- 1College of Science and Technology, Hebei Agricultural University, Huanghua, Hebei, China
- 2Intensive Care Unit of Wangjing Hospital, China Academy of Chinese Medical Sciences, Beijing, China
- 3College of Life Science, CangZhou Normal University, Cangzhou, Hebei, China
- 4College of Chemistry and Chemical Engineering, Cangzhou Normal University, Cangzhou, China
Millet is one of the major coarse grain crops in China. Its geographical origin and Fusarium fungal contamination with ergosterol and deoxynivalenol have a direct impact on food quality, so the rapid prediction of the geographical origins and fungal toxin contamination is essential for protecting market fairness and consumer rights. In this study, 600 millet samples were collected from twelve production areas in China, and traditional algorithms such as random forest (RF) and support vector machine (SVM) were selected to compare with the deep learning models for the prediction of millet geographical origin and toxin content. This paper firstly develops a deep learning model (wavelet transformation-attention mechanism long short-term memory, WT-ALSTM) by combining hyperspectral imaging to achieve the best prediction effect, the wavelet transformation algorithm effectively eliminates noise in the spectral data, while the attention mechanism module improves the interpretability of the prediction model by selecting spectral feature bands. The integrated model (WT-ALSTM) based on selected feature bands achieves optimal prediction of millet origin, with its accuracy exceeding 99% on both the training and prediction datasets. Meanwhile, it achieves optimal prediction of ergosterol and deoxynivalenol content, with the coefficient of determination values exceeding 0.95 and residual predictive deviation values reaching 3.58 and 3.38 respectively, demonstrating excellent model performance. The above results suggest that the combination of hyperspectral imaging with a deep learning model has great potential for rapid quality assessment of millet. This study provides new technical references for developing portable and rapid hyperspectral imaging inspection technology for on-site assessment of agricultural product quality in the future.
1 Introduction
Millet (Setaria italica (L.) Beauv.) is the seed of the grass species in the family of Poaceae, and it is considered one of the oldest cultivated crops (Yang et al., 2012). It originated in the Yellow River basin of China and became one of the major cereal crops in ancient China. At present, China is the main production area for millet, accounting for 80% of the world’s production (Wu and Qu, 2018). Millet has rich nutrients and provides various essential amino acids, fats, vitamins, minerals, and other nutritional components for the human body (He et al., 2007; Dasa and Nguyen, 2020; Yang et al., 2021; Shi et al., 2023). Many pharmacological studies have indicated that millet has various health benefits, including lowering blood glucose levels (Balli et al., 2023), anti-tumor properties (Saleem et al., 2023), reducing cholesterol levels, as well as anti-inflammatory effects (Onipe and Ramashia, 2022). Attributed to its combined medicinal and nutritional value, millet is highly favored in several regions of China (Mahajan et al., 2021).
Millet from different regions differs in quality and price. In China, the five provinces of Shaanxi, Shanxi, Gansu, Ningxia, and Inner Mongolia account for 15.6% of the national millet production (Yang et al., 2019), Hebei, Shandong, and Henan provinces account for 64.3% of the national millet production, while the three northeastern provinces (Heilongjiang, Jilin, and Liaoning) account for 13.9% of the national millet production (Wu and Qu, 2018). The Wu’an millet (HBWA) from Wu’an City, Hebei Province, the Chaoyang millet (LNCY) from Chaoyang City, Liaoning Province, and the Qinzhou millet (SXQX) from Qinxian County, Shanxi Province are certified as Protected Geographical Indication (PGI) products in China. These three production areas have unique natural environments such as altitude, climate, and soil, along with strict and standardized planting regulations, so millet products in these areas have better quality and higher market value. However, there are often cases in the market where inferior products are sold as high-quality ones and non-PGI products from other regions are falsely labeled as PGI products, seriously disrupting market order. Therefore, it is of great significance to implement source tracing and brand protection measures for millet products.
Pathogenic fungi of the Fusarium genus are common in the production of grains such as millet (Femenias et al., 2021; Teixido-Orries et al., 2023). Infection by Fusarium species usually leads to a sharp decrease in crop yield, and the fungal toxin residues caused by Fusarium microbial contamination have a serious impact on the quality of millet, ultimately leading to agricultural economic losses (Dowell et al., 1999). The number of microorganisms of the Fusarium genus attached to the surface of grains is linearly related to the content of ergosterol (C28H44O, ZC), a metabolite produced by these microorganisms. The ZC content in grains is widely adopted as an important criterion for evaluating the degree of fungal contamination and grain quality (He et al., 2007). Meanwhile, deoxynivalenol (C15H20O6, DON), also known as vomitoxin, is a mycotoxin secreted by microorganisms of Fusarium species. DON is widely present in millet grains and has high toxicity. It can cause vomiting, diarrhea, miscarriage, and other damage to humans and animals. The World Health Organization has identified DON as one of the high-risk food contaminants (Yao and Long, 2020; Zhao et al., 2020). Therefore, efficient and rapid detection of ZC and DON content in millet grains has great significance for determining the degree of Fusarium fungal contamination and ensuring millet quality and food safety.
Traditional methods for determining the origin of agricultural products mainly rely on the mineral element content of the target, stable isotope ratios with regional variations (Wang et al., 2022b), chemical fingerprinting of the target substance (Yan et al., 2023), etc. The conventional quantitative detection methods for the content prediction of low-concentration toxins of DON and ZC mainly include high-performance liquid chromatography (HPLC), high-performance liquid chromatography-mass spectrometry tandem (HPLC-MS) (Antonios et al., 2010), enzyme-linked immunosorbent assay (ELISA), thin layer chromatography (TLC) (Rocha et al., 2017), etc. Though these evaluation methods have advantages such as high accuracy and sensitivity, they have some defects, including sample destruction, high detection costs, low time efficiency (inability to conduct batch testing in a single run), and environmental contamination due to the use of organic reagents (Wang et al., 2024a; Wang et al., 2024b). These issues should not be ignored.
The external environmental factors of millet planting include soil and climate in the production area, which lead to differences in crop chemical fingerprint spectra (Lu et al., 2014). Meanwhile, fungal contamination causes the denaturation of chemical nutrients, resulting in significant differences in hyperspectral characteristics (Teixido-Orries et al., 2023). Hyperspectral imaging (HSI) technology could produce chemical reflectance data across hundreds of bands to reflect the physical and chemical information of the measured samples (An et al., 2023). In recent years, HSI technology has gained increasing popularity as a rapid inspection technique that can meet the demands of today’s market for fast and batch testing. HSI has great advantages such as non-destructive sample detection, high throughput, fast detection speed, and environmental friendliness in experimental techniques (Wang et al., 2024a; Wang et al., 2024b). At present, combining HSI technology with classic machine learning algorithms is a common method for the rapid prediction of geographical origins and chemical compositions of various agricultural products. Traditional machine learning algorithms, such as PLSDA (partial least squares discriminant analysis), random forest (RF) and support vector machine (SVM) have achieved generally satisfactory results in previous hyperspectral research, and they have obvious advantages such as short training time, simple computation, and strong generalization ability. HSI combined with models such as RF and SVM has been successfully applied to the identification of origins for cereal of Coix seeds (Wang et al., 2023) as well as to the quality or variety identification of small grains such as wheat (Safdar et al., 2023), oats (Teixido-Orries et al., 2023), and sorghum (Bu et al., 2023). The combination of HSI with chemometric models like partial least squares regression (PLSR) has been applied to the prediction of DON and ZC content in corn and wheat (Femenias et al., 2021; Borras-Vallverdu et al., 2024), various saponin content in ginseng (Wang et al., 2024a; Wang et al., 2024b), as well as starch and protein content in Coix seeds (Wang et al., 2023). However, it is worth noting that traditional model parameter optimization involves a certain degree of human subjectivity, and model optimization often cannot take into account data feature extraction to improve model performance (Wang et al., 2023; Zhang et al., 2020). Deep learning based on neural networks can address the above concerns.
Currently, combining HSI with artificial intelligence deep learning models to predict the quality of agricultural products has been a prominent research focus. Compared to traditional machine learning algorithms (including RF and SVM), deep learning models, like recurrent neural networks (RNNs) and long short-term memory (LSTM), have more evident advantages such as self-learning, self-reasoning, and no need for subjective parameter selection. As a result, these models can make more objective and reliable predictions than traditional algorithms (Li et al., 2021). Existing research has shown that using feature data extracted from HSI to reduce the dimensionality of hyperspectral data can simplify model calculations and improve prediction efficiency (Wang et al., 2022d; Wang et al., 2024a). Meanwhile, by assigning variable weights to HSI bands, the application of deep learning attention mechanism (AM) modules allows for the selection of important feature wavelengths. This enhances not only the prediction performance of the model but also the interpretability of deep learning (Wang et al., 2023). Additionally, denoising complex and redundant hyperspectral data across multiple bands provides an important means for improving model prediction effectiveness (Wang et al., 2022c; Wang et al., 2022d). However, there is a lack of research both domestically and internationally on the application of HSI technology combined with deep learning models, including data denoising, to the prediction of millet origin, fungal microbial contamination, and toxin content.
Considering this, this study aims to: (1) establish a large-scale hyperspectral database for millet from multiple origins; (2) compare the effectiveness of LSTM with traditional machine learning models such as RF, SVM, and partial least squares discriminant analysis (PLSDA or PLSR) in predicting the origin of millet and the content of fungal toxins, to determine the optimal prediction model; (3) reveal the effects of combining different denoising methods, including multiplicative scatter correction (MSC), wavelet transformation (WT), and standard normal variate (SNV), with prediction models, and determine the optimal combination; (4) evaluate the effectiveness of the deep learning AM algorithm in selecting feature bands, to provide more options for reducing the dimensionality of HSI data and improving the interpretability of prediction models in the future. This study attempts to achieve rapid quality inspection of millet by combining HSI with deep learning models, thereby providing references for the further development of portable, intelligent agricultural product inspection equipment in the future.
2 Materials and methods
2.1 Sample collection and preparation
Millet samples were collected from 12 major production areas in 9 provinces of China from October 2022 to January 2023, including three high-quality samples from regions designated with PGI status (Table 1). In the sample collection, uniformly sized and clean millet seeds were selected and naturally air-dried. All the samples were collected from 10 plots in each production area, and 5 parallel samples (500 g each) were selected from each plot. Finally, 50 samples (10 × 5) were collected from each production area, and 600 sets of HSI data (10 × 5 × 12) were acquired from all 12 production areas. In terms of origin prediction, to ensure the stability of the samples, the test samples are stored at a low temperature of 4°C for a long time. Regarding fungal toxin contamination, millet samples were processed in a controlled environment with a temperature of 20°C and a humidity of 70% for 30 days, resulting in toxin enrichment. After data collection, all samples were processed into 50-mesh powder (stored at a low temperature of 4°C) for subsequent chemical content analysis (600 sets) to benchmark and correct the prediction effect of the established model.
2.2 Acquisition of hyperspectral data
Hyperspectral data was collected using a visible and shortwave/longwave near-infrared imaging spectrometer (VIS-NIR-HSI, HySpex VNIR-1800/HySpex SWIR 384, Norsk ElektroOptikk, Oslo, Norway). The VIS-NIR-HSI spectrometer has a wavelength range of 350–1000 nm covering visible light and short wave near-infrared parts. The SWIR spectrometer has a wavelength range of 900–2550 nm covering short and middle short-wave near-infrared parts, showing higher sensitivity to organic compounds. The spectrometer mainly consists of two tungsten halogen lamps (150 W/12 V, H-LAM, Norsk ElektroOptikk, Oslo, Norway), two lenses (VNIR and SWIR), a conveyor belt, and a data analysis computer. The two tungsten halogen lamps were used as light sources, angled at 45 degrees. The two lenses, VNIR and SWIR, were set up with exposure times of 3.5 ms and 4.5 ms, respectively. The spectral resolution was approximately 5 nm, and the samples were positioned 22 cm from the lenses. The conveyor belt moved at a speed of 2.5 mm/s. To avoid noise fluctuations at the edges of the wavelength range, the effective spectral information collected from 410 to 950 nm and 950 to 2500 nm, covering 396 bands in total, was manually merged. After the collection of the HSI data, black and white board correction was performed on the original hyperspectral images to eliminate the influence of external factors such as instrument and current on the sample data (Wang et al., 2023). Finally, ENVI 5.3 software (Research Systems Inc., Boulder, CO, United States) was employed to extract the regions of interest and calculate the relative reflectance of the regions of interest.
2.3 Measurement of ZC and DON content in millet samples
2.3.1 Extraction and HPLC quantification of ZC and DON in millet samples
As previously documented in literature, the main organic nutritional components of millet include protein (10–15%), amylose (10–28%), and fat (3–5%), leading to the frequent contamination with Fusarium fungal and high levels of ergosterol and deoxynivalenol content (He et al., 2007; Dasa and Nguyen, 2020; Yang et al., 2021; Shi et al., 2023). According to the operating procedures listed in GBT 25221-2010 (General Administration of Quality Supervision, 2011), ZC was extracted from millet samples followed by quantification. First, 5 g of the above-mentioned sample powder was taken, and through processes such as cold condensation reflux extraction, rotary evaporation concentration, and nitrogen blow drying, ZC extraction solution dissolved in n-hexane was obtained. Then, quantitative detection was performed using HPLC (1290, Agilent, United States) with an Inertsil ODS-3 silica column (length: 250 mm, inner diameter: 4.6 mm, particle size: 5 μm). The detection conditions were as follows: the mobile phase consisted of n-hexane: isopropanol = 99:1 (v/v), with an elution time of 18 min. The flow rate was set to 2 mL/min, the column temperature was 40°C, the detection wavelength was 282 nm, and the injection volume was 10 μL.
Following the method described in GB5009.111-2016 (General Administration of Quality Supervision, 2017) (2016), DON was extracted from the millet samples, and its content was determined. First, 2 g of the millet sample powder was placed in a 50 mL centrifuge tube, and 20 mL of acetonitrile-water solution (84% acetonitrile, v/v) was added. Then, the mixture was subjected to ultrasonic extraction for 20 min, followed by centrifugation at 10,000 r/min for 5 min to obtain the supernatant, which was reserved for detection. Next, the solution to be tested was purified using an immunoaffinity column (IAC-030-3, PriboLab, China). The column was eluted with methanol, and the eluate was subjected to nitrogen blow drying to obtain the DON extract. Subsequently, quantitative detection was performed using HPLC (1290, Agilent, United States) with a Waters ACQUITY UPLC HSS T3 column (2.1 mm × 100 mm, 1.8 μm, Waters, United States). The detection conditions were as follows: the mobile phase consisted of 80% methanol solution (methanol: water = 80:20, v/v), with an elution time of 20 min. The flow rate was set to 0.8 mL/min, the column temperature was 35°C, the detection wavelength was 218 nm, and the injection volume was 10 μL.
2.3.2 Preparation of standard solutions for ZC and DON
First, 20 mg of ZC standard (57-87-4, Sigma-Aldrich, United States) was dissolved in 100 mL of n-hexane to prepare the stock solution (200 μg/mL). Then, by using the purchased 200 μg/mL DON standard solution (CRM46911, Sigma-Aldrich, United States) and based on the test results of the mixed sample (20 samples were randomly selected from different origins), working solutions in the concentration range of 0.01–100 μg/mL were prepared using acetonitrile solvent to establish the standard quantitative curve. The detection limit and quantification limit of the method were determined based on a signal-to-noise ratio of 3:1 (Supplementary Table S1). Finally, the chemical indicator content of millet samples was determined based on the standard content curve.
2.4 Model data analysis
2.4.1 Spectral data preprocessing
In the modeling process, appropriate preprocessing methods are usually employed to mitigate noise interference during data collection, thereby improving model prediction effectiveness and stability. This study compared three spectral preprocessing methods: SNV, MSC, and WT. Among them, SNV and MSC are commonly utilized to eliminate scattering effects in spectral data, and they are widely used to perform scattering correction (Wu et al., 2019). WT, characterized by its high resolution and good time-frequency properties, performs a multiscale detailed analysis of signals through scaling and shifting operations, thus greatly reducing random noise to smooth the spectrum (He et al., 2018).
2.4.2 Prediction models for millet origin and chemical indicators
Four models, PLSDA, SVM, RF, and LSTM, were utilized in this study for origin prediction. Meanwhile, the prediction of the two chemical indicators was achieved using four regression models: PLSR, SVM, RF, and LSTM.
The PLSDA model explores the linear relationship between spectral data (X) and the predicted region (Y) based on the correlation between variables X and Y (Wang et al., 2023). In this study, leave-one-out cross-validation was employed to determine the optimal number of latent variables (between 5–10) for the PLSDA model based on the minimum root mean square error (RMSE) obtained through cross-validation. SVM is a classic machine learning method that constructs a hyperplane to achieve better separation of observations (Liang et al., 2020). It can well handle complex spectral data, including linear and nonlinear patterns (Yu et al., 2019). This study used the radial basis kernel function for SVM modeling. Optimization was conducted for both the penalty factor (C) and the kernel parameter (γ), with reference ranges defined as 100 to 2500 for C and 2−8 to 28 for γ. The RF model integrates the predictions of multiple decision trees through a majority voting scheme. It introduces two random factors, namely the number of trees (n-tree) and the number of variables to consider at each split (mtry), to enhance prediction accuracy and avoid overfitting. In this study, through leave-one-out cross-validation, it was determined that n-tree of 500 and mtry of 2 are suitable for balancing model accuracy and efficiency (Liu et al., 2020; Jia et al., 2021). The PLSR model, unlike PLSDA, is used to solve regression prediction problems. In the PLSR model, latent variables measure the covariance between the independent variables and the target variable to achieve higher prediction accuracy. In this study, leave-one-out cross-validation was employed, and based on the minimum RMSE value obtained through cross-validation, the optimal number of latent variables for the model was determined to range between 6 and 10.
The LSTM model is a classic deep-learning neural network that can capture long-term dependencies in information. It addresses the common issue of gradient vanishing or explosion in large amounts of spectral data by introducing gate mechanisms. In this study, an LSTM model with 64 hidden units was constructed, and its detailed architecture is illustrated in Figure 1. In the LSTM layer, two types of states were constructed: the output state (at time step t denoted as ht) and the cell state (at time step t denoted as ct). These states are controlled by input gates (it), forget gates (ft), cell candidate gates (gt), and output gates (ot). These gates enable the network to determine whether to discard or add information, thus forgetting and remembering the corresponding information. In the model training process, the LSTM model combines input weights, recurrent weights, and bias parameters with a dropout strategy to ensure a balance between performance and computational complexity, thus preventing overfitting (Mou et al., 2021). In the LSTM layer initializer, the parameter settings include “Orthogonal” and “Dropout rate = 0.2.” In the fully connected initializer, the parameter settings include “Kaiming” and “Bach size = 40.” The parameter settings of the optimizer are “Adam,” “Loss = MSE (content prediction),” and “Loss = Cross Entropy (origin prediction).”
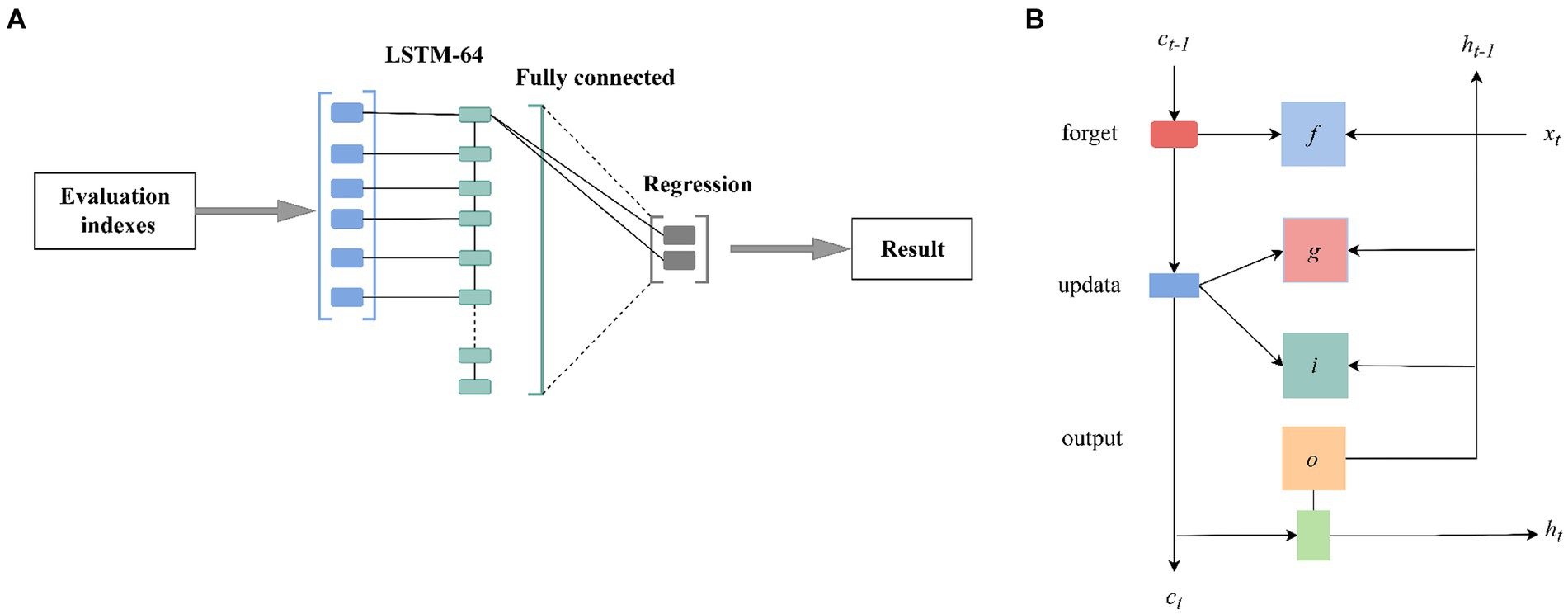
Figure 1. The structure of the LSTM model. The LSTM layers have 64 hidden units; two states, namely the output state (time step t as ht) and the cell state (time step t as ct), are constructed in the LSTM layer, and both states are controlled by the input gate (it), the forget gate (ft), the cell candidate (gt), and the output gate (ot).
The AM originated from research on human vision and can be employed to extract the important features for variable selection. The screening process of AM involves two steps: (1) calculating the attention distribution on all input information, and (2) computing the weighted average of input information based on the attention distribution (Fan et al., 2022) (Figure 2). AM can help the LSTM model eliminate redundant information based on the information content and importance of the target value, thereby enhancing the model’s generalization performance.
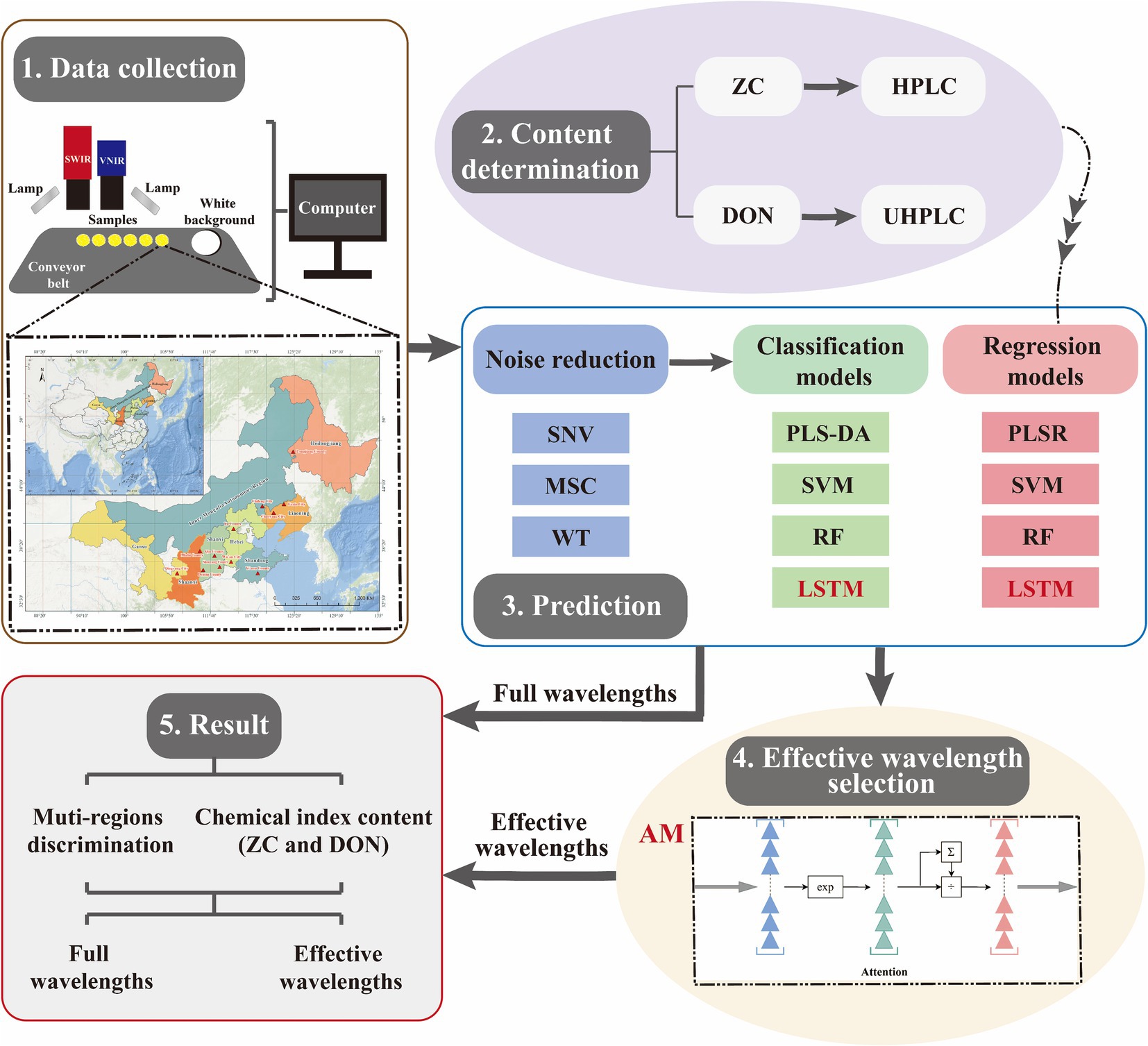
Figure 2. The workflow of the proposed method. It mainly includes spectral data acquisition, chemical composition analysis, model prediction (including data denoising, origin discrimination, and content regression prediction), and feature wavelength selection by the AM algorithm (AM focuses on the important part of the target while ignoring the rest by calculating the attention distribution and weighting the average value based on all input information).
2.4.3 Model data splitting and performance evaluation
In the model analysis, the SPXY algorithm (based on joint x–y distance) (Galvao et al., 2005) was utilized to randomly split the 600 samples (10 plots × 5 replicates × 12 origins) into a training set (420 samples) and a prediction set (180 samples) at a ratio of 7:3. The main advantage of the SPXY algorithm lies in its consideration of the variability of multidimensional spatial data, including both x and y dimensions. The model parameters were selected and optimized through 5-fold cross-validation. All models were implemented with Python 3.9 software in the Spyder environment. The performance of the models in predicting the origin of millet was evaluated in terms of the accuracy on the prediction set. For content prediction, metrics such as mean absolute error (MAE), coefficient of determination (R), RMSE, and residual predictive deviation (RPD) were employed to evaluate the performance of the regression models (Wang et al., 2023).
3 Results
3.1 Explicable wavelengths selected via attention mechanism in origin discrimination and chemical content prediction
By using the AM algorithm to assign weights to all bands, the top 15 variables with higher weights were selected for predicting the origin and the content of the two chemical indicators in millet (as shown in Figure 3). As for origin prediction, the feature bands are related to the differences in the main organic compound content of millet from different production areas. Specifically, the wavelengths at 998 nm are related to the third overtone region of –CH (CH/CH2/CH3) and the third overtone region of –OH from oil nutrients (Weinstock et al., 2006; Balbino et al., 2022); the wavelengths at 1156 nm and 1521 nm are, respectively, related to the second overtone regions of –OH and –CH (CH/CH2/CH3) and the first overtone region of –NH from protein in the protein compound (Wang et al., 2013; Lv et al., 2016); the wavelength at 1254, 1276, 1314, and 1467 nm are closely related to the starch compounds and reflect the –CH second overtone and combination (Workman and Weyer, 2007; Ma et al., 2017). Additionally, the wavelengths at 486, 546, and 562 nm in the visible light range represent potential color differences in millet samples due to environmental factors in different production areas.
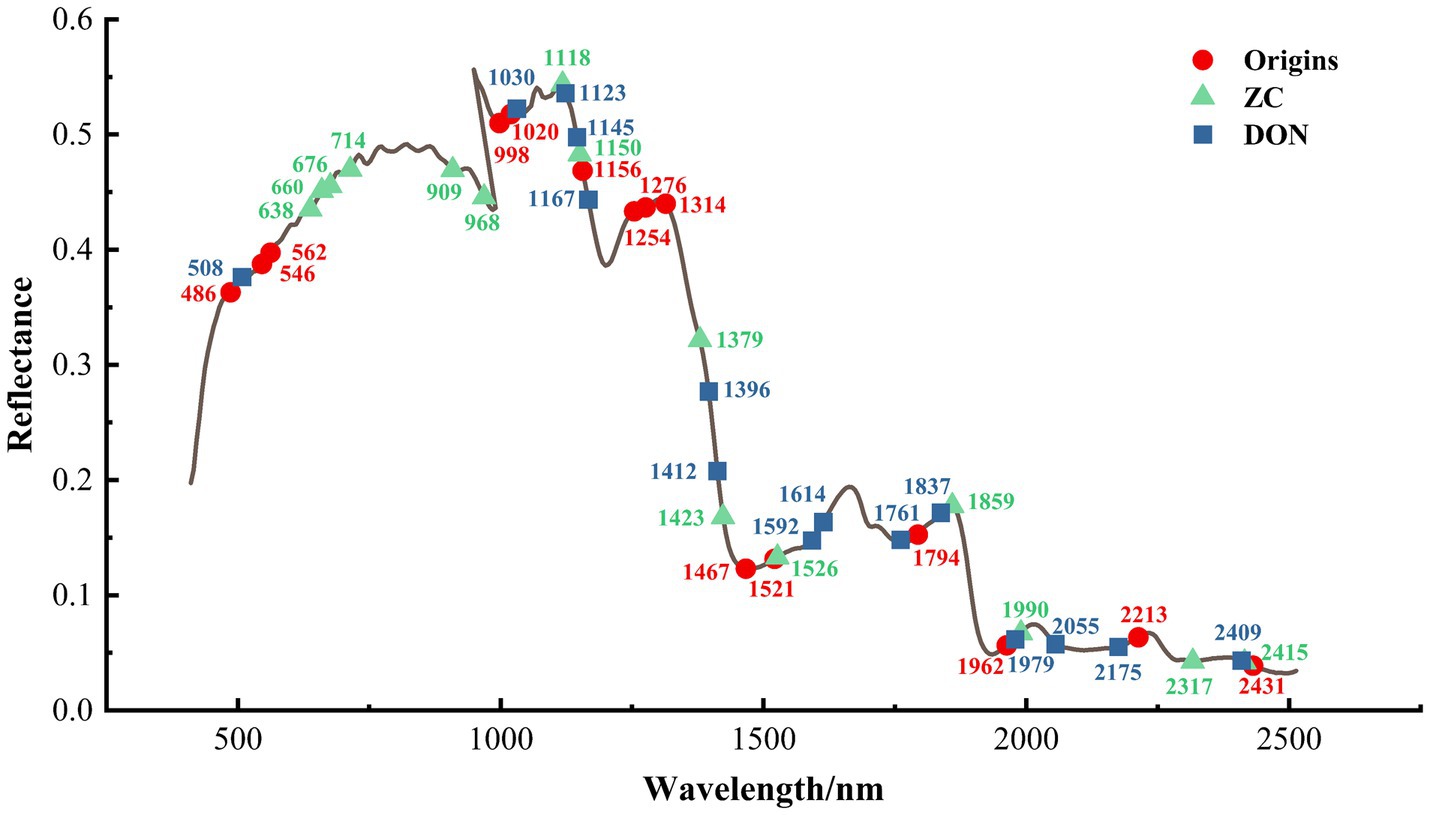
Figure 3. Feature wavelengths obtained by the AM module. In the context, “Origin,” “ZC,” and “DON” represent the feature wavelengths for millet origin discrimination, ZC prediction, and DON content prediction, respectively, where each group was labeled with the 15 most important feature wavelengths.
In the prediction of DON and ZC content, the main feature wavelengths indicate that the contamination by fungi of the Fusarium genus mainly leads to changes in the organic properties of millet and potential discoloration. Regarding DON, wavelengths in the visible light range of 486, 546, and 562 nm represent potential color differences in millet caused by various environmental factors in different production areas. Meanwhile, the wavelengths at 1396 and 1412 nm are associated with the moisture content of millet (Femenias et al., 2021). The wavelengths at 1123, 1145, and 1167 nm correspond to the second overtone regions of –OH and –CH (CH/CH2/CH3) in the protein compound (Wang et al., 2013; Lv et al., 2016); additionally, the wavelengths at 1592 and 1614 nm bands are related to the –NH stretch first overtone and –CH first overtone in the protein compound (Eldin and Akyar, 2011).
As for the ZC group, the wavelengths at 638, 660, 676, and 714 nm represent potential color differences in millet caused by environmental factors in different production areas. The wavelengths at 909 and 968 nm are, respectively, related to the third overtone region of –CH (CH/CH2/CH3) and the third overtone region of –OH from oil nutrient (Weinstock et al., 2006; Balbino et al., 2022). The effective spectra of 1118 nm and 1150 nm correspond to the second overtone regions of –OH and –CH (CH/CH2/CH3) in the protein compound (Wang et al., 2013; Lv et al., 2016); additionally, the wavelength at 1423 nm is associated to the moisture content of millet (Femenias et al., 2021).
3.2 Results of geographical origin discrimination of XM
3.2.1 Results of geographical origin discrimination based on full wavelengths
Based on the full spectrum of HSI, the results of millet origin discrimination using PLSDA, SVM, RF, and LSTM prediction models combined with three preprocessing methods (SNV, MSC, and WT) are presented in Table 2. The results showed that compared to the group of using original data without denoising processing (ORI), the three preprocessing groups exhibited higher origin prediction accuracy, reflecting the effectiveness of denoising methods. Among them, the WT preprocessing group obtained the best results, with the accuracy of prediction exceeding 85% for all models. The WT-LSTM model achieved the highest prediction performance for millet origin discrimination, with the highest accuracy reaching 94.4% on the prediction set.
3.2.2 Improved effect of geographical origin discrimination based on the selected wavelengths
After using the selected feature wavelengths, PLSDA, SVM, RF, and LSTM models showed higher overall accuracy on both the training and prediction sets (Table 3), with their prediction accuracy all exceeding 85%, demonstrating the effectiveness of the AM algorithm in selecting feature wavelengths. Overall, the WT data denoising preprocessing method showed the best results, with improvements in prediction accuracy across all models. In terms of regression models, the LSTM model combined with different data preprocessing methods achieved a prediction accuracy of over 98% on the prediction set. Overall, the WT-LSTM model achieved the best performance, with training and testing accuracy of 99.5 and 99.4%, respectively.
3.3 Results of DON and ZC content prediction
3.3.1 Results of DON and ZC content prediction based on full wavelengths
The results of ZC and DON content prediction based on the full wavelength are presented in Table 4. In the content prediction of both chemical indicators, after WT preprocessing, all models exhibited higher R values, lower MAE and RMSE values, and higher RPD values (all above 2.50) compared to other preprocessing groups, indicating good model performance and the outstanding denoising effect of the WT method. From the perspective of content regression models, the LSTM model obtained higher R values than the traditional PLSR, SVM, and RF models, reflecting a better linear relationship; meanwhile, the LSTM model obtained significantly lower MAE and RMSE values than other models, indicating that the LSTM model had smaller prediction errors. Additionally, the RPD values of the LSTM model are generally the highest, demonstrating the excellent predictive performance of the model. Overall, the WT-LSTM model is the best model for ZC and DON content prediction, with the highest R values (all above 0.90), lowest MAE and RMSE values, and RPD values all above 3.30, demonstrating the excellent predictive performance of these models (Table 4).
3.3.2 Improved effect of ZC and DON content prediction based on the selected wavelengths
As shown in the above prediction results using the full spectrum, the WT preprocessing method exhibited the best denoising effect. Meanwhile, redundant spectral data can lead to issues such as high model complexity, long data processing times, and poor predictive performance. However, these issues can be addressed by selecting effective wavelengths. In this section, feature wavelengths are extracted using the AM algorithm, and four regression models (PLSR, SVM, RF, and LSTM) were combined with the WT preprocessing method to predict the content of two chemical indicators. The results are presented in Table 5. In the content prediction of the two chemical indicators, the four models using selected wavelengths all obtained better results than those using the full spectrum group (higher R and RPD values, lower RMSE values), demonstrating the effectiveness of feature wavelength selection. Moreover, compared to the ORI group, the WT data denoising group demonstrated better prediction results (higher R and RPD values, lower RMSE values), highlighting the outstanding denoising effect of the WT method.
For the content prediction of the two chemical indicators, the WT-LSTM model demonstrated the best performance. Its R values both exceeded 0.95, and the RPD values were higher than 3.50, indicating outstanding predictive capabilities of the model (Table 5). In the prediction of ZC content, compared to the full wavelength group (WT-LSTM), the R value of the selected full wavelength group was increased by 5.52%, the MAE and RMSE values were significantly decreased by about 47.97 and 41.78%, respectively, and the RPD value was increased by 5.9%. In the prediction of DON content, compared to the full wavelength group (WT-LSTM), the R value of the selected full wavelength group was increased by 0.41%, while the MAE and RMSE values were, respectively, decreased by approximately 6.93 and 4.62%, and the RPD value is increased by 1.1%, remaining at a similar level (Table 5).
4 Discussion
The quality attributes of millet determine its commercial value, and there is high variability in the quality and price of millet on the market. Due to differences in growing regions and environmental factors, millet from different origins has significant differences in appearance and nutritional content, leading to notable price disparities (Wang et al., 2022a). The frequent occurrence of counterfeit geographical indications undermines market fairness and brand value. Meanwhile, during storage or processing, grains such as millet are susceptible to mycotoxin contamination (Zhao et al., 2020; Femenias et al., 2021; Teixido-Orries et al., 2023), posing serious issues of food safety and quality (Bai et al., 2024). Therefore, developing a rapid and accurate method to predict the geographical origin and fungal contamination of millet is crucial for preventing fraud and protecting consumer rights. HSI emerges as a promising non-destructive detection technique, and it is widely used in the assessment of agricultural product quality (Wang et al., 2021).
Currently, HSI research has achieved good results in predicting the content of low-concentration compounds, such as toxins (DON and ZC) in maize and wheat (Femenias et al., 2021; Borras-Vallverdu et al., 2024), and ginsenosides in Panax ginseng (Wang et al., 2024a; Wang et al., 2024b), overcoming the challenges of trace compound prediction. In this study, HSI combined with a deep learning model (WT-ALSTM) also obtained satisfactory results in millet origin classification, with training and testing accuracy of 99.5 and 99.4%, respectively. In the prediction of low-concentration compounds, such as ZC and DON, the WT-ALSTM model’s RPD values exceeded 3.0, and the R values were greater than 0.9, demonstrating the excellent predictive performance and the immense potential of HSI technology for low-concentration compound prediction. Furthermore, the WT-ALSTM model made satisfactory regression predictions, outperforming traditional and individual deep learning models. The AM model’s characteristic bands provided reasonable explanatory and satisfactory prediction outcomes. Generally, the combination of the WT-ALSTM model with HSI technology provides a promising approach for developing portable equipment for rapid and effective quality prediction of millet in the future.
Hyperspectral data involve diverse noise sources, which may originate from instruments or samples. Preprocessing methods are commonly used to improve the prediction performance of models. The WT denoising method used in this study is typically sensitive to various sources of noise such as environmental conditions, instrument errors, and sample variations (He et al., 2018; Wang et al., 2022c). Existing studies, including predictions of total polysaccharides and total flavonoid content in Chrysanthemum (He et al., 2018), and assessments of total alkylamide content (TALC) and volatile oil content (VOC) in Sichuan pepper (Wang et al., 2022c), all validated the applicability of the WT denoising method to HSI data. Similarly, in this study, the AM module was used as a feature wavelength selection method, which significantly reduced the computational burden caused by redundant data, contributing to higher model performance. Consistent with this study, the combination of the AM module with deep learning models in the field of HSI research has also yielded some encouraging results. For example, the CLSTM model successfully used the AM module to screen feature bands for predicting starch and protein content in Coix seed (Wang et al., 2023); the fusion of the AM module and the SCNN model can successfully predict wheat’s susceptibility to herbicide stress (Chu et al., 2022); additionally, the AM module was combined with CNN models to predict single particle oil content in maize seeds (Zhang et al., 2022).
The deep learning model LSTM used in this study demonstrated higher prediction performance than traditional machine learning models. The LSTM module, integrated with multi-layer neural networks, shows a strong capability to handle complex and nonlinear spectral data (Fan et al., 2022). Furthermore, compared to traditional machine learning models, the LSTM model enhances compatibility with spectral time-series sequences through gated recurrent units and improves generalization and stability through dropout strategies to address overfitting issues (Wang et al., 2023). Similar studies in the literature, such as the prediction of amino acid content in beef (Dong et al., 2024) and corn variety identification (Wang et al., 2018), have confirmed the outstanding prediction performance of LSTM models and the advantages of deep learning models in terms of self-inference, avoidance of subjective parameter tuning, and more objective and reliable model output, indicating that they can be successfully used in food research in the future (Wang et al., 2023).
In future research, considering the great challenges posed by external environmental factors, simultaneously normalizing and denoising both spectral and chemical content data can suppress the impact of individual differences. Meanwhile, collecting more representative samples from various geographical origins can further improve the applicability and reliability of prediction models. Compared to traditional spectral techniques, HSI has the advantage of acquiring both spectral and image information from samples. With the prominent advantages of deep learning techniques in processing image information, it is necessary to integrate image and spectral information to further develop prediction models for millet samples, thereby broadening the application scope of HSI technology. Moreover, based on the effective wavelengths selected by the AM module in HSI, further efforts should be made to develop specialized, portable, and miniaturized hyperspectral systems to meet the demand for on-site rapid testing in future markets.
Data availability statement
The original contributions presented in the study are included in the article/Supplementary material, further inquiries can be directed to the corresponding authors.
Author contributions
SN: Writing – original draft. WG: Writing – original draft. SL: Writing – review & editing. ML: Data curation, Formal analysis, Writing – review & editing. TL: Data curation, Formal analysis, Writing – review & editing. JR: Data curation, Formal analysis, Writing – review & editing. SR: Data curation, Writing – review & editing. JW: Writing – review & editing.
Funding
The author(s) declare that financial support was received for the research, authorship, and/or publication of this article. This research was supported by the Hebei Province High-level Talent Funding Project (Grant no. C20231003); Science and Technology Research Project of Hebei Universities (Grant no. QN2024068); Cangzhou Normal University Research and Innovation Team (cxtdl2301); Hebei Natural Science Foundation (C2022110007); Hebei Agricultural University Talent Introduction Research Special Project (YJ2022009); Research project on basic scientific research business expenses of provincial universities in Hebei Province (KY2023023).
Acknowledgments
We thank Youyou Wang (State Key Laboratory for Quality Ensurance and Sustainable Use of Dao-di Herbs, National Resource Center for Chinese Materia Medica, China Academy of Chinese Medical Sciences) for his suggestions on writing this article and thank all the reviewers who participated in the review, as well as MJEditor (www.mjeditor.com) for providing English editing services during the preparation of this manuscript.
Conflict of interest
The authors declare that the research was conducted in the absence of any commercial or financial relationships that could be construed as a potential conflict of interest.
Publisher’s note
All claims expressed in this article are solely those of the authors and do not necessarily represent those of their affiliated organizations, or those of the publisher, the editors and the reviewers. Any product that may be evaluated in this article, or claim that may be made by its manufacturer, is not guaranteed or endorsed by the publisher.
Supplementary material
The Supplementary material for this article can be found online at: https://www.frontiersin.org/articles/10.3389/fsufs.2024.1454020/full#supplementary-material
Abbreviations
AM, attention mechanism; R, coefficient of determination; CNN, convolutional neural networks; DON, deoxynivalenol; ZC, ergosterol; HSI, hyperspectral imaging; HPLC-MS, high-performance liquid chromatography-mass spectrometry tandem; LSTM, long short-term memory; MAE, mean absolute error; MSC, multiplicative scatter correction; ORI, original data without denoising processing; PGI, protected geographical indication; PLSDA, partial least squares discriminant analysis; PLSR, partial least squares regression; RF, random forest; RNNs, recurrent neural networks; RMSE, root mean square error; RPD, residual predictive deviation; SNV, standard normal variate; SVM, support vector machine; WT, wavelet transformation; WT-ALSTM, wavelet transformation-attention mechanism long short-term memory.
References
An, D., Zhang, L., Liu, Z., Liu, J., and Wei, Y. (2023). Advances in infrared spectroscopy and hyperspectral imaging combined with artificial intelligence for the detection of cereals quality. Crit. Rev. Food Sci. Nutr. 63, 9766–9796. doi: 10.1080/10408398.2022.2066062
Antonios, D., Guitton, V., Darrozes, S., Pallardy, M., and Azouri, H. (2010). Monitoring the levels of deoxynivalenol (DON) in cereals in Lebanon and validation of an HPLC/UV detection for the determination of DON in crushed wheat (bulgur). Food Addit. Contam. Part B Surveill. 3, 45–51. doi: 10.1080/19440040903514507
Bai, R., Zhou, J., Wang, S., Zhang, Y., Nan, T., Yang, B., et al. (2024). Identification and classification of Coix seed storage years based on hyperspectral imaging technology combined with deep learning. Food Secur. 13:30498. doi: 10.3390/foods13030498
Balbino, S., Vincek, D., Trtanj, I., Egredija, D., Gajdos-Kljusuric, J., Kraljic, K., et al. (2022). Assessment of pumpkin seed oil adulteration supported by multivariate analysis: comparison of GC-MS, colourimetry and NIR spectroscopy data. Food Secur. 11:835. doi: 10.3390/foods11060835
Balli, D., Bellumori, M., Masoni, A., Moretta, M., Palchetti, E., Bertaccini, B., et al. (2023). Proso millet (Panicum miliaceum L.) as alternative source of starch and phenolic compounds: a study on twenty-five worldwide accessions. Molecules 28:6339. doi: 10.3390/molecules28176339
Borras-Vallverdu, B., Marin, S., Sanchis, V., Gatius, F., and Ramos, A. J. (2024). NIR-HSI as a tool to predict deoxynivalenol and fumonisins in maize kernels: a step forward in preventing mycotoxin contamination. J. Sci. Food Agric. 104, 5495–5503. doi: 10.1002/jsfa.13388
Bu, Y., Jiang, X., Tian, J., Hu, X., Han, L., Huang, D., et al. (2023). Rapid nondestructive detecting of sorghum varieties based on hyperspectral imaging and convolutional neural network. J. Sci. Food Agric. 103, 3970–3983. doi: 10.1002/jsfa.12344
Chu, H., Zhang, C., Wang, M., Gouda, M., Wei, X., He, Y., et al. (2022). Hyperspectral imaging with shallow convolutional neural networks (SCNN) predicts the early herbicide stress in wheat cultivars. J. Hazard. Mater. 421:126706. doi: 10.1016/j.jhazmat.2021.126706
Dasa, F., and Nguyen, B. (2020). Relation among proximate compositions, rheological properties and injera making quality of millet varieties. Adv. Crop. Sci. Technol. 8:453.
Dong, F., Bi, Y., Hao, J., Liu, S., Yi, W., Yu, W., et al. (2024). A new comprehensive quantitative index for the assessment of essential amino acid quality in beef using Vis-NIR hyperspectral imaging combined with LSTM. Food Chem. 440:138040. doi: 10.1016/j.foodchem.2023.138040
Dowell, F., Ram, M., and Seitz, L. (1999). Predicting scab, vomitoxin, and ergosterol in single wheat kernels using near-infrared spectroscopy. Cereal Chem. 76, 573–576. doi: 10.1094/CCHEM.1999.76.4.573
Eldin, A., and Akyar, I. (2011). Near infrared spectroscopy. Wide spectra of quality control. 237–248
Fan, J., Bi, S., Xu, R., Wang, L., and Zhang, L. (2022). Hybrid lightweight deep-learning model for sensor-fusion basketball shooting-posture recognition. Measurement 189:110595. doi: 10.1016/j.measurement.2021.110595
Femenias, A., Gatius, F., Ramos, A., Sanchis, V., and Marin, S. (2021). Near-infrared hyperspectral imaging for deoxynivalenol and ergosterol estimation in wheat samples. Food Chem. 341:128206. doi: 10.1016/j.foodchem.2020.128206
Galvao, R., Araujo, M., Jose, G., Pontes, M., Silva, E., and Saldanha, T. (2005). A method for calibration and validation subset partitioning. Talanta 67, 736–740. doi: 10.1016/j.talanta.2005.03.025
General Administration of Quality Supervision (2011), inspection and quarantine of the People’s Republic of China, standardization administration. Inspection of grain and oils-determination of ergosterol in grain-Normal phase high performance liquid chromatography: GB/T 25221-2010, 12. Beijing: Standards Press of China, 1–8.
General Administration of Quality Supervision (2017), inspection and quarantine of the People’s Republic of China, standardization administration. Determination of deoxynivalenol and its acetylated derivatives in national food safety standards: GB5009.111-2016 [S]. Beijing: Standards Press of China, 1–21.
He, J., Chen, L., Chu, B., and Zhang, C. (2018). Determination of total polysaccharides and total flavonoids in Chrysanthemum morifolium using near-infrared hyperspectral imaging and multivariate analysis. Molecules 23:2395. doi: 10.3390/molecules23092395
He, X., Guo, D., Lan, S., Zhang, H., Li, W., Ding, J., et al. (2007). Feasibility of ergosterol as an index to estimate grain safety degree. Grain Storage. 6, 22–26.
Jia, P., Shang, T., Zhang, J., and Sun, Y. (2021). Inversion of soil pH during the dry and wet seasons in the Yinbei region of Ningxia, China, based on multi-source remote sensing data. Geoderma Reg. 25:e00399. doi: 10.1016/j.geodrs.2021.e00399
Li, X., Yi, X., Liu, Z., Liu, H., Chen, T., Niu, G., et al. (2021). Application of novel hybrid deep leaning model for cleaner production in a paper industrial wastewater treatment system. J. Clean. Prod. 294:126343. doi: 10.1016/j.jclepro.2021.126343
Liang, K., Huang, J., He, R., Wang, Q., Chai, Y., and Shen, M. (2020). Comparison of Vis-NIR and SWIR hyperspectral imaging for the non-destructive detection of DON levels in Fusarium head blight wheat kernels and wheat flour. Infrared Phys. Technol. 106:103281. doi: 10.1016/j.infrared.2020.103281
Liu, G., Zhou, X., Li, Q., Shi, Y., Guo, G., Zhao, L., et al. (2020). Spatial distribution prediction of soil as in a large-scale arsenic slag contaminated site based on an integrated model and multi-source environmental data. Environ. Pollut. 267:115631. doi: 10.1016/j.envpol.2020.115631
Lu, W., Jiang, Q., Shi, H., Niu, Y., Gao, B., and Yu, L. (2014). Partial least-squares-discriminant analysis differentiating Chinese wolfberries by UPLC-MS and flow injection mass spectrometric (FIMS) fingerprints. J. Agric. Food Chem. 62, 9073–9080. doi: 10.1021/jf502156n
Lv, C., Jiang, X., Zhang, Y., Zhang, X., and Mao, W. (2016). Variable selection based near infrared spectroscopic quantitative analysis on wheat crude protein content. Trans. Chin. Soc. Agric. Mach. 47, 340–346. doi: 10.6041/j.issn.1000-1298.2016.S0.052
Ma, H., Wang, J., Chen, Y., Cheng, J., and Lai, Z. (2017). Rapid authentication of starch adulterations in ultrafine granular powder of Shanyao by near-infrared spectroscopy coupled with chemometric methods. Food Chem. 215, 108–115. doi: 10.1016/j.foodchem.2016.07.156
Mahajan, P., Bera, M., Panesar, P., and Chauhan, A. (2021). Millet starch: a review. Int. J. Biol. Macromol. 180, 61–79. doi: 10.1016/j.ijbiomac.2021.03.063
Mou, L., Zhou, C., Zhao, P., Nakisa, B., Rastgoo, M., Jain, R., et al. (2021). Driver stress detection via multimodal fusion using attention-based CNN-LSTM. Expert Syst. Appl. 173:114693. doi: 10.1016/j.eswa.2021.114693
Onipe, O., and Ramashia, S. (2022). Finger millet seed coat-a functional nutrient-rich cereal by-product. Molecules 27:227837. doi: 10.3390/molecules27227837
Rocha, D., Oliveira, M., Furlong, E., Junges, A., Paroul, N., Valduga, E., et al. (2017). Evaluation of the TLC quantification method and occurrence of deoxynivalenol in wheat flour of southern Brazil. Food. Additives Contamin. 34, 2220–2229. doi: 10.1080/19440049.2017.1364872
Safdar, L. B., Dugina, K., Saeidan, A., Yoshicawa, G. V., Caporaso, N., Gapare, B., et al. (2023). Reviving grain quality in wheat through non-destructive phenotyping techniques like hyperspectral imaging. Food. Energy. Secur. 12:e498. doi: 10.1002/fes3.498
Saleem, S., Mushtaq, N., Shah, W., Rasool, A., Hakeem, K., Seth, C., et al. (2023). Millets as smart future food with essential phytonutrients for promoting health. J. Food. Compost. Anal. 124:105669. doi: 10.1016/j.jfca.2023.105669
Shi, P., Zhao, Y., Qin, F., Liu, K., and Wang, H. (2023). Understanding the multi-scale structure and physicochemical properties of millet starch with varied amylose content. Food Chem. 410:135422. doi: 10.1016/j.foodchem.2023.135422
Teixido-Orries, I., Molino, F., Femenias, A., Ramos, A., and Marin, S. (2023). Quantification and classification of deoxynivalenol-contaminated oat samples by near-infrared hyperspectral imaging. Food Chem. 417:135924. doi: 10.1016/j.foodchem.2023.135924
Wang, Y., Kang, L., Zhao, Y., Xiong, F., Yuan, Y., Nie, J., et al. (2022b). Stable isotope and multi-element profiling of Cassiae semen tea combined with chemometrics for geographical discrimination. J. Food. Compost. Anal. 107:104359. doi: 10.1016/j.jfca.2021.104359
Wang, X., Liao, W., An, D., and Wei, Y. (2018). Maize haploid identification via LSTM-CNN and hyperspectral imaging technology. arXiv doi: 10.48550/arXiv.1805.09105
Wang, B., Sun, J., Xia, L., Liu, J., Wang, Z., Li, P., et al. (2021). The applications of hyperspectral imaging technology for agricultural products quality analysis: a review. Food Rev. Int. 39, 1043–1062. doi: 10.1080/87559129.2021.1929297
Wang, Y., Wang, S., Bai, R., Li, X., Yuan, Y., Nan, T., et al. (2024a). Prediction performance and reliability evaluation of three ginsenosides in Panax ginseng using hyperspectral imaging combined with a novel ensemble chemometric model. Food Chem. 430:136917. doi: 10.1016/j.foodchem.2023.136917
Wang, L., Wang, Q., Liu, H., Liu, L., and Du, Y. (2013). Determining the contents of protein and amino acids in peanuts using near-infrared reflectance spectroscopy. J. Sci. Food Agric. 93, 118–124. doi: 10.1002/jsfa.5738
Wang, F., Wang, C., and Song, S. (2022a). Origin identification of foxtail millet (Setaria italica) by using green spectral imaging coupled with chemometrics. Infrared Phys. Technol. 123:104179. doi: 10.1016/j.infrared.2022.104179
Wang, Y., Wang, S., Yuan, Y., Li, X., Bai, R., Wan, X., et al. (2024b). Fast prediction of diverse rare ginsenoside contents in Panax ginseng through hyperspectral imaging assisted with the temporal convolutional network-attention mechanism (TCNA) deep learning. Food Control 162:110455. doi: 10.1016/j.foodcont.2024.110455
Wang, Y., Xiong, F., Zhang, Y., Wang, S., Yuan, Y., Lu, C., et al. (2023). Application of hyperspectral imaging assisted with integrated deep learning approaches in identifying geographical origins and predicting nutrient contents of Coix seeds. Food Chem. 404:134503. doi: 10.1016/j.foodchem.2022.134503
Wang, Y., Yang, J., Yu, S., Fu, H., He, S., Yang, B., et al. (2022c). Prediction of chemical indicators for quality of Zanthoxylum spices from multi-regions using hyperspectral imaging combined with chemometrics. Front. Sustain. Food. Syst. 6:1036892. doi: 10.3389/fsufs.2022.1036892
Wang, Y., Zhang, Y., Yuan, Y., Zhao, Y., Nie, J., Nan, T., et al. (2022d). Nutrient content prediction and geographical origin identification of red raspberry fruits by combining hyperspectral imaging with chemometrics. Front. Nutr. 9:980095. doi: 10.3389/fnut.2022.980095
Weinstock, B., Janni, J., Hagen, L., and Wright, S. (2006). Prediction of oil and oleic acid concentrations in individual corn (Zea mays L.) kernels using near-infrared reflectance hyperspectral imaging and multivariate analysis. Appl. Spectrosc. 60, 9–16. doi: 10.1366/000370206775382631
Workman, J. R., and Weyer, L. (2007). Practical guide to interpretive near-infrared spectroscopy, vol. 344. Boca Raton: CRC Press.
Wu, Y., Peng, S., Xie, Q., Han, Q., Zhang, G., and Sun, H. (2019). An improved weighted multiplicative scatter correction algorithm with the use of variable selection: application to near-infrared spectra. Chemometr. Intell. Lab. Syst. 185, 114–121. doi: 10.1016/j.chemolab.2019.01.005
Wu, L., and Qu, L. (2018). A review on the resource and processing of the millet. Food Res Dev 39, 191–196. doi: 10.5555/20183280206
Yan, Y., Abdulla, R., Ma, Q., and Aisa, H. (2023). Comprehensive identification of chemical fingerprint and screening of potential quality markers of Aloe vera (L.) Burm. f. from different geographical origins via ultra-high-performance liquid chromatography hyphenated with quadrupole-orbitrap-high-resolution mass spectrometry combined with chemometrics. J. Chromatogr. Sci. 61, 312–321. doi: 10.1093/chromsci/bmad009
Yang, L., Li, R., Cui, Y., Qin, X., and Li, Z. (2021). Comparison of nutritional compositions of foxtail millet from the different cultivation regions by UPLC-Q-Orbitrap HRMS based metabolomics approach. J. Food Biochem. 45:e13940. doi: 10.1111/jfbc.13940
Yang, X., Wan, Z., Perry, L., Lu, H., Wang, Q., Zhao, C., et al. (2012). Early millet use in northern China. Proc. Natl. Acad. Sci. USA 109, 3726–3730. doi: 10.1073/pnas.1115430109
Yang, Y., Zhang, H., Wang, R., Deng, L., Qin, L., Chen, E., et al. (2019). Determination of yellow pigment content in foxtail millet. J. Chin. Cereal. Oil. Assoc. 34, 121–125.
Yao, Y., and Long, M. (2020). The biological detoxification of deoxynivalenol: a review. Food Chem. Toxicol. 145:111649. doi: 10.1016/j.fct.2020.111649
Yu, X., Wang, J., Wen, S., Yang, J., and Zhang, F. (2019). A deep learning based feature extraction method on hyperspectral images for nondestructive prediction of TVB-N content in Pacific white shrimp (Litopenaeus vannamei). Biosyst. Eng. 178, 244–255. doi: 10.1016/j.biosystemseng.2018.11.018
Zhang, L., An, D., Wei, Y., Liu, J., and Wu, J. (2022). Prediction of oil content in single maize kernel based on hyperspectral imaging and attention convolution neural network. Food Chem. 395:133563. doi: 10.1016/j.foodchem.2022.133563
Zhang, C., Wu, W., Zhou, L., Cheng, H., Ye, X., and He, Y. (2020). Developing deep learning based regression approaches for determination of chemical compositions in dry black goji berries (Lycium ruthenicum Murr.) using near-infrared hyperspectral imaging. Food Chem. 319:126536. doi: 10.1016/j.foodchem.2020.126536
Keywords: millet, hyperspectral imaging, deep learning model, geographical origin, fungal contamination
Citation: Nie S, Gao W, Liu S, Li M, Li T, Ren J, Ren S and Wang J (2024) Hyperspectral imaging combined with deep learning models for the prediction of geographical origin and fungal contamination in millet. Front. Sustain. Food Syst. 8:1454020. doi: 10.3389/fsufs.2024.1454020
Edited by:
Yiming Zhang, Zhejiang Agriculture and Forestry University, ChinaReviewed by:
Gengjun Chen, Kansas State University, United StatesKristian Pastor, University of Novi Sad, Serbia
Copyright © 2024 Nie, Gao, Liu, Li, Li, Ren, Ren and Wang. This is an open-access article distributed under the terms of the Creative Commons Attribution License (CC BY). The use, distribution or reproduction in other forums is permitted, provided the original author(s) and the copyright owner(s) are credited and that the original publication in this journal is cited, in accordance with accepted academic practice. No use, distribution or reproduction is permitted which does not comply with these terms.
*Correspondence: Shasha Liu, bGl1c2hhc2hhOTgxQDEyNi5jb20=; Jian Wang, d2FuZ2ppYW4zNzkwQDEyNi5jb20=
†These authors have contributed equally to this work and share first authorship