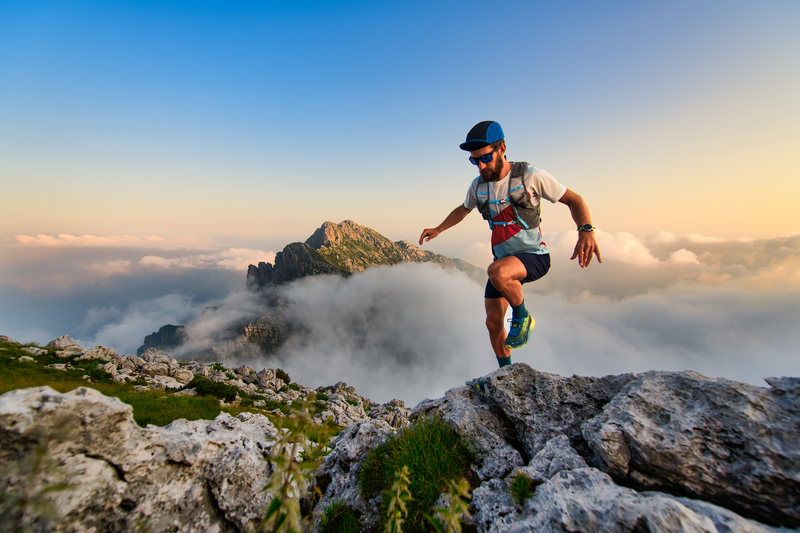
94% of researchers rate our articles as excellent or good
Learn more about the work of our research integrity team to safeguard the quality of each article we publish.
Find out more
COMMUNITY CASE STUDY article
Front. Sustain. Food Syst. , 04 June 2024
Sec. Urban Agriculture
Volume 8 - 2024 | https://doi.org/10.3389/fsufs.2024.1345598
This article is part of the Research Topic Urban Agriculture as Local Food Systems: Benefits, Challenges, and Ways Forward View all 8 articles
High-tech vegetable production is becoming a priority in agricultural development in Vietnam in the context of digital economic development. This study aims at identifying factors involving the adoption of high technology in vegetable production by local farmers in Hanoi. We used the theory of planned behavior and other farmers’ personal, social and economic factors to develop empirical model and hypotheses. Primary data was collected from a survey of 450 vegetable producers in Hanoi using cluster sampling method combined with random selection. Then, binary logit model was used to analyze the impact of influencing factors. Results showed that there were 7 factors having significant influences the decision to apply technology in vegetable production of farmers including attitude on high tech production, access to information, size of farm, member of extension organization, education level, access to credit and perceived behavior control, in which attitude variable was the most influential factor. Main management implications raised included enhancing access to technological information, providing demostration visits, giving more extension services, improving social inclusion and implementing hi- tech training for farmers in vegetable production.
In the context of the industrial revolution 4.0, high-tech agriculture plays a very important role in the process of restructuring agricultural production and is a solution to solve the problem of food security and improve quality. Agricultural products and environmentally friendly (Cavatassi et al., 2011; Aung et al., 2021; Truong et al., 2022). Currently, the application of high technology in agricultural production has been replicated in developed and developing countries to improve productivity, meet the market’s demand for agricultural product quality and ensure food security (Cavatassi et al., 2011; Dalton et al., 2011; Kassie et al., 2015; Basuki et al., 2019). In the field of vegetable production, to achieve the above goals, high tech vegetable (HTV) practices have been introduced and applied in many forms from managing soil structure, saving irrigation water, diversifying crops. and use organic fertilizers. HTV brings many benefits to farmers and depending on specific conditions, the benefits may be different (Bokusheva et al., 2012; Fisher et al., 2015; Fischer, 2016; Khonje et al., 2018). The general benefits of proven technology models include being environmentally friendly, protecting ecosystems, using resources efficiently and having high economic value. Despite the above superior attributes and clear potential benefits, the use of high technology in vegetable growing is still low in developing countries that rely heavily on agriculture (Katengeza et al., 2018; Lam et al., 2018; Truong et al., 2022).
As to Liu et al. (2018), HTV practices adoption is a dynamic process that depends on factors such as farming households’, farms’ features, environmental challenges, and government supporting policies. For example, Moser and Barrett (2016) argued that HTV producing depended on farmers’ personal, economic, social and cultural characteristics. Pardey et al. (2016a,b) also implied that factors such as financial investment and knowledge of HTV might explain their application. However, the literature concluded that there were no universal factors can explain HTV adoption and that factor differ based on the contexts (Teklewold et al., 2013; Rapsomanikis, 2015; Sharma, 2015). In developed nations, for example, Kassie et al. (2015) indicated that factors like capital, social networking, and access to information are positively related to HTV adoption. Larsen (2018) also found that HTV practices adoption is positively related to education level, gender, land tenure and farm size.
With favorable conditions and diverse climates, Vietnam has long been known for its well-developed agriculture, in which growing vegetables is essential because green vegetables are an important source of the Vietnam people’s food (Le and Nguyen, 2019, Mai and Truong, 2022). Facing with increasingly complex challenges, such as climate change, international competition, and growing demands for food safety, Vietnam has been accelerating agricultural modernization and improving methods in vegetable production (Le and Truong, 2019). Although HTV growing has contributed to agricultural development and improved farmer welfare, policies promoting high-tech agriculture in general and HTV growing in particular in Vietnam still have shortages. The fundamental knowledge for growing vegetables is still based on traditional processes and there is a great need to improve reliable scientific information to promote the application of modern and sustainable vegetable growing technologies (Dat and Truong, 2020; Truong et al., 2022).
This growing need is based on the fact that high-tech vegetables have economic, health, ecological and cultural value (Le and Truong, 2019). Firstly, high-tech vegetable growing with superior attributes over traditional vegetable growing can contribute to poverty reduction, malnutrition and ensuring food security. In the context of people in Vietnamese urban areas increasingly favoring foods of origin and safety, high-tech vegetables will help customers better identify production processes, safety features and domestically brands (Dat and Truong, 2020). High-tech vegetables also contribute to eliminating nutritional deficiencies in the meals of children and women. They also have higher market prices with an inexpensive investment process if knowledge and support are available, thereby helping farmers improve their livelihood income and long-term economic incentives for community (Truong et al., 2022). Secondly, switching to high-tech vegetable growing will also help improve social aspects of agricultural production, which is the participation of women and the poor in management processes and application of new technology, hence contributing to increasing knowledge for the community. In addition, the dissemination of new techniques and supporting information will also make the network of local civic organizations grow stronger, which increases social inclusion in agricultural and community development (Le and Nguyen, 2019). Thirdly, high-tech vegetables are often better adapted to harsh climatic conditions and are short duration crops. With usually shorter growing cycles than staple crops, high-tech vegetables can be less affected by environmental threats such as temperature fluctuations and drought. Basically, they require less space than traditional crops and can maximize natural resources when water and nutrients are scarce. This makes them suitable for Vietnam as the area continues to experience shorter and unreliable rainfall patterns under rapidly changing and unpredictable climatic conditions (Le and Truong, 2019; Dat and Truong, 2020; Truong et al., 2022).
In the literature, up to now, the majority of studies on technology application in agricultural production focus on a certain group of solutions or an acceptance model in the form of ‘Yes’ or ‘No’ with influencing factors are separate from each other. There are studies that focus on psychological factors such as studies by Moser and Barrett (2016), Lam et al. (2018), and Mulema et al. (2022). Besides, there are studies focusing on physical factors and socio-economic characteristics of farming households. Some other studies give priority to factors belonging to support policies and the external social environment (Kassie et al., 2015; Katengeza et al., 2018; Aung et al., 2021). There have not been many studies that combine these groups of factors to have a more complete picture of the drivers of technology adoption in agricultural production by farmers in developing countries (Lam et al., 2018; Dat and Truong, 2020; Bassyouni et al., 2022).
This article fills in the above gap with the purpose of analyzing factors affecting technology acceptance in vegetable production by smallholder farmers in a country with an emerging economy, Vietnam. While most recent literature only analyzed the impact of factors separately (e.g., experience, education, training or access to credit), this study analyzed them simultaneously, in particular, the interaction between psychological factors, farmer characteristics and environmental factors in driving the application of technology in vegetable production. Therefore, this is one of the first studies to mix groups of factors to find the interaction between them and their influence on the technology acceptance behavior of farmers. We believe that such an approach is essential to design policies and solutions to promote technology applications in agricultural production in general and vegetable production in particular in developing countries.
Our article is organized as follows: section 2 introduces the analysis framework and model development; section 3 describes data collection and analysis process; section 4 presents the study results and discussions; section 5 includes conclusions and management implications.
According to Mukasa (2018), it is possible to plan solutions to promote agricultural application of high technology among farmers if the factors determining their behavior to apply high technology in production are identified. So far, researchers have used a number of adoption models to explain intentions actual behaviors, in which Theory of Planned Behavior (TPB) is one of the most commonly used theories. The TPB model assumes that a behavior can be predicted or explained by intentions to perform that behavior. Ajzen (1991) believed that intention is a function of three influencing factors including, attitudes toward behavior; subjective norms and perceived behavioral control. Dima (2013) implied that TPB theory is suitable for empirical research in identifying important factors from which policies and solutions can be proposed - it is one of the best models to implement policies and solutions after research. TPB has been applied in many empirical studies on technology acceptance behavior of individuals, households and businesses and is suitable for the context of many countries around the world (Gadenne et al., 2011; Dima, 2013; Elmustapha et al., 2018).
In addition, the decision to adopt new technology is often based on comparing the volatile benefits of new initiatives with the costs of adoption (Kristjanson et al., 2015; Ankuyi and Tham, 2022). Lambrecht et al. (2014) added social network factors to the factors affecting the application of technology. Although there are many ways to categorize factors to determine the application of technology, the classification depends on the current technology being studied, the location, and the researcher’s interest in choosing the suitable study (Larsen, 2018). In this study, we combine factors from the TPB and other personal, social and economic factors of households identified in previous studies to analyze determinants of high-tech vegetable production in Hanoi. The proposed analytical framework is shown in Figure 1.
Attitude on high tech production: TPB proves that individual attitude is a crucial factor that directly affects intentional behavior, and this hypothesis has been verified through various research papers in technological application behaviors (Ajzen, 1991; Khonje et al., 2015). Basically, an individual positive or negative attitude toward a behavior is related to his evaluation of the outcome of that behavior (Baumgartetz et al., 2012; Kristjanson et al., 2015). Elmustapha et al. (2018) found that farmer’ attitudes toward high tech production relating to their evaluation about the form, price, process and benefits of the application. In addition, Dima (2013) indicated that attitudes not only include subjective perceptions and personal feelings about the advantages or disadvantages of a solution but also involve the compatibility between the values that the solution may bring with that individual’s expectations. When people have a positive attitude toward technology, their likelihood of accepting the use of technology may also increase (Gadenne et al., 2011; Kassie et al., 2015; Negatu and Parikh, 2019).
Hypothesis H1: Attitude on high tech production has positive impact on the adoption of HTV production.
Perceived benefits: Perceived benefits are related to the willingness to adopt something new compared to traditional practice (Kristjanson et al., 2015; Elmustapha et al., 2018). User behavior is shaped by the perception of higher benefits achieving through the use of a specific solution (Gadenne et al., 2011). Mottaleb et al. (2016) argued in their theoretical framework that perceived benefits can be defined as the extent to which users believe that using products/services will yield significant effectiveness for them. In the case of applying technology to agricultural production, Mukasa (2018) believed that the characteristics of the technology play an essential role in determining its application. When farmers consider technology adoption, they decide whether the technology has positive, efficient, and profitable (Muthumanickam et al., 2022). Additionally, farmers expect high farm income to increase their capital, enabling them to increase such as improved cultivars, seeds, and fertilizer quality. The relationship between perceived benefits and technology adoption plays a vital role in household decisions about technology adoption in agriculture production (Negatu and Parikh, 2019).
Hypothesis H2: Perceived benefit has positive impact on the adoption of HTV production.
Subjective norm: Ajzen (1991) defined subjective norms, also known as social influence, as the perceptions of influencers who think that an individual should or should not perform a behavior. Subjective norms can be described as an individual’s perception of social pressures to perform or not perform a behavior (Dima, 2013). According to TPB, subjective norms can be formed through sensing normative beliefs from people or social factors that influence consumers (such as family, friends, colleagues, media...). The degree of impact of subjective normative belief factors on consumers’ buying tendency depends on: (1) the level of support/opposition for the consumer’s purchase and (2) the consumer’s motivation (Gadenne et al., 2011; Liu et al., 2018). In case of farming, farmers may follow the wishes of influencers. The degree of influence of related people on application behavioral trends and the motivation to follow related people are two basic factors to evaluate subjective norms. The stronger the level of intimacy of the people involved with the individual, the greater the influence on their high tech application. Researches by Meijer et al. (2015) and Moser and Barrett (2016) found that social factors such as influence from family and society are important sources affecting people’s interest in applying new technology. Some other studies built and tested a model based on TPB with the effects of social agents (family, society, government, media, and communication) in addition to attitudes also show significant relation with actual behaviors (Matuschke and Qaim, 2001; Njuki et al., 2018; Negatu and Parikh, 2019).
Hypothesis H3: Subjective norm has positive impact on the adoption of HTV production.
Perceived behavioral control: Each individual’s perceived behavioral control is related to their self-assessment of the difficulty or ease of performing a behavior (Pardey et al., 2016a,b; Elmustapha et al., 2018). According to Ajzen (1991), this perceived control factor comes from the confidence of the individual who intends to perform the behavior and the easy and favorable conditions for performing the behavior. The more resources and opportunities they have, the less resistance they think there will be, and the greater the perceived control over behavior will be. Ntshangase et al. (2018) believed that perceived behavioral control is measured through the person intending to perform the behavior’s awareness of having sufficient information and other necessary conditions for his or her decision.
Hypothesis H4: Perceived behavioral control has positive impact on the adoption of HTV production.
Farm size: The size of the farm has a positive or negative influence on the decision to apply technology in farming in many empirical studies (Lowder et al., 2016; Ojiako et al., 2017; Noack and Larsen, 2019). A study in Malawi demonstrated a positive relationship between farm size and farmers’ decision to apply technology (Orr et al., 2015). Prager and Posthumus (2020) also found a similar relationship in Europe in the case of coffee farmers. However, some studies show a negative relationship between farm size and the application of new technologies in agriculture (Noack and Larsen, 2019). Small-scale farms are often encouraged to adopt technology, especially in cases where innovation requires limited inputs such as labor or land. In addition, Ojiako et al. (2017) in Nigeria indicated that farmers with small land had higher motivation to apply land-saving technology to increase productivity.
Hypothesis H5: Farm size has positive impact on the adoption of HTV production.
Household size: There is a relationship between household size and technology application in agricultural production (Sharma, 2015; Shiferaw et al., 2015). Research by Sitko et al. (2014) discovered that there is a positive relationship between these two variables in Zambia. Households with many members will have an easier time meeting the number of workers, leading to reduced pressure on labor costs in the early stages of technology application. Therefore, they tend to apply higher technology than families with fewer members. However, Sodjinou et al. (2016) found a negative relationship exists between household size and the adoption of new technology in rice farming in Benin. The author explains that when households have more members, they need more spending for other household purposes and less funds to adopt new technology, so they tend to reduce interest. in long-term investments for technology adoption. There are also some studies that do not find a significant relationship between household size and technology adoption in agriculture (Rapsomanikis, 2015; Teshome et al., 2016).
Hypothesis H6: Household size has positive impact on the adoption of HTV production.
Education: Education level is positively related to technology application in agriculture in many previous studies (Teklewold et al., 2013; Ogada et al., 2014; Shiferaw et al., 2018). The higher the level of education of farmers, the easier it will be for them to access and use information related to the application of new technology. Studies on applying new technologies in aquaculture and organic fertilizers have concluded that educational attainment significantly affects households’ technology adoption (Ndiritu et al., 2014; Aung et al., 2021). Highly educated farm owners often accumulate more knowledge and experience over time, providing a better evaluation of the potential of applying technology.
Hypothesis H7: Education level has positive impact on the adoption of HTV production.
Membership of agricultural extension organizations: Agricultural extension activities have an important impact on farmers’ technology adoption behavior (Mulema et al., 2022). In developing countries, local agricultural extension associations are the main focal points for disseminating information about new technologies in agricultural production and the benefits of these technologies. When farmers join agricultural extension associations, they have a higher chance of receiving technology information and technical assistance. Agricultural extension organizations also connect farmers with technology distributors for direct consultation and installation, thereby increasing the ability of farmers to apply technology in production. Many previous studies have demonstrated this positive relationship in developing countries (Marenya and Barrett, 2011; Grabowski et al., 2016; Lam et al., 2018).
Hypothesis H8: Membership of agricultural extension organizations has positive impact on the adoption of HTV production.
Access to credit: Access to credit influenced a farmer’s decision to adopt improved technology positively in many experimental studies (Fischer, 2016; Floro et al., 2018; Dissanayake et al., 2022). Farmers having access to credit are more likely to adopt new technology normally, partly because new technologies will come with investments and increased costs such as labor and fuel. Receiving financial support will help farmers reduce the burden of initial investment as well as in the process of operating technology. This leads to a higher likelihood of adopting their technology. Ogundari and Bolarinwa (2018) showed that credit access had a positive relationship with technology adoption by farmers but to different degrees and this is the most important factor determining technology adoption by farmers. New technology is often associated with an initial investment and will bring long-term profits to farmers. This economic problem will determine the acceptance of technology by farmers. And better access to credit will help make economic aspects more feasible (Baumgartetz et al., 2012; Simtowe et al., 2019).
Hypothesis H9: Access to credit has positive impact on the adoption of HTV production.
Access to information about new technology: Farmers will carefully study existing technology and the effectiveness of new technologies before deciding whether to adopt them (Kabunga et al., 2012; Burton, 2014; Dissanayake et al., 2022). They do not simply apply but also proceed from the initial step of awareness, then learn about the technology before finally deciding its application in their agricultural production (Di Falco et al., 2018). There is a positive relationship between access to information and technology adoption by farmers in studies by Di Falco and Bulte (2013) and Brown et al. (2019). Access to information can be done in traditional ways such as meeting farmers directly to disseminate techniques at agricultural fairs, agricultural extension associations, farmer associations or projects to strengthen the capacity of farmers. However, accessing information can also be done indirectly through television, promotional programs and especially social networks in today’s modern society.
Hypothesis H10: Access to information about new technology has positive impact on the adoption of HTV production.
The model in this study was estimated using data obtained from primary and secondary data of vegetable producers in Hanoi. First, we mapped the main vegetable production districts of Hanoi and then collected secondary data on vegetable farmers through the District Statistics Office and Agriculture Departments. Information collected includes the number of vegetable growing households, list of households, vegetable growing models, vegetable growing area and the current status of local vegetable production activities. To collect primary data, the study used the following formula to estimate the sample size (Hair et al., 2013):
In which n is the sample size, N is the total number of producers in population, e is accepted errors.
With a total number of households growing vegetables of 10,723 and 5% errors, the calculated sample to ensure reliability was 435. In fact, a stratified random sample of 450 producers was surveyed. The survey is conducted by the authors focusing on districts of producing vegetables such as Dan Phuong, Dong Anh, Ung Hoa, Phuc Tho and Thanh Tri districts. The total surveyed area was more than 5,000 hectares. The area of vegetables applying high technology was nearly 1,260 hectares, reaching nearly 26% of total high technology vegetable area in Hanoi. To select households for interview and research, first research the distribution of 90 households in each of the above districts, and then randomly draw 3 wards with vegetable production in each district. In each ward, we randomly selected 30 households according to the list provided by the local government. The research team approached households in the evening when the head of the household was usually present. At each household, we introduced the objectives of the study and asked for households’ consent to participate. If they agreed, they would check the “agree” box and sign the survey form. Absent households were replaced by a list of 10 backup households also randomly drawn from the list of households. The official investigation was conducted in July and August 2023 in Hanoi.
Based on the empirical model proposed in Section 2, the binary logit model was used to analyze the factors affecting the adoption of high technology in vegetable production in Hanoi. The dependent variable Y had two values, 0 and 1, in which 0 represented the no application of high technology application, and 1 represented the adoption of high technology in vegetable production. The probability function was expressed as:
Pi : probability of high technology adoption of farming households.
Xi : impact factors.
βi : coefficient of marginal impact.
Experimentally, the regression equation was expressed as follows:
The description and measurement of variables is present in Table 1.
Since 2009, Hanoi’s high-tech agricultural application program has been promoted. After more than 14 years of implementation, it has had a positive impact on the growth of Hanoi’s agricultural industry, reaching an average growth rate of 8.5%/year (Department of Agricultural and Rural Development, 2022). High-tech agricultural production achieved an average income more than 2 times higher than the average production value of the entire city, typically: high-quality vegetables reach about 450–500 million VND/ha/year, many models reach 2 billion VND/hectare/year. Currently, the area of high-tech vegetables alone reached 13,655 hectares, accounting for nearly 22.1% of the total cultivated vegetable area. To achieve the above achievements, Hanoi has invested in research, built models, and applied new technical solutions and technologies. Common technologies applied included biotechnology in breeding (in vitro plant tissue culture, vegetable grafting technology), net house technology, drip irrigation technology with pressurization and fertilizer supply system, post-harvest technologies, heat drying technology, film forming technology using automatic or semi-automatic machines in harvesting products. In addition, application of water-saving irrigation technology is also a relatively common model of use in vegetable growing areas with irrigation forms such as drip, local sprinkler and local underground irrigation. The advantage of this system is that it saves 30–60% of water compared to traditional methods, reduces labor, improves crop productivity and quality, and can especially provide fertilizer through a small irrigation system.
Survey results in Table 2 showed that within 450 producers out of a total of 436 respondents, 53.9% were male, and 46.1% were female; the ratio of men and women participating in the interview was quite balanced. The average age of respondents was 42.1, of which the age group from 41 to 50 accounts for the highest proportion (51.2%), this is also the common age group of household heads and the main labor force of farming households in Hanoi. On average, each household participating in the interview had 4.4 people. Over 59% of respondents had graduated from secondary school and the average number of years of schooling was 9.8 years. In the research sample, 34.1% of households applied technology in vegetable production and 65.9% applied traditional methods. The average vegetable growing area per household ranged from 2 to 20 sao (1 sao = 1,000 m2). Up to 62.5% of households had average income, high income households accounted for only 5.7% and low income households accounted for 17.6%.
Table 3 showed the results of analyzing the relationship between independent variables and the probability adoption of vegetable production in study area. We used binary logit regression with maximum likelihood estimation. Firstly, the results of model goodness of fit had significance value of 0.000 < 0.05. Thus, the regression model was consistent. The −2 Log likelihood (−2LL) measured how well the model fitted the data. In this case, the -2LL for the empty model (no independent variables) was 134.32. This significant reduction in -2LL indicated that the independent variables included in the model improved their fit compared to the empty model. Furthermore, the Cox & Snell R2 and Nagelkerke R2 values were greater than 0.5, suggesting that the regression model had a good fit and explained a substantial portion of the variability of the dependent variable.
Logit regression results indicated that there were 8 factors having a significant influence on the application of technology in vegetable production in Hanoi including ATT, PBC, FSIZE, EDU, MEO, ACRE and ACIF, in which ACIF had the strongest impact on farmers’ decisions to apply technology (Table 3).
The logit regression equation is written as follows:
From the results, farmer households’ attitude toward technology was the factor that had the strongest influence on their decision to apply high technology in vegetable production. This result is consistent with the TPB model, which says that the attitude factor is crucial in individual intention-making. This result was also discovered in previous studies on technological application behavior of farmers (Gadenne et al., 2011; Kassie et al., 2015; Negatu and Parikh, 2019). The results show that when attitude increases by 1 point, technology acceptance increases by 13.2%. According to Kristjanson et al. (2015), attitude was farmers’ perception of the application of technology. The more they understand the superiority of technology, the attitude will improve. Study by Elmustapha et al. (2018) in Malawi also found that demonstration field visits help improve farmers’ attitudes toward technology adoption. Dima (2013) found similar results in their study on the determinants of technology adoption by vegetable farmers in Malaysia. Technology demonstrations give farmers the opportunity to evaluate new technology before deciding whether to adopt it. Additionally, field demonstrations promote positive adaptive behavior among farmers as they have the opportunity to interact closely with technology enablers, thereby dispelling any doubts they may have about a particular technology. This result was also consistent with researches by Kristjanson et al. (2015) and Baumgartetz et al. (2012).
Access to information is the second most influential factor in farmers’ decisions to apply technology in production. Applying new technology is a complex process and the more information farmers have, the more confident they are in their decision-making process. In previous studies by Kabunga et al. (2012), Burton (2014), access to information was also proven to be one of the most important factors in the decision-making process to apply technology for farming households. There are many channels that can provide information about technology applications in production to people. Dissanayake et al. (2022) showed that traditional forms of providing information such as agricultural associations, training and disseminating agricultural knowledge directly have a stronger impact than indirect channels such as community media. However, Brown et al. (2019) in the study in Asia pointed out those social networks were the most important channel for disseminating knowledge and information for farmers. Whether provided directly or indirectly, access to information is always an important factor in promoting technology adoption behaviors whether in developed or developing countries (Di Falco and Bulte, 2013; Lam et al., 2018).
Participation in local agricultural extension organizations was the third most influential factor in the application of technology in vegetable growing by farmers. The positive sign of the coefficient was as expected that when farmers join agricultural extension organizations, the level of technology adoption would increase. This result is consistent with other findings from studies by Marenya and Barrett (2011), Grabowski et al. (2016), and Lam et al. (2018). Extension agencies were expected to increase awareness among farmers about techniques in agriculture production. Furthermore, adequate accompaniment of farmers by extension officers and other technical experts is crucial for technology adoption because their presence increases farmers’ confidence in the applied technologies.
Farm size was proposed to have a positive influence on farmers’ technology adoption decisions. As expected, this variable was statistically significant at the 5% significance level. This means that the larger farm, the more likely farmers were to adopt new technology. Although the vegetable growing area in the study area was fairly small, it was common for farmers to have multiple vegetable growing areas in different locations for different crops. This might spread production risks. Other studies also supported this hypothesis such as Lowder et al. (2016), Ojiako et al. (2017) and Noack and Larsen (2019). However, Noack and Larsen (2019) found a negative significant relationship between farm size and farmers’ technology adoption.
Access to credit had also a positive effect on farmers’ decision to apply advanced technology in our analysis. This result was consistent with initial expectation that access to credit had a direct impact on technology adoption. Farmers with access to credit were more likely to adopt new technologies (Fischer, 2016; Floro et al., 2018; Dissanayake et al., 2022). This makes sense because some technologies involve additional production costs, higher demands on labor and resources. Therefore, farmers’ ability to cover these additional costs by taking out loans was an important factor in their decision to adopt the production technology.
The educational attainment of farmers was also recognized as a positive influence on the decision to embrace new technologies. A higher level of education equips farmers with the capability to access and utilize information on the adoption of new technology effectively. This phenomenon can be attributed to the fact that a robust educational background tends to foster broader and more analytical thinking, enabling individuals to assess the advantages presented by innovative technology more comprehensively. The observed relationship between education level and the decision to apply advanced vegetable technology further substantiates this notion. Specifically, it becomes evident that farmers with higher levels of education are inclined to adopt a more expansive and accurate perspective, which enables them to make informed decisions regarding technology adoption. This aligns coherently with a similar study on factors influencing agricultural technology adoption in Asia and Africa such as Ndiritu et al. (2014), Ogada et al. (2014), and Aung et al. (2021).
Finally, perceived behavioral control was the factor with the weakest significant influence on the adoption of technology by farmers. For every 1 point increased in this variable, the likelihood of people accepting the technology increased by 5.6%. Perceived behavioral control is an individual’s perception of how easy or difficult it is to perform a behavior; it represents the degree of control over performing the behavior, not the outcome of the behavior (Ajzen, 1991). This outcome aligned harmoniously with a study on the impact of farmers’ use of information technology by Pardey et al. (2016a,b), Elmustapha et al. (2018), and Negatu and Parikh (2019). It implied a universal trend where farmers are more likely to recognize the advantages of applying technology; they tend to accept higher technology in agricultural production.
This study addressed the gap in the lack of scientific information about factors affecting farmers’ decisions to apply high technology in vegetable production in Hanoi. The study also mixed the psychological factors of the TPB model with the characteristics of the farming household and the supporting factors of the external environment in the model. Since then, logit analysis has found 7 main factors that affect the acceptance of technology in agricultural production by farming households. Understanding the factors affecting technology adoption will play an essential role in promoting the development of high-tech agriculture in Hanoi and Vietnam. From the results and discussions, the following management implications are proposed:
Firstly, it is necessary to improve the attitude of farming households toward applying technology in production. This is the most decisive factor in this study on farmer behavior. To change attitudes, it is important to raise farmers’ awareness about technology adoption. This can be done through a number of specific solutions including (i) taking farmers to visit demonstration sites on applying technology in vegetable growing so that they can see the benefits of applying technology, (ii) disseminate benefits and technological solutions through media and social networks to farmers, (iii) promote the integration of high-tech agricultural production in agricultural extension activities in the community.
Secondly, regulatory agencies also need to strengthen access to technology information and extension services, to increase their awareness and technology application capacity. Information on agricultural technology and related extension services is vital in promoting awareness and application of high technology in agricultural production. Farmers might evaluate existing and new technologies, and then make consideration for application. They do not have to apply immediately, but can learn information carefully before making a final decision. In addition, through extension service, farmers can access information about effective technologies and the benefits of using new technologies through distribution agents. These agents help connect technology suppliers with users, reducing the cost of transmitting information about new technology to many farmers, thereby reducing the gap between them and the technology. Moreover, through the information dissemination network, farmers will be updated with knowledge and skills about specific technological solutions, suitable to the production situation of farming households. Farmers can also share information and learn from each other in specific agricultural innovation situations while engaging in practical production models. From there, they might evaluate the effectiveness of applying new technology in production and, from there, make progress together. Localities need to focus on propaganda to raise public awareness of the importance of technological development in agriculture. At the same time, it is necessary to build a mentality for people who are always ready to mobilize and be creative to find new technological solutions to overcome the difficulties of agriculture with a limited starting point.
Thirdly, strengthen farmers’ capacity in technology in production through training. Training human resources with the proper knowledge, skills, qualities, and attitude is crucial in applying high-tech agriculture. This human resource is the decision factor for the modernization of agriculture, closely linked with new rural construction and creating breakthroughs in agricultural production. This also contributes to promoting rural economic development in the context of international economic integration. To successfully apply new technology to production, it is necessary to increase farmers’ awareness about technology applications and choose methods suitable to family economic conditions and situations. In the future, the suburbs of Hanoi need to strengthen the policy of training human resources through various forms. This includes attracting quality human resources and raising awareness among households, businesses, cooperatives, and cooperative groups about changing and applying new technologies in production. The promotion of human resources related to high-technology should agriculture also focus on improving the qualifications of technical and administrative staff. Vocational training methods need to be renewed, focusing on improving practical capacity, core skills, techniques, and soft skills to adapt and promote in the modern technology in agricultural production.
Last but not least, the state needs to continue to strengthen financial solutions to support farmers to apply technology in production. Options could include increasing farmers’ access to micro credit, providing interest rate subsidies when applying production technology, or supporting part of the initial investment capital when establishing new technologies by farming households.
The raw data supporting the conclusions of this article will be made available by the authors, without undue reservation.
BN: Conceptualization, Formal analysis, Methodology, Writing – original draft, Writing – review & editing. DT: Conceptualization, Data curation, Formal analysis, Writing – original draft.
The author(s) declare that financial support was received for the research, authorship, and/or publication of this article. This study was funded by the National Economics University, Vietnam.
The authors declare that the research was conducted in the absence of any commercial or financial relationships that could be construed as a potential conflict of interest.
All claims expressed in this article are solely those of the authors and do not necessarily represent those of their affiliated organizations, or those of the publisher, the editors and the reviewers. Any product that may be evaluated in this article, or claim that may be made by its manufacturer, is not guaranteed or endorsed by the publisher.
Ajzen, I. (1991). The theory of planned behavior. Organ. Behav. Hum. Decis. Process. 50, 179–211. doi: 10.1016/0749-5978(91)90020-T
Ankuyi, F., and Tham, A. (2022). Drivers of knowledge, attitude, practice, aspiration, and perceptions of sustainable agriculture standard: evidence from certified cocoa farmers in Ghana. Russian J. Agricul. Socio-Econ. Sci. 131, 125–140.
Aung, Y., Khor, L., and Tran, N. (2021). The impact of sustainable aquaculture technologies on the welfare of small-scale fish farming households in Myanmar. Aquac. Econ. Manag. 27, 1–30.
Bassyouni, G., El-Habal, A., and Abdulrazzaq, A. (2022). Impact of farmers’ use of information technology to improve agricultural marketing extension services in Wasit governorate, Iraq. Advan. Soc. Sci. Res. J. 9, 298–314.
Basuki, R., Adiyoga, W., and Gunadi, N. (2019). Impact of improved vegetable farming technology on farmers' livelihoods in Indonesia. Acta Hortic. 809, 101–112.
Baumgartetz, A., Stalker, L., and Floress, K. (2012). Why farmers adopt best management practice in the United States: a meta-analysis of the adoption literature. J. Environ. Manag. 96, 17–25. doi: 10.1016/j.jenvman.2011.10.006
Bokusheva, R., Finger, R., Fischler, M., Berlin, R., Marín, Y., Pérez, F., et al. (2012). Factors determining the adoption and impact of a postharvest storage technology. Food Secur. 4, 279–293. doi: 10.1007/s12571-012-0184-1
Brown, P., Daigneault, A., and Dawson, J. (2019). Age, values, farming objectives, past management decisions, and future intentions in New Zealand agriculture. J. Environ. Manag. 231, 110–120. doi: 10.1016/j.jenvman.2018.10.018
Burton, R. (2014). The influence of farmer demographic characteristics on environmental behaviour: a review. J. Environ. Manag. 135, 19–26. doi: 10.1016/j.jenvman.2013.12.005
Cavatassi, R., Lipper, L., and Narloch, U. (2011). Modern variety adoption and risk management in drought prone areas: insights from the sorghum farmers of eastern Ethiopia. Agric. Econ. 42, 279–292. doi: 10.1111/j.1574-0862.2010.00514.x
Dalton, T. J., Lilja, N. K., Johnson, N., and Howeler, R. (2011). Farmer participatory research and soil conservation in southeast Asian cassava systems. World Dev. 39, 2176–2186. doi: 10.1016/j.worlddev.2011.05.011
Dat, T. T., and Truong, D. D. (2020). Resource management, environment and climate change towards development sustainability in Vietnam from an economic perspective. J. Econ. Dev. 278, 2–11.
Department of Agricultural and Rural Development (2022). Annual report. Agricultural Publisher: Hanoi.
Di Falco, S., and Bulte, E. (2013). The impact of kinship networks on the adoption of risk-mitigating strategies in Ethiopia. World Dev. 43, 100–110. doi: 10.1016/j.worlddev.2012.10.011
Di Falco, S., Feri, F., Pin, P., and Vollenweider, X. (2018). Ties that bind: network redistributive pressure and economic decisions in village economies. J. Dev. Econ. 131, 123–131. doi: 10.1016/j.jdeveco.2017.12.001
Dima, A. (2013). Green purchasing behavior: green products and Ajzen's theory of planned behavior. Econ. Behav. 34, 45–59.
Dissanayake, C., Jayathilake, W., and Wickramasuriya, J. (2022). A review on factors affecting technology adoption in agricultural sector. J. Agricul. Sci. Sri Lanka. 17, 280–296. doi: 10.4038/jas.v17i2.9743
Elmustapha, H., Hoppe, T., and Bressers, H. (2018). Consumer renewable energy technology adoption decision-making; comparing models on perceived attributes and attitudinal constructs in the case of SWH in Lebanon. J. Clean. Prod. 172, 347–357. doi: 10.1016/j.jclepro.2017.10.131
Fischer, K. (2016). Why new crop technology is not scale-neutral – a critique of the expectations for a crop-based African green revolution. Res. Policy 45, 1185–1194. doi: 10.1016/j.respol.2016.03.007
Fisher, M., Abate, T., Alemayehu, Y., and Madulu, R. B. (2015). Drought tolerant maize for farmer adaptation to drought in sub-Saharan Africa: determinants of adoption in eastern and southern Africa. Clim. Chang. 133, 283–299. doi: 10.1007/s10584-015-1459-2
Floro, V. O., Labarta, R. A., Becerra López-Lavalle, L. A., Martinez, J. M., and Ovalle, T. M. (2018). Household determinants of the adoption of improved cassava varieties using DNA fingerprinting to identify varieties in farmer fields: a case study in Colombia. J. Agric. Econ. 69, 518–536. doi: 10.1111/1477-9552.12247
Gadenne, D., Sharma, B., Kerr, D., and Smith, T. (2011). The influence of consumers' environmental beliefs and attitudes on energy saving behaviours. Energy Policy 39, 7684–7694. doi: 10.1016/j.enpol.2011.09.002
Grabowski, P. P., Kerr, J. M., Haggblade, S., and Kabwe, S. (2016). Determinants of adoption and disadoption of minimum tillage by cotton farmers in eastern Zambia. Agriculture. Ecosyst. Environ. 231, 54–67. doi: 10.1016/j.agee.2016.06.027
Hair, J., Hult, G. T., and Ringle, C. (2013). A primer on partial least squares structural equation modeling (PLS-SEM). Sage publications.
Kabunga, N. S., Dubois, T., and Qaim, M. (2012). Heterogeneous information exposure and technology adoption: the case of tissue culture bananas in Kenya. Agric. Econ. 43, 473–486. doi: 10.1111/j.1574-0862.2012.00597.x
Kassie, M., Teklewold, H., Jaleta, M., Marenya, P., and Erenstein, O. (2015). Understanding the adoption of a portfolio of sustainable intensification practices in eastern and southern Africa. Land Use Policy 42, 400–411. doi: 10.1016/j.landusepol.2014.08.016
Katengeza, S. P., Holden, S. T., and Lunduka, R. W. (2018). Adoption of drought tolerant maize varieties under rainfall stress in Malawi. J. Agric. Econ. 70, 1–17.
Khonje, M., Manda, J., Alene, A. D., and Kassie, M. (2015). Analysis of adoption and impacts of improved maize varieties in eastern Zambia. World Dev. 66, 695–706. doi: 10.1016/j.worlddev.2014.09.008
Khonje, M. G., Manda, J., Mkandawire, P., Tufa, A. H., and Alene, A. D. (2018). Adoption and welfare impacts of multiple agricultural technologies: evidence from eastern Zambia. Agric. Econ. 49, 599–609. doi: 10.1111/agec.12445
Kristjanson, P., Okike, I., Tarawali, S., Singh, B. B., and Manyong, V. M. (2015). Farmers’ perceptions of benefits and factors affecting the adoption of improved dualpurpose cowpea in the dry savannas of Nigeria. Agric. Econ. 32, 195–210. doi: 10.1111/j.0169-5150.2005.00338.x
Lam, S., Pham, G., and Nguyen, V. H. (2018). Emerging health risks from agricultural intensification in Southeast Asia: a systematic review. Int. J. Occup. Environ. Health 23, 250–260. doi: 10.1080/10773525.2018.1450923
Lambrecht, I., Vanlauwe, B., Merckx, R., and Maertens, M. (2014). Understanding the process of agricultural technology adoption: mineral fertilizer in eastern DR Congo. World Dev. 59, 132–146. doi: 10.1016/j.worlddev.2014.01.024
Larsen, A. F. (2018). When knowledgable neighbors also share seedlings: diffusion of banana cultivation in Tanzania. Agric. Econ. 17, 1–15.
Le, TN., and, Nguyen, KH. (2019). Impact of removing industrial tariffs under the European–Vietnam free trade agreement: a computable general equilibrium approach. J. Econ. Dev. 21, 2–17.
Le, K. N., and Truong, D. K. (2019). Trade credit use by shrimp farmers in Ca Mau province. J. Econ. Dev. 21, 270–284.
Liu, T., Bruins, R. J. G., and Heberling, M. T. (2018). Factors influencing farmers’ adoption of best management practices: a review and synthesis. Sustain. For. 10, 1–26. doi: 10.3390/su10020432
Lowder, S. K., Skoet, J., and Raney, T. (2016). The number, size, and distribution of farms, smallholder farms, and family farms worldwide. World Dev. 87, 16–29. doi: 10.1016/j.worlddev.2015.10.041
Mai, N. T., and Truong, D. D. (2022). Farming Households’ Perception on Natural Disaster Impacts to Livelihoods and Adaptation Practices: A Case Study of Coastal Provinces in Central Vietnam. International Journal of Sustainable Development and Planning 17, 579–592.
Marenya, P. P., and Barrett, C. B. (2011). Household-level determinants of adoption of improved natural resources management practices among smallholder farmers in western Kenya. Food Policy 32, 515–536. doi: 10.1016/j.foodpol.2006.10.002
Matuschke, I., and Qaim, M. (2001). The impact of social networks on hybrid seed adoption in India. Agric. Econ. 40, 493–505. doi: 10.1111/j.1574-0862.2009.00393.x
Meijer, S. S., Catacutan, D., Ajayi, O. C., Sileshi, G. W., and Nieuwenhuis, M. (2015). The role of knowledge, attitudes and perceptions in the uptake of agricultural and agroforestry innovations among smallholder farmers in sub-Saharan Africa. Int. J. Agric. Sustain. 13, 40–54. doi: 10.1080/14735903.2014.912493
Moser, C. M., and Barrett, C. B. (2016). The complex dynamics of smallholder technology adoption: the case of SRI in Madagascar. Agric. Econ. 35, 373–388.
Mottaleb, K. A., Krupnik, T. J., and Erenstein, O. (2016). Factors associated with smallscale agricultural machinery adoption in Bangladesh: census findings. J. Rural. Stud. 46, 155–168. doi: 10.1016/j.jrurstud.2016.06.012
Mukasa, A. N. (2018). Technology adoption and risk exposure among smallholder farmers: panel data evidence from Tanzania and Uganda. World Dev. 105, 299–309. doi: 10.1016/j.worlddev.2017.12.006
Mulema, A., Cramer, L., and Huyer, S. (2022). Stakeholder engagement in gender and climate change policy processes: lessons from the climate change, agriculture, and food security research program. Front. Sustain. Food Syst. 6:3389. doi: 10.3389/fsufs.2022.862654
Muthumanickam, D., Chenniappan, P., and Ramalingam, K. (2022). Smart farming: internet of things (IOT)-based sustainable agriculture. Agriculture 12:1745. doi: 10.3390/agriculture12101745
Ndiritu, S. W., Kassie, M., and Shiferaw, B. (2014). Are there systematic gender differences in the adoption of sustainable agricultural intensification practices? Evidence from Kenya. Food Policy 49, 117–127. doi: 10.1016/j.foodpol.2014.06.010
Negatu, W., and Parikh, A. (2019). The impact of perception and other factors on the adoption of agricultural technology in the Moret and Jiru Woreda (district) of Ethiopia. Agric. Econ. 21, 205–216. doi: 10.1111/j.1574-0862.1999.tb00594.x
Njuki, J. M., Mapila, M. T., Zingore, S., and Delve, R. (2018). The dynamics of social capital in influencing use of soil management options in the Chinyanja triangle of southern Africa. Ecol. Soc. 13, 22–39.
Noack, F., and Larsen, A. (2019). The contrasting effects of farm size on farm incomes and food production. Environ. Res. Lett. 14, 10–24. doi: 10.1088/1748-9326/ab2dbf
Ntshangase, N. L., Muroyiwa, B., and Sibanda, M. (2018). Farmers’ perceptions and factors influencing the adoption of no-till conservation agriculture by smallscale farmers in Zashuke, KwaZulu-Natal province. Sustainability (Switzerland) 10, 1–16.
Ogada, M. J., Mwabu, G., and Muchai, D. (2014). Farm technology adoption in Kenya: a simultaneous estimation of inorganic fertilizer and improved maize variety adoption decisions. Agric. Food Econ. 2, 1–28. doi: 10.1186/s40100-014-0012-3
Ogundari, K., and Bolarinwa, O. D. (2018). Impact of agricultural innovation adoption: a meta-analysis. Aust. J. Agric. Resour. Econ. 62, 217–236. doi: 10.1111/1467-8489.12247
Ojiako, I. A., Manyong, V. M., and Ikpi, A. E. (2017). Determinants of rural farmers’ improved soybean adoption decisions in northern Nigeria. J. Food, Agricul. Environ. 5, 215–223.
Orr, A., Kambombo, B., Roth, C., Harris, D., and Doyle, V. (2015). Adoption of integrated food-energy systems: improved cookstoves and pigeonpea in southern Malawi. Exp. Agric. 51, 191–209. doi: 10.1017/S0014479714000222
Pardey, P. G., Andrade, R. S., Hurley, T. M., Rao, X., and Liebenberg, F. G. (2016a). Returns to food and agricultural R & D investments in sub-Saharan Africa, 1975–2014. Food Policy 65, 1–8. doi: 10.1016/j.foodpol.2016.09.009
Pardey, P. G., Chan-Kang, C., Dehmer, S. P., and Beddow, J. M. (2016b). Agricultural R&D is on the move. Nature 537, 301–303. doi: 10.1038/537301a
Prager, K., and Posthumus, H. (2020). “Socio-economic factors influencing farmers’ adoption of soil conservation practices in Europe” in Human dimensions of soil and water conservation, number January chapter 12. ed. T. L. Napier (New York: Nova Science Publishers Inc), 203–223.
Rapsomanikis, G. (2015). The economic lives of smallholder farmers (technical report). Rome: Food and Agriculture Organization of the United Nations.
Sharma, R. (2015). Access to modern agricultural technologies and farmer household welfare: evidence from India. Int. J. Asian Stud. 6, 19–43. doi: 10.1177/0976399614563222
Shiferaw, B., Kebede, T., Kassie, M., and Fisher, M. (2015). Market imperfections, access to information and technology adoption in Uganda: challenges of overcoming multiple constraints. Agric. Econ. 46, 475–488. doi: 10.1111/agec.12175
Shiferaw, B. A., Kebede, T. A., and You, L. (2018). Technology adoption under seed access constraints and the economic impacts of improved pigeonpea varieties in Tanzania. Agric. Econ. 39, 309–323. doi: 10.1111/j.1574-0862.2008.00335.x
Simtowe, F., Zeller, M., and Diagne, A. (2019). The impact of credit constraints on the adoption of hybrid maize in Malawi. Rev. Agricul. Environ. Stud. 90, 5–22.
Sitko, N. J., Chamberlin, J., and Hichaambwa, M. (2014). Does smallholder land titling facilitate agricultural growth? An analysis of the determinants and effects of smallholder land titling in Zambia. World Dev. 64, 791–802. doi: 10.1016/j.worlddev.2014.07.014
Sodjinou, E., Glin, L., Nicolay, G., Tovignan, S., and Hinvi, J. (2016). Socioeconomic determinants of organic cotton adoption in Benin, West Africa. Agric. Food Econ. 3, 1–22.
Teklewold, H., Kassie, M., and Shiferaw, B. (2013). Adoption of multiple sustainable agricultural practices in rural Ethiopia. J. Agric. Econ. 64, 597–623. doi: 10.1111/1477-9552.12011
Teshome, A., de Graaff, J., and Kassie, M. (2016). Household-level determinants of soil and water conservation adoption phases: evidence from North-Western Ethiopian highlands. Environ. Manag. 57, 620–636. doi: 10.1007/s00267-015-0635-5
Keywords: binary logit regression, high-technology agriculture, social inclusion, vegetable production, theory of planned behavior
Citation: Nhuong BH and Truong DD (2024) Factors affecting the adoption of high technology in vegetable production in Hanoi, Vietnam. Front. Sustain. Food Syst. 8:1345598. doi: 10.3389/fsufs.2024.1345598
Received: 28 November 2023; Accepted: 13 May 2024;
Published: 04 June 2024.
Edited by:
Tapan Kumar Nath, University of Nottingham Malaysia Campus, MalaysiaReviewed by:
Muhammad Rashed Al Mamun, Kyushu University, JapanCopyright © 2024 Nhuong and Truong. This is an open-access article distributed under the terms of the Creative Commons Attribution License (CC BY). The use, distribution or reproduction in other forums is permitted, provided the original author(s) and the copyright owner(s) are credited and that the original publication in this journal is cited, in accordance with accepted academic practice. No use, distribution or reproduction is permitted which does not comply with these terms.
*Correspondence: Dinh Duc Truong, truongdd@neu.edu.vn
Disclaimer: All claims expressed in this article are solely those of the authors and do not necessarily represent those of their affiliated organizations, or those of the publisher, the editors and the reviewers. Any product that may be evaluated in this article or claim that may be made by its manufacturer is not guaranteed or endorsed by the publisher.
Research integrity at Frontiers
Learn more about the work of our research integrity team to safeguard the quality of each article we publish.