- 1Liaoning Technical University, Fuxin, China
- 2The University of Sydney, Camperdown, NSW, Australia
Introduction: Agriculture faces significant challenges in ensuring global food security while minimizing resource costs and environmental impacts. The development of digital infrastructure offers transformative potential for agricultural systems and aligns with the United Nations Sustainable Development Goals. This study examines the role of digital infrastructure in enhancing grain production capacity in China, a key player in the global food system.
Methods: We analyzed data from 277 prefecture-level cities in China from 2011 to 2021. A double machine learning model was employed to empirically assess the impact of digital infrastructure on grain production capacity, allowing for robust insights into causal relationships.
Results: Results reveal that digital infrastructure significantly enhances grain production. Mechanism analysis results indicate that digital infrastructure construction drives agricultural technological advancements and farmland scale, contributing to increased production capacity. Heterogeneity analysis results show that the impact of digital infrastructure construction is significant in major grain-producing regions and the central-eastern regions, while its effects are relatively limited in grain production-consumption balanced regions, main grain consumption regions, and the western regions.
Discussion: The results underscore the importance of strengthening digital infrastructure in rural areas to improve grain production capacity. Tailored policy implications are suggested to enhance sustainable food production and contribute to global food security, particularly in regions with varying agricultural dynamics.
1 Introduction
Food security is essential for national stability and a pressing concern for the global community. With the world population expected to surpass 9.7 billion by 2050, the challenge of increasing food production by 70% to meet future demand becomes increasingly urgent (FAO, 2022). However, in many developing countries like China, the agricultural systems are struggling to keep pace, hampered by slowing productivity growth, the intensifying effects of climate change (Zhao et al., 2017), pressuring water and soil resources (Wang et al., 2023), rising production costs (Tian et al., 2020; Giller et al., 2021), and geopolitical uncertainties (Sun et al., 2021) that disrupt supply chains (Warsame et al., 2022). In response, the United Nations’ Sustainable Development Goals (SDGs) offer a framework to foster innovation and resilience in agriculture, ensuring the long-term sustainability of global food systems. Addressing these challenges requires a transformative approach, integrating technological innovation and sustainable practices to achieve the SDGs and ensure future food security.
Various strategies and technological innovations have been developed globally in response to these challenges. Water-saving technologies, such as drip irrigation, desalination, and wastewater treatment, have been essential in reducing water shortages (Naqvi et al., 2024; Morchid et al., 2024). Agroecological approaches like crop rotation, agroforestry, and agricultural waste recycling have also been adopted to increase productivity while promoting sustainability (Jose et al., 2024; Maroušek et al., 2023). Modern agriculture has greatly benefited from technological advancements, especially machine learning models. Early yield prediction made possible by these models helps farmers optimize crop management and minimize resource waste (Lutz and Coradi, 2022; Kliestik et al., 2023; Leukel et al., 2023). Furthermore, precision agriculture enhances economic results and sustainability by enabling precise management of water, fertilizers, and pesticides through the use of big data and the Internet of Things (Lima et al., 2020; Storm et al., 2024; Son et al., 2024). New digital twin technologies simulate agricultural systems in real time, which further improves resource efficiency (Kliestik et al., 2024). These technological advances, driven by digital infrastructure, are revolutionizing global agriculture, fostering more sustainable and resilient food systems, and contributing significantly to the achievement of the UN Sustainable Development Goals (SDGs) (Shamdasani, 2021).
China has a vital role in the world’s food supply as one of the biggest producers of grains worldwide. Even with notable progress—the country’s grain output increased significantly from 304.8 million metric tons in 1978 to 695.4 million metric tons in 2023 (National Bureau of Statistics of China, 2023)—it still faces formidable obstacles in increasing its capacity for producing grains. To address these challenges, China has focused on several key measures, including protecting cultivated land (Cao et al., 2023), strengthening agricultural insurance (Xie et al., 2024), deepening agricultural subsidy policies (Zhang et al., 2021; Yang et al., 2023), promoting technological innovation (Basso et al., 2021), and optimizing production models (Guo et al., 2021). Among these strategies, the strengthening of digital infrastructure has emerged as the most critical component (Ding et al., 2024).
Both in China and internationally, building digital infrastructure forms a crucial foundation for modern agricultural practices. Existing research shows that robust digital infrastructure improves agricultural productivity and eco-efficiency by enabling more efficient information flow and fostering the widespread adoption of technological innovations (Shamdasani, 2021; Ren et al., 2024). Over the past decade, substantial improvements in agricultural infrastructure—driven by digital innovation and supported by advanced communication networks—have transformed grain production worldwide. The integration of digital technologies into agricultural industries has proven instrumental in enhancing agricultural supply chains, boosting productivity, and promoting sustainability (Massruhá et al., 2023; Dolgui and Ivanov, 2022; Verdecchia et al., 2022). In particular, digital infrastructure has extended agricultural services, improved food security, and increased farmers’ incomes through the adoption of technologies such as precision agriculture and automated machinery (Ren et al., 2024; Hao et al., 2024). By digitalizing agricultural practices and introducing new technologies, rural economies have strengthened, resulting in higher production efficiency and improved quality of life for farming communities (Chen et al., 2022; Wu et al., 2021). This transformation has been particularly evident in China, where recent investments in digital infrastructure have significantly improved agricultural eco-efficiency and overall productivity (Ren et al., 2024).
Notwithstanding these developments, scholarly research on the direct effects of digital infrastructure development on food production is still lacking, underscoring the need for a more thorough analysis of how new technologies can improve food security. Although the amount of research on the subject is increasing, more investigation is required to completely grasp and utilize the potential of digital infrastructure in agriculture. A thorough search of Google Scholar was done using terms like “digital transformation,” “ICT infrastructure,” “digital technology,” and “grain production,” with a focus on the recent five years, to determine the study’s uniqueness and applicability. The search yielded approximately 30 articles on digital transformation in agriculture, 18 on ICT infrastructure, and 25 on digital technology’s role in grain yield. While there is substantial research on agricultural digitalization, few studies focus specifically on the impact of digital infrastructure on grain production capacity, revealing a clear gap in empirical research on this subject.
This paper aims to fill the gap by exploring whether digital infrastructure construction can improve grain production capacity and identify the specific pathways through which this occurs. Using data from 277 prefecture-level cities in China from 2011 to 2021, the study employs a double machine learning model to examine the impact of digital infrastructure on grain production. It focuses on promoting agricultural technology progress and expanding cultivated land scale operations, thereby broadening the research scope on digital infrastructure and grain production capacity.
The main contributions of this paper are as follows:
(1) Comprehensive examination of digital infrastructure and grain production: It extensively investigates the impact and mechanism of digital infrastructure construction on grain production capacity. While previous research has predominantly focused on the influence of digital and technology on agricultural development, this study hones in on the specific category of broadband infrastructure among digital infrastructures. It broadens the scope of research on technological progress in grain production and lays the groundwork for understanding the implementation effects of the “Broadband China” pilot policy on grain production. The research also contributes to the realization of the United Nations Sustainable Development Goals (SDGs), particularly those related to food security, innovation, and infrastructure.
(2) Mechanistic insights into agricultural technological advancements: The study investigates how digital infrastructure promotes agricultural technological progress and expands the scale of agricultural operations, thereby contributing to improved grain security. It provides insights into how regional grain producers utilize digital resources to maximize production, with varying impacts across different regions.
(3) Innovative research methodology: Methodologically, the study employs a doubly robust machine learning model to assess the policy effects of the “Broadband China” pilot on grain production capacity. Leveraging the algorithm’s advantages in high-dimensional, non-parametric forecasting, this approach mitigates estimation bias and model specification bias seen in traditional econometric models, thereby enhancing the stability and accuracy of parameter estimation and bolstering the reliability of research conclusions.
2 Policy background and theoretical mechanisms
2.1 Policy context of the “Broadband China” strategy
Broadband networks, recognized as strategic public infrastructure, play a pivotal role in advancing informatization, fostering economic growth, and augmenting national competitiveness (Pradhan et al., 2018). To reinforce strategic direction and systematic deployment, and to propel the rapid and sustainable development of broadband infrastructure, the State Council of China promulgated the “Broadband China” strategy and its implementation plan in 2013 (State Council of China, 2013). Subsequently, in 2014, 2015, and 2016, three waves of policy pilot cities were designated with the overarching goal of achieving objectives such as fiber optic connectivity to urban households, widespread broadband coverage in rural areas, and attainment of a certain level of penetration for fixed broadband households, alongside substantial enhancements in broadband application proficiency (Ministry of Industry and Information Technology, 2016). As the “Broadband China” strategy progresses, rural Internet infrastructure has undergone swift expansion. The proliferation of network coverage and accelerated access speeds in rural regions has catalyzed the diffusion and maturation of information technology, thereby propelling the momentum of digital infrastructure development in rural areas (China Internet Network Information Center, 2020).
2.2 Theoretical analysis and research hypotheses
Digital infrastructure construction serves as a “booster” for enhancing grain production capacity. According to agricultural production factor theory, the precise allocation of production factors and the implementation of innovative technological methods are the core drivers of improvements in agricultural productivity. Firstly, digital infrastructure facilitates the restructuring of agricultural production factor allocation, enabling digitalized production and operations. It integrates data elements with traditional agricultural production factors (Dayıoğlu and Turker, 2021), promoting the transformation of grain production methods (Basso et al., 2021), optimizing resource allocation, and enhancing the efficiency of resource utilization (Hu et al., 2023; Cheng et al., 2024). Secondly, the incorporation of information technologies such as the Internet, the Internet of Things, cloud computing, and big data allows digital infrastructure to integrate autonomous perception, intelligent decision-making, and precise control (Son et al., 2024). By establishing agricultural information platforms and utilizing smart agricultural equipment, grain producers can access up-to-date technologies, market insights, and meteorological data, enabling more informed and scientific production decisions (Massruhá et al., 2023). Finally, digital infrastructure construction significantly reduces knowledge acquisition costs by removing information barriers (Fabregas et al., 2019). It enhances the coordination between supply chain inputs and outputs, improves access to rural financial services, reduces financing costs for grain producers (Xiong et al., 2024), and strengthens the synergy between the grain production industry, production systems, and management systems, thereby improving the adaptability and competitiveness of grain production.
Therefore, Hypothesis 1 is proposed: Digital infrastructure construction helps improve grain production capacity.
Hypothesis 2 is proposed: Digital infrastructure construction enhances grain production capacity by promoting technological advancement.
As digital infrastructure construction continues to improve, the rapid diffusion of digital technology into the agricultural field has significantly enhanced the level of intelligence, informatization, and specialization of agricultural production (Chen et al., 2022; Broo and Schooling, 2023). Firstly, digital infrastructure construction promotes the integration of digital technology with traditional production factors, improves the digitization, informatization, and automation of agricultural production (Verdecchia et al., 2022), breaks through the constraints of low production and high risk in traditional production and operation efficiency, improves production efficiency (Deichmann et al., 2016), reduces labor costs and production marginal costs (Yang C. et al., 2024), guides small-scale producers to shift from dispersed production to scaled production, and enhances the enthusiasm of grain producers to expand cultivated land scale (Trendov et al., 2019). Secondly, digital infrastructure construction promotes the sharing and circulation of agricultural information (Fabregas et al., 2019), making land circulation and integration more convenient. Through information platforms, grain producers can learn more about land circulation information and make more reasonable land resource allocations, thereby expanding the scale of cultivated land (Du et al., 2023). Finally, the construction of digital infrastructure can introduce more capital and financial support into the agricultural production field, providing grain producers with more convenient financial services to expand production scale and introduce advanced technologies and equipment, accelerating the expansion of cultivated land scale management (Zhang et al., 2023; Xiong et al., 2024). Moderate scale management can reduce the production cost per unit area, improve the overall efficiency of grain production (Xu et al., 2019), and further enhance grain production capacity.
Therefore, Hypothesis 3 is proposed: Digital infrastructure construction enhances grain production capacity by promoting the scale of cultivated land (Figure 1).
3 Model construction and research design
3.1 Model construction
The “Broadband China” plan is used as an exogenous policy shock proxy to investigate the effects of digital infrastructure on grain production capacity, with a double machine learning model employed to assess the strategy’s impact. The double machine learning method is more suited for this study’s research issues because it overcomes the drawbacks of conventional causal estimation techniques and offers distinct benefits in variable selection, model estimation, and causal inference (Athey et al., 2019). Additionally, several other elements also affect the capability for producing grains, such as the degree of social and economic development, the cost of labor, the availability of arable land, and the climate. It is essential to account for the influence of other factors on grain production capacity to appropriately evaluate the consequences of policies. Double machine learning can automatically select high-dimensional variables, orthogonalize to address bias, and avoid problems caused by the “curse of dimensionality,” redundant variables, and estimation bias (Chernozhukov et al., 2018). Furthermore, there can be nonlinear correlations between factors in the context of how digital infrastructure affects grain production. Double machine learning efficiently avoids the issue of model specification bias in traditional linear regression models by using machine learning methods to handle nonlinear data (Yang et al., 2020). Consequently, in order to assess the policy implications of the “Broadband China” plan and investigate the influence of digital infrastructure on grain production capacity, this study employs a double machine learning model.
We use the “Broadband China” pilot programs that were put into effect in 2014, 2015, and 2016 as quasi-natural experiments to do an empirical test on the effect of building digital infrastructure on grain production capacity. Following the approach outlined by Chernozhukov et al. (2018), we construct a double machine learning model. The partial linear model is formulated as follows:
In Equations (1) and (2), represents the dependent variable, which is grain production capacity, is a binary variable indicating the “Broadband China” policy; represents cities, represents years; is the estimated coefficient of interest, representing the policy effect of the “Broadband China” policy on grain production capacity. is the set of high-dimensional control variables, and its specific form, is estimated using a machine learning algorithm, is the error term, with a conditional mean of 0. By directly estimating the above model, we obtain the estimator for .
In Equation (3), N represents the sample size. Next, we consider the bias of the estimator, denoted as:
In Equation (4), , follows a normal distribution with a mean of 0. b = In addition, dual machine learning uses machine learning and its regularization algorithm to estimate the specific function form , which inevitably introduces “regularization bias.” Although it can prevent the variance of the estimator from being too large, it also leads to its lack of unbiasedness. Specifically, the convergence speed of to is slow, > . Therefore, as tends to infinity, also tends to infinity, and is difficult to converge to .
To reduce estimation bias and accelerate convergence speed, we construct auxiliary regression models1:
In Equations (5) and (6), represents the regression of treatment variables on high-dimensional control variables, where is the error term with a conditional mean of 0. The specific derivation process is as follows:
Estimate the auxiliary regression model using machine learning algorithms, and calculate the residual , according to the following Equation (7):2
Similarly, estimate the main model , using machine learning algorithms, obtaining the following Equation (8):
Use as an instrumental variable for , and conduct regression to obtain unbiased coefficient estimates:
According to the Equation (9), we obtain:
In Equation (10), Follows a normal distribution with mean 0, after two uses of machine learning, the convergence speed of is , which represents the convergence speed of to and to , Compared to Equation (3), converges faster to 0, thus obtaining an unbiased estimate of the coefficient.
3.2 Variable setting
3.2.1 Dependent variable
Based on the research by Bastos et al. (2020), grain production capacity is chosen as the dependent variable, represented by grain yield per unit area. Grain yield per unit area is calculated by dividing total grain production by the total sown area of grains.
3.2.2 Core explanatory variable: “Broadband China” policy dummy variable
The list of pilot cities and the pilot time of the “Broadband China” strategy are matched. A policy dummy variable named “Broadband” is set as a proxy variable for digital infrastructure construction. If region i joins the “Broadband China” demonstration area in year t, then Broadband is set to 1 for that region in year t and all subsequent years, otherwise it is set to 0 (Lv et al., 2023).
3.2.3 Control variables
To ensure the accuracy and stability of policy effect estimates, this study, based on the research by Zhang et al. (2021), as well as Peng et al. (2022), controls for a series of factors that may affect grain production capacity, covering socioeconomic factors, human capital levels, and natural endowments, considering the availability of urban data. Control variables are set as follows:
Socioeconomic Level: Urbanization rate, industrial structure, and level of transportation. Human Capital Level: Education level of rural residents, agricultural labor force, and rural per capita income level; Natural Resource Endowment: Arable land resources, water resources, cropping index, planting structure, and geographical conditions, all reflecting the influence of natural geographic resources on grain production.
We investigate the mechanism through which digital infrastructure construction influences grain production capacity. To elucidate this mechanism, the study will examine two aspects: promoting technological progress and cultivating land management scale. In terms of technological progress, the paper refers to research by He et al. (2021), selecting mechanization level and green technology progress as proxy variables. Specifically, mechanization level is measured by the ratio of total agricultural machinery power to the number of people employed in the primary industry, and agricultural machinery power per agricultural laborer, while green technology progress is measured by the number of green patent applications. Regarding cultivated land management scale, the study draws on research by Duan et al. (2021), utilizing per capita arable land area as a measurement indicator. Through analysis of these mechanism variables, the study aims to comprehensively understand the influence mechanism of digital infrastructure construction on grain production capacity, providing a scientific basis for relevant policy formulation.
3.2.4 Data processing
The sample data mainly comes from various sources such as the “China Statistical Yearbook,” “China Rural Statistical Yearbook,” “China Urban Statistical Yearbook,” provincial and municipal statistical yearbooks, and the official website of the Ministry of Industry and Information Technology. Considering the availability of sample data, a total of 277 prefecture-level cities in China from 2011 to 2021 are selected as the research sample range, and missing data are supplemented using linear interpolation (Table 1).
4 Empirical results analysis and discussion
4.1 Baseline results analysis
Using a double machine learning model, we evaluate the impact of the “Broadband China” policy on grain production capacity.3 The sample is split in a 1:4 ratio, and a gradient boosting algorithm is applied for regression prediction.4 Results in Table 2 column (1) show that, controlling for city and time fixed effects and first-order control variables, the “Broadband China” policy significantly enhances grain production capacity at the 1% level, confirming Hypothesis 1.
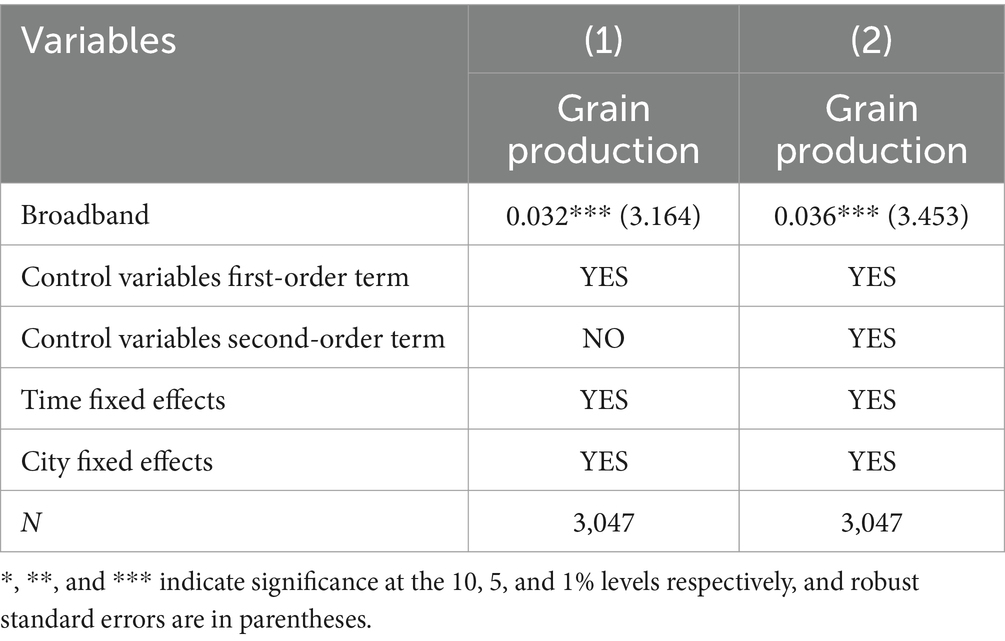
Table 2. Baseline regression results on the impact of digital infrastructure construction on grain production capacity.
In Table 2 column (2), after adding second-order control variables, the positive effect remains significant with minimal change in the coefficient. This confirms that the promotion effect of digital infrastructure on grain production capacity is robust, further verifying Hypothesis 1.
4.2 Handling endogeneity issues
To avoid endogeneity issues caused by omitted variables, we construct a partially linear instrumental variable model using double machine learning, as suggested by Chernozhukov et al. (2018).
In this model, serves as the instrumental variable for . Following Zhang and Li (2023) and Yang S. et al. (2024), this study uses the interaction between 1984 postal and telecommunications data for each prefecture-level city and the time trend as an instrumental variable. Historical postal and telecommunications infrastructure, foundational to modern internet technology, reflects the initial level of digital infrastructure and influences broadband construction, satisfying the relevance condition. Additionally, as 1984 postal data are unrelated to current grain production capacity, the exclusion restriction is met. Column (1) of Table 3 shows a significantly positive coefficient at the 1% level, indicating the “Broadband China” policy enhances grain production capacity. Additionally, following Lv et al. (2023), the interaction between the lagged “Broadband China” policy and the annual national internet growth rate is used as another instrumental variable. This approach meets the relevance and exclusion conditions, as the national internet growth rate is unaffected by city-specific factors. Column (2) of Table 3 shows a significantly positive coefficient at the 5% level, confirming the robustness of the “Broadband China” policy’s impact on grain production capacity.
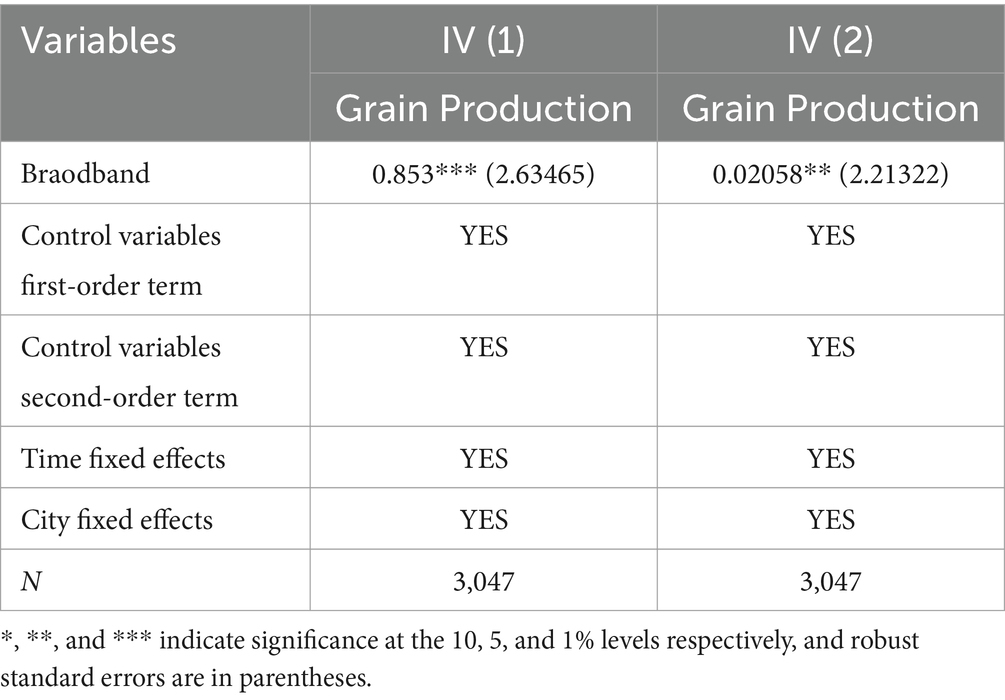
Table 3. Instrumental variable regression results on the impact of digital infrastructure construction on grain production capacity.
4.3 Robustness testing
4.3.1 Excluding the impact of concurrent policies
The sample period of this study is from 2011 to 2021. During this period, other policies related to digital infrastructure construction might affect the robustness of the baseline estimation results. Therefore, we control for other concurrent and similar policies. Upon reviewing relevant policies, we identified that the “National Big Data Comprehensive Pilot Zones” and “Smart Cities” policies might overlap with the “Broadband China” policy. Consequently, we included dummy variables for the “National Big Data Comprehensive Pilot Zones” and “Smart Cities” policies in the regression analysis. Column (1) of Table 4 shows that, even after excluding the interference of these two concurrent policies, the conclusion of this study remains valid.
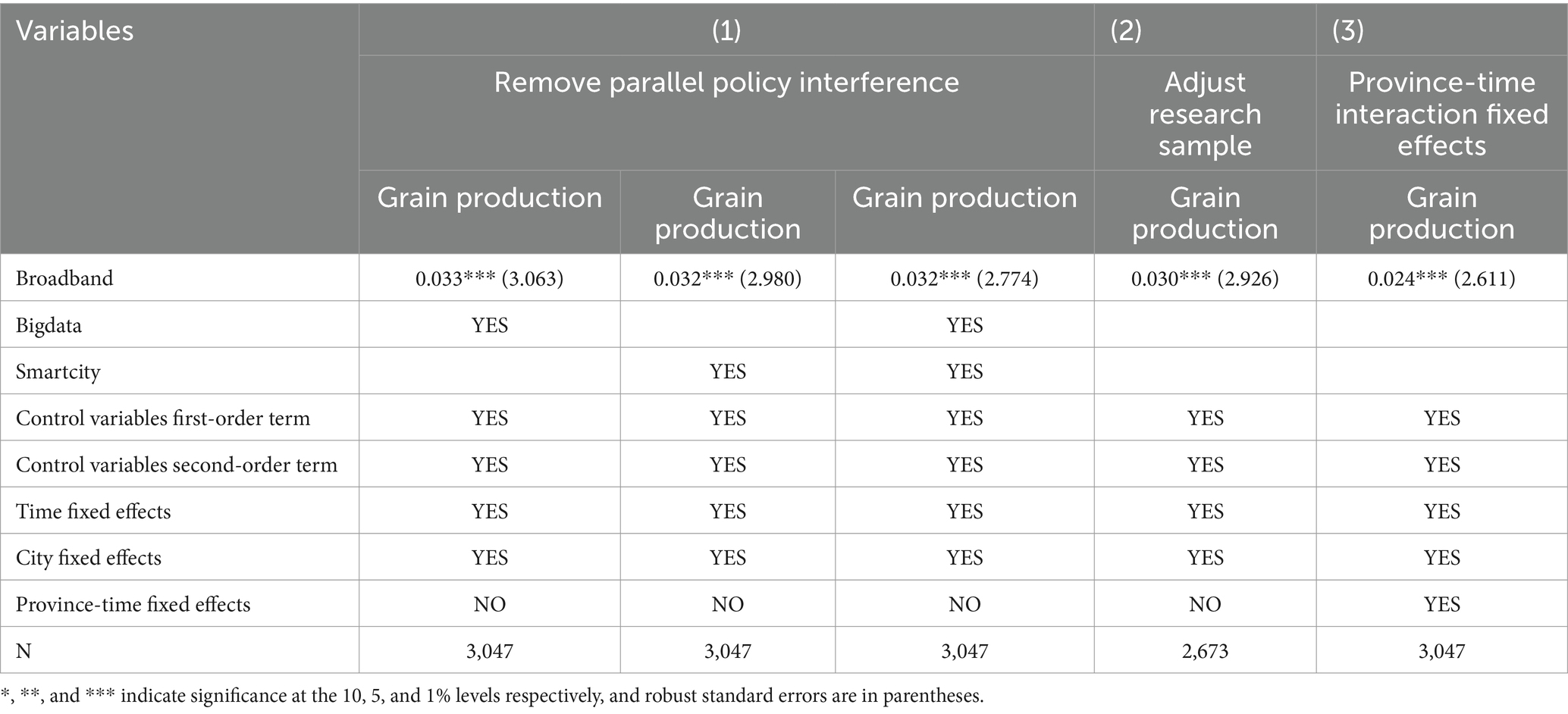
Table 4. Robustness regression results on the impact of digital infrastructure construction on grain production capacity.
4.3.2 Adjusting the research sample
There are significant differences in the levels of economic development and broadband infrastructure among Chinese cities, with central cities having distinct economic advantages. To better identify the impact of the “Broadband China” policy on grain production capacity, we excluded data from municipalities directly under the central government, provincial capital cities, and sub-provincial cities, and re-ran the model. The regression results, as shown in Column (2) of Table 4, indicate that the estimated coefficients remain significantly positive, further affirming the robustness of the baseline regression conclusion.
4.3.3 Province-time interaction fixed effects
Provinces are a crucial administrative level in China’s government structure, and cities within the same province often share similar policy environments, potentially leading to similar external influences on grain production processes. To more accurately estimate the impact of the “Broadband China” strategy on grain production, we included province-time interaction fixed effects to control for potential temporal variations within provinces. The regression results, as shown in Column (3) of Table 4, indicate that the impact of the “Broadband China” policy on grain production remains significantly positive, confirming that the original conclusion holds true.
4.3.4 Respecifying the double machine learning model
To ensure the model’s robustness, the sample splitting ratio in the double machine learning model was adjusted from the baseline 1:4 to 1:2 and 1:7. The regression results in Column (4) of Table 5 remained significantly positive, supporting the original conclusion. Additionally, a more flexible interactive model was employed to avoid specification bias, and the results in Column (5) of Table 5 confirmed the robustness of the “Broadband China” policy’s positive effect on grain production capacity. Finally, alternative algorithms, including lasso, ridge, and random forest regressions, were tested, and the results in Column (6) of Table 5 continued to show a significant positive impact, validating the consistency of the findings.
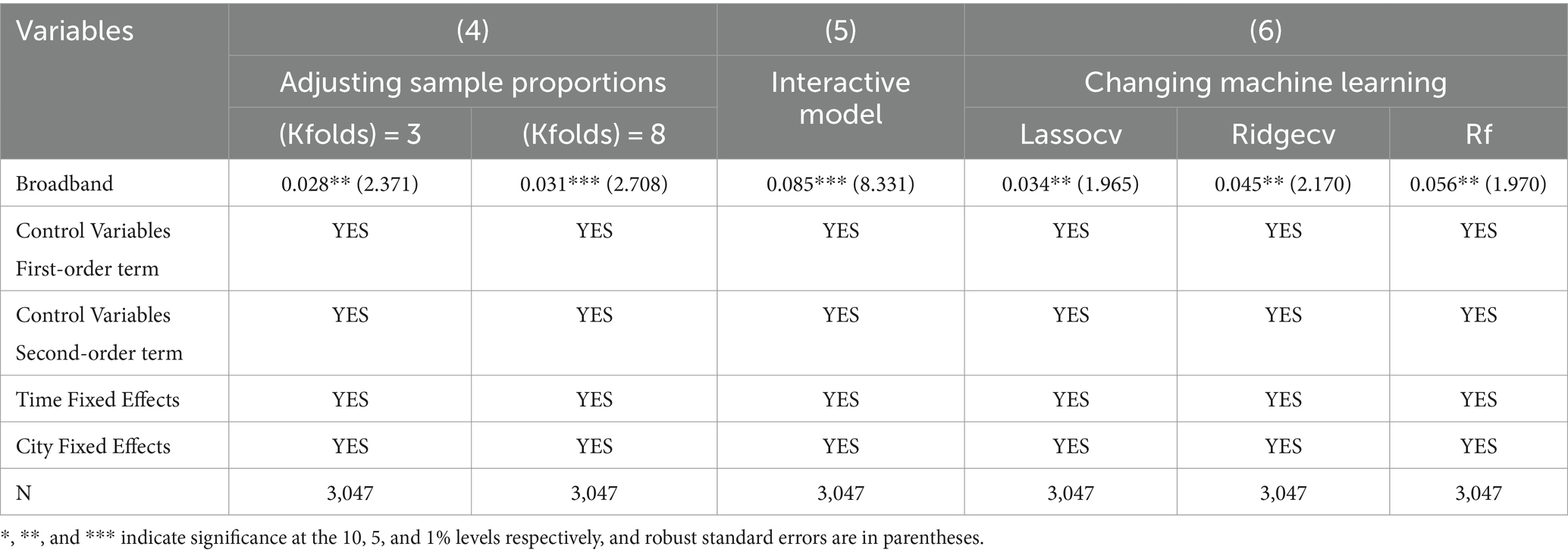
Table 5. Robustness regression results on the impact of digital infrastructure construction on grain production capacity.
4.4 Mechanism examination
This study explores how digital infrastructure development enhances grain production through technological advancement. The results in Column (1) of Table 6 show that the “Broadband China” policy has a significantly positive impact on mechanization levels and green technological innovation at the 1% statistical level. This indicates that digital infrastructure facilitates information sharing, reduces knowledge acquisition costs, accelerates mechanization, and integrates green technology into grain production, fostering innovation-driven growth. As a result, technological advancements contribute to improved grain production capacity, confirming Hypothesis 2, which posits that digital infrastructure construction enhances grain production capacity by promoting technological advancement.
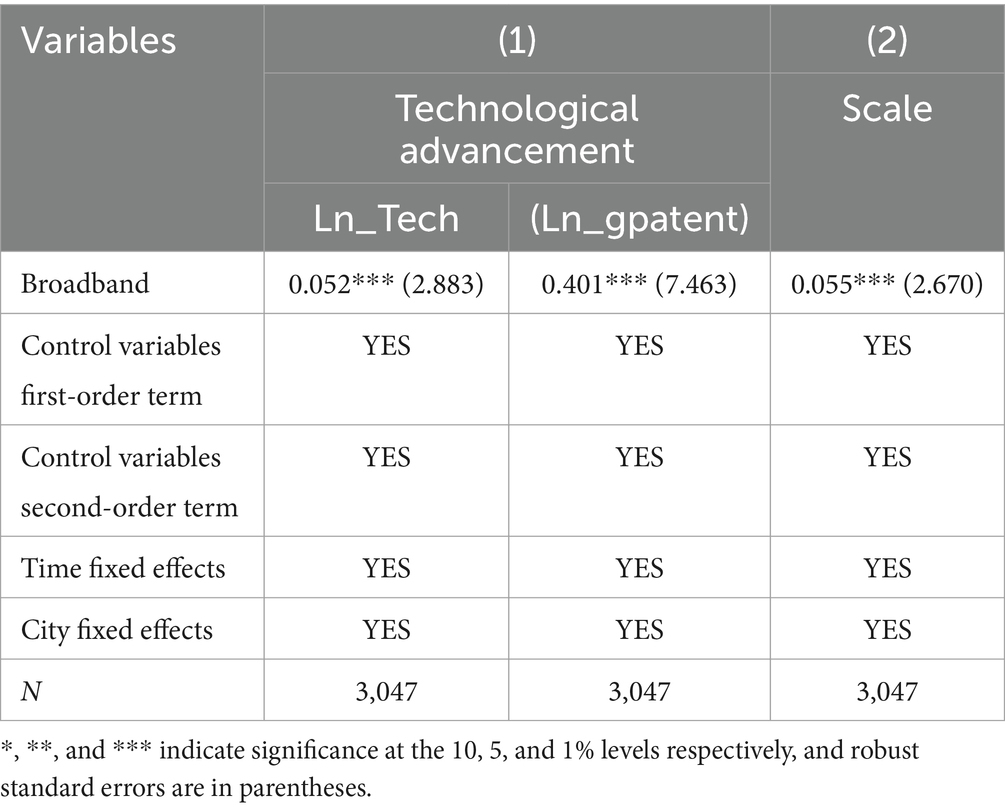
Table 6. Mechanism regression results on the impact of digital infrastructure construction on agricultural technological advancement and farmland scale of grain production.
Furthermore, the results in Column (2) of Table 6 demonstrate that the “Broadband China” policy significantly promotes the scale operation of cultivated land at the 1% statistical level. This suggests that digital infrastructure reduces unit production costs and enhances efficiency by supporting large-scale land management, thereby validating Hypothesis 3, which posits that digital infrastructure construction enhances grain production capacity by promoting the scale of cultivated land management.
4.5 Heterogeneity analysis
This study examines the heterogeneity of digital infrastructure’s impact on grain production capacity across different agricultural regions, dividing the sample into grain production zones, main consumption zones, and production-consumption balance zones. The results, shown in Table 7, Column (1), indicate that the “Broadband China” policy significantly boosts grain production in all zones, with the strongest effect in grain production zones, further supporting hypothesis 1.
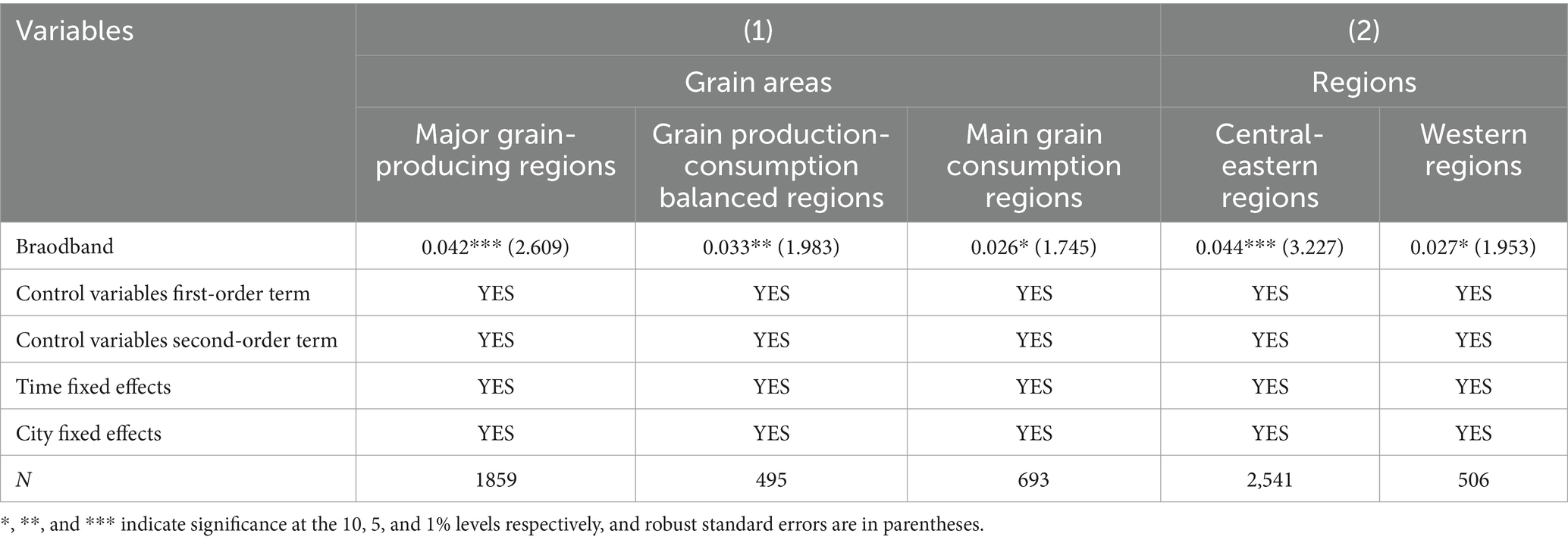
Table 7. Heterogeneity regression results on the impact of digital infrastructure construction on different grain production areas.
In grain production zones, where large areas are dedicated to grain planting, digital infrastructure optimizes resource allocation and modernizes traditional systems, leading to higher efficiency and productivity. In production-consumption balance zones, where both production and market activity are balanced, easier access to knowledge and technology results in a significant positive effect. Main consumption zones, although economically advanced with high digital coverage, have a more moderate impact due to their lower grain production levels.
To further analyze the heterogeneity of the impact of digital infrastructure on grain production, this study divides the sample into regions in the eastern and western parts of China based on the level of economic development. Regression results are presented in Column (2) of Table 7, showing that the impact is more significant in eastern regions, which have better economic development, broadband coverage, and digital infrastructure, allowing for more efficient application of digital technologies in grain production. In western regions, the effect is less pronounced due to lower levels of economic development and less advanced digital infrastructure.
5 Research conclusion and policy recommendations
5.1 Conclusion
In the context of the ongoing global food crisis and the United Nations Sustainable Development Goal (SDG) of achieving “zero hunger,” China plays a pivotal role due to its substantial grain production capacity. As the nation modernizes its agricultural sector, digital infrastructure has become a crucial factor in improving sustainable grain production. This study, based on panel data from 277 prefecture-level cities from 2011 to 2021, investigates the mechanisms through which digital infrastructure enhances agricultural productivity. The findings can be summarized as follows:
Firstly, digital infrastructure construction significantly enhances grain production capacity, a finding that remains robust across various tests. Secondly, digital infrastructure promotes grain production by advancing agricultural technology and expanding farmland management. Thirdly, heterogeneity analysis shows that the impact is most pronounced in major grain-producing regions and the central and eastern areas, while the grain production-consumption balance areas, main sales areas, and western regions show more limited growth potential. Therefore, further efforts are needed to optimize the role of digital infrastructure in boosting grain production in these regions.
5.2 Policy implications
Based on the research results, the following policy recommendations are proposed:
Firstly, it is crucial to strengthen digital infrastructure, particularly in underdeveloped regions, to further strengthen the role of digital infrastructure in increasing grain production capacity and achieving the United Nations Sustainable Development Goals (SDGs). Governments should prioritize expanding broadband networks, reducing the cost of digital access for farmers, and encouraging the development of digital platforms that provide agricultural information. Secondly, policymakers should promote smart agriculture by offering targeted financial support, including subsidies for research and development, equipment purchases, and farmer training programs. Enhancing innovation and improving access to modern agricultural tools will directly contribute to the achievement of the SDGs, particularly in boosting agricultural efficiency and ensuring the long-term sustainability and security of food systems. Thirdly, local factors should be taken into consideration while developing digital infrastructure. Addressing regional disparities through targeted financing, technical assistance, and talent development programs will enhance digital capabilities, especially in developing countries. This focused strategy will enable the digital revolution of agriculture and fully utilize its potential to drive sustainable grain production.
Collectively, these measures will drive more efficient and environmentally sustainable agricultural practices, contributing to the achievement of SDG 2 (Zero Hunger) by ensuring food security, and SDG 9 (Industry, Innovation, and Infrastructure) through the enhancement of technological capabilities in agriculture.
5.3 Research limitations
While this study makes valuable contributions, it has certain limitations. The study’s only source of data is Chinese, which could not accurately reflect the variety of agricultural techniques found around the world. Furthermore, differences in infrastructure development between regions imply that certain communities need more specialized, customized interventions to fully benefit from digital improvements. Future studies should examine how digital infrastructure affects food production over the long run in different nations and areas, as well as how these technologies might be modified for use in a variety of agricultural settings, and the role of digital infrastructure in other industries can be explored.
Data availability statement
The original contributions presented in the study are included in the article/supplementary material, further inquiries can be directed to the corresponding author.
Author contributions
BH: Writing – original draft, Writing – review & editing. TG: Writing – original draft, Writing – review & editing. XC: Writing – review & editing.
Funding
The author(s) declare financial support was received for the research, authorship, and/or publication of this article. This work was supported by Liaoning Provincial Department of Education Project “Research on Industrial Digitalization Empowering New Quality Productivity of Liaoning Equipment Manufacturing Industry” of China (Grant number: LJ112410147080).
Conflict of interest
The authors declare that the research was conducted in the absence of any commercial or financial relationships that could be construed as a potential conflict of interest.
Publisher’s note
All claims expressed in this article are solely those of the authors and do not necessarily represent those of their affiliated organizations, or those of the publisher, the editors and the reviewers. Any product that may be evaluated in this article, or claim that may be made by its manufacturer, is not guaranteed or endorsed by the publisher.
Footnotes
1. ^In Double Machine Learning, orthogonality ensures that errors in the nuisance functions (i.e., the machine learning predictions for the treatment and outcome models) do not bias the estimate of the treatment effect. The orthogonal score function is used to achieve this and is constructed as follows:
Where: is the treatment effect parameter of interest, are the nuisance functions, represents the entire data set (including , , ), is the model for the treatment variable, and is the model for the outcome.
2. ^Orthogonality in Double Machine Learning ensures that errors in the nuisance functions (machine learning models for the treatment and outcome) do not bias the treatment effect estimate. Residuals from the first stage, , are orthogonal to second-stage errors . This implies , making the residuals centered around zero, and eliminating the need for a constant term in the second-stage regression.
3. ^We implemented the method using the ddml package as described by Zhang and Li (2023).
4. ^The 1:4 split refers to a holdout method used to create training and testing datasets. In this process: 80% of the data was used for training the machine learning models, and 20% was reserved for testing to evaluate model performance. We employed K-fold cross-validation (with K = 5) within the training set to tune hyperparameters and avoid overfitting.
References
Athey, S., Tibshirani, J., and Wager, S. (2019). Generalized random forests. J. Mach. Learn. Res. 47, 1148–1178. doi: 10.1214/18-AOS1709
Basso, B., Jones, J. W., Antle, J., Martinez-Feria, R. A., and Verma, B. (2021). Enabling circularity in grain production systems with novel technologies and policy. Agric. Syst. 193:103244. doi: 10.1016/j.agsy.2021.103244
Bastos, L. M., Carciochi, W., Lollato, R. P., Jaenisch, B. R., Rezende, C. R., Schwalbert, R., et al. (2020). Winter wheat yield response to plant density as a function of yield environment and tillering potential: a review and field studies. Front. Plant Sci. 11:54. doi: 10.3389/fpls.2020.00054
Broo, D. G., and Schooling, J. (2023). Digital twins in infrastructure: definitions, current practices, challenges and strategies. Int. J. Constr. Manag. 23, 1254–1263. doi: 10.1080/15623599.2021.1966980
Cao, X., Wu, N., Adamowski, J., and Wu, M. (2023). Assessing the contribution of China's grain production during 2005–2020 from the perspective of the crop-water-land nexus. J. Hydrol. 626:130376. doi: 10.1016/j.jhydrol.2023.130376
Chen, W., Wang, Q., and Zhou, H. (2022). Digital rural construction and farmers’ income growth: theoretical mechanism and micro experience based on data from China. Sustain. For. 14:11679. doi: 10.3390/su141811679
Cheng, M., Wang, J., Yang, S., and Li, Q. (2024). The driving effect of technological innovation on green development: from the perspective of efficiency. Energy Policy 188:114089. doi: 10.1016/j.enpol.2024.114089
Chernozhukov, V., Chetverikov, D., Demirer, M., Duflo, E., Hansen, C., Newey, W., et al. (2018). Double/debiased machine learning for treatment and structural parameters. Econ. J. 21, C1–C68. doi: 10.1111/ectj.12097
China Internet Network Information Center . (2020). Statistical report on internet development in China. Available online at: https://www.cnnic.net.cn
Dayıoğlu, M. A., and Turker, U. (2021). Digital transformation for sustainable future – agriculture 4.0: a review. Agric. Sci. 27, 373–399. doi: 10.15832/ankutbd.986431
Deichmann, U., Goyal, A., and Mishra, D. (2016). Will digital technologies transform agriculture in developing countries? Agric. Econ. 47, 21–33. doi: 10.1111/agec.12300
Ding, W., Wu, Q., and Xu, X. (2024). Digital infrastructure construction and improvement of non-farm employment quality of rural labor force—from the perspective of informal employment. Sustain. For. 16:5345. doi: 10.3390/su16135345
Dolgui, A., and Ivanov, D. (2022). 5G in digital supply chain and operations management: fostering flexibility, end-to-end connectivity and real-time visibility through internet-of-everything. Int. J. Prod. Res. 60, 442–451. doi: 10.1080/00207543.2021.2002969
Du, X., Wang, X., and Hatzenbuehler, P. (2023). Digital technology in agriculture: a review of issues, applications and methodologies. China Agric. Econ. Rev. 15, 95–108. doi: 10.1108/CAER-01-2022-0009
Duan, J., Ren, C., Wang, S., Zhang, X., Reis, S., Xu, J., et al. (2021). Consolidation of agricultural land can contribute to agricultural sustainability in China. Nat Food 2, 1014–1022. doi: 10.1038/s43016-021-00415-5
Fabregas, R., Kremer, M., and Schilbach, F. (2019). Realizing the potential of digital development: the case of agricultural advice. Science 366:eaay3038. doi: 10.1126/science.aay3038
Giller, K. E., Delaune, T., Silva, J. V., Descheemaeker, K., van de Ven, G., Schut, A. G. T., et al. (2021). The future of farming: who will produce our food? Food Secur. 13, 1073–1099. doi: 10.1007/s12571-021-01184-6
Guo, Y., Zhao, H., Zhang, S., Wang, Y., and Chow, D. (2021). Modeling and optimization of environment in agricultural greenhouses for improving cleaner and sustainable crop production. J. Clean. Prod. 285:124843. doi: 10.1016/j.jclepro.2020.124843
Hao, S., Wang, G., Yang, Y., Zhao, S., Huang, S., Liu, L., et al. (2024). Promoting grain production through high-standard farmland construction: evidence in China. J. Integr. Agric. 23, 324–335. doi: 10.1016/j.jia.2023.11.021
He, P., Zhang, J., and Li, W. (2021). The role of agricultural green production technologies in improving low-carbon efficiency in China: necessary but not effective. J. Environ. Manag. 293:112837. doi: 10.1016/j.jenvman.2021.112837
Hu, J., Zhang, H., and Irfan, M. (2023). How does digital infrastructure construction affect low-carbon development? A multidimensional interpretation of evidence from China. J. Clean. Prod. 396:136467. doi: 10.1016/j.jclepro.2023.136467
Jose, A., Deepak, K. S., and Rajamani, N. (2024). Innovation in agriculture and the environment: a roadmap to food security in developing nations. Food Secur. 9, 152–164. doi: 10.1007/s11356-023-15432-7
Kliestik, T., Kral, P., Bugaj, M., and Durana, P. (2024). Generative artificial intelligence of things systems, multisensory immersive extended reality technologies, and algorithmic big data simulation and modelling tools in digital twin industrial metaverse. Equilibrium 19, 429–461. doi: 10.24136/eq.3108
Kliestik, T., Nica, E., Durana, P., and Popescu, G. H. (2023). Artificial intelligence-based predictive maintenance, time-sensitive networking, and big data-driven algorithmic decision-making in the economics of industrial internet of things. Oeconomia Copernicana 14, 1097–1138. doi: 10.24136/oc.2023.033
Leukel, J., Zimpel, T., and Stumpe, C. (2023). Machine learning technology for early prediction of grain yield at the field scale: a systematic review. Comput. Electron. Agric. 207:107721. doi: 10.1016/j.compag.2023.107721
Lima, G. C., Figueiredo, F. L., Barbieri, A. E., and Seki, J. (2020). Agro 4.0: enabling agriculture digital transformation through IoT. Rev. Ciênc. Agron. 51:e20207771. doi: 10.5935/1806-6690.20200100
Lutz, É., and Coradi, P. C. (2022). Applications of new technologies for monitoring and predicting grains quality stored: sensors, internet of things, and artificial intelligence. Measurement 188:110609. doi: 10.1016/j.measurement.2021.110609
Lv, K., Li, J., and Zhao, Y. (2023). Can internet construction promote urban green development? A quasi-natural experiment from the “broadband China”. Int. J. Environ. Res. Public Health 20:4709. doi: 10.3390/ijerph20064709
Maroušek, J., Minofar, B., Maroušková, A., Strunecký, O., and Gavurová, B. (2023). Environmental and economic advantages of production and application of digestate biochar. Environ. Technol. Innov. 30:103109. doi: 10.1016/j.eti.2023.103109
Massruhá, S. M. F. S., Leite, M. A. A., Luchiari Júnior, A., and Evangelista, S. R. M. (2023). Digital transformation in the field towards sustainable and smart agriculture. Available at: http://www.alice.cnptia.embrapa.br/alice/handle/doc/1156698
Ministry of Industry and Information Technology . (2016). Announcement on the pilot cities for the "broadband China" strategy. Available online at: http://www.miit.gov.cn
Morchid, A., Alblushi, I. G. M., Khalid, H. M., and El Alami, R. (2024). High-technology agriculture system to enhance food security: a concept of smart irrigation system using internet of things and cloud computing. J. Agric. Res. 78, 110–125. doi: 10.1016/j.agrres.2024.02.011
Naqvi, R. Z., Farooq, M., Zahid, R., Imran, I., and Mahmood, M. A. (2024). Recent advances in plant phenomics and speed breeding for climate-smart agriculture. J. Sustain. Agric. 14, 113–130. doi: 10.1201/b23372-5
National Bureau of Statistics of China . (2023). National statistical data. Available online at: http://www.stats.gov.cn
Peng, Y., Liu, B., and Zhou, M. (2022). Sustainable livelihoods in rural areas under the shock of climate change: evidence from China labor-force dynamic survey. Sustain. For. 14:7262. doi: 10.3390/su14127262
Pradhan, R. P., Mallik, G., and Bagchi, T. P. (2018). Information communication technology (ICT) infrastructure and economic growth: a causality evinced by cross-country panel data. IIMB Manag. Rev. 30, 91–103. doi: 10.1016/j.iimb.2018.01.001
Ren, J., Chen, X., Shi, L., Liu, P., and Tan, Z. (2024). Digital Village construction: a multi-level governance approach to enhance Agroecological efficiency. Agriculture 14:478. doi: 10.3390/agriculture14030478
Shamdasani, Y. (2021). Rural road infrastructure and agricultural production: evidence from India. J. Dev. Econ. 152:102686. doi: 10.1016/j.jdeveco.2021.102686
Son, N., Chen, C. R., and Syu, C. H. (2024). Towards artificial intelligence applications in precision and sustainable agriculture. Agronomy 14:239. doi: 10.3390/agronomy14020239
State Council of China . (2013). Broadband China strategy and implementation plan. Available online at: http://www.gov.cn
Storm, H., Seidel, S. J., Klingbeil, L., Ewert, F., Vereecken, H., Amelung, W., et al. (2024). Research priorities to leverage smart digital technologies for sustainable crop production. Eur. J. Agron. 156:127178. doi: 10.1016/j.eja.2024.127178
Sun, T. T., Su, C. W., Mirza, N., and Umar, M. (2021). How does trade policy uncertainty affect agriculture commodity prices? Pac. Basin Financ. J. 66:101514. doi: 10.1016/j.pacfin.2021.101514
Tian, X., Yi, F., and Yu, X. (2020). Rising cost of labor and transformations in grain production in China. China Agric. Econ. Rev. 12, 158–172. doi: 10.1108/CAER-04-2018-0067
Trendov, N. M., Varas, S., and Zeng, M. (2019). Digital technologies in agriculture and rural areas. Available at: https://openknowledge.fao.org/handle/20.500.14283/CA4887EN
Verdecchia, R., Lago, P., and De Vries, C. (2022). The future of sustainable digital infrastructures: a landscape of solutions, adoption factors, impediments, open problems, and scenarios. Sustain. Comput. 35:100767. doi: 10.1016/j.suscom.2022.100767
Wang, C. C., Zhang, Q. C., Kang, S. G., Li, M. Y., Zhang, M. Y., Xu, W. M., et al. (2023). Heavy metal(loid)s in agricultural soil from main grain production regions of China: bioaccessibility and health risks to humans. Sci. Total Environ. 858:159819. doi: 10.1016/j.scitotenv.2022.159819
Warsame, A. A., Sheik-Ali, I. A., Jama, O. M., Hassan, A. A., and Barre, G. M. (2022). Assessing the effects of climate change and political instability on sorghum production: empirical evidence from Somalia. J. Clean. Prod. 360:131893. doi: 10.1016/j.jclepro.2022.131893
Wu, W., Cui, H., Han, F., Liu, Z., Wu, X., Wu, Z., et al. (2021). Digital monitoring of grain conditions in large-scale bulk storage facilities based on spatiotemporal distributions of grain temperature. Biosyst. Eng. 210, 247–260. doi: 10.1016/j.biosystemseng.2021.08.028
Xie, S., Zhang, J., Li, X., Xia, X., and Chen, Z. (2024). The effect of agricultural insurance participation on rural households' economic resilience to natural disasters: evidence from China. J. Clean. Prod. 434:140123. doi: 10.1016/j.jclepro.2023.140123
Xiong, Q., Guo, X., and Yang, J. (2024). Digital financial inclusion, land circulation and high-quality development of agriculture. Sustain. For. 16:4775. doi: 10.3390/su16114775
Xu, Y., Xin, L., Li, X., Tan, M., and Wang, Y. (2019). Exploring a moderate operation scale in China’s grain production: a perspective on the costs of machinery services. Sustain. For. 11:2213. doi: 10.3390/su11082213
Yang, T., Chandio, A. A., Zhang, A., and Liu, Y. (2023). Do farm subsidies effectively increase grain production? Evidence from major grain-producing regions of China. Food Secur. 12:1435. doi: 10.3390/foods12071435
Yang, J. C., Chuang, H. C., and Kuan, C. M. (2020). Double machine learning with gradient boosting and its application to the big N audit quality effect. J. Econ. 216, 268–283. doi: 10.1016/j.jeconom.2020.01.018
Yang, C., Ji, X., Cheng, C., Liao, S., Obuobi, B., and Zhang, Y. (2024). Digital economy empowers sustainable agriculture: implications for farmers’ adoption of ecological agricultural technologies. Ecol. Indic. 159:111723. doi: 10.1016/j.ecolind.2024.111723
Yang, S., Li, H., Liu, X., Wei, Y., Shen, J., and Cheng, B. (2024). Spatial analysis of the digital economy’s influence on urban sustainable development: a decade-long study of Chinese prefecture-level cities. Ecol. Indic. 166:112338. doi: 10.1016/j.ecolind.2024.112338
Zhang, T., and Li, J. (2023). Network infrastructure, inclusive green growth, and regional inequality: from causal inference based on double machine learning. J. Quant. Technol. Econ 40, 113–135.
Zhang, D., Wang, H., and Lou, S. (2021). Research on grain production efficiency in China’s main grain-producing areas from the perspective of grain subsidy. Environ. Technol. Innov. 22:101530. doi: 10.1016/j.eti.2021.101530
Zhang, H., Zhang, Q., An, M., Zhang, Z., and He, N. (2023). Unveiling the impact of digital financial inclusion on low-carbon green utilization of farmland: the roles of farmland transfer and management scale. Sustain. For. 15:3556. doi: 10.3390/su15043556
Keywords: food security, sustainable grain production, digital infrastructure, double machine learning, technology innovation
Citation: Han J, Ge W and Chou Y (2024) Leveraging digital infrastructure for sustainable grain production: evidence from China. Front. Sustain. Food Syst. 8:1440321. doi: 10.3389/fsufs.2024.1440321
Edited by:
Albie F. Miles, University of Hawaii–West Oahu, United StatesReviewed by:
Stanislav Škapa, Brno University of Technology, CzechiaMiguel Sellitto, University of the Rio dos Sinos Valley, Brazil
Michael Phillips, Consultant, Pasadena, CA, United States
Copyright © 2024 Han, Ge and Chou. This is an open-access article distributed under the terms of the Creative Commons Attribution License (CC BY). The use, distribution or reproduction in other forums is permitted, provided the original author(s) and the copyright owner(s) are credited and that the original publication in this journal is cited, in accordance with accepted academic practice. No use, distribution or reproduction is permitted which does not comply with these terms.
*Correspondence: Jiabin Han, Z3d0MTEzNTM5MzgwN0AxNjMuY29t