soil nutrients while others accumulate excessively. The imbalanced use of soil nutrients affects soil fertility balance and microbial activity, increasing the risk of carbon emissions (Zhang, 2015). Structural technological progress can also cause land-use changes, leading to non-agricultural land use and increased carbon emissions (Fan, 2022). Therefore, structural technological progress is also considered a carbon-promoting technology.
Resource-saving technological progress refers to the use of scientific and technological means to more effectively utilize resources in agricultural production, thereby improving efficiency, reducing production costs, and minimizing negative environ-mental impacts. Precision fertilization technology accurately determines crop nutrient requirements, reducing fertilizer use and improving nutrient utilization efficiency while decreasing greenhouse gas emissions (Zhang et al., 2023; Xia et al., 2017). Yrjälä et al. (2022) explored the role of agricultural waste as a resource in the circular economy and carbon reduction, identifying biochar production through biomass pyrolysis as a highly feasible method. Additionally, research has found that the application and promotion of efficient, low-carbon irrigation technologies can significantly reduce energy consumption and carbon emissions (Qin et al., 2024). Biochemical technological progress focuses on utilizing biological and chemical knowledge and techniques to play a crucial role in reducing carbon emissions. Bachleitner et al. (2023) found that converting carbon dioxide and its derived carbon substrates into valuable compounds through microbial processes can contribute to carbon neutrality and sustainable development. Innovation and improvement in chemical fertilizers have great potential for reducing greenhouse gases. Gao and Cabrera Serrenho (2023) predicted that by improving fertilizer production technologies and optimizing application methods, fertilizer-related carbon emissions could be reduced by approximately 80% by 2050.
To explore the relationship between agricultural technological progress and carbon emissions, existing literature has employed a variety of econometric methods and models. Some scholars have used double fixed-effects models, endogenous technology models, autoregressive distributed lag models, and granger causality test to investigate the directional impact of agricultural technological progress on carbon emissions (Liu et al., 2023; Gerlagh, 2007; Koondhar et al., 2021; Ismael et al., 2018). Others have examined the non-linear relationship between the two using threshold effect models (Liu et al., 2023). Additionally, some researchers have explored spatial effects through spatial Durbin models (Wang et al., 2024). However, these methods fall short in simultaneously measuring the positive and negative effects of agricultural technological progress on carbon emissions, i.e., the bidirectional impact. To address this gap, the two-tier stochastic frontier (2TSF) model emerges as a promising tool. This model was proposed by Kumbhakar and Parmeter (2009), which allows for the estimation of two interdependent stochastic frontier functions simultaneously. In recent years, it has been applied to various economic issues, including auctions (Verteramo Chiu et al., 2022), bargaining processes (Xu et al., 2021), and investment efficiency analyses (Xie and Li, 2018). Notably, it has been used to examine the bidirectional impact of China's outward direct investment on carbon emissions (Wang et al., 2023). The 2TSF model is particularly well-suited for analyzing the bidirectional effects of agricultural technological progress on carbon emissions. Therefore, it can simultaneously estimate how technological advancements influence emission levels and how emission constraints affect technological adoption and development in agriculture.
In summary, scholars have investigated the impact of agricultural production activities on carbon emissions from multiple perspectives, establishing a solid foundation for understanding the mechanisms and characteristics of agricultural carbon emissions. However, as research has deepened, some limitations in the existing literature have become apparent. Firstly, there is no consensus on the impact of agricultural technological progress on carbon emissions. Some studies suggest that technological advancements can reduce emissions, while others reach opposite conclusions. This discrepancy reflects the potentially complex relationship between agricultural technological progress and carbon emissions. Secondly, existing research often focuses on single technologies or specific aspects, lacking a systematic analysis of the relationship between agricultural technological progress and carbon emissions. Agricultural production involves multiple stages, and different types of technological advancements may have varied or even opposing effects on carbon emissions. Thirdly, few studies have quantitatively measured the bidirectional impact of agricultural technological progress on carbon emissions. Most research focuses on overall effects, neglecting the potential dual role of technological progress in both promoting and inhibiting carbon emissions. Lastly, there is insufficient research on the regional heterogeneity of the impact of agricultural technological progress on carbon emissions. Given China's vast territory and significant regional differences in natural conditions and economic development levels, the carbon emission effects of technological progress may vary considerably across regions.
Based on these research gaps, this study makes several important contributions to the existing literature. First, this study establishes a comprehensive theoretical framework by categorizing agricultural technological progress into four types: mechanical, structural, resource-saving, and biochemical, providing a systematic foundation for understanding its diverse impacts on agricultural carbon emissions. Second, this study innovatively investigates and quantifies the impact of agricultural technological progress on agricultural carbon emissions from both carbon-promoting and carbon-suppressing perspectives. Third, the empirical analysis reveals significant regional heterogeneity in the impact of agricultural technological progress on agricultural carbon emissions. Overall, China shows a “strong South, weak North” pattern, with the Southwest region displaying unique “dual optimal” characteristics. Finally, based on the multidimensional impacts of technological progress and regional heterogeneity characteristics, this study proposes a differentiated policy recommendation framework, providing theoretical basis and practical guidance for formulating precise regional agricultural emission reduction policies.
The remainder of this paper is structured as follows: Section 2 introduces the data sources and variable composition; Section 3 presents the research methodology, constructing the 2TSF model; Section 4 presents the empirical results and analysis, including the overall effect of agricultural technological progress on carbon emissions, differentiated impacts of various types of technological progress, regional heterogeneity analysis, and discussion of influencing factors; Section 5 concludes the paper with main research findings, policy recommendations, limitations, and future research directions.
This study utilizes panel data from 30 provincial-level regions in China spanning from 2002 to 2021. The primary data sources include the China Statistical Yearbook (2002–2021), China Rural Statistical Yearbook (2002–2021), China Population and Employment Statistical Yearbook (2002–2021), National Bureau of Statistics of China (2002–2021), and the Wind database. Missing data were supplemented using provincial statistical yearbooks.
The calculation of agricultural carbon emissions (C) primarily employs three methods: the emission factor method, model simulation method, and field measurement method. Each approach has its own scope of application, advantages, and disadvantages (Hu et al., 2023). The model simulation method requires extensive parameter inputs and is primarily utilized for simulating carbon emissions within ecological systems. While the field measurement method yields precise results, its application is confined to microscopic emission sources due to cost constraints. In comparison, the emission factor method offers greater data accessibility and proves more suitable for the macro-level emission sources selected in this study (Chen et al., 2024; Lauk et al., 2024). This method multiplies the activity level data of agricultural carbon emission sources by their respective emission coefficients to obtain the emissions of various greenhouse gases in the agricultural sector. These are then summed to derive the total carbon emissions. The specific calculation formula is as follows:
where Cit represents the total agricultural carbon emissions of province i in year t; Tnit denotes the activity level of the nth carbon emission source in province i in year t; and σn is the carbon emission coefficient for the nth carbon emission source. Based on existing research, the carbon emission coefficients for various input factors are shown in Table 1. It is important to note that different greenhouse gases have varying global warming potentials. To ensure consistency in results, this study converts all greenhouse gases to standard carbon dioxide equivalents. According to the IPCC Fourth Assessment Report, the conversion factors for carbon, methane, and nitrous oxide are 44/12, 25, and 298, respectively.
To mitigate the impact of agricultural economic growth on carbon emission data, it is necessary to further measure the carbon emission intensity per unit of agricultural gross domestic product. This study employs the Agricultural Carbon Emission Intensity (CI) index, which represents the carbon emissions produced per 10,000 yuan of agricultural GDP (tons/10,000 yuan). A higher index value indicates greater carbon emission intensity in agricultural production, and vice versa. The calculation formula is as follows:
where CIit is the agricultural carbon emission intensity of province i in year t, and GDPit is the agricultural gross domestic product of province i in year t.
This study employs a broad definition of agricultural technological progress, following Zhang et al. (2023), using Agricultural Total Factor Productivity (ATFP) as a proxy indicator. ATFP is measured and decomposed using Data Envelopment Analysis (DEA) and the Malmquist index method. DEA, first proposed by Charnes et al. (1978), is a non-parametric method that uses linear programming to evaluate the efficiency of decision-making units of the same type. To analyze inter-temporal consumption changes, Malmquist (1953) constructed a distance function and introduced the Malmquist index concept. Fare et al. (1994) further developed the Malmquist index and effectively combined it with DEA theory. Subsequently, the DEA-Malmquist index has become an important tool for measuring production efficiency. The Malmquist index can be decomposed into two components: effch and tech. Effch represents efficiency change, reflecting the distance between decision-making units and the current production frontier. It is closely related to efficient resource utilization and optimal resource allocation. Tech represents technological change, capturing the movement of the production frontier from period t to t+1. It primarily stems from technological advancements brought about by new knowledge, skills, organizational structures, and innovations. Furthermore, when considering variable returns to scale, efficiency change (effch) can be further decomposed into scale efficiency change (sech) and pure efficiency change (pech).
For the input-output indicators, this study employs agricultural gross output value (100 million yuan) as the output measure, deflated to 2002 prices to eliminate the impact of price factors. Input indicators are selected from four aspects: agricultural labor input, agricultural machinery input, fertilizer input, and land input. These are represented by the number of employees in the primary industry (10,000 people), total power of agricultural machinery (10,000 kilowatts), pure amount of fertilizer (10,000 tons), and total sown area of crops (10,000 hectares), respectively. Finally, the ATFP panel data for 30 Chinese provinces from 2002 to 2021 is calculated and decomposed using DEAP2.1 software.
This study employs the two-tier stochastic frontier models to disentangle the positive and negative effects of agricultural technological progress on carbon emissions. Four types of technological progress are introduced as covariates: resource-saving technological progress (RSTP), biochemical technological progress (BTP), mechanical technological progress (MTP), and structural technological progress (STP).
Resource-saving technological progress (RSTP) is represented by water-saving irrigation technology, a typical example of resource-efficient techniques. The adoption rate of water-saving irrigation technology (ratio of water-saving irrigation area to total crop sowing area) serves as a proxy for RSTP.
Biochemical technological progress (BTP) primarily involves germplasm innovation and the use of new fertilizers and pesticides. This study uses the application rate of compound fertilizers (proportion of compound fertilizer application to total fertilizer application) as a proxy for BTP.
Mechanical technological progress (MTP) is influenced by both per-machine carbon emissions and the number of agricultural machines. Considering the ongoing increase in both the variety and total number of agricultural machinery, this study uses the total power of agricultural machinery per unit area of cultivated land as a measurement indicator for MTP.
Structural technological progress (STP) is represented by the proportion of agriculture within the primary industry, calculated as the ratio of agricultural output value to the total output value of agriculture, forestry, animal husbandry, and fishery.
To systematically analyze the dual effects of agricultural technological progress on carbon emissions, it is necessary to control for other influencing factors. Based on existing research, this study selects control variables from three aspects: agricultural production conditions, government support, and rural human capital.
Agricultural Production Conditions: (1) Agricultural Economic Development Level (ey): Measured by per capita agricultural GDP. Improved agricultural economic development typically leads to enhanced production efficiency, potentially affecting carbon emissions through optimized resource allocation and increased crop yields (Zang et al., 2022). (2) Agricultural Land Management Scale (sl): Calculated as the ratio of crop sowing area to the number of primary industry employees. The development of land transfer markets has enabled large-scale farming, contributing to reduced production costs and green agricultural development (Ma et al., 2023). (3) Total agricultural machinery power (mp): Represented by the total power of agricultural machinery (10,000 kilowatts) in each province. This factor is both an indicator of technological progress and directly related to carbon emissions (Guan et al., 2023). To mitigate heteroscedasticity, a logarithmic form (lnmp) is used. (4) Agricultural Disaster Impact (ad): Measured by the ratio of disaster-affected agricultural area to total crop sowing area. Kirat et al. (2024) found that climate-induced natural disasters significantly impact energy consumption and carbon emissions. (5) Agricultural External Dependence (ed): Represented by the ratio of total agricultural product import and export value to primary industry added value. Gao et al. (2021) noted that foreign trade significantly promotes carbon emissions, with effects varying across regions and time periods. Import and export data are sourced from the Ministry of Commerce's monthly statistical reports, converted from USD to CNY using year-end exchange rates from the Wind database.
Government Support: (6) Agricultural Fiscal Support (fs): Measured by the ratio of agricultural expenditure to total government fiscal expenditure. Guo et al. (2022) found that fiscal support significantly reduces agricultural carbon emissions. For data after 2006, expenditure on agriculture, forestry, and water affairs under public finance is used as a proxy.
Rural Human Capital Conditions: (7) Human Capital Level (hc): The improvement of human capital, such as the educational level of agricultural producers, enables farmers to make better choices in agricultural production tools and methods, serving as an important pathway to promote green agricultural production and reduce agricultural carbon emissions (Shabani, 2024). This study uses the average education level in each province to measure this indicator. The population aged 6 and above is categorized by education level into primary school, junior high school, high school and vocational school, and college and above. The average years of education for each province are calculated by multiplying the proportion of each category by its corresponding years of education (assigned values of 6, 9, 12, and 15 years, respectively), summing these products, and then dividing by the total population aged 6 and above. (8) Urbanization Level (ur): Measured by the ratio of urban population to total population. Higher urbanization levels typically indicate a more modernized economic structure, potentially facilitating the development of green ecological agriculture and reducing carbon emissions (Prastiyo et al., 2020). (9) Rural Per Capita Income Level (lnpi): Represented by the logarithm of annual per capita net income of rural residents. Increased income may have dual effects on agricultural carbon emissions. It could lead to higher use of agricultural inputs, increasing emissions, or enable the adoption of more advanced, efficient agricultural practices, potentially reducing emissions (Chang et al., 2022).
The descriptive statistics for all variables used in this study are presented in Table 2.
Following Kumbhakar and Parmeter (2009) and Papadopoulos (2021), a framework of the 2TSF model is constructed to analyze the impact of agricultural technological progress on agricultural carbon emission intensity. The agricultural carbon emission intensity can be expressed as:
where CI represents the actual level of agricultural carbon emission intensity. Influenced by agricultural technological progress, the value of CI may range from a lower limit CI to an upper limit . Agricultural technological progress can affect carbon emission intensity in two opposing directions: a positive effect and a negative effect. The parameter η (0 ≤ η ≤ 1) is an unobservable coefficient that measures the relative impact of these two effects of agricultural technological progress on carbon emission intensity. When η =1, , indicating that carbon emission intensity reaches its maximum value, suggesting that agricultural technological progress only has positive effect on agricultural carbon emission intensity. Conversely, when η = 0, CI = CI, signifying that carbon emission intensity reaches its minimum value, implying that agricultural technological progress only has negative effect on agricultural carbon emission intensity.
To better identify the positive and negative effects of agricultural technological progress on carbon emission intensity, the Equation 3 can be rewritten as:
where μ(x) represents the baseline level of agricultural carbon emission intensity at a specific economic development level, without considering agricultural technological progress. The positive effect (PE) and negative effect (RE) of agricultural technological progress on carbon emission intensity can be expressed as:
Consequently, the net effect (NE) of agricultural technological progress on carbon emission intensity can be represented as:
Under this framework of carbon emission, the 2TSF model is used to estimate the double-edged sword effect of agricultural technological progress on carbon emission intensity, and can be expressed as:
where CIit represents the actual agricultural carbon emission intensity for province i in year t; include ATP, ey, sl, lnmp, ad, ed, fs, hc, ur, lnpi; β is the parameter to be estimated. represents the positive effect of agricultural technological progress on carbon emission intensity. uit = (1 − η)(μ(x) − CI) ≥ 0 represents the negative effect of agricultural technological progress on carbon emission intensity. wit and uit are independently distributed, following exponential distributions: wit ~ i.i.d.Exp(σw) and uit ~ i.i.d.Exp(σu). vit is a random disturbance term following a standard normal distribution: .
Based on the above settings, the conditional expectation of wit and uit can be derived as respectively:
In this model: , , , , and ∅(·) denote the cumulative distribution function and probability density function of the standard normal distribution, respectively.
Given that the dependent variable is in logarithmic form, the following equations are used to obtain accurate percentage indicators of agricultural carbon intensity increase or decrease due to the positive or negative effects of agricultural technological progress:
The net effect (NE) of agricultural technological progress can then be expressed as:
A positive net effect indicates that agricultural technological progress increases carbon emission intensity, while a negative net effect suggests a decrease.
Based on the research of Parmeter (2018) and Liu et al. (2019), this study incorporates covariates to explain the one-sided error terms wit and uit. As wit and uit follow exponential distributions, we can get the following equations:
where zw and zu are covariates affecting wit and uit, and δw and σu are their respective estimated parameters. zw includes the covariates TGTP and SGTP, zu includes the covariates AEGTP and BGTP. The parameters δw and σu set the scale range for each individual mean, independent of zw and zu.
To ensure the robustness of our empirical results, we employed three econometric models: mixed effect model, two-way fixed effects model, and two-way random effects model. The estimation results are presented in Table 3. Across all three models, agricultural technological progress (ATFP) demonstrates a significant negative correlation with agricultural carbon emission intensity at the 1% significance level. Specifically, the coefficients of ATFP are −0.4171, −0.2020, and −0.2508 respectively, indicating that technological advancement in agriculture consistently contributes to reducing carbon emission intensity. Among the control variables, several factors show significant effects across all models. The agricultural land management scale (sl) exhibits positive coefficients, suggesting that larger-scale agricultural operations tend to increase carbon emission intensity. Agricultural disaster impact (ad) and external dependence (ed) both show positive and significant effects, implying that natural disasters and international trade integration may increase carbon emissions. Conversely, fiscal support for agriculture (fs) and rural residents' income (lnpi) demonstrate significant negative effects, indicating that both government support and increased rural income contribute to reducing agricultural carbon emissions. Moreover, the consistency of coefficient signs and significance levels across all three models supports the robustness of our findings.
To further validate the reliability of the empirical results, this study employed two distinct robustness tests. The first test involved removing provinces with relatively low carbon emissions from the sample to examine whether the findings were potentially influenced by regional heterogeneity. This approach helps ensure that the results are not driven by outliers or specific regional characteristics. The second test divided the study period into two sub-periods: 2002–2011 and 2012–2021. This temporal division allows investigation of whether the relationship between agricultural technological progress and carbon emissions remains stable across different time periods, particularly considering China's significant policy changes and economic development during these two decades. According to Table 4, the results from both robustness tests strongly support the main findings. When excluding provinces with low carbon emissions, the regression results maintained their statistical significance and directional consistency with the baseline model. The coefficients of key variables, such as agricultural fiscal support (fs), urbanization level (ur), and rural per capita income (lnpi), retained their signs and significance levels, with only minor variations in magnitude. This consistency suggests that the findings are not driven by specific regional characteristics or outliers in the data. The temporal analysis revealed interesting patterns while confirming the overall stability of the results. During both sub-periods (2002–2011 and 2012–2021), the fundamental relationship between agricultural technological progress and carbon emissions remained consistent with the main findings. However, a slightly stronger effect was observed in the 2012–2021 period, possibly reflecting the enhanced effectiveness of China's environmental policies and technological advancement in recent years. This temporal stability provides additional confidence in the robustness of the conclusions and suggests that the findings are not sensitive to specific time period selections. These comprehensive robustness checks demonstrate that the empirical results are stable across different sample specifications and time periods, thereby strengthening the reliability and generalizability of the findings. The consistency of results across these alternative specifications provides strong evidence that the conclusions about the relationship between agricultural technological progress and carbon emissions are robust and reliable.
To verify the robustness of the research findings, this study conducted rigorous tests for potential endogeneity issues in the model. The relationship between agricultural technological progress and carbon emissions may exhibit bidirectional causality. Agricultural technology progress affects carbon emission intensity, while carbon emission constraints may conversely drive agricultural technology progress. Additionally, omitted variables and measurement errors could also lead to endogeneity problems.
This study employed three methods for robustness testing: one-period lag substitution, two-stage least squares (2SLS) with instrumental variables, and dynamic panel GMM estimation. In the one-period lag substitution model, the coefficient of agricultural technological progress was −0.0960, significant at the 10% level, indicating a significant negative impact on carbon emission intensity. For the 2SLS estimation, the lagged terms of agricultural technological progress were selected as instrumental variables. The results showed that the impact coefficient of agricultural technological progress on carbon emission intensity was −0.1784, significant at the 1% level. This aligned with the direction of the results in Table 5, with a stronger magnitude of influence. In the dynamic GMM estimation, the lagged dependent variable served as an instrumental variable, controlling for two-way fixed effects. The Sargan test p-value of 1 indicated appropriate instrumental variable selection. The p-values for first-order and second-order autocorrelation tests were 0.1987 and 0.6173, respectively, suggesting no autocorrelation issues. The estimation revealed that agricultural technological progress had a coefficient of −0.4406 on carbon emission intensity, significant at the 1% level. The lagged dependent variable coefficient of 0.6269 (significant at 1%) demonstrated significant dynamic persistence in carbon emission intensity. Regarding control variables, the agricultural economic development level (ey) showed significant negative coefficients across all three models: −0.1396 (5%), −0.1385 (1%), and −0.0434 (1%). Agricultural land operation scale (sl) was insignificant in the lag substitution model but significantly positive in both 2SLS (1%) and GMM (10%) models. Agricultural external dependence (ed) showed significant positive effects in the lag substitution and 2SLS models but was insignificant in the GMM model. The findings from these three methods consistently demonstrate that the negative impact of agricultural technological progress on carbon emission intensity remains significant after controlling for endogeneity, further confirming the reliability of this study's conclusions.
Table 6 presents the estimation results of the two-tier stochastic frontier model. Models (1)-(4) are four different 2TSF models that are used to examine the double-edged effect of agricultural technological progress on carbon emissions. Model (1) is a traditional 2TSF model without covariates, including only conventional agricultural input-output data. Model (2) introduces resource-saving (RSTP) and biochemical (BTP) technological progress as covariates to explain uit, examining their negative effects on agricultural carbon emission intensity. Model (3) incorporates mechanical (MTP) and structural (STP) technological progress as covariates to explain wit, investigating their positive effects on carbon emission intensity. Model (4) includes all types of agricultural technological progress to assess their double-edged effects on carbon emission intensity.
The maximum likelihood function values and AIC results for Models (1)–(4) indicate that Model (4) has the highest likelihood value and lowest AIC. Based on model selection criteria, Model (4) is optimal. Model (4) in Table 6 reveals that the impact of China's agricultural technological progress on agricultural carbon emission intensity is statistically significant at the 1% level, with a negative regression coefficient. This indicates that agricultural technological progress has a negative effect on agricultural carbon emission intensity.
Further analysis of different types of technological progress shows that mechanical technological progress (MTP) has a positive coefficient, significant at the 1% level. This suggests that increased mechanization has led to higher carbon emission intensity. Conversely, resource-saving (RSTP) and biochemical (BTP) technological progress have negative coefficients, both significant at the 1% level, indicating their effectiveness in reducing carbon emission intensity. These findings demonstrate that agricultural technological progress exhibits a “double-edged sword” effect on carbon emission intensity, with both positive and negative impacts. It is noteworthy that the coefficient for structural technological progress (STP) is not statistically significant. This may be due to the complex nature of China's agricultural structural changes, which do not follow a simple linear development path, making its impact on agricultural carbon emission intensity difficult to estimate.
Analysis of control variables provides further insights into other factors affecting agricultural carbon emissions. All control variables, except for agricultural economic development level (ey), are significant at various levels. Agricultural land management scale (sl), total agricultural machinery power (lnmp), agricultural disaster rate (ad), agricultural external dependence (ed), and urbanization level (ur) have significantly positive coefficients, indicating positive correlations with agricultural carbon emission intensity. In contrast, fiscal support for agriculture (fs), rural human capital level (hc), and rural per capita net income (lnpi) have significantly negative coefficients, suggesting negative correlations with agricultural carbon emission intensity.
Based on the estimation results of model (6) and in conjunction with Equations 12–14, we calculated the positive, negative, and net effects of agricultural technological progress on carbon emission intensity. The results are presented in Table 7. On average, the positive effect of agricultural technological progress increases agricultural carbon emission intensity by 9.01%, while the negative effect reduces it by 12.18%. Consequently, the net effect of agricultural technological progress leads to an average decrease of 3.17% in agricultural carbon emission intensity. At different quantile levels, the net effect of agricultural technological progress varies. At Q1 and Q2 quantiles, the net effects are negative, at −8.25% and −1.83% respectively. However, at the Q3 quantile, the positive effect exceeds the negative effect, resulting in a positive net effect.
Table 7. Double-edged sword effect and net effect of agricultural technological progress on agricultural carbon emission intensity in Chinese provinces.
Figure 1 illustrates the temporal changes in the negative, positive, and net effects of China's agricultural technological progress on agricultural carbon emission intensity from 2002 to 2021. Throughout this period, the net effect remained negative, indicating that the negative effect consistently outweighed the positive effect, thereby reducing carbon emission intensity. The absolute value of the net effect showed a general decreasing trend from 2003 to 2012, followed by an increasing trend from 2012 to 2021. This suggests that the carbon reduction effect of agricultural technological progress diminished from 2003 to 2012 but strengthened overall from 2012 to 2021. In 2021, there was a sharp increase in the carbon emission intensity reduction effect of agricultural technological progress. This may be attributed to the enhanced innovation and promotion of low-carbon agricultural technologies following the introduction of the “dual carbon strategy” in 2020, leading to the application of more environmentally friendly technologies in agricultural production.
Figure 1. Positive, negative and net effects of agricultural technology progress on agricultural carbon emission intensity in China, 2002–2021.
Considering the diverse natural conditions and resource endowments across China, there are significant differences in agricultural production methods and planting structures among regions. Based on geographical location, topography, climate characteristics, and economic development levels, this study divides China into seven geographical regions: Northeast, East China, North China, Central China, South China, Southwest, and Northwest.
As shown in Table 8, several significant patterns emerge in the regional distribution of agricultural technological progress's impact on carbon emissions. The net effects analysis reveals that the Southwest, South China, Central China, Northeast, and Northwest regions demonstrate negative effects, indicating that agricultural technological advancement in these regions generally contributes to carbon reduction. Conversely, North China and East China regions exhibit positive effects, suggesting that technological progress has intensified carbon emissions. These regional disparities may be attributed to variations in agricultural development stages and technological application pathways across regions. The analysis of effect intensity distribution demonstrates that coastal regions predominantly show positive effects, while inland areas exhibit more significant negative effects. A distinct “South-strong, North-weak” pattern is observed, with southern regions displaying stronger negative emission reduction effects compared to northern regions. This phenomenon may be associated with the earlier implementation of agricultural green transformation and ecological agriculture demonstration zones in southern regions. The Southwest region exhibits unique “dual-optimal” characteristics. Its positive effect is merely 6.00%, the lowest among the seven regions, while its negative effect reaches 21.14%, the highest among all regions. This effect combination results in a net effect of −15.14%, making the Southwest region the most significant area where agricultural technological progress effectively inhibits carbon emissions. The mountainous terrain in the Southwest region objectively limits the application of mechanization-oriented technological progress due to relatively low agricultural mechanization levels, thereby suppressing positive effects. Simultaneously, due to the region's environmental sensitivity, both government authorities and farmers demonstrate stronger environmental awareness, favoring resource-conserving and biochemical technologies, which promotes negative effects.
Table 8. Effects of agricultural technology progress on agricultural carbon emission intensity in seven geographic regions of China.
The scale of agricultural land management significantly influences land utilization methods and agricultural production efficiency, thereby affecting the impact of agricultural technological progress on carbon emission intensity in agriculture. This study categorizes 30 Chinese provinces into three equal groups based on their scale of agricultural land management, from small to large, with each group comprising 10 provinces.
According to Table 9, in provinces with small and medium scales of agricultural land management, the net effect of agricultural technological progress on carbon emission intensity is negative. Agricultural technological advancements have reduced carbon emission intensity by an average of 7.56% and 2.69% respectively in these regions. However, in provinces with large-scale agricultural land management, the net effect is positive. Overall, as the scale of agricultural land management increases, the positive effect of technological progress on agricultural carbon emissions intensifies, while the negative effect diminishes.
Table 9. The effect of agricultural technological progress on the intensity of agricultural carbon emissions (grouped by the scale of agricultural land management).
Figure 2 illustrates the dynamic evolution of agricultural technological progress's net effect on carbon emissions from the perspective of farmland operation scale. The three scale groups demonstrate distinct differentiation characteristics, which can be analyzed from several aspects. From the overall trend perspective, large-scale and medium-scale groups exhibit relatively stable patterns with minimal fluctuations and weak carbon reduction effects. These larger-scale operators tend to adopt highly mechanized production methods, resulting in substantial positive effects. Due to their scale and significant initial investments, these operators often experience path dependency, making it more challenging to implement technological transitions and improvements in production methods. The small-scale group demonstrates notably larger fluctuations in net effects compared to the other two groups, reflecting higher sensitivity to external environmental changes. This phenomenon can be attributed to two primary factors. First, recent agricultural policies have provided targeted support to small-scale farmers, with government fiscal support reducing the cost threshold for adopting green technologies. Second, enhanced environmental awareness and the widespread promotion of green technologies have made low-carbon agricultural technologies more accessible and applicable for small-scale operators. Turning point analysis identifies 2011 as a crucial temporal node. Prior to 2011, the net effects among the three scale groups showed relatively minor differences. However, significant divergence emerged after 2011. This differentiation trend closely aligns with China's agricultural policy transformation period, reflecting the differential impact of policy regulations on operators of varying scales.
Analysis of data from Table 10 reveals a significant gradient pattern: the impact of agricultural technological advancement on carbon emissions varies notably with the intensity of fiscal support for agriculture. Specifically, in regions with high fiscal agricultural support, technological progress generates a 7.66% positive emission effect alongside a 15.64% negative reduction effect, resulting in a net emission reduction of 7.98%. In contrast, regions with moderate fiscal support achieve only a 1.97% net reduction effect, while areas with low fiscal support exhibit negative net emission reduction effects. This gradient distribution indicates a significant positive correlation between fiscal agricultural support intensity and the emission reduction effectiveness of technological advancement. The underlying mechanisms may be attributed to three factors: First, higher fiscal support reduces barriers for farmers to adopt green, low-carbon technologies, enhancing their motivation for technological updates. Second, fiscal support typically integrates with complementary technical extension services, facilitating effective technology implementation. Third, adequate fiscal funding enables local governments to provide more comprehensive infrastructure and technical service systems for agricultural green transformation.
Table 10. The effect of agricultural technological progress on the intensity of agricultural carbon emissions (grouped by the fiscal support for agriculture).
Temporal analysis of the evolutionary trends in Figure 3 identifies distinctly different development trajectories among the three groups during 2002–2021. Regions with high fiscal agricultural support demonstrate the most pronounced dynamic characteristics, with their net effect curve showing substantial fluctuations. A notable inflection point emerged after 2011, marking a significant enhancement in emission reduction effects. This transition likely correlates with China's agricultural policy transformation around 2011, coinciding with the initiation of the 12th Five-Year Plan, which strengthened policy support for agricultural green development. In comparison, regions with moderate and low fiscal support exhibit relatively stable net effect curves with minimal fluctuations, indicating more consistent impacts of agricultural technological progress on carbon emissions. Notably, the disparity between regions with different fiscal support levels has progressively widened over time. This “Matthew Effect” suggests the cumulative impact of fiscal support in agricultural green transformation and emphasizes the necessity of increasing fiscal support for less developed regions.
Similar to the previous analysis, this section categorizes 30 Chinese provinces into three groups based on rural human capital levels, ranging from low to high. Table 11 illustrates the impact of agricultural technological progress on agricultural carbon emission intensity for each group. Overall, agricultural technological progress exhibits a negative effect on carbon emissions across all three groups, indicating a reduction in agricultural carbon emission intensity. Regions with low human capital levels demonstrate the most significant carbon reduction effect, with an average decrease of 4.84% in agricultural carbon emission intensity. Conversely, areas with high human capital levels show the least pronounced effect. This suggests that the carbon reduction efficacy of agricultural technological progress diminishes as human capital levels increase. This phenomenon may be attributed to the late-mover advantage in regions with low human capital levels, allowing for faster adoption and dissemination of advanced low-carbon technologies. In contrast, high human capital regions may have already implemented advanced low-carbon technologies, resulting in weaker emission reduction effects from further technological progress.
Table 11. The effect of agricultural technological progress on the intensity of agricultural carbon emissions (grouped by human capital levels).
Figure 4 illustrates the temporal evolution of the net effect of agricultural technological progress on carbon emission intensity across different groups. Prior to 2011, the net effects were relatively similar across all three groups, generally reducing agricultural carbon emission intensity, except for an increase in low human capital regions in 2002. From 2012 onwards, the carbon reduction effects began to diverge significantly among the groups, with low human capital regions exhibiting the most pronounced reduction in agricultural carbon emission intensity. In 2021, high human capital regions experienced a significant enhancement in the carbon reduction effect, surpassing medium human capital areas. This may be attributed to the emergence of more advanced and efficient technologies in recent years, which are more readily adopted and applied in regions with high human capital levels.
The empirical findings of this study reveal several important theoretical and practical implications that warrant further discussion. First, the double-edged sword effect of agricultural technological progress on carbon emissions demonstrates the complexity of agricultural modernization. This finding challenges the simple linear relationship assumed in previous literature (Liu et al., 2023; Gerlagh, 2007; Koondhar et al., 2021; Ismael et al., 2018) and suggests that the impact of technological progress on agricultural carbon emissions follows a more nuanced pattern. The coexistence of positive and negative effects indicates that technological advancement in agriculture should not be viewed through a single lens like existing literatures (Shan et al., 2022; Liu et al., 2023; Wang et al., 2024; Xie et al., 2021; Li and Wang, 2023). Furthermore, the regional heterogeneity identified in this study reflects the structural differences in China's agricultural development. This study reveals two distinctive characteristics in regional heterogeneity patterns. First, the spatial distribution demonstrates a clear “stronger South, weaker North” pattern, which corroborates the findings of He and Ding (2023). However, their study did not conduct an in-depth analysis from both positive and negative effect dimensions. Consequently, they failed to capture a significant characteristic: the unique “dual advantage” possessed by the southwestern region. The temporal evolution of technological effects, particularly the significant divergence observed after 2011, coincides with China's agricultural policy transformation period. This temporal pattern suggests that the effectiveness of agricultural technology in reducing carbon emissions is not merely a function of technological capability but is deeply embedded in the broader institutional and policy environment. The finding contributes to the institutional perspective in agricultural environmental economics. The study also reveals an interesting paradox regarding human capital levels. Contrary to conventional view (Yao et al., 2021), regions with lower human capital levels demonstrated more significant carbon reduction effects in recent years. This unexpected finding suggests that the relationship between human capital and environmental performance in agriculture might be more complex than previously thought, possibly involving factors such as risk aversion, traditional farming wisdom, and local ecological knowledge.
These findings collectively suggest that the path toward agricultural carbon reduction requires a more sophisticated understanding of the interplay between technological progress, institutional arrangements, and local conditions. Future research could benefit from exploring these interactions through more nuanced theoretical frameworks and methodological approaches.
As global climate change intensifies, China, the world's largest carbon emitter, faces significant pressure to reduce emissions. The agricultural sector, a major source of carbon emissions, has garnered attention for its potential in carbon reduction. This study focuses on the impact of agricultural technological progress on agricultural carbon emissions, aiming to investigate whether it can effectively reduce agricultural carbon emission intensity and the underlying mechanisms. The research first analyzes the double-edged effect of agricultural technological progress on carbon emissions from a theoretical perspective. It then employs panel data from 30 Chinese provinces for the period 2002–2021, utilizing the DEA-Malmquist index to measure agricultural total factor productivity and the emission factor method to calculate agricultural carbon emissions. The 2TSF models are constructed to empirically analyze the impact of agricultural technological progress on agricultural carbon emission intensity.
Key conclusions include: (1) Agricultural technological progress exhibits a significant double-edged effect on carbon emission intensity. Empirical results show that the positive effect increases intensity by 9.01% on average, while the negative effect reduces it by 12.18%. Overall, the negative effect outweighs the positive, resulting in an average net reduction of 3.17% in agricultural carbon emission intensity. (2) Different types of agricultural technological progress have varying impacts on carbon emission intensity. Mechanical progress significantly increases intensity, while resource-saving and biochemical advancements reduce it. (3) The impact of agricultural technological progress on carbon emission intensity shows notable regional heterogeneity. The net effect is negative in Southwest, South, Central, Northeast, and Northwest China, indicating a reduction in emission intensity. However, North and East China exhibit positive net effects, suggesting increased emission intensity due to technological progress. (4) Farm operation scale, fiscal support for agriculture, and agricultural human capital levels significantly influence the carbon reduction effect of technological progress. Provinces with smaller-scale operations, higher fiscal support, and lower human capital levels demonstrate more pronounced carbon reduction effects. (5) Time series analysis reveals that from 2002 to 2021, the net effect of China's agricultural technological progress on carbon emission intensity remained negative, consistently contributing to intensity reduction. Since 2011, the carbon reduction efficacy has increased annually, with 2021 showing the largest increase.
Based on the conclusions drawn, the impact of agricultural technological progress on agricultural carbon emission intensity varies significantly across regions, necessitating targeted and precise policy interventions. To leverage the role of agricultural technological advancement in reducing carbon emissions, promote green agricultural transformation, and contribute to China's agricultural “dual carbon” goals, the following policy recommendations are proposed:
Firstly, implement differentiated agricultural technology promotion strategies. For regions with larger farm sizes and higher agricultural human capital levels, governments should increase support for high-tech research and development. These areas typically have better infrastructure and financial resources to facilitate the adoption of low-carbon technologies. Governments can establish research funding programs, technical training, and incentive mechanisms to accelerate the dissemination of new technologies and further reduce agricultural carbon emission intensity. For regions with lower agricultural economic development and human capital levels, the focus should be on food production security and sustainable agricultural development. While ensuring food security, these regions can learn from successful experiences elsewhere and gradually introduce proven low-carbon agricultural technologies. Governments should provide technical training, financial support, and policy guidance to assist these areas in achieving green agricultural transformation and improving production efficiency while reducing carbon emissions.
Secondly, incentivize low-carbon agricultural technology innovation and application. The government can encourage agricultural innovation through increased fiscal subsidies and dedicated research and development funds. Environmental sustainability should be embedded in the design, development, and promotion of new technologies to ensure both economic and ecological benefits. Emphasis should be placed on developing disruptive carbon reduction technologies that are both cost-effective and user-friendly. Collaboration with agricultural extension departments and research institutions should be strengthened through regular seminars, exhibitions, and training programs. This will enable farmers to access the latest research findings and market information, shortening the cycle from technology development to practical application.
Thirdly, develop “carbon-smart” agricultural management systems tailored to regional characteristics. Given the significant regional heterogeneity in the carbon reduction effects of agricultural technological progress, it is crucial to establish region-specific agricultural management systems. These systems should integrate carbon emission monitoring, technological assessment, and decision-making support functions. For regions like the Southwest that demonstrate strong negative effects, the focus should be on preserving and enhancing their existing advantages in resource-saving and biochemical technologies. For regions showing positive net effects, such as North and East China, the systems should prioritize the transformation of mechanical technologies toward more environmentally friendly alternatives. Local governments should establish carbon emission monitoring platforms that can provide real-time data on agricultural carbon emissions, helping stakeholders make informed decisions about technology adoption and agricultural practices. This approach aligns with the study's finding that different types of agricultural technologies have varying impacts on emission intensity, with mechanical technologies increasing emissions while resource-saving and biochemical technologies reducing them.
Finally, enhance agricultural human capital to drive green agricultural development. Governments can strengthen cooperation between agricultural universities and research institutions to promote talent cultivation and research commercialization. The China Ancient Village Science and Technology Institute, established by China Agricultural University, Yunnan Agricultural University, and Dali Prefecture Government, serves as an exemplary model. It aims to protect Erhai Lake while promoting farmers' income and agricultural green transformation. This institute has made significant contributions to China's high-quality agricultural development and can be replicated nationwide.
Regarding the measurement of agricultural technological progress, future studies should consider incorporating undesirable outputs into the evaluation system. Additionally, the calculation of agricultural carbon emissions should account for regional and temporal variations in emission coefficients to improve accuracy. Future research should focus on refining these methodologies to provide more precise assessments of agricultural carbon emissions and technological progress.
This study has certain limitations. Due to data constraints, the carbon emission sources selected in this research are not sufficiently comprehensive. Additionally, the two-tier stochastic frontier model makes specific assumptions about the distribution of random error terms, which may not always align with empirical data. Future research directions could focus on expanding carbon emission sources and refining econometric methodologies.
Publicly available datasets were analyzed in this study. This data can be found here: China Statistical Yearbook, https://www.stats.gov.cn/sj/ndsj/2023/indexeh.htm.
SZ: Conceptualization, Formal analysis, Funding acquisition, Methodology, Project administration, Writing – original draft. YL: Formal analysis, Methodology, Writing – review & editing. SW: Data curation, Formal analysis, Writing – review & editing. JL: Supervision, Writing – review & editing. JC: Methodology, Software, Writing – review & editing. WY: Conceptualization, Funding acquisition, Project administration, Software, Visualization, Writing – review & editing.
The author(s) declare that no financial support was received for the research, authorship, and/or publication of this article.
This work was supported by the Office of Research Administration, Chiang Mai University, and was also supported by the China–ASEAN High-Quality Development Research Center at the Shandong University of Finance and Economics. In addition, thanks to the Claude 3.5 Sonnet model for assisting in polishing the English sections of this article.
The authors declare that the research is conducted in the absence of any commercial or financial relationships that might be construed as a potential conflict of interest.
The author(s) declared that they were an editorial board member of Frontiers, at the time of submission. This had no impact on the peer review process and the final decision.
All claims expressed in this article are solely those of the authors and do not necessarily represent those of their affiliated organizations, or those of the publisher, the editors and the reviewers. Any product that may be evaluated in this article, or claim that may be made by its manufacturer, is not guaranteed or endorsed by the publisher.
Acemoglu, D., Akcigit, U., and Celik, M. A. (2022). Radical and incremental innovation: the roles of firms, managers, and innovators. Am. Econ. J. 14, 199–249. doi: 10.1257/mac.20170410
Anser, M. K., Ahmad, M., Khan, M. A., Zaman, K., Nassani, A. A., Askar, S. E., et al. (2021). The role of information and communication technologies in mitigating carbon emissions: evidence from panel quantile regression. Environ. Sci. Pollut. Res. 28, 21065–21084. doi: 10.1007/s11356-020-12114-y
Bachleitner, S., Ata, Ö., and Mattanovich, D. (2023). The potential of CO2-based production cycles in biotechnology to fight the climate crisis. Nat. Commun. 14:6978. doi: 10.1038/s41467-023-42790-6
Chang, H., Heerink, N., Wu, W., and Zhang, J. (2022). More use or cleaner use? Income growth and rural household energy-related carbon emissions in central China. Energy Sustain. Dev. 70, 146–159. doi: 10.1016/j.esd.2022.07.013
Charnes, A., Cooper, W. W., and Rhodes, E. (1978). Measuring the efficiency of decision making units. Eur. J. Oper. Res. 2, 429–444. doi: 10.1016/0377-2217(78)90138-8
Chen, J., Wang, S., Zhong, H., Chen, B., and Fang, D. (2024). Assessing agricultural greenhouse gas emission mitigation by scaling up farm size: an empirical analysis based on rural household survey data. Sci. Total Environ. 933:173077. doi: 10.1016/j.scitotenv.2024.173077
Cillis, D., Maestrini, B., Pezzuolo, A., Marinello, F., and Sartori, L. (2018). Modeling soil organic carbon and carbon dioxide emissions in different tillage systems supported by precision agriculture technologies under current climatic conditions. Soil Tillage Res. 183, 51–59. doi: 10.1016/j.still.2018.06.001
Dosi, G., Piva, M., Virgillito, M. E., and Vivarelli, M. (2021). Embodied and disembodied technological change: the sectoral patterns of job-creation and job-destruction. Res. Policy 50:104199. doi: 10.1016/j.respol.2021.104199
Duan, H., Zhang, Y., and Zhao, J. (2011). Analysis of the carbon footprint of China's farmland ecosystem. J. Soil Water Conserv. 25, 203–208. doi: 10.13870/j.cnki.stbcxb.2011.05.020
Fan, D. S. (2022). Advancements in agricultural technology, optimization of agricultural structure, and intensity of agri-cultural carbon emissions. Stat. Decis. Mak. 38, 154–158. doi: 10.13546/j.cnki.tjyjc.2022.20.030
Fare, R., Grosskopf, S., and Lovell, C. K. (1994). Production Frontiers. Cambridge: Cambridge University Press. doi: 10.1017/CBO9780511551710
Gallardo, R. K., and Sauer, J. (2018). Adoption of labor-saving technologies in agriculture. Ann. Rev. Resour. Econ. 10, 185–206. doi: 10.1146/annurev-resource-100517-023018
Gao, J., Gao, F., Yin, B., and Zhang, M. (2021). International trade as a double-edged sword: the perspective of carbon emissions. Front. Energy Res. 9:764914. doi: 10.3389/fenrg.2021.764914
Gao, Y., and Cabrera Serrenho, A. (2023). Greenhouse gas emissions from nitrogen fertilizers could be reduced by up to one-fifth of current levels by 2050 with combined interventions. Nature Food 4, 170–178. doi: 10.1038/s43016-023-00698-w
Gerlagh, R. (2007). Measuring the value of induced technological change. Energy Policy 35, 5287–5297. doi: 10.1016/j.enpol.2006.01.034
Gorli, R. (2017). Future of smart farming with internet of things. J. Agric. Water Works Eng. 1, 1–12.
Guan, N., Liu, L., Dong, K., Xie, M., and Du, Y. (2023). Agricultural mechanization, large-scale operation and agricultural carbon emissions. Cogent Food Agric. 9:2238430. doi: 10.1080/23311932.2023.2238430
Guo, L., Guo, S., Tang, M., Su, M., and Li, H. (2022). Financial support for agriculture, chemical fertilizer use, and carbon emissions from agricultural production in China. Int. J. Environ. Res. Public Health 19:7155. doi: 10.3390/ijerph19127155
He, H., and Ding, R. (2023). Spatiotemporal heterogeneity effect of technological progress and agricultural centrality on agricultural carbon emissions in China. Front. Environ. Sci. 10:1078357. doi: 10.3389/fenvs.2022.1078357
Hu, Y., Zhang, K., Hu, N., and Wu, L. (2023). Review on measurement of agricultural carbon emission in China. Chinese J. Eco-Agric. 31, 163–176.
Huang, H. F., and Zhu, N. (2022). Rural financial efficiency, agricultural technological progress and agricultural carbon emissions: evidence from China. Nat. Environ. Pollut. Technol. 21, 61–69. doi: 10.46488/NEPT.2022.v21i01.007
Ismael, M., Srouji, F., and Boutabba, M. A. (2018). Agricultural technologies and carbon emissions: evidence from Jordanian economy. Environ. Sci. Pollut. Res. 25, 10867–10877. doi: 10.1007/s11356-018-1327-5
Jha, P., Chinngaihlian, S., Upreti, P., and Handa, A. (2023). A machine learning approach to assess implications of Climate Risk Factors on Agriculture: the Indian case. Clim. Risk Manag. 41:100523. doi: 10.1016/j.crm.2023.100523
Kirat, Y., Prodromou, T., and Suardi, S. (2024). Unveiling the Nexus: Climate change, green innovation, and the pendulum of energy consumption and carbon emissions. Energy Econ. 138:107727. doi: 10.1016/j.eneco.2024.107727
Koondhar, M. A., Udemba, E. N., Cheng, Y., Khan, Z. A., Koondhar, M. A., Batool, M., et al. (2021). Asymmetric causality among carbon emission from agriculture, energy consumption, fertilizer, and cereal food production–a nonlinear analysis for Pakistan. Sustain. Energy Technol. Assessm. 45:101099. doi: 10.1016/j.seta.2021.101099
Kumbhakar, S. C., and Parmeter, C. F. (2009). The effects of match uncertainty and bargaining on labor market outcomes: evidence from firm and worker specific estimates. J. Product. Anal. 31, 1–14. doi: 10.1007/s11123-008-0117-3
Lauk, C., Magerl, A., Le Noë, J., Theurl, M. C., and Gingrich, S. (2024). Analyzing long-term dynamics of agricultural greenhouse gas emissions in Austria, 1830–2018. Sci. Total Environ. 911:168667. doi: 10.1016/j.scitotenv.2023.168667
Lavanya, G., Rani, C., and Ganeshkumar, P. (2019). An automated low cost IoT based Fertilizer Intimation System for smart agriculture. Sustain. Comput. Inform Syst. 28:100300. doi: 10.1016/j.suscom.2019.01.002
Li, S., and Wang, Z. (2023). The effects of agricultural technology progress on agricultural carbon emission and carbon sink in China. Agriculture 13:793. doi: 10.3390/agriculture13040793
Liu, J., Yuan, Y., Lin, C., and Chen, L. (2023). Do agricultural technical efficiency and technical progress drive agricultural carbon productivity? Based on spatial spillovers and threshold effects. Environ. Dev. Sustain. 2023, 1–25. doi: 10.1007/s10668-023-04217-6
Liu, Y., Yao, X., and Wei, T. (2019). Energy efficiency gap and target setting: a study of information asymmetry between governments and industries in China. China Econ. Rev. 57:101341. doi: 10.1016/j.chieco.2019.101341
Lu, Y., Liu, M., Li, C., Liu, X., Cao, C., Li, X., et al. (2022). Precision fertilization and irrigation: progress and applications. AgriEngineering 4, 626–655. doi: 10.3390/agriengineering4030041
Ma, G., Dai, X., and Luo, Y. (2023). The effect of farmland transfer on agricultural green total factor productivity: evidence from rural China. Int. J. Environ. Res. Public Health 20:2130. doi: 10.3390/ijerph20032130
Malmquist, S. (1953). Index numbers and indifference surfaces. Trabajos Estadistica 4, 209–242. doi: 10.1007/BF03006863
Mantoam, E. J., Angnes, G., Mekonnen, M. M., and Romanelli, T. L. (2020). Energy, carbon and water footprints on agricultural machinery. Biosyst. Eng. 198, 304–322. doi: 10.1016/j.biosystemseng.2020.08.019
Papadopoulos, A. (2021). Measuring the effect of management on production: a two-tier stochastic frontier approach. Empir. Econ. 60, 3011–3041. doi: 10.1007/s00181-020-01946-9
Parmeter, C. F. (2018). Estimation of the two-tiered stochastic frontier model with the scaling property. J. Prod. Anal. 49, 37–47. doi: 10.1007/s11123-017-0520-8
Pongnumkul, S., Chaovalit, P., and Surasvadi, N. (2015). Applications of smartphone-based sensors in agriculture: a systematic review of research. J. Sensors 2015:195308. doi: 10.1155/2015/195308
Prastiyo, S. E., Irham, Hardyastuti, S., and Jamhari, F. (2020). How agriculture, manufacture, and urbanization induced carbon emission? The case of Indonesia. Environ. Sci. Pollut. Res. 27, 42092–42103. doi: 10.1007/s11356-020-10148-w
Qin, J., Duan, W., Zou, S., Chen, Y., Huang, W., and Rosa, L. (2024). Global energy use and carbon emissions from irrigated agriculture. Nat. Commun. 15:3084. doi: 10.1038/s41467-024-47383-5
Shabani, Z. D. (2024). Renewable energy and CO2 emissions: does human capital matter? Energy Rep. 11, 3474–3491. doi: 10.1016/j.egyr.2024.03.021
Shan, T., Xia, Y., Hu, C., Zhang, S., Zhang, J., Xiao, Y., et al. (2022). Analysis of regional agricultural carbon emission efficiency and influencing factors: case study of Hubei Province in China. PLoS ONE 17:e0266172. doi: 10.1371/journal.pone.0266172
Shih, H. Y., and Chang, T. L. S. (2009). International diffusion of embodied and disembodied technology: a network analysis approach. Technol. Forecast. Soc. Change 76, 821–834. doi: 10.1016/j.techfore.2008.09.001
Smith, P., Bustamante, M., Ahammad, H., Clark, H., Dong, H., Elsiddig, E. A., et al. (2014). “Agriculture, forestry and other land use (AFOLU),” in Climate Change 2014: Mitigation of Climate Change. Contribution of Working Group III to the Fifth Assessment Report of the Intergovernmental Panel on Climate Change (Cambridge University Press), 811–922. doi: 10.1017/CBO9781107415416.017
Stetter, C., and Sauer, J. (2022). Greenhouse gas emissions and eco-performance at farm level: a parametric approach. Environ. Resour. Econ. 81, 617–647. doi: 10.1007/s10640-021-00642-1
Verteramo Chiu, L. J., Tauer, L. W., and Gröhn, Y. T. (2022). Pricing efficiency in livestock auction markets: A two-tier frontier approach. Agric. Econ. 53, 139–151. doi: 10.1111/agec.12735
Wang, M., Liu, J., Rahman, S., Sun, X., and Sriboonchitta, S. (2023). The effect of China's outward foreign direct investment on carbon intensity of Belt and Road Initiative countries: a double-edged sword. Econ. Anal. Policy 77, 792–808. doi: 10.1016/j.eap.2022.12.030
Wang, Y., Zhang, T., Wang, X., Jiang, B., and Huang, X. (2024). Impact of technical progress and fiscal support to agriculture on agricultural carbon emissions. Cogent Food Agric. 10:2300186. doi: 10.1080/23311932.2023.2300186
Wortmann, F., and Flüchter, K. (2015). Internet of things. Bus. Inf. Syst. Eng. 57, 221–224. doi: 10.1007/s12599-015-0383-3
Xia, L., Lam, S. K., Chen, D., Wang, J., Tang, Q., and Yan, X. (2017). Can knowledge-based N management produce more staple grain with lower greenhouse gas emission and reactive nitrogen pollution A meta-analysis. Glob. Change Biol. 23, 1917–1925.
Xie, C., and Li, L. (2018). The empirical test on investment efficiency and influence of equity incentive in supply-side structural reform: Based on the two-tier stochastic frontier approach. Appl. Stochastic Models Bus. Ind. 34, 730–745. doi: 10.1002/asmb.2304
Xie, Z., Wu, R., and Wang, S. (2021). How technological progress affects the carbon emission efficiency? Evidence from national panel quantile regression. J. Clean. Prod. 307:127133. doi: 10.1016/j.jclepro.2021.127133
Xu, C., Yang, L., Zhang, B., and Song, M. (2021). Bargaining power and information asymmetry in China's water market: an empirical two-tier stochastic frontier analysis. Empir. Econ. 61, 2395–2418. doi: 10.1007/s00181-020-01972-7
Yao, Y., Zhang, L., Salim, R., and Rafiq, S. (2021). The effect of human capital on CO2 emissions: macro evidence from China. Energy J. 42, 91–120. doi: 10.5547/01956574.42.6.yyao
Yrjälä, K., Ramakrishnan, M., and Salo, E. (2022). Agricultural waste streams as resource in circular economy for biochar production towards carbon neutrality. Curr. Opin. Environ. Sci. Health 26:100339. doi: 10.1016/j.coesh.2022.100339
Zang, D., Hu, Z., Yang, Y., and He, S. (2022). Research on the relationship between agricultural carbon emission intensity, agricultural economic development and agricultural trade in China. Sustainability 14:11694. doi: 10.3390/su141811694
Zhang, H., Chen, Z., Wang, J., Wang, H., and Zhang, Y. (2023). Spatial-temporal pattern of agricultural Total factor productivity change (Tfpch) in China and its implications for agricultural sustainable development. Agriculture 13:718. doi: 10.3390/agriculture13030718
Zhang, Y. X. (2015). The detrimental impacts of monoculture on nature and society. Sci. Plant. Breed. 11, 57–58. doi: 10.1111/jipb.12431
Keywords: TFP, agricultural technological progress, agricultural carbon emissions, two-tier stochastic frontier models, carbon intensity
Citation: Zhu S, Li Y, Wang S, Liu J, Cui J and Yamaka W (2024) The double-edged sword effect of agricultural technological progress on agricultural carbon emissions: empirical evidence from China. Front. Sustain. Food Syst. 8:1489448. doi: 10.3389/fsufs.2024.1489448
Received: 01 September 2024; Accepted: 29 November 2024;
Published: 19 December 2024.
Edited by:
Jiachao Peng, Wuhan Institute of Technology, ChinaReviewed by:
Fuyou Guo, Qufu Normal University, ChinaCopyright © 2024 Zhu, Li, Wang, Liu, Cui and Yamaka. This is an open-access article distributed under the terms of the Creative Commons Attribution License (CC BY). The use, distribution or reproduction in other forums is permitted, provided the original author(s) and the copyright owner(s) are credited and that the original publication in this journal is cited, in accordance with accepted academic practice. No use, distribution or reproduction is permitted which does not comply with these terms.
*Correspondence: Woraphon Yamaka, d29yYXBob24uZWNvbkBnbWFpbC5jb20=
Disclaimer: All claims expressed in this article are solely those of the authors and do not necessarily represent those of their affiliated organizations, or those of the publisher, the editors and the reviewers. Any product that may be evaluated in this article or claim that may be made by its manufacturer is not guaranteed or endorsed by the publisher.
Research integrity at Frontiers
Learn more about the work of our research integrity team to safeguard the quality of each article we publish.