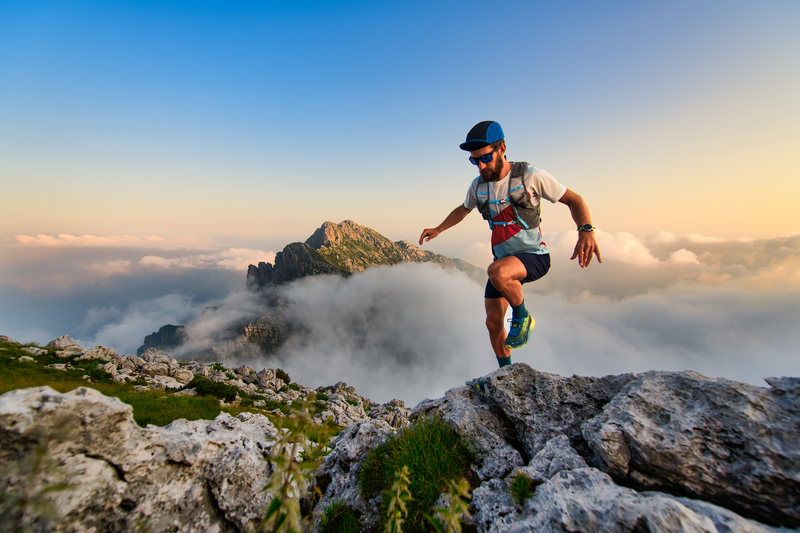
94% of researchers rate our articles as excellent or good
Learn more about the work of our research integrity team to safeguard the quality of each article we publish.
Find out more
ORIGINAL RESEARCH article
Front. Sustain. Food Syst. , 11 March 2024
Sec. Agricultural and Food Economics
Volume 8 - 2024 | https://doi.org/10.3389/fsufs.2024.1337637
The infusion of digital technologies imparts impetus to the development of agricultural circular economy. Study focuses on the nexus of digital rural development, agricultural circular economy growth, employing entropy, Critic, and TOPSIS models to calculate the evolution of agricultural circular economy from 2011 to 2021. Furthermore, the temporal and spatial dynamics of agricultural circular economy development are explored. In addition, spatial Durbin models and threshold models are utilized to explore the spatial spillover effects of digital rural development on agricultural circular economy, and the threshold characteristics of agricultural circular economy with varying levels of digital rural development. The research findings reveal that arable land area, forest coverage, and mechanization play pivotal roles in shaping the trajectory of rural circular economy development. The synthetical index of rural circular economy development shows a consistent yearly increment, yet pronounced regional disparities exist, reflecting an olive-shaped distribution with dual cores in Heilongjiang and Chongqing and a more prominent central. The implementation of digital rural not only elevates the local agricultural circular economy, but also demonstrates conspicuous spatial spillover effects on neighboring regions. The relationship between digital rural development and agricultural circular economy growth is characterized by a singular threshold effect. Pre- and post-threshold, the promotive impact of digital rural development rises from 0.048 to 0.058. Building upon these findings, policy recommendations are formulated to guide future endeavors in this domain.
Agriculture, at the heart of these rural concerns, provides essential resources such as food and agricultural products to humanity (Duque-Acevedo et al., 2020), exerting a substantial influence on both farmer incomes and the overall welfare of the nation (Lange et al., 2018). The significant water demands of agricultural activities underscore the pivotal role that water resources play in determining the extent of sustainable agricultural development (Winter et al., 2017). Simultaneously, agricultural production and operations exert varying degrees of impact on the environment, climate, and related domains (Maurício et al., 2022).
Over time, China has witnessed rapid growth in its agricultural economy, leading to a continuous increase in its aggregate output. However, the traditional extensive development model has also brought forth a host of latent hazards, escalating contradictions between population and resources, as well as the underutilization of resources. Consequently, ecological and environmental pressures have progressively intensified. Moreover, the trajectory of agricultural advancement in China is confronted with an array of challenges, including declining rural populations, rural land transfers, and deficiencies in governance systems (Liu, 2022), all of which impede the progression of Chinese-style modernized agriculture. Constrained by multifaceted factors, the evolution of Chinese agriculture necessitates the identification of a trajectory congruent with ecological civilization.
The concept of circular economy has emanated from multiple sources, with its initial formulation dating back to 1998 (Zhu, 1998). Subsequent refinements to the concept were introduced by Kumar et al. (2021) and Ronaghi (2022). Diverging from the conventional economic approach of resource depletion to fulfill consumption demands (Dantas et al., 2021), the essence of which lies in minimizing resource consumption to achieve sustainable development objectives (Cantzler et al., 2020).
Circular economy represents an advanced developmental paradigm that, in principle, is applicable to all natural resources (Schroeder et al., 2019), exerting a profound influence on both production and lifestyle domains. Synthesizing the tenets of circularity and economic advancement, this model places emphasis on the cyclic utilization of resources. It not only ensures the quality and safety of agricultural products but also mitigates environmental pollution. As such, the circular economy is considered a pivotal pathway toward the realization of modernized agriculture (Guo et al., 2011).
Given its progressive principles and immense developmental potential, circular economy has received extensive attention from governments, experts, and scholars, owing to its capacity to address challenges such as resource scarcity (Kiselev et al., 2019; Velasco-Muñoz et al., 2021). Various nations have displayed a heightened focus on the circular economy, utilizing its principles to tackle ecological and environmental issues. Notably, a significant number of countries globally, a total of 196, have acceded to the Paris Agreement, aimed at combatting global warming. Advanced nations like Switzerland have integrated the circular economy into waste management strategies (Acerbi and Taisch, 2020; Batlles-delaFuente et al., 2022), while China has propagated the model of ecological industrial parks.
The recognition of the significance of circular economy development has extended beyond governmental domains, as various industries have embraced the concept and propelled its implementation. The convergence of circular economy with other technologies has become instrumental in fostering rapid circular economy growth, exemplified by instances like blockchain-based circular economy (Davide et al., 2023), artificial intelligence, industrial applications (Lieder and Rashid, 2016), energy sectors (Kiselev et al., 2019; Saidani et al., 2019), agriculture (Thiru et al., 2023), among others.
China has embarked on a comprehensive journey toward high-quality development, vigorously promoting the construction of digital rural areas as a potent lever for realizing the development of agriculture. Digital rural development embodies traits of efficiency, intelligence, and convenience, not only contributing to socioeconomic progress but also intricately intertwining with agricultural pursuits. It has evolved into a pivotal pillar supporting the edifice of agricultural circular economy. In 2022, the average grade of cultivated land quality was 4.76, the availability of agricultural water resources increased by 0.7% compared with 2021, the consumption of chemical fertilizer decreased by 2.16%, the recovery rate of waste agricultural mulching film reached 60.6%, and the comprehensive utilization rate of straw industry was about 90%. China’s digital rural construction time is short, the urban–rural gap still exists. The Internet, 5G did not achieve full coverage, cannot meet the big data of new technologies such as the support of green agriculture, in addition, the rural digital professional and technical personnel and agricultural development does not match, which failed to apply digital technology to agricultural production, it lead to insufficient agricultural circular economy development. Therefore, it is urgent to develop digital rural technology. Confronting the challenges of agricultural development, rationally allocating agricultural resources within the framework of digital rural development becomes paramount, ultimately propelling the realization of agricultural circular economy.
Given this context, the pertinent question arises: what precisely is the impact of digital rural development on the progression of the agricultural circular economy? Amid the backdrop of heightened governmental attention to rural issues across different regions, standardized performance assessment criteria and shared goals for agricultural modernization have heightened the interregional spatial connectivity. Consequently, this sets the stage for investigating the distinctive characteristics through which digital rural development, observed in spatial view, propels the agricultural circular economy. Moreover, does digital rural development exhibit heterogeneity across different stages? Addressing these queries accurately holds the core to evaluating the influence of digital rural development on the agricultural circular economy, bearing significant implications for the construction of the facilitation of modernized agricultural development.
In the present landscape, research concerning agricultural circular economy has evolved from qualitative approaches to quantitative methodologies. Some scholars have measured the efficiency (Guo et al., 2023). The selection of evaluation indicators is pivotal for accurate measurement. Recognizing that a single indicator is insufficient to holistically capture the essence of agricultural circular economy, a multidimensional approach encompassing financial, societal, and environmental aspects has been adopted (Musyarofah et al., 2023). Yang has employed dimensions such as social development, resource input, and resource circulation to gauge the capacity of agricultural circular economy (Hui et al., 2014). In 2007, the NDRC introduced an evaluation indicator system for circular economy development (Geng et al., 2012), from which Yuan and others selected macro-level indicators for assessment (Hu et al., 2018).
While research on circular economy has experienced incremental growth, investigations into the influence of digital technology on circular economy remain relatively limited (Wynn and Jones, 2022). Existing studies have demonstrated that digital technology addresses various environmental concerns such as solid waste and electronic waste (Francesca et al., 2020; Gagan et al., 2020). Moreover, digital technology contributes to energy conservation, efficiency improvements in production and manufacturing, and cost reduction (Vincent et al., 2021; Zhao et al., 2023), effectively fostering circular economy and sustainable development (Ali et al., 2023; Li et al., 2023). Geographically, scholars have examined European countries (Moreno et al., 2019; García-Muiña et al., 2021; Luciano et al., 2021), while others have shifted their focus toward emerging economies (Bag et al., 2020; Khan et al., 2021; Abdul-Hamid et al., 2023).
The existing body of research in the field is relatively abundant; however, many studies predominantly employ quantitative methodologies for analysis, with limited application of econometric models to quantify the circular economy (Luo et al., 2022; Laura, 2023; Li, 2023). Some limitations persist in the extant literature: Firstly, regarding research scope, the majority of papers focus on the nexus between digital technology and the circular economy (Khan et al., 2022), with fewer delving into agricultural and rural development, despite their pivotal role within the context of Chinese-style modernization. Secondly, concerning research methodologies, many rely on conventional statistical models r case analysis to gauge the impact of digital technology, often overlooking the potential nonlinear relationships inherent between these two variables (Liu et al., 2022). Thirdly, in terms of research perspectives, empirical analyses predominantly approach the impact of digital technology from a non-spatial angle (Schöggl et al., 2023), inadvertently neglecting the inherent fluidity of the circular economy, which poses challenges in elucidating the intricate relationship between them.
The marginal contributions are threefold: Firstly, in the research perspective, it centers its focus on the agricultural industry and new rural construction, investigating the effect of digital rural on agricultural circular economy, which provide a new application direction for the digital rural construction. Secondly, from the research method, it employs spatial econometric models to examine the spatial effects of digital rural development on the advancement of the agricultural circular economy. Meanwhile, it constructs a panel threshold model to verify the nonlinear impact of digital rural development on the agricultural circular economy at varying levels of digital rural development. Lastly, from the perspective of research significance, under the background of “digital China,” it has important significance to explore the influence of digital rural construction on agricultural circular economy, to optimize the construction of smart countryside, build a beautiful and livable countryside, and promote the harmonious development of man and nature.
During the 14th Five-Year Plan, China embarked upon a novel journey toward agricultural modernization, delving into pathways and methodologies for the resource utilization and recycling of organic waste, waste gasses, waste materials, sewage, and the like. Thus, for elucidate the essence of agricultural circular economy, a framework is established according to the 4R (reduce, reuse, recycle, recover).
Given the discrepancies between the 2007 circular economy indicators and China’s prevailing circumstances, a redesign of indicators aligned with current developmental contexts is warranted (Pan et al., 2016; Hu et al., 2023; Chen et al., 2024).
Consequently, grounded in the logical structure of agricultural circular economy development measurement, and while ensuring data availability, an assessment framework for evaluating agricultural circular economy development indicators was formulated. This framework encompasses four dimensions: Agricultural economic, social development, diminished resource input, resource recycling, and resource-environment security. In total, it encompasses 18 indicators (Table 1).
Which is used to evaluate the current situation and potential of agricultural circular economy development. Agricultural GDP and total output value of agriculture, forestry, animal husbandry, and fishery reflect the total scale and results of agricultural production. The higher the indicator, the higher the agricultural economic benefits. Agricultural income is the main sources of income for rural residents, which reflects the level of agricultural economic prosperity. The unit livestock and poultry product rate is a manifestation of the commercialization of livestock and poultry products, and animal husbandry is a concentrated manifestation of regional agricultural economic characteristics. Agricultural machinery is an important component of agricultural economy and a driving force for agricultural economic growth. The improvement of this indicator means an improvement in agricultural efficiency and benefits. Grain production is closely related to people’s livelihoods, food security and stability are the key to controlling the overall economic development. The dimension is assessed through indicators including unit area agricultural GDP output, rural residents’ income, agricultural machinery power, unit output of livestock and poultry products, per capita grain production et al.
Which reflects the current situation of the input end of agricultural circular economy. Pesticide, films, and diesel are the main sources of agricultural carbon emissions. Although these resources are helpful for agricultural production, the more investment, the greater the pollution generated, which goes against the original intention of agricultural circular economy. Power supply services effectively ensure the development of modern agriculture. The higher the electricity consumption in rural areas, the more unfavorable it is for environmental protection. Effective irrigation coefficient refers to the proportion of effective irrigation per unit of cultivated land, the higher the index, the higher the effective level of management; The dimension of diminished resource input is evaluated through indicators encompassing fertilizer application intensity, pesticide application level, plastic film usage level, agricultural diesel fuel consumption, rural electricity usage intensity, and effective irrigation coefficient.
Which reflects the degree of resource recycling of agricultural circular economy. The multiple cropping index represent the degree of repeated utilization of cultivated land resources. The higher the multiple cropping index, the higher the utilization rate of land resources. The effective utilization coefficient of fertilizers is one of the important indicators of agricultural production, representing the degree of fertilizer resource utilization. The higher the indicator, the stronger the technical and economic effects. The effective utilization rate of machinery refers to the total output value of the planting industry at a unit level of agricultural mechanization, representing the return on investment in agricultural machinery. The higher the indicator, the greater the return on investment. The dimension of resource recycling is gauged through indicators such as cropping index, fertilizer utilization efficiency, machinery utilization efficiency.
Which evaluates the strength of the external environment support in the development process of agricultural circular economy, which belongs to the environmental research index. Forests are the key to the control of soil erosion, if the index decreases, it will lead to crop production. Cultivated land is the lifeblood of grain production. Holding the lifeblood of agricultural economic development, can bring sustainable economic benefits to farmers. Agricultural production is greatly affected by meteorological and climatic conditions, the situation that agriculture depends on the weather has not been fundamentally changed. The risk still exists. These indicators have affected the agricultural circular economy. The dimension of resource-environment security is quantified using indicators including forest coverage rate, arable land area, and farmland disaster incidence rate.
Currently, cutting-edge research on CRITIC include CRITIC-EDAS et al., the use of the CRITIC-EDAS was first proposed by Mallick et al. (2023). CRITIC-EDAS is more comprehensive and advantageous, which is mainly used for the comparison and evaluation of multiple schemes, mainly for attribute conflict problems. Moreover, this paper evaluates the level of the agricultural circular economy over the years, rather than choosing the best alternative. Therefore, it is more realistic to adopt the Critic-Entropy combination empowerment method, Critic-Entropy amalgamates the advantages of Critic, which eradicates overlap between indicators’ information and entropy, which handles inter-indicator correlation (Diakoulaki et al., 1995; Abdel-Basset and Mohamed, 2020). The entropy method determines weights according as the degree of dispersion among indexes (Chang, 2019), addressing Critic’s limitations. Consequently, the Critic-Entropy is used to compute the weights of indicators.
I. Assuming that there are m study samples, n indicators, the original matrix of m * n is formed as:
II. Perform dimensionless processing:
Where, and are the maximum, minimum values, and is normalized data.
III. Calculating information carrying capacity.
is the variability, which use Eqs. (4) and (5) to calculate. is the conflict, Eq. (6) is a mathematical expression of the correlation coefficient, is the correlation coefficient of each indicator, and is the amount of information, which use Eq. (7) to calculate.
IV. Weighting calculations
The following formula was used to measure the weights of the indicators , as shown in Eq. (9).
I. Matrix construction. The same original matrix constructed with Critic data, see Eq. (1).
II. Dimensionless processing. The same as Critic data normalization processing, see Eqs. (2) and (3).
III. Calculation of indicator information entropy , as shown in Eq. (10).
IV. Calculation of the coefficient of variation , as shown in Eq. (11).
V. Calculation of weights , as shown in Eq. (12).
Calculation of composite weights for indicator j, as shown in Eq. (13).
In this paper, we assume that the assignments have the same importance and take = 0.5.
The TOPSIS model ranks the sample units according to their proximity to the idealized target. The steps as follows:
I. Constructing a weighting matrix , as shown in Eq. (14).
II. Determination of positive ideals and negative ideal , as shown in Eqs. (15) and (16).
III. Calculate the distance from the sample cell to the positive and negative ideal solution , , as shown in Eqs. (17) and (18).
IV. The closeness coefficient of the sample cell to the ideal solution , as shown in Eq. (19).
The calculation of the level of agricultural circular economy is shown in Figure 1.
To guarantee the continuity and availability of sample units, the study covers the period from 2011 to 2021, with data sourced from “China Rural Statistical Yearbook,” as well as yearbooks from various provinces.
Calculation was performed using both the Critic and entropy methods, yielding weight coefficients for each indicator (Table 2). Larger values denote greater weights, signifying the indicators’ relative importance.
Three representative years, namely 2011, 2016, and 2021, were selected. Over the inspection period, which exhibited a steady upward trend, approximately 2.19%. By 2021, the index reached its peak at 0.385. On a regional basis, during the years 2011–2021, the eastern demonstrated the highest mean value, the western displayed the lowest, indicating an uneven development. Employing the method of average standard classification, the comprehensive development index was categorized as follows: 0–0.296 denoting low level, 0.297–0.358 representing relatively low level, 0.359–0.419 indicating moderate level, 0.420–0.481 signifying relatively high level, and 0.481–1 corresponding to high level. Utilizing Stata software, visualization of China’s agricultural circular economy development level was achieved, facilitating a more intuitive presentation of temporal and spatial evolution trends. As shown in Figure 2.
The number of provinces categorized as having low levels gradually diminished, while those categorized as having high levels increased. The overall level continuously improved. In 2011, there were no provinces at high or relatively high levels. In 2016, Heilongjiang province was at a relatively high level, and by 2021, Chongqing and Heilongjiang provinces were both at high levels. Provinces categorized as having low levels decreased from 10 in 2011 to 6 in 2016, and subsequently to 2 in 2021. Provinces categorized as having relatively low levels reduced from 18 in 2011 to 11 in 2016, further declining to 7 in 2021. Provinces at relatively high levels or above increased from none in 2011 to 1 in 2016, and then to 7 in 2021.
The new economic geography theory asserts that economic activities are inherently constrained by geographical space, and the agricultural circular economy is also influenced by spatial factors. Spatial correlation reflects the spatial relationships among various variables and is a prerequisite for employing spatial models. Correlation is generally separate into two components: global and local spatial autocorrelation. The former can be assessed using Moran’s I, Geary’s C tests. The computation formula for Moran’s I:
Where, is spatial weight matrix, x is specific value, n is amount of samples, and is the mean value. Geary’s C:
Where, Geary’s C value takes the range of [0,2].
Global spatial autocorrelation tests the spatial relationship between different samples, while local spatial autocorrelation evaluates the spatial clustering phenomenon of a particular distribution. The formula was calculated as:
Eq. (22), a positive value of the local Moran’s index indicates the high values of the sample are adjoin to the high values, and vice versa.
The agricultural circular economy, being a form of ecological economy, possesses spatial characteristics inherent to ecological economics. It demands inter-regional sharing and collaboration of agricultural resources, thereby establishing a dependency of local agricultural circular economies on their neighboring regions. With the advancement of economic and social development, the mobility of agricultural assets has gradually increased, leading to a closer and more coordinated development of inter-regional agricultural circular economies. Thus, to incorporate spatial factors into the research problem, spatial econometric models are employed on the basis of Ordinary Least Squares (OLS) regression models.
Commonly utilized spatial econometric models encompass SDM, SEM and SAR. Each model presents its own strengths and limitations, with the possibility of mutual transformation under specific conditions. The formulation of the models is as follows:
Models (23), (24), and (25) represent SDM, SEM, and SAR, respectively. is the agricultural circular economy, is the core explanatory variable digital countryside construction; is the control variables, mainly including rural finance, rural population density, rural development level, and agricultural openness to the outside world. is the random disturbance term, i,t denotes province and city and year respectively, and is the estimated coefficient. is the elasticity coefficient of the explanatory variables, and is the elasticity coefficient of the spatial lagged term. denotes the weight matrix, denotes the spatial autocorrelation coefficient, is the spatial autocorrelation error term.
The operation is performed using partial differential matrices to obtain the matrix of partial derivatives of the expected value of the dependent variable. The computational procedure is as follows:
Eq. (26) reflects the meaning of the spatial dubin model parameters given in the form of a partial derivative, which is the conversion form of the SDM model. Organize to get:
Eq. (27) is the collated expression form of Eq. (26). Total, direct, and indirect effects, respectively:
Eqs. (28–30) is the decomposed total effect, direct effect and indirect effect.
In addition, rural surface pollution is serious, and the degree of agricultural pollution varies greatly between different provinces. To validate the nonlinear threshold effect of digital village development on agricultural circular economy under different rural surface pollution. According to the practice of Wu et al. (2021), the threshold model is constructed based on the basis of the SDM, single, double and triple threshold models are constructed as follows:
In Eqs. (31), (32), and (33), R is the threshold dependent variable rural surface pollution, and is the indicative function when, , , and are the first, second, and third thresholds to be estimated, respectively. z is the threshold variable.
Dependent variable: agricultural circular economy (Cireco). The results are obtained from the above part of the calculation.
Independent variable: digital rural construction (Drc). Expressed using rural broadband access users/rural population.
Control variable. Farming-related loans (Loan). Expressed using per capita farm-related loans. Population density (Pop). Expressed using rural population/cultivated land area. Engel’s coefficient (Eng). Expressed as Engel’s coefficient for rural households. Transaction situation (Trade). Expressed as trade in agricultural products/gross output value.
In this study, a sample of 30 provinces in China for the years 2011 to 2021 was employed. Data were sourced from publications such as the “Rural Statistical Yearbook” et al. For some instances of missing values, the mean imputation method was utilized to complete the dataset (Table 3).
To prevent potential issues of spurious regression in subsequent analysis, the LLC and IPS tests were conducted (Table 4). The results indicate that all variables are stationary.
To examine the spatial effects of agricultural circular economy and assess the feasibility of employing spatial econometric models, the global spatial autocorrelation of agricultural circular economy was calculated using Eqs. (20) and (21), as presented in Table 5.
From the Table 5, it is observed that the Geary’s C coefficient of agricultural circular economy falls within the range of [0, 1], while Moran’s I coefficient is positive. This indicates a significant positive spatial autocorrelation and agglomeration feature in the agricultural circular economy, implying notable spatial spillover effects from neighboring regions to the local area. Over time, Geary’s C coefficient of agricultural circular economy exhibits an upward trend after fluctuations, while Moran’s I coefficient demonstrates a declining trend after fluctuations. This suggests a gradual reduction in the degree of agglomeration within the agricultural circular economy.
Further examination of local spatial agglomeration of observed values, i.e., identifying regions exhibiting spatial clustering phenomena, was conducted for representative years 2011, 2016, and 2021. The results are depicted in Figure 3.
Figure 3 illustrates the spatial correlation among regions. Provinces in the first quadrant exhibit higher levels of agricultural circular economy both locally and in their surrounding areas, forming a High-High (H-H) agglomeration pattern. Provinces in the second quadrant show lower local levels but higher levels in their neighboring areas, forming a Low-High (L-H) agglomeration pattern. Provinces in the third quadrant have both lower local and neighboring levels, forming a Low-Low (L-L) agglomeration pattern. Provinces in the fourth quadrant possess higher local levels but lower levels in their surrounding areas, forming a High-Low (H-L) agglomeration pattern. The results indicate that in 2011, there were 28 provinces forming H-H and L-L agglomeration patterns, accounting for 93.3% of the total; this decreased to 24 provinces in 2016 and 2021, representing approximately 80%, primarily in H-H and L-L patterns, though with a reduction in proportion. In 2011, H-H agglomeration was mainly concentrated in central and western provinces such as Fujian, Heilongjiang and Guangxi, while L-L agglomeration was more prominent in provinces like Shanxi, Hebei, and Inner Mongolia. The spatial distribution remained remarkably similar over the years. In 2021, Inner Mongolia move from L-L to H-L, while Jilin, Hebei transitioned from H-H and L-L to H-L zones, respectively.
The aforementioned results highlight significant global and local positive spatial correlations within the agricultural circular economy, aligning with the prerequisites for constructing spatial econometric models. Consequently, the subsequent analysis is undertaken.
The spatial econometric model degradation tests, including the LR, the Wald test, were conducted to examine whether the SDM model could degrade into either the SAR or SEM (Table 6). SDM was more suitable.
When the spatial lag coefficient is significantly nonzero, the results obtained from the SDM model will exhibit bias. To accurately assess the influence, the spatial spillover effects include direct, indirect, total effects using partial differentiation methods (Table 7). The estimated coefficient value of ρ in the SDM model was 0.296, indicating a significant positive spatial relationship in the agricultural circular economy. There is a certain level of correlation between the local and neighboring agricultural circular economies, implying the presence of substantial direct and spillover effects.
Examining the direct effects, the estimated coefficient of digital rural development was 0.022 t, indicating that digital rural development effectively promotes local agricultural circular economy development. Regarding the indirect effects, the spatial spillover effect was 0.043.
To guarantee the dependability of the spatial econometric results, this study take robust tests: (1) Changing weight matrices - the spatial weight matrix was replaced with economic weight matrix and adjacency weight matrix; (2) Replacing core variables - the results of agricultural circular economy obtained by replacing the dependent variable with the one obtained from entropy-based weighted estimation. The outcomes are presented in Table 8. Comparing with Table 7, the estimated coefficient of digital rural development and its significance level remain largely consistent.
As demonstrated earlier, digital rural development significantly affects the local and neighboring agricultural circular economy. However, due to substantial variations in the degree of digital rural and economic growth among different provinces, a panel threshold model is employed to further investigate whether there exists a turning point in digital rural development.
Taking the digital rural as threshold variable, the existence of a threshold is examined before estimating the threshold. Firstly, a single threshold effect is tested. If it is found to exist, subsequent tests for double and multiple thresholds are performed (see Table 9). The results indicate that only the single threshold test is confirmed. The F-value for the double threshold effect is 3.20, p-value is 0.833, indicating the double threshold effect is not established. Consequently, the influence of digital rural development exhibits a single threshold effect.
The results of the further estimation of the threshold (Table 10). The threshold estimate is −2.7669.
The estimated results of the threshold model are presented in Table 11. The findings reveal that the impact of digital rural development on the agricultural circular economy is divided into two intervals. When the natural logarithm of digital rural development is less than the value of −2.7669, the effect of digital rural development on the agricultural circular economy is significantly positive, with an elasticity coefficient of 0.048. As the level of digital rural development surpasses the threshold value, the enhancing effect of digital rural development on the agricultural circular economy intensifies, with an elasticity coefficient of 0.058. On the one hand, the digital rural infrastructure construction is incomplete; on the other hand, talent is insufficient. When the digital rural construction is within the first threshold range, which can not well cover agricultural production and operation, Therefore, it has a limited effect, especially in Inner Mongolia and other farming and animal pastoral areas, the key to the development of agricultural circular economy lies in accelerating the transformation of digital technology to traditional agriculture in various fields and links. When the level of digital rural construction continues to improve, agricultural industry information technology standards are gradually improved. Establishing a circulation system of agricultural big data collection, analysis and application, deeply integrating digital technology with resource utilization rate, labor utilization rate and land yield rate, digital technology will be fully utilized. Therefore, the promoting effect has been enhanced. This is in line with the actual situation, in Zhejiang, Fujian, Chongqing and other provinces beyond the threshold value, the development level of agricultural circular economy is generally high.
(1) Arable land area, forest coverage, and efficient machinery utilization emerge as pivotal factors. Arable land constitutes the fundamental basis of agricultural production and a key element in agricultural modernization. Vegetation resources possess inherent regenerative capabilities, bolstering agricultural ecological potential. Mechanization plays a crucial role in enhancing agricultural production conditions and augmenting both economic and ecological benefits.
(2) The developmental trajectory of agricultural circular economy displays a steady ascent. The province of Heilongjiang consistently maintains an absolute advantage in agricultural circular economy development, while Chongqing exhibits a latecomer surge. This ultimately configures a spatial structure centered around Heilongjiang and Chongqing as primary cores, with secondary cores encompassing Guizhou, Sichuan, Guangdong, Hainan, and Fujian. This spatial arrangement showcases an olive-shaped pattern characterized by fewer regions at the extremities and greater concentration in the middle. Heilongjiang province boasts fertile land and the most abundant agricultural resource endowment, consistently securing the top position in annual grain production. Moreover, the province’s complete agricultural industry value chain elevation fosters resource conservation and provides favorable conditions for bolstering green food production. Leveraging its strategic geographic position, Chongqing possesses abundant water resources and high soil organic matter content, with forest coverage exceeding 55%. Consequently, Chongqing exhibits a higher degree of agricultural circular economy development.
The spatial and resource-driven dynamics showcased by Heilongjiang and Chongqing underscore the significance of regional resource endowments and geographical attributes in shaping the agricultural circular economy landscape in China. These observations align with the broader goals of promoting sustainability and balanced development across different regions of the country.
(3) The estimated coefficient of 0.022 for digital rural development, supported by a significant 1% level of significance, underscores its effective act on boosting local agricultural circular economy. This could be attributed to the convenience digital rural development brings to new rural construction. Local governments, aiming to bridge the urban–rural gap, are inclined to increase financial investment in promoting digital rural development. On one hand, the integration of digital technologies in rural areas not only augments crop yield per unit area but also frees up labor, reducing costs and enhancing labor productivity. This consequently generates economic benefits. On the other hand, the introduction of green technologies via digital rural development effectively curbs excessive use of fertilizers and pesticides, safeguarding the environment for green agricultural. Catering to the trend of green consumption, products gradually transition toward being pollution-minimized, environmentally friendly, and free from contamination. Furthermore, digital rural development provides networking convenience, bolstering the promotion of green agricultural products during sales and marketing phases, thereby advancing agricultural circular economy development.
(4) The influence of digital rural development on the agricultural circular economy exhibits a single threshold effect. As the level of digital rural development crosses the threshold value, the elasticity coefficient of its impact on the agricultural circular economy increases from 0.048 to 0.058. In general, digital rural development acts as a driving force. With the elevation of digital rural development levels, its role in promoting agricultural circular economy development showcases a marginal increase. Which can be owed to the incremental nature of digital rural development, which encompasses information infrastructure, digital economy, and intelligent green practices. Comparatively advanced regions, equipped with higher education levels and rapid adoption of digitalization, demonstrate a higher utilization of digital technology and a greater propensity to foster green development and resource utilization in agriculture through digital rural development. Consequently, these regions are more likely to earn profit.
In conclusion, the integration of digital technologies through rural development initiatives holds substantial potential for advancing sustainable agricultural practices, enhancing resource efficiency, and fostering green economic growth.
Effectively promoting the development of circular agriculture is an essential path for rural revitalization. Based on calculations of the agricultural circular economy from 2011 to 2021 across 30 provinces in China, this study employed spatial econometric and threshold panel models to empirically explore the spatial spillover effects of digital rural development on agricultural circular economy and the threshold effects of varying levels of digital rural development on the latter. The study findings indicate that per capita arable land, forest coverage rate, and machinery utilization are crucial factors influencing the agricultural circular economy. While the level of agricultural circular economy development is gradually increasing, regional disparities persist, presenting an overall “low at the ends, high in the middle” olive-shaped pattern. Digital rural development exhibits a positive direct effect and spatial spillover effect. Which suggests that advancements in digital rural development exert a promotive influence on both local and neighboring regions’ agricultural circular economy development. The impact of digital rural development on the agricultural circular economy demonstrates a single threshold effect, with its influence gradually intensifying as digital rural development levels rise. Specifically, when the logarithm of the digital rural level is less than −2.7669, the coefficient of its impact on the agricultural circular economy is 0.048. Beyond this threshold, the coefficient increases to 0.058. This conclusion has certain enlightenment for the government and scholars.
The conclusions of this paper include three contributions, academics, politicians and practitioners engaged in agricultural production may be interested in the above conclusions.
Knowledge contribution: The research conclusion expands the boundary of the academic field of Beautiful China, and complement the range of applications of threshold theory and space theory, scholars may be interested in this conclusion, which provides a research direction;
Practical contribution 1: The research conclusion reveals the current situation of agricultural circular economy development, and understand its barrier factors, politicians may be interested in this conclusion, which provide relevant decision-making basis for the politicians to improve the key constraints of the agricultural industry and formulate rural development plans, agricultural policies.
Practical contribution 2: The research conclusion reveals that the influence of rural digital development on agricultural circular economy shows the law of increasing marginal effect, practitioners engaged in agricultural production may be interested in this conclusion. Practitioners actively participate in the construction of digital rural areas with relying on information technology, network and big data to improve agricultural economic benefits.
Besides, it still has some shortcomings in this paper. First, due to the limited availability of relevant data in rural China, this paper selects Provincial level data for analysis, the following research should obtain primary data through field visits, interviews and other methods. Second, the construction of the index system of agricultural circular economy needs to be improved, and there is no authoritative conclusion at present. Therefore, there may be some errors in choosing some relevant indicators to measure the level of agricultural circular economy.
Drawing from the conclusions above, several insights are derived:
First, address the insufficient development of rural circular economy and narrow provincial disparities. Despite the continuous improvement in China’s agricultural circular economy development index, the overall level remains relatively low, with a declining growth rate. Measures such as comprehensive utilization of undeveloped land, revitalization of abandoned land in “hollow villages,” and adjusting the area of arable land are to be implemented.
Second, establish a collaborative mechanism for interregional digital development and agricultural industry to expedite the fusion of digital rural development and agriculture. On one hand, augment the radiative effect of digital rural development on neighboring provinces to elevate the capacity of agricultural circular economy development. Gradually eliminate administrative barriers, facilitate the free flow of information resources, digital technology resources, and other resources, and realize efficient resource circulation and recycling to propel green, low-carbon, and efficient development in the agricultural industry. On the other hand, utilize smart agricultural big data platforms for real-time data monitoring in various stages of farming, planting, and fertilizing. This will facilitate information sharing and interconnection along the agricultural industry chain. Additionally, synchronize the development and industrial layout of agriculture in interregional planning.
Third, concentrate on the threshold characteristics between digital rural development and agricultural circular economy development. While comprehensively considering the objectives of digital rural and agricultural circular economy development, progress in digital rural development should be tailored according to the different development stages of regional digital rural construction. Address information infrastructure gaps, bridge the urban–rural digital divide, and simultaneously enhance digital literacy training among farmers.
The original contributions presented in the study are included in the article/supplementary material, further inquiries can be directed to the corresponding author.
JX: Conceptualization, Data curation, Formal analysis, Investigation, Methodology, Project administration, Software, Validation, Visualization, Writing – original draft, Writing – review & editing.
The author(s) declare that no financial support was received for the research, authorship, and/or publication of this article.
The author declares that the research was conducted in the absence of any commercial or financial relationships that could be construed as a potential conflict of interest.
All claims expressed in this article are solely those of the authors and do not necessarily represent those of their affiliated organizations, or those of the publisher, the editors and the reviewers. Any product that may be evaluated in this article, or claim that may be made by its manufacturer, is not guaranteed or endorsed by the publisher.
Abdel-Basset, M., and Mohamed, R. (2020). A novel plithogenic TOPSIS-CRITIC model for sustainable supply chain risk management. J. Clean. Prod. 247:119586. doi: 10.1016/j.jclepro.2019.119586
Abdul-Hamid, A. Q., Ali, M. H., Tseng, M. L., Lan, S., and Kumar, M. (2023). Impeding challenges on industry 4.0 in circular economy: palm oil industry in Malaysia. Comput. Oper. Res. 123:105052. doi: 10.1016/j.cor.2020.105052
Acerbi, F., and Taisch, M. A. (2020). Literature review on circular economy adoption in the manufacturing sector. J. Clean. Prod. 273:123086. doi: 10.1016/j.jclepro.2020.123086
Ali, Z. A., Zain, M., Pathan, M. S., and Mooney, P. (2023). Contributions of artificial intelligence for circular economy transition leading toward sustainability: An explorative study in agriculture and food Industries of Pakistan. Environ. Dev. Sustain. :14. doi: 10.1007/s10668-023-03458-9
Bag, S., Wood, L. C., Mangla, S. K., and Luthra, S. (2020). Procurement 4.0 and its implications on business process performance in a circular economy. Resour. Conserv. Recycl. 152:104502. doi: 10.1016/j.resconrec.2019.104502
Batlles-delaFuente, A., Abad-Segura, E., González-Zamar, M.-D., and Cortés-García, F. J. (2022). An evolutionary approach on the framework of circular economy applied to agriculture. Agronomy 12:620. doi: 10.3390/agronomy12030620
Cantzler, J., Creutzig, F., Ayargarnchanakul, E., Javaid, A., Wong, L., and Haas, W. (2020). Saving resources and the climate? A systematic review of the circular economy and its mitigation potential. Environ. Res. Lett. 15:12. doi: 10.1088/1748-9326/abbeb7
Chang, C. W. (2019). Using entropy to construct and evaluate player’s value and sustainable development model. J. Inform. Optimiz. Sci. 40, 1337–1349. doi: 10.1080/02522667.2019.1645395
Chen, Y., Wang, Y., and Zhao, C. (2024). From riches to digitalization: the role of AMC in overcoming challenges of digital transformation in resource-rich regions. Technol. Forecast. Soc. Chang. 200:123153. doi: 10.1016/j.techfore.2023.123153
Dantas, T. E., De-Souza, E., Destro, I., Hammes, G., Rodriguez, C., and Soares, S. (2021). How the combination of circular economy and industry 4.0 can contribute towards achieving the sustainable development goals. Sustain. Product. Consum. 26, 213–227. doi: 10.1016/j.spc.2020.10.005
Davide, B., Idiano, D., Valerio, G., and Paolo, R. (2023). Digitalizing circular economy through Blockchains: the Blockchain circular economy index. J. Ind. Prod. Eng. 40, 233–245. doi: 10.1080/21681015.2023.2173317
Diakoulaki, D., Mavrotas, G., and Papayannakis, L. (1995). Determining objective weights in multiple criteria problems: the CRITIC method. Comput. Oper. Res. 22, 763–770. doi: 10.1016/0305-0548(94)00059-H
Duque-Acevedo, M., Belmonte-Ureña, L. J., Plaza-Úbeda, J. A., and Camacho-Ferre, F. (2020). The Management of Agricultural Waste Biomass in the framework of circular economy and bioeconomy: An opportunity for greenhouse agriculture in Southeast Spain. Agronomy 10:489. doi: 10.3390/agronomy10040489
Francesca, F., Raffaella, S., Stefania, M., Roberto, D. F., Paolo, V., Luigi, P., et al. (2020). An innovative IoT-oriented prototype platform for the management and valorisation of the organic fraction of municipal solid waste. J. Clean. Prod. 247:119618. doi: 10.1016/j.jclepro.2019.119618
Gagan, D. S., Anshita, Y., and Ritika, C. (2020). Artificial intelligence and effective governance: a review, critique and research agenda. Sustainable Futures 2:100004. doi: 10.1016/j.sftr.2019.100004
García-Muiña, F., Medina-Salgado, M. S., González-Sánchez, R., Huertas-Valdivia, I., Ferrari, A. M., and Settembre-Blundo, D. (2021). Industry 4.0-based dynamic social organizational life cycle assessment to target the social circular economy in manufacturing. J. Clean. Prod. 327:129439. doi: 10.1016/j.jclepro.2021.129439
Geng, Y., Fu, J., and Sarkis, J. (2012). Towards a National Circular Economy Indicator System in China: An evaluation and critical analysis. J. Clean. Prod. 23, 216–224. doi: 10.1016/j.jclepro.2011.07.005
Guo, X., Liao, Z., and Zhang, M. (2011). Basic situation and countermeasures of modern agricultural circular economy development. Agric. Econ. Issues 35:10.
Guo, C., Zhang, R., and Zou, Y. (2023). The efficiency of China’s agricultural circular economy and its influencing factors under the rural revitalization strategy: a DEA–Malmquist–Tobit approach. Agriculture 13:1454. doi: 10.3390/agriculture13071454
Hu, Y., He, X., and Poustie, M. (2018). Can legislation promote a circular economy? A material flow-based evaluation of the circular degree of the Chinese economy. Sustain. For. 10:990. doi: 10.3390/su10040990
Hu, F., Qiu, L., Wei, S., Zhou, H., Bathuure, I. A., and Hu, H. (2023). The spatiotemporal evolution of global innovation networks and the changing position of China: a social network analysis based on cooperative patents. R&D Manag. doi: 10.1111/radm.12662
Hui, Y., Cuixia, L., and Yang, Y. (2014). A study on the efficiency evaluation of recycling economy of agriculture in Heilongjiang Province. BioTechnology 10:11709.
Khan, S., Piprani, A. Z., and Yu, Z. (2022). Digital technology and circular economy practices: future of supply chains. Oper. Manag. Res. 15, 676–688. doi: 10.1007/s12063-021-00247-3
Khan, S. A. R., Zia-ul-haq, H. M., Umar, M., and Yu, Z. (2021). Digital technology and circular economy practices: An strategy to improve organizational performance. Bus. Strateg. Environ. 4, 482–490. doi: 10.1002/bsd2.176
Kiselev, A., Magaril, E., Magaril, R., Panepinto, D., Ravina, M., and Zanetti, M. C. (2019). Towards circular economy: evaluation of sewage sludge biogas solutions. Resources 8:91. doi: 10.3390/resources8020091
Kumar, S., Raut, R. D., Nayal, K., Kraus, S., Yadav, V. S., and Narkhede, B. E. (2021). To identify industry 4.0 and circular economy adoption barriers in the agriculture supply chain by using ISM-ANP. J. Clean. Prod. 293:126023. doi: 10.1016/j.jclepro.2021.126023
Lange, G., Wodon, Q., Carey, K., Lange, G.-M., Wodon, Q., and Carey, K. (2018) The changing wealth of nations 2018: Building a sustainable future. The World Bank: Washington, DC, USA
Laura, P. (2023). The sustainability impact of a digital circular economy. Curr. Opin. Environ. Sustain. 61:101251. doi: 10.1016/j.cosust.2022.101251
Li, L. (2023). An empirical analysis of rural labor transfer and household income growth in China. J. Chin. Hum. Resour. Manag. 14, 106–116. doi: 10.47297/wspchrmWSP2040-800505.20231401
Li, J., Zhang, G., Ned, J. P., and Sui, L. (2023). How does digital finance affect green technology innovation in the polluting industry? Based on the serial two-mediator model of financing constraints and research and development (R&D) investments. Environ. Sci. Pollut. Res. 30, 74141–74152. doi: 10.1007/s11356-023-27593-y
Lieder, M., and Rashid, A. (2016). Towards circular economy implementation: a comprehensive review in context of manufacturing industry. J. Clean. Prod. 115, 36–51. doi: 10.1016/j.jclepro.2015.12.042
Liu, Z. (2022). Rural population decline, cultivated land expansion, and the role of land transfers in the farming-pastoral ecotone: a case study of Taibus, China. Land 11:256. doi: 10.3390/land11020256
Liu, Q., Trevisan, A., Yang, M., and Mascarenhas, J. (2022). A framework of digital technologies for the circular economy: digital functions and mechanisms. Bus. Strateg. Environ. 31, 2171–2192. doi: 10.1002/bse.3015
Luciano, A., Cutaia, L., Cioffi, F., and Sinibaldi, C. (2021). Demolition and construction recycling unified management: the DECORUM platform for improvement of resource efficiency in the construction sector. Environ. Sci. Pollut. Res. 28, 24558–24569. doi: 10.1007/s11356-020-09513-6
Luo, J., Zhao, C., Chen, Q., and Li, G. (2022). Using deep belief network to construct the agricultural information system based on internet of things. J. Supercomput. 78, 379–405. doi: 10.1007/s11227-021-03898-y
Mallick, R., Pramanik, S., and Giri, B. C. (2023). Neutrosophic MAGDM based on critic-EDAS strategy using geometric aggregation operator. Yugoslav J. Operat. Res. 33, 683–698. doi: 10.2298/YJOR221017016M
Maurício, D., Priscila, R. G., Marco, A., Abdelghani, E. A., Pia, B., Adili, J. C., et al. (2022). International circular economy strategies and their impacts on agricultural water use. Cleaner Engin. Technol. 8:100504
Moreno, M., Court, R., Wright, M., and Charnley, F. (2019). Opportunities for redistributed manufacturing and digital intelligence as enablers of a circular economy. Int. J. Sustain. Eng. 12, 77–94. doi: 10.1080/19397038.2018.1508316
Musyarofah, S. A., Tontowi, A. E., Masruroh, N. A., Wibowo, B. S., Warmadewanthi, I. D. A. A., Nasution, A. H., Bhawika, G. W., Handiwibowo, G. A., and Rusydi, M. K. Developing a circular economy index to measure the macro level of circular economy implementation in Indonesia. Manag. Syst. Product. Engin., (2023), 31, 208–215, doi: 10.2478/mspe-2023-0022
Pan, H. Y., Zhang, X. H., Wang, Y. Q., Qi, Y., Wu, J., and Lin, L. L. (2016). Emergy evaluation of an Industrial Park in Sichuan Province, China: a modified Emergy approach and its application. J. Clean. Prod. 135, 105–118. doi: 10.1016/j.jclepro.2016.06.102
Ronaghi, M. H. (2022). The influence of artificial intelligence adoption on circular economy practices in manufacturing industries. Environ. Dev. Sustain. 25, 14355–14380. doi: 10.1007/s10668-022-02670-3
Saidani, M., Yannou, B., Leroy, Y., Cluzel, F., and Kendall, A. (2019). A taxonomy of circular economy indicators. J. Clean. Prod. 207, 542–559. doi: 10.1016/j.jclepro.2018.10.014
Schöggl, J. P., Rusch, M., Stumpf, L., and Baumgartner, R. J. (2023). Implementation of digital technologies for a circular economy and sustainability management in the manufacturing sector. Sustain. Product. Consum. 35, 401–420. doi: 10.1016/j.spc.2022.11.012
Schroeder, P., Anggraeni, K., and Weber, U. (2019). The relevance of circular economy practices to the sustainable development goals. J. Ind. Ecol. 23, 77–95. doi: 10.1111/jiec.12732
Thiru, S., Lumgailu, P., Kumar, K. M., Guleria, V., Ramesh, R. K., Bhardwaj, D. R., et al. (2023). Circular economy in agriculture: unleashing the potential of integrated organic farming for food security and sustainable development. Front. Sustain. Food Syst. 7:1170380. doi: 10.3389/fsufs.2023.1170380
Velasco-Muñoz, J. F., Mendoza, J. M. F., Aznar-Sánchez, J. A., and Gallego-Schmid, A. (2021). Circular economy implementation in the agricultural sector: definition, strategies and indicators. Resour. Conserv. Recycl. 170:105618. doi: 10.1016/j.resconrec.2021.105618
Vincent, K. T., Abdulrasheed, Z., and Irfan, K. (2021). The environmental footprint of China-Africa engagement: an analysis of the effect of China – Africa partnership on carbon emissions. Sci. Total Environ. 756:143603. doi: 10.1016/j.scitotenv.2020.143603
Winter, J. M., Lopez, J. R., Ruane, A. C., Young, C. A., Scanlon, B. R., and Rosenzweig, C. (2017). Representing water scarcity in future agricultural assessments. Anthropocene 18, 15–26. doi: 10.1016/j.ancene.2017.05.002
Wu, Z. H., Dang, J. Q., Pang, Y. P., and Xu, W. (2021). Threshold effect or spatial spillover? The impact of agricultural mechanization on grain production. J. Appl. Econ. 24, 478–503. doi: 10.1080/15140326.2021.1968218
Wynn, M., and Jones, P. (2022). Digital technology deployment and the circular economy. Sustain. For. 14:9077. doi: 10.3390/su14159077
Zhao, S., Zhang, L., An, H., Peng, L., Zhou, H., and Hu, F. (2023). Has China’s low-carbon strategy pushed forward the digital transformation of manufacturing enterprises? Evidence from the low-carbon city pilot policy. Environ. Impact Assess. Rev. 102:107184. doi: 10.1016/j.eiar.2023.107184
Keywords: agricultural circular economy, digital rural development, spatial spillover effects, threshold characteristics
Citation: Xu J (2024) Spatial spillover and threshold effects of digital rural development on agricultural circular economy growth. Front. Sustain. Food Syst. 8:1337637. doi: 10.3389/fsufs.2024.1337637
Received: 14 November 2023; Accepted: 21 February 2024;
Published: 11 March 2024.
Edited by:
Christian Bux, University of Bari Aldo Moro, ItalyReviewed by:
Surapati Pramanik, Nandalal Ghosh BT College, IndiaCopyright © 2024 Xu. This is an open-access article distributed under the terms of the Creative Commons Attribution License (CC BY). The use, distribution or reproduction in other forums is permitted, provided the original author(s) and the copyright owner(s) are credited and that the original publication in this journal is cited, in accordance with accepted academic practice. No use, distribution or reproduction is permitted which does not comply with these terms.
*Correspondence: Jiehua Xu, Zmxvd2Vyc3hqaEAxNjMuY29t
Disclaimer: All claims expressed in this article are solely those of the authors and do not necessarily represent those of their affiliated organizations, or those of the publisher, the editors and the reviewers. Any product that may be evaluated in this article or claim that may be made by its manufacturer is not guaranteed or endorsed by the publisher.
Research integrity at Frontiers
Learn more about the work of our research integrity team to safeguard the quality of each article we publish.