- 1Research Institute for Geo-Hydrological Protection, National Research Council, Perugia, Italy
- 2Department of Civil and Environmental Engineering, University of Perugia, Perugia, Italy
- 3Department of Geodesy and Geoinformation, Vienna University of Technology (Tu Wien), Vienna, Austria
- 4International Center for Environmental Monitoring CIMA Research Foundation, University of Genoa, Savona, Italy
- 5Hydro-Climate Extremes Lab (H-CEL), Department of Environment, Ghent University, Ghent, Belgium
- 6Meteorological Environmental Earth Observation s.r.l., Ferrara, Italy
- 7Earth Observation Data Centre for Water Resources Monitoring GmbH, Vienna, Austria
- 8Department of Civil, Chemical, Environmental and Materials Engineering, Alma Mater Studiorum-University of Bologna, Bologna, Italy
- 9Institute for Earth Observation, Eurac Research, Bolzano, Italy
- 10Image Processing Laboratory, University of València, Valencia, Spain
- 11European Space Agency, European Space Research Institute (ESRIN), Frascati, Italy
Abstract
Climate change is profoundly affecting the global water cycle, increasing the likelihood and severity of extreme water-related events. Better decision-support systems are vital to accurately predict and monitor water-related environmental disasters and optimally manage water resources. These must integrate advances in remote sensing, in situ, and citizen observations with high-resolution Earth system modeling, artificial intelligence (AI), information and communication technologies, and high-performance computing. Digital Twin Earth (DTE) models are a ground-breaking solution offering digital replicas to monitor and simulate Earth processes with unprecedented spatiotemporal resolution. Advances in Earth observation (EO) satellite technology are pivotal, and here we provide a roadmap for the exploitation of these methods in a DTE for hydrology. The 4-dimensional DTE Hydrology datacube now fuses high-resolution EO data and advanced modeling of soil moisture, precipitation, evaporation, and river discharge, and here we report the latest validation data in the Mediterranean Basin. This system can now be explored to forecast flooding and landslides and to manage irrigation for precision agriculture. Large-scale implementation of such methods will require further advances to assess high-resolution products across different regions and climates; create and integrate compatible multidimensional datacubes, EO data retrieval algorithms, and models that are suitable across multiple scales; manage uncertainty both in EO data and models; enhance computational capacity via an interoperable, cloud-based processing environment embodying open data principles; and harness AI/machine learning. We outline how various planned satellite missions will further facilitate a DTE for hydrology toward global benefit if the scientific and technological challenges we identify are addressed.
Key points
- The increasing likelihood and severity of extreme water-related events necessitate high-precision decision-support systems to predict and monitor water-related environmental disasters and optimally manage water resources.
- A Digital Twin Earth (DTE) of the terrestrial water cycle offers a ground-breaking solution for monitoring and simulation, but it requires high-resolution (1 km, 1 hour) satellite Earth observation (EO) data and an understanding of the human impact on hydrological processes.
- High-resolution EO data are now being integrated with advanced and spatially distributed modeling systems, enabling large-scale applications of a DTE for hydrology to be explored to forecast flash floods and landslides, enable precision agriculture, monitor fires, and develop what-if scenarios for flood risk assessment and water resources management.
- To scale up a DTE for hydrology, we need to assess high-resolution products across regions and climates, integrate compatible, multi-scale EO data and models, manage uncertainty in data, and implement collaborative research infrastructures.
- Various planned satellite missions will further facilitate a DTE for hydrology toward global benefit if the scientific and technological challenges we identify are addressed.
Introduction
Human-induced climate change is affecting all components of the global water cycle, increasing the likelihood and severity of extreme water-related events, including heavy precipitation, flooding, landslides, drought, and wildfires (1, 2). These events are already causing substantial mortality, agricultural disruption, economic damage, and ecological impacts, and their intensity and impact are projected to increase (1, 2). A new generation of decision-support systems is vital to better predict and monitor water-related environmental disasters and optimally manage water resources.
In recent years, high-resolution observations (<1 km, <1 day) now available from remote sensing, in situ monitoring networks, and new sensor technologies and techniques (including drones and citizen science research) have been coupled with increased computational and storage capacity and advanced machine learning methods to produce high-resolution hydrology modeling systems.
In the past two decades, the challenge of reconstructing the terrestrial water cycle at high resolution has been addressed mainly by the development of global hydrological and land surface modeling (3–8). Several global hydrological and land surface models now exist and the first intercomparison studies have improved our knowledge of the global water cycle (9, 10). The pioneering studies on hyper-resolution global hydrological modeling were performed by Wood et al. (3) and Bierkens et al. (5), who highlighted the challenges in building a Digital Twin Earth (DTE) of the water, energy, and carbon cycles even before the term DTE had been coined by the community. These challenges were related to processing representation and parameterization, limited availability of high-resolution observations for model inputs and model testing (11, 12), high computational expenses, and particularly the inclusion of human impacts on the water cycle (6). Indeed, modeling any of the Earth’s physical processes becomes even more challenging when including the effects of human behaviors. To address these challenges, some authors have proposed the use of “hybrid” modeling approaches (13) that couple physical (process-based) models with the versatility of (data-driven) machine learning to estimate variables for which our knowledge remains limited (14). These approaches facilitate a seamless incorporation of recently available satellite datasets (e.g., soil moisture or groundwater storage) that are typically difficult to integrate into physically based models (15). Nowadays, hybrid approaches are also being used to characterize traditionally elusive components of the terrestrial hydrological cycle, such as evaporation (16).
Earth observation (EO) provides different products for describing the water cycle but mostly at coarse spatial resolution (>10 km). For instance, remote sensing soil moisture products at coarse resolution can be obtained from different satellite platforms and sensors, such as the Soil Moisture and Ocean Salinity (SMOS), the Soil Moisture Active and Passive (SMAP), or the Advanced SCATterometer (ASCAT) platforms. More recently, the Sentinel-1 Copernicus satellites have enabled native high-resolution (<1 km) soil moisture datasets (i.e., not considering products obtained through downscaling) (17–21). Exploitation of the Sentinel constellation (Sentinel-1, -2, and -3) has also allowed this for variables such as evaporation (22–24), precipitation [e.g., Karger et al. (25); Filippucci et al. (26); He et al. (27)], snow depth (28), and river discharge (29–31). Moreover, the integration of Sentinel with Landsat, Moderate Resolution Imaging Spectroradiometer (MODIS), and Visible Infrared Imaging Radiometer Suite (VIIRS) provides the potential to develop long-term datasets for evaporation [e.g., Jaafar et al. (32)] and river discharge (33) at high spatial resolution.
This article explores how recent high-resolution data of both space (<1 km) and time (<1 day), e.g., from the Sentinel constellation, have provided the possibility to obtain an accurate and high-resolution DTE of the water cycle, and it offers a roadmap for the future exploitation of these methods toward global benefit. Indeed, these current EO capabilities allow high-resolution data to be integrated into modeling systems both as forcing data and as validation datasets, thereby warranting an update of the requirements and the challenges to be addressed beyond those previously identified (3, 5). While we differ in our approach from Rigon et al. (34), who described an example of theoretical architecture for building a DTE in hydrology without specifying its content (i.e., without showing real-world examples or an actual implementation of the concept), we concur with these authors regarding the need to promote a new participatory way of doing hydrological science, where researchers can contribute cooperatively to characterize and control model outcomes in different regions worldwide. We also build on the study by Alfieri et al. (35), who described the main results obtained from the integration of remote sensing data and advanced modeling over the Po River Basin under the first phase of the DTE Hydrology project funded by the European Space Agency (ESA).
First, we briefly describe the overall framework of DTE Hydrology in which, for the first time, high spatiotemporal resolution satellite products of soil moisture, precipitation, evaporation, snow depth, and river discharge are integrated into advanced hydrological modeling systems (35). Second, we focus on the main challenges (e.g., quality assessment for soil moisture and evaporation, products, and model selection, ensuring a balance between product consistency and independence and uncertainty assessment) to be addressed when developing a reliable remote-sensing-based datacube for hydrology, specifically for the key variables of the water terrestrial balance: precipitation, evaporation, soil moisture, and river discharge. Third, we explore the capabilities of future satellite missions. Finally, we outline the priority steps necessary to develop an operational, high-resolution DTE for hydrology.
DTE Hydrology: a prototype for exploiting high-resolution EO in hydrology
The overall objective of DTE Hydrology is the 4-dimensional (4D) reconstruction of the terrestrial water cycle at high resolution through the integration of the most recent satellite observations and advanced hydrological modeling (Figures 1, 2 show an example over the Po River Basin).
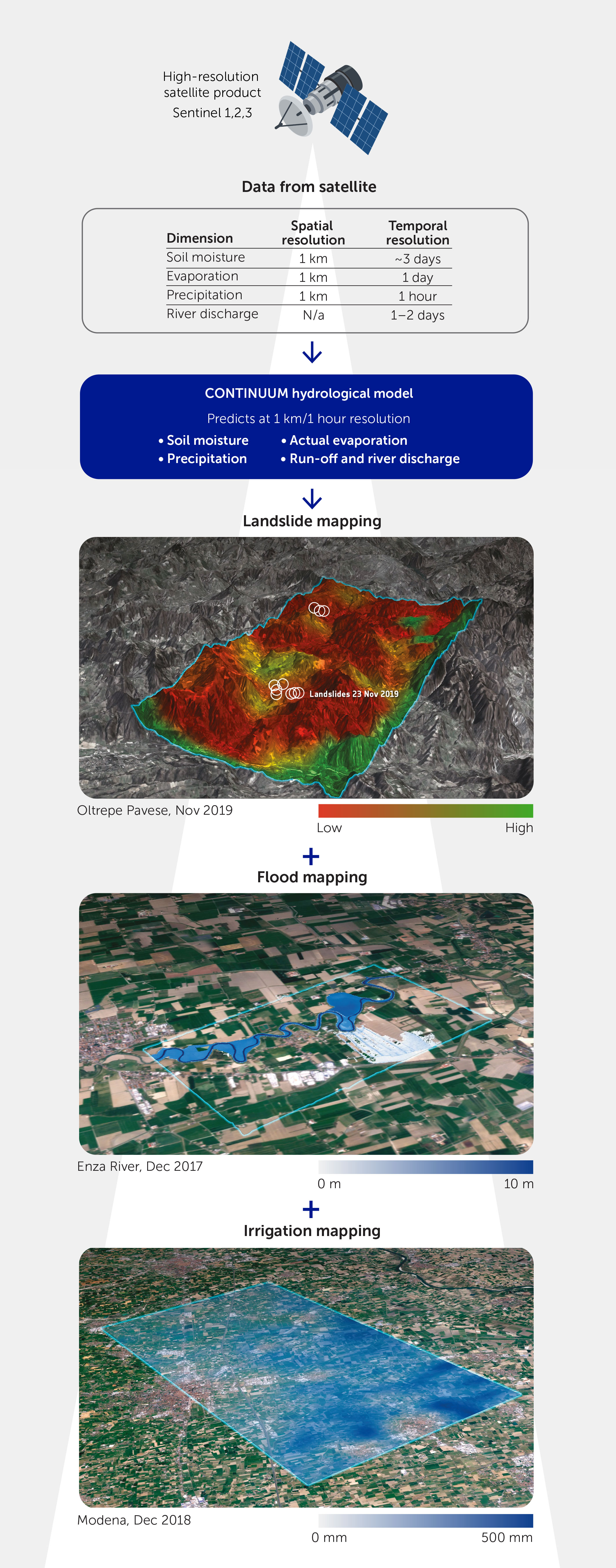
Figure 1 High-level description of the Digital Twin Earth (DTE) Hydrology modeling framework. The four-dimensional, i.e., three dimensions in space and one in time, the DTE Hydrology datacube (soil moisture, precipitation, evaporation, and river discharge) fuses earth observation and modeled data from high-resolution satellite products. This is integrated into the advanced hydrological modeling system CONTINUUM to predict high-resolution (1 km, 1 hour) soil moisture, evaporation, and river discharge. The modeling output is combined with satellite observations in the application modules for landslide prediction, flooding simulation, and irrigation.
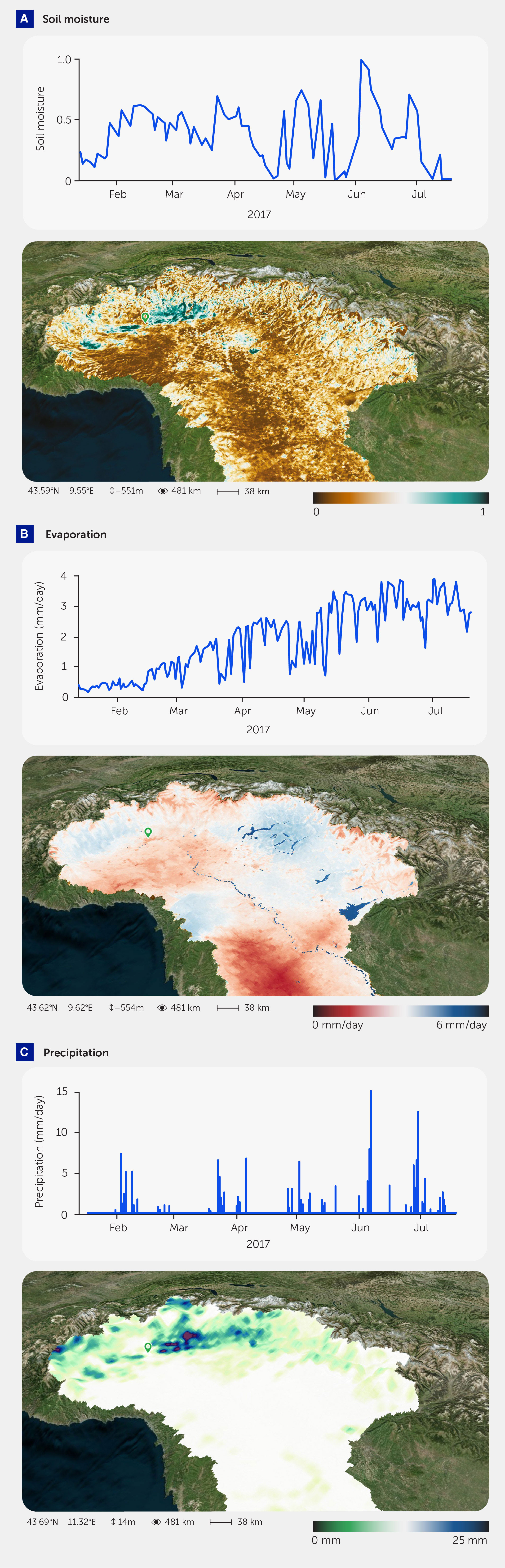
Figure 2 Digital Twin Earth (DTE) Hydrology sample outputs for (A) soil moisture, (B) evaporation, and (C) precipitation.
The Po River Basin in northern Italy was selected as the first case study owing to the availability of high-quality ground observations to calibrate and test the quality of the satellite observations and modeling system. The 4D DTE Hydrology datacube is currently available for the whole Mediterranean Basin, and its development for the whole of Europe is planned. Three applications of the model were investigated: flooding simulation (36), landslide prediction, and irrigation water management. What-if scenarios are developed to provide decision-makers with a digital modeling platform to visualize, monitor, and forecast natural and human activity on the planet in support of sustainable development and the prediction and management of environmental disasters (see https://explorer.dte-hydro.adamplatform.eu/).
Building the DTE Hydrology tool involved four sequential steps:
● building the 4D DTE Hydrology datacube—a high-resolution (1 km, daily/hourly, 2016–2022) EO- and modeling-based dataset (Table 1),
● developing a high-resolution modeling system utilizing the 4D DTE Hydrology datacube (e.g., as input data, for parameterization, in a data assimilation framework, etc.) to provide a 4D reconstruction of the terrestrial water cycle (Figure 3),
● integrating the modeling system into the cloud-based DTE Hydrology simulation and visualization tool,
● exploiting the DTE Hydrology tool to develop user-oriented case studies and what-if scenarios focusing on flood and landslide hazard mapping and water resources management (Figure 3).
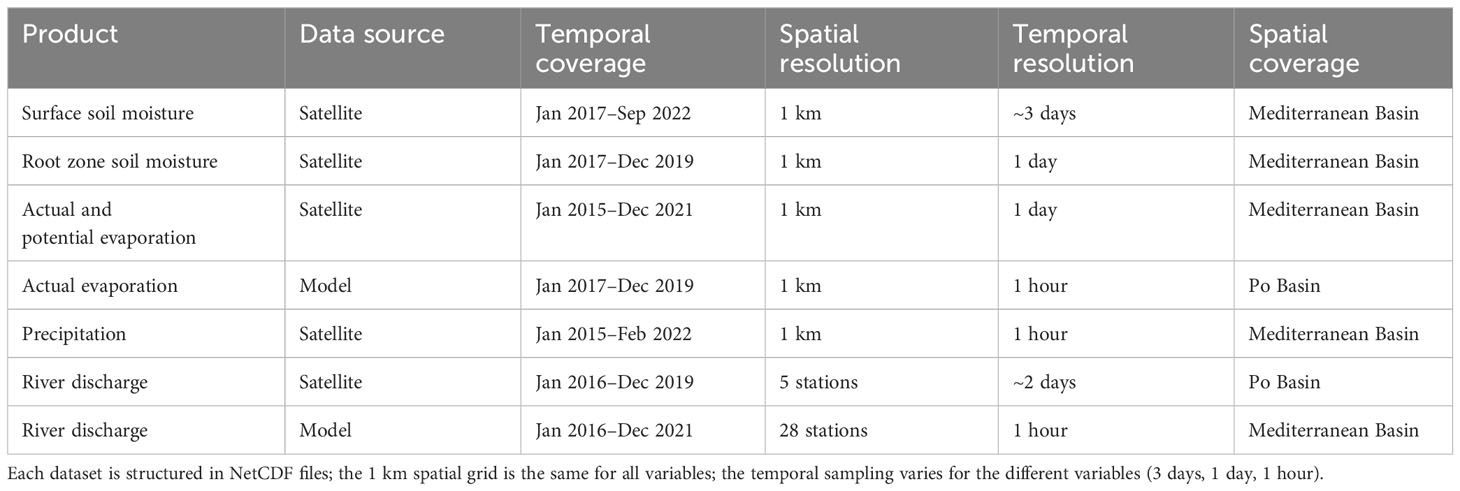
Table 1 Products contained in the four-dimensional (4D) Digital Earth Twin (DTE) Hydrology datacube.
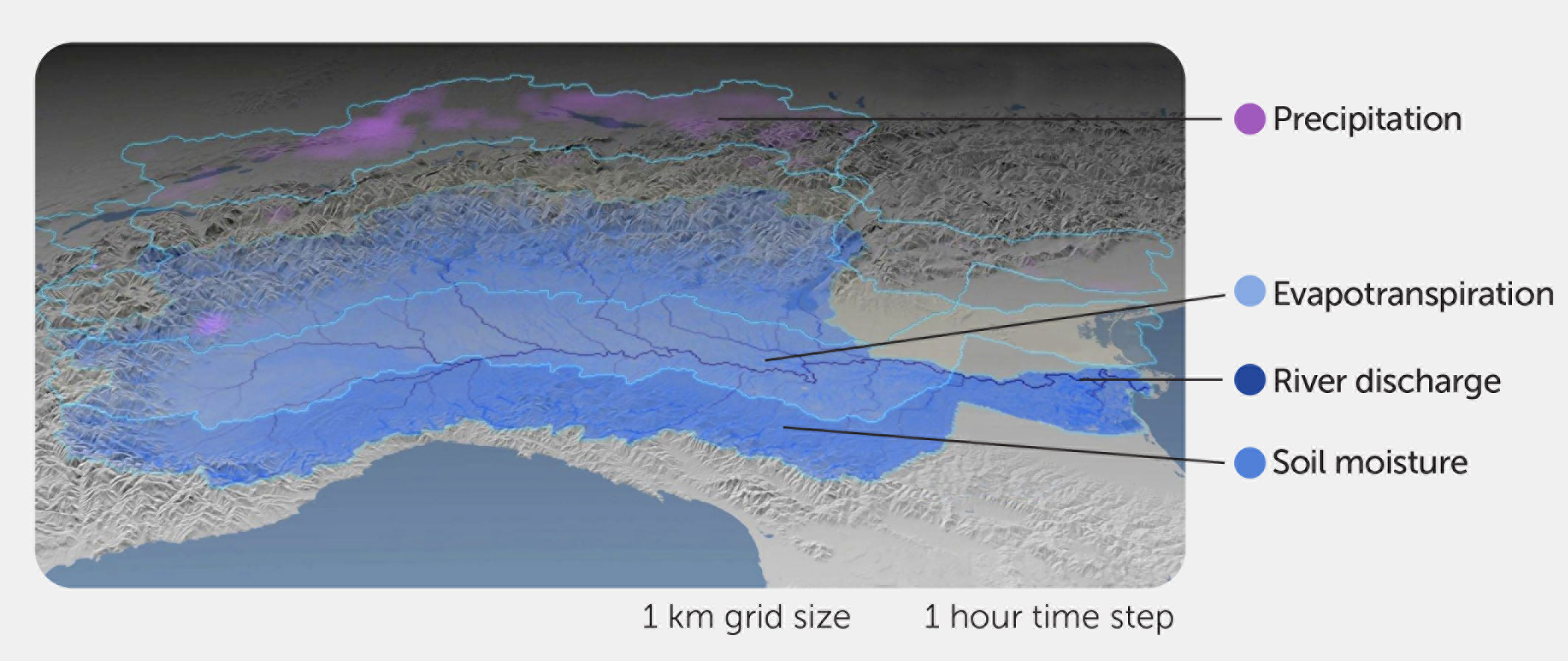
Figure 3 Visualization of the Digital Twin Earth (DTE) Hydrology datacube over the Po River Basin, Italy.
This work led to a regional DTE prototype focusing on the terrestrial water cycle, hydrological processes, and their impacts (35).
High-resolution EO-data hydrological cycle reconstruction
The first step in the DTE Hydrology project was the building of the 4D DTE Hydrology datacube, offering an advanced data-driven reconstruction of the hydrological cycle. Multiple satellite products were tested for the variables of soil moisture, precipitation, evaporation, and river discharge. Based on the results of the validation and the modeling simulation, a final version of the 4D DTE Hydrology datacube was selected and made available openly to the science community (see Figure 3 for the Po River Basin; the dataset for the whole Mediterranean Basin can be found here: https://viewer.earthsystemdatalab.net/?dataset=hydrology).
The 4D DTE Hydrology datacube fuses satellite and modeled data from high-resolution satellite products.
Satellite data
Soil moisture
An improved high-resolution (1 km) soil moisture product was obtained by applying the RT1 algorithm (37) to Sentinel-1 observations. The resulting soil moisture product has a spatial and temporal resolution of 1 km and nearly 3 days, respectively, and a temporal coverage from 2017 to 2022 for the Mediterranean Basin. The RT1 algorithm is a first-order expansion of the radiative transfer equation, modeling backscatter as the result of scattering from a (rough) surface covered by vegetation. It is assumed that multiple scattering does not contribute to the signal at the sensor. As for all soil moisture retrieval algorithms from microwave observations, the challenge is to separate the signal coming from the soil from that of vegetation. This is even more important at high spatial resolution, as the signal is not an aggregation over multiple vegetation types. DTE Hydrology leverages high-resolution products to obtain the most reliable soil moisture at 1 km and parametrizes vegetation dynamically using the Leaf Area Index from the Copernicus Global Land Service (300 m version). Quantitative validation of soil moisture at high spatial resolution is challenging owing to a lack of reference data at the scale necessary. Therefore, validation was carried out with ERA5-Land soil moisture at 9 km (38). High accuracy was found, especially over croplands (39). The high spatial detail can potentially provide more reliable soil moisture information over areas of complex topography in comparison to coarse-resolution soil moisture products.
Precipitation
A first-of-its-kind satellite precipitation product with an hourly timescale and 1 km spatial resolution was obtained through the integration of three parent products (1): the Integrated Multi-satellitE Retrievals for Global precipitation measurement (IMERG) late-run product (10 km, 1 hour) (2), the SM2RAIN-ASCAT product (40) based on the application of the Soil Moisture to RAINfall (SM2RAIN) algorithm (41, 42) to the Advanced SCATterometer (ASCAT) soil moisture (10 km, 1 day), and (3) SM2RAIN applied to Sentinel-1 soil moisture from RT1 (26) (1 km, 3 day). The integration of the three products was optimized considering the pixel-based signal-to-noise ratios obtained via triple collocation analysis (35). The resulting precipitation product has a spatial and temporal resolution of 1 km and 1 hour and temporal coverage from 2016 to 2019 over the Po River Basin, and it has been tested for the first time in DTE Hydrology (35). The product is being extended for the whole Mediterranean Basin, and a first release is available in the datacube from 2015 to 2022 at a daily time scale.
Evaporation
The high-resolution version of the Global Land Evaporation Amsterdam Model [GLEAM; Miralles et al. (43)] evaporation product (25 km, 1 day) was obtained by making use of high-resolution static and dynamic datasets describing the land surface, e.g., the MODIS fractional vegetation cover (44) and the 3D soil hydraulic database (45). Furthermore, datasets from the DTE Hydrology project were used: Sentinel-1-based soil moisture retrievals (37) were assimilated through a Newtonian nudging scheme, and the merged precipitation product (see above) was used as input for GLEAM. Further advances in making use of high-resolution observations in GLEAM include the application of Sentinel 3 land surface temperature retrievals for creating high-resolution and gap-free temperature and net radiation forcing data as well as the use of high-resolution vegetation properties (46). The assimilation of Sentinel-1 backscatter observations using either a traditional or machine-learning-based forward operator has also been explored (47). The resulting product (1 km, 1 day) of actual and potential evaporation is available from 2015 to 2021 for the Mediterranean Basin. The satellite-based high-resolution evaporation product has been evaluated, with a few stations in Europe showing a performance similar to the well-established GLEAM product at 25 km resolution (35). However, given the low availability of eddy-covariance towers, performances are evaluated mainly over time, and a full spatial assessment revealing the value of the improved resolution cannot be performed through comparison to in situ data.
River discharge
The integrated river discharge product first developed under the ESA RIDESAT project has been further improved in DTE Hydrology and tested along the Po River at five hydrometric stations. The product has a temporal resolution of 1–2 days, thanks to the integration of multiple altimetry tracks (Cryosat-2, Sentinel-3, Saral/AltiKa) and multiple near-infrared observations (from MODIS onboard AQUA and TERRA satellites, OLCI onboard Sentinel-3, and MSI onboard Sentinel-2). The river discharge was calculated as the product of flow area multiplied by the flow velocity. Specifically, the flow area is calculated as a function of the altimetry water level (even in the absence of bathymetry), whereas the flow velocity is described by the reflectance index measured by the near-infrared signal of the multispectral sensor. The coefficients used to estimate the flow area and velocity were calibrated by minimizing the root mean square error (RMSE) between the simulated river discharges and those recorded at ground-gauged stations. The comparison with the discharge data recorded at the gauged stations along the Po River shows high performances with an average Nash–Sutcliffe (NS) of 0.81, Kling-Gupta Efficiency (KGE) of 0.88, and relative RMSE (rRMSE) of 26 %. These performance metrics highlight the capability of the satellite discharge product to reproduce almost daily variations with good accuracy.
Snow depth
To reconstruct water-balance timing and seasonality at high elevations, EO-based snow depth data were also used in DTE Hydrology. These data were retrieved from the Sentinel-1 empirical change detection approach developed by Lievens et al. (28) and show a resolution of 1 km and daily granularity. This product, called C-SNOW, was not developed during the period we used DTE Hydrology, and thus it is not included in the datacube of this article. Given the essential contribution of snow in driving the terrestrial water cycle, readers are referred to Lievens et al. (28) and Lievens et al. (48); data are freely available at https://ees.kuleuven.be/project/c-snow.
Modeled data
In addition to EO-based products, we have used the fully distributed cryospheric model Snow Multidata Mapping and Modeling (S3M) (49) and the hydrological model CONTINUUM (50) to develop a high-resolution (1 km, 1 hour) modeled product for root-zone soil moisture, actual evaporation, and river discharge. The modeled dataset covers the period 2016–2021, with the year 2015 being used for model warm-up. The model allows us to obtain hourly estimates of hydrological variables by using forcing satellite observations of potential evaporation and precipitation. In Alfieri et al. (35) we have carried out a set of experiments where satellite observations are used: (i) as forcing data (precipitation and evaporation), (ii) for parameter calibration (river discharge), and (iii) in data assimilation (soil moisture and snow depth). Different configurations have been analyzed and compared by also considering a combination of ground-based and satellite products. We have obtained important insights into the quality of satellite products, the possibility of integrating such high-resolution observations into hydrological modeling, and the limitations that must be overcome to optimally integrate model and satellite products into a data assimilation framework. Specifically, Alfieri et al. (35) found that satellite-based evaporation and snow depths slightly improved the mean KGE at 27 river gauges compared with a baseline simulation forced by high-quality conventional ground data. River discharge showed the largest sensitivity to satellite precipitation, though it generally led to accurate results.
The current version of the 4D DTE Hydrology datacube contains remote sensing of 1 km and modeled data for the Mediterranean Basin in the period 2015–2022 (Table 1).
Quality assessment and exploitation of the DTE Hydrology datacube
A DTE for hydrology must be carefully tested to ensure it provides robust and accurate predictions for decision-makers. However, the validation of high-resolution satellite products is very challenging because in situ observations with the same spatial resolution and coverage are not available for most variables except for precipitation (for which the meteorological radar offers an hourly reference dataset for 1 km). In DTE Hydrology, we have performed a first validation of satellite data products and modeling results against in situ observations (35). Here we report an additional quality assessment exploiting the most recent version of the DTE Hydrology datacube.
Hydrological validation
This latest assessment of the DTE Hydrology precipitation product was conducted using the data forcing in the semi-distributed conceptual hydrological model known as Modello Idrologico SemiDistribuito in continuo (MISDc) (51, 52). This model allowed us to assess multiple precipitation products by recalibrating it for each forcing dataset (whereas the same process is more challenging for a fully distributed model owing to the high computational burden). Specifically, we used two ground-based datasets: (i) Precipitation observation (Pobs) based on in situ rain gauge data and (ii) modified conditional merging (MCM) based on the integration of data from national meteorological radar and rain-gauge networks. Moreover, four satellite-based products were considered: (i) IMERG Late Run, (ii) SM2RAIN-ASCAT, SM2RASC, (iii) the integration of IMERG and SM2RAIN-ASCAT, i.e., IMERG+SM2RASC, and (iv) the DTE Hydrology product.
The following procedure was used to evaluate the reliability of each product for flood simulation. For each product, the MISDc model was calibrated over the period 2016–2019 and the simulated river discharge time series was compared against the in situ observed data. For simplicity, only 18 of 27 in situ gauging stations available across the Po Basin were considered for this analysis. Through a sequential calibration (53), the MISDc model was calibrated over 11 gauging stations; the remaining seven stations were used for validation.
Figure 4 shows the results (in terms of KGE) for each product and the 18 stations. The overall performances of the in situ data are very good in accordance with Camici et al. (52). While Pobs and MCM show similar median values, MCM performs slightly better. The observed data outperforms the single satellite precipitation data. In particular, among the investigated precipitation products, IMERG shows the lowest median KGE value (0.66) whereas SM2RASC performs better (0.77). Integrating the products improves the flood modeling performance. In particular, the DTE Hydrology product increases the median KGE value to 0.82, a value slightly higher than that obtained using Pobs (0.79) and MCM (0.81). Considering the high density of rain gauge stations in the Po River Basin (640 stations), these results are very promising when striving toward a satellite-based high-resolution (1 km, 1 hour) precipitation product in Europe.
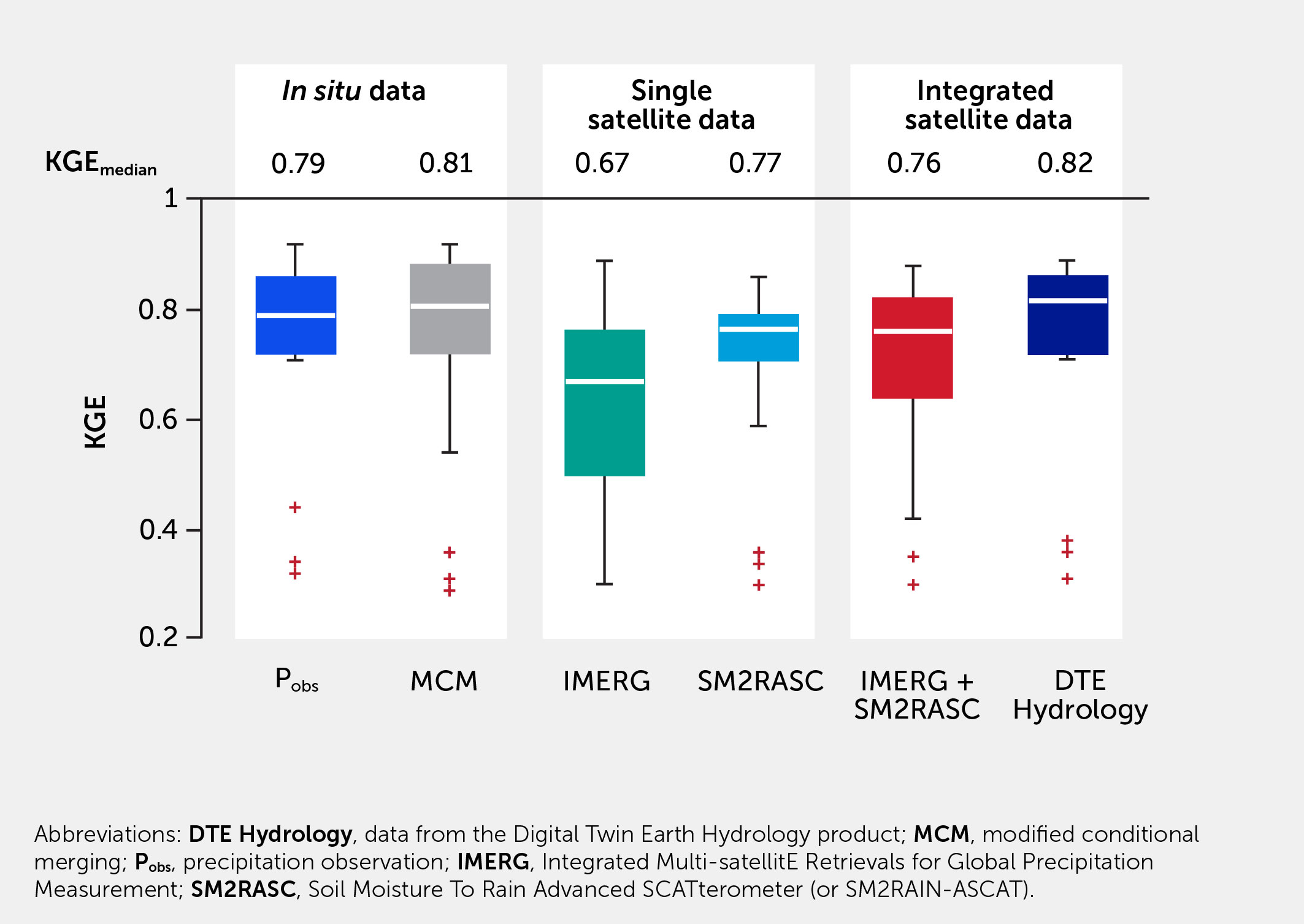
Figure 4 The latest assessment of the Digital Twin Earth (DTE) Hydrology precipitation product for discharge modeling shows very good performance. The figure shows a boxplot of Kling-Gupta Efficiency (KGE) scores computed by comparing observed river discharge for 18 stations with modeled river discharge obtained using different rainfall products as input into the hydrological model MISDc. For the boxplot of each variable, the numbers above each indicate the median KGE value, the buffers indicate the minimum and maximum value, and the three horizontal lines in the rectangle indicate the 25th, 50th, and 75th percentiles.
Irrigation water use
The products contained in the DTE Hydrology datacube have been exploited also for irrigation water assessment. Indeed, the capability to capture irrigation dynamics at high spatial resolution has been investigated through the soil-moisture (SM)-based inversion approach (54–56), which allows backward estimation of irrigation rates from satellite soil moisture data. Such a method has been implemented through RT1 soil moisture, potential evapotranspiration rates from GLEAM, and rainfall from MCM. The first experiment was carried out over a 15 km × 30 km tile north of the city of Reggio Emilia (in the Po River valley), where estimates of irrigation water use at 1 km resolution were produced for the irrigation season of 2018. Figure 5 shows the outcome of this application: the largest water amounts were obtained on the eastern side of the domain, where irrigation amounts >300 mm were retrieved. The product is currently extended to the whole Po Valley as well as the Ebro Basin in Spain and Murray-Darling in Australia; the dataset is freely available, and all details are reported in Dari et al. (57).
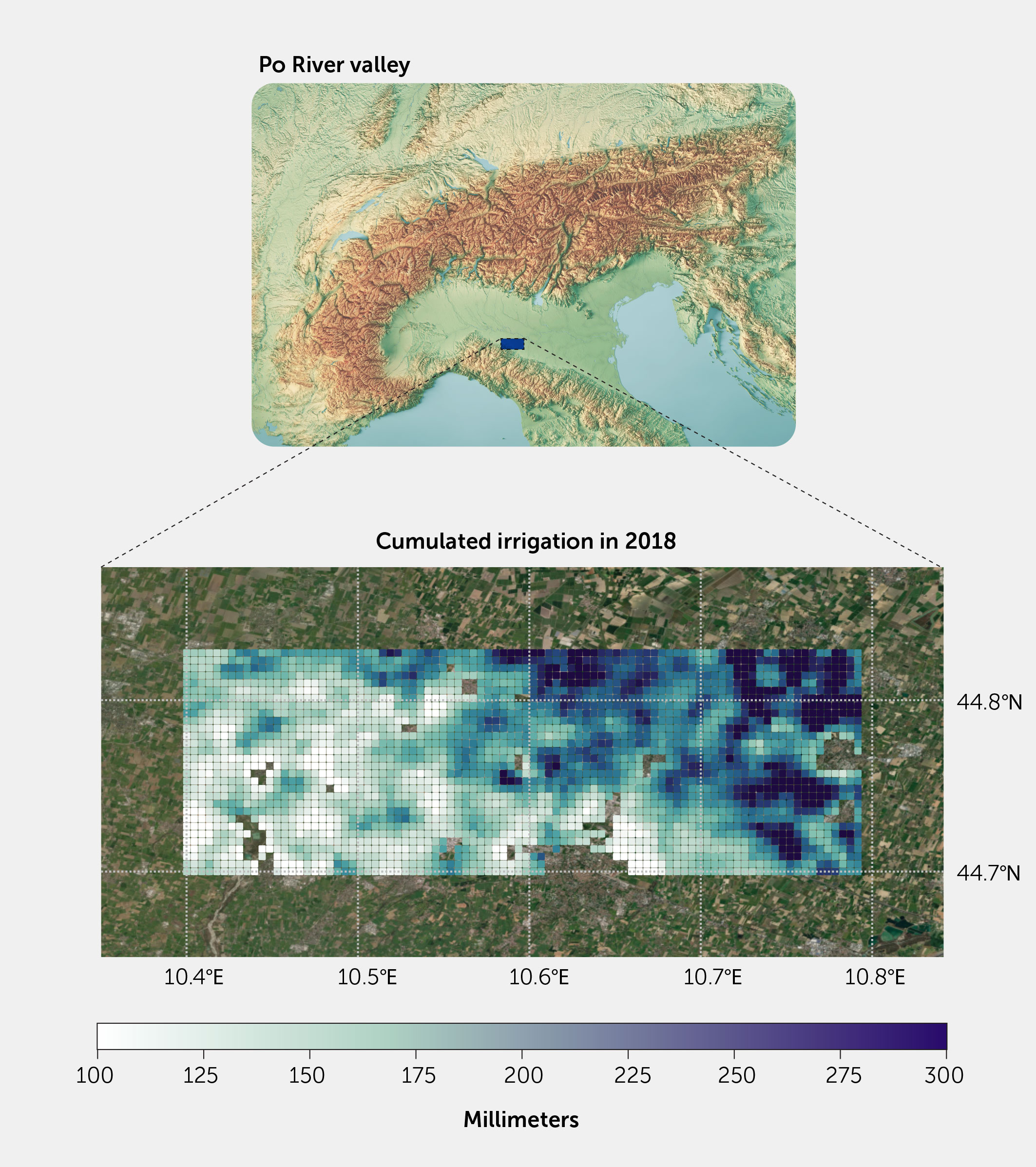
Figure 5 Irrigation water management case study. Cumulated irrigation amounts at 1 km spatial resolution over a portion of the Po River valley, Italy (whose location is indicated on the left side) during 2018.
High-resolution (≤1 km) satellite data available in the Mediterranean Basin provided a clearer spatial match with the extent of agricultural fields, compared with coarse resolution products (54), thereby allowing a more dynamic monitoring of water use for irrigation across fields. However, to advance applications in agriculture, a further improvement in resolution, <100 m, is required.
What-if scenario for flood risk assessment and water resources management
The final results of the DTE Hydrology project have been showcased in the what-if scenarios for flood risk assessment (https://explorer.dte-hydro.adamplatform.eu/?use_case=3) and water resources management (https://explorer.dte-hydro.adamplatform.eu/?use_case=5) (see Figure 6 and the related Video 1 for examples of the visualization of the DTE Hydrology Platform) implemented as a prototype over the Po River Basin (this potentially can be extended over the whole Mediterranean Basin). The what-if scenarios show ease of use and understanding of tools, providing practical information to decision-makers involved in flood risk and water resources management. The full details of the implemented approaches are described in Brocca et al. (58); here, we only summarize the main aspects and functionalities of the developed tools. In both approaches, the high-resolution DTE Hydrology datacube has been integrated into the modular approach by Camici et al. (52), which has been adapted and improved to include the human impact on the water cycle for agricultural, civil, and industrial water use. The modeling approach has been run with a number of different configurations for the input data to obtain a database of simulations describing potential future scenarios for the water cycle.
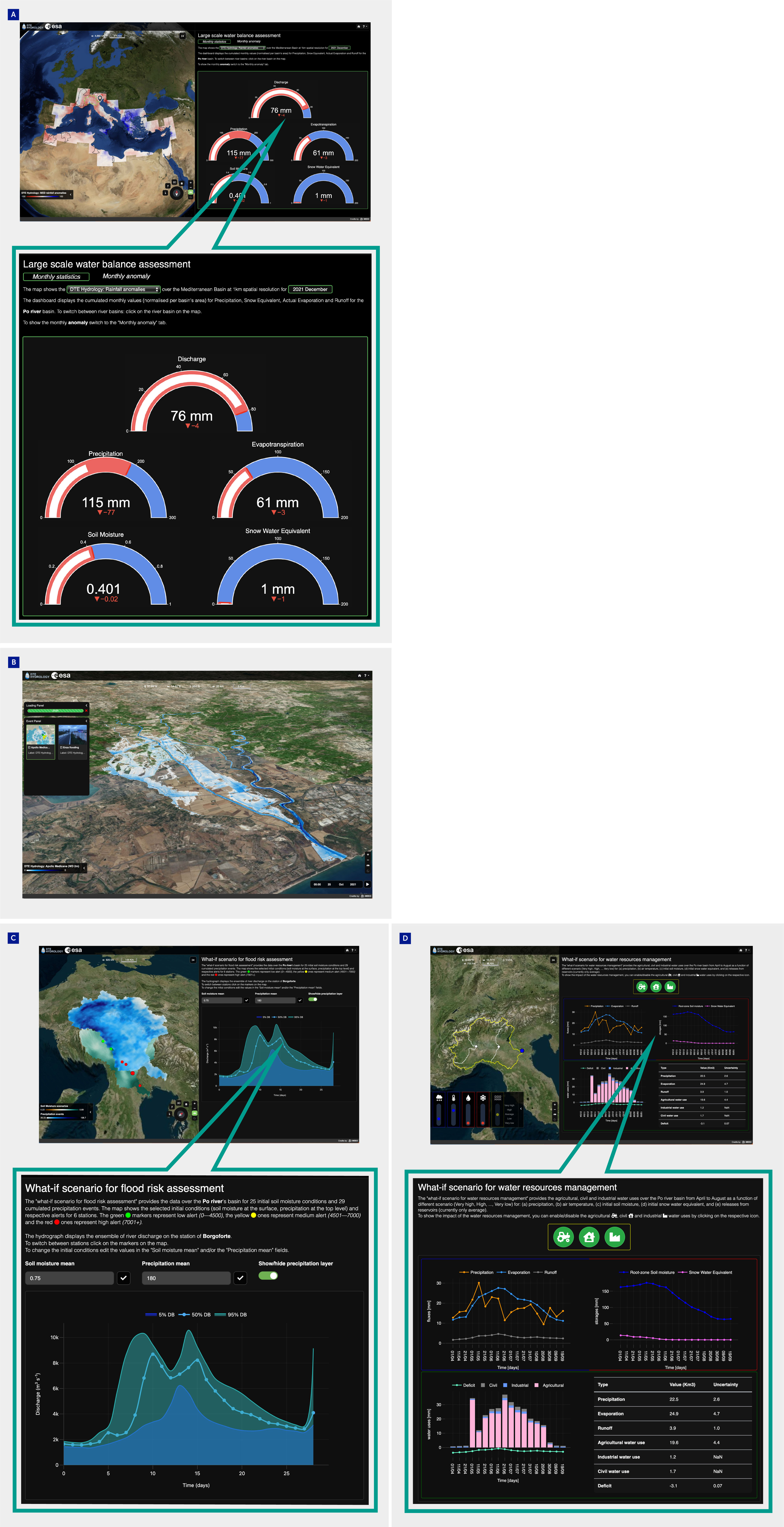
Figure 6 This integrated and interactive system reconstructs and simulates the water cycle and the hydrological processes and interactions with human activities at unprecedented resolutions and accuracies. (A) Large-scale water balance assessment for drought monitoring over the whole Mediterranean Basin; (B) Flooding simulation for the Medicane Apollo, a tropical-like Mediterranean cyclone that occurred in October 2021; (C) What-if scenario for flood risk assessment in the Po River Basin; and (D) What-if scenario for water resources management.
Specifically, the what-if scenario for flood risk assessment provides river discharge forecasts at six different stations along the Po River as a function of predefined conditions for initial soil moisture and 30-day precipitation (see Figure 6C). Similarly, the what-if scenario for water resources management provides 5-month forecasts for the main fluxes (precipitation, evaporation, and runoff), storages (soil moisture and snow water equivalent), and water uses (civil, industrial, and agricultural) as a function of predefined conditions for initial soil moisture and snow water equivalent and 5-month forecasts for precipitation and air temperature (see Figure 6D). We note that for this scenario, satellite observations for precipitation, evaporation, snow water equivalent, surface and root-zone soil moisture, and irrigation water use are used as forcing data and for the calibration of the parameter values of the model. Therefore, the modular hydrological model has been found able to reproduce all the variables of the terrestrial water cycle, not only river discharge as is usually done, thus obtaining a robust tool for decision-making.
Challenges and limitations of EO-based datasets
The key variables that characterize the hydrological cycle of a region include precipitation (rainfall and snowfall), evaporation, soil moisture, snow water equivalent, groundwater storage, and river discharge. The quality and usability of satellite-based hydrological products have recently been enhanced, which is in part thanks to the wealth of new data sources from the Sentinel constellation. Indeed, besides the products used in the DTE Hydrology project, additional high-resolution products for soil moisture [e.g., the plot-scale S2MP dataset (59)] and prototype products for evaporation [e.g., Sen-ET, Guzinski et al. (24) and ECOSTRESS, Fisher et al. (60)] are available, and a large-scale, comprehensive comparison of their characteristics and accuracy is a research priority. Here, we present an overview of the challenges in EO-based datasets and the potential role of AI and machine learning in addressing these gaps (Figure 7).
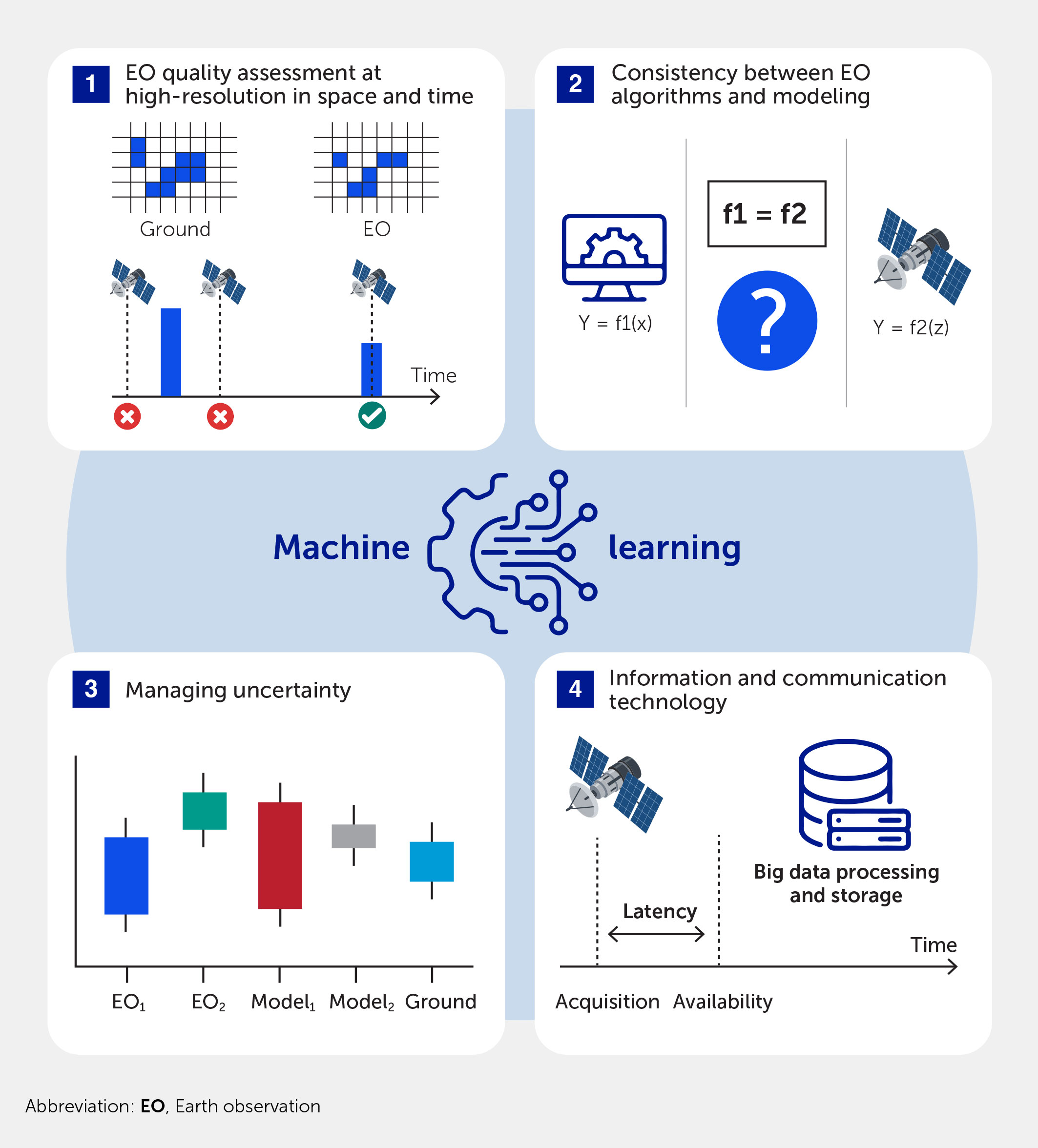
Figure 7 Addressing challenges and limitations in Earth observation (EO)-based datasets toward an improved Digital Twin Earth (DTE) Hydrology. The key challenges for DTE Hydrology are as follows: 1) difficulties in assessing EO products’ quality at high spatial and temporal resolution across different regions and climates; 2) ensuring consistency between satellite data retrieval algorithms and modeling at high resolution; 3) managing the inherent uncertainties in the accuracy of data from EO and model products; and 4) availability of information and communication infrastructures that could support higher computational capacity while optimizing data latency and allowing long time series. Artificial intelligence and machine learning techniques will play a vital role in addressing these challenges.
How do we assess the quality of high-resolution products?
The high-resolution EO-based products developed within the last couple of years under the DTE Hydrology project are among the most advanced satellite-based hydrological products currently available. These have been validated for the first time during the DTE Hydrology project but over a limited region (70,000 km2) and temporal span (4 years). Therefore, all products need to be tested further in different regions and climates to comprehensively assess their quality, reliability, and usability for high-resolution hydrological applications.
The stringent tests necessary to assess these products’ performance at high spatial and temporal resolutions are very challenging owing to the unavailability of ground observations at relevant spatial and temporal scales (except for precipitation in areas covered by meteorological radars). Indirect assessment and novel approaches must therefore be developed [e.g., Crow et al. (61)], for instance, by applying the data in case studies for which high spatial and/or temporal resolution is mandatory.
A soil moisture or evaporation product with a spatial resolution of 1 km should be able to distinguish between the water variability of neighboring agricultural fields that might reflect irrigation practices. To correctly identify irrigated fields, the spatial resolution of EO-based products must be consistent with the extent of irrigated fields (62–64). Similarly, high-resolution soil moisture products can be tested in areas affected by fires, as their occurrence significantly changes the hydrological regime at high spatial resolution [(e.g., by forming a crust soil layer at a shallow depth, reducing infiltration rates/capacity (65)]. These changes in the hydrological regime should be distinguished when high-resolution products are considered.
An issue related to high resolution in space is the need for dense temporal sampling for processes characterized by high temporal variability (e.g., precipitation and flash flooding). Indeed, when studying processes occurring at small scales, we must complement the high spatial resolution (e.g., 1 km) with a high temporal resolution (sub-daily or even hourly). For instance, data at high spatial resolution allow us to investigate not only large river flooding but also flash flooding occurring over small basins. Hourly or sub-hourly data are necessary for this application as these events develop quickly and occur over very short time periods. Zappa et al. (64) have underlined the need for high temporal resolution also for detecting irrigation events and particularly for estimating irrigation water amounts.
High-resolution products may also be assessed by comparison with high-resolution modeling. For example, Gruber et al. (66) have highlighted the key role of land surface model outputs in the evaluation of coarse-resolution satellite soil moisture products. In this context, a good validation practice for high-resolution datasets can indeed benefit from model simulations at high spatial resolution. However, while models can provide regional- or global-scale simulations at the desired temporal and spatial resolution, they are characterized by representativeness errors owing to input data, such as the original spatial resolution of meteorological forcing data and errors in model parameterization Gruber et al. (66). In particular, the latter implies uncertainties in soil texture and vegetation/crop type parameterizations as well as outdated input information about anthropogenic activities (e.g., areas equipped for irrigation; irrigation maps), which can critically affect high spatial resolution outputs (67, 68). All those limitations affect the accuracy of modeled products and should be considered when assessing high-resolution satellite products.
Consistency within retrieval algorithms and modeling
Importantly, when assessing high-resolution satellite products or developing high-resolution models, one cannot merely scale up the computer power and apply algorithms developed at coarse spatial scales. The algorithms must be advanced to make them fit for the increased physical complexity at the finer scales. This is due to the need to consider processes that (i) were not previously included, and (ii) require more complex parameterization to account for a wide range of soil, topography, and land use types. As a consequence, the algorithms become ever more data intensive, necessitating urgent work toward the integration of satellite data streams, combining data from different spectral bands, measurement techniques, and processing levels. Leveraging these data will require the creation of multidimensional datacube systems that allow us to develop advanced satellite data retrieval algorithms and to connect the different data streams with the models. For example, global applications of high-resolution Copernicus data require systems connecting existing analysis-ready datacubes for Sentinel-1 (69) and Sentinel-2 (70).
Moreover, consistency should be ensured between EO-based products and hydrological/land surface modeling. This can be achieved in two different steps. The first step would require that the same ancillary datasets (e.g., land use and land cover, soil texture, and topography) are used in the modeling and the retrieval algorithms of satellite products. The second step involves the development of physically based models linking high-resolution satellite observations from across the entire electromagnetic spectrum to hydrological models. This would not only help to better exploit the synergistic physical information content of satellite observations from the optical, infrared, and microwave domains but would eventually also lead to better consistency within and between the different data records. These two targets are potentially achievable, but they require a strong collaboration between the modeling and the remote sensing communities to integrate their expertise.
We would like also to underline a potential counterpoint regarding the relative merit of consistency. If models and remote sensing retrieval algorithms use the same static spatial inputs, their errors will be spatially correlated, leading to spurious agreement between modeled and remotely sensing products. Upon evaluation, independence may thus often be more desired than consistency.
Managing uncertainty in EO and model products
Uncertainties inherently affect both EO and model products’ accuracy. On the one hand, models are imperfect representations of reality with uncertainties reflecting the model physics’ description, parameterization and input meteorological forcings, initial conditions, and unresolved scales (71, 72). On the other hand, EO is characterized by sensor, retrieval, and representativeness errors (66, 73, 74). Therefore, the integration of EO and models through merging applications strongly depends on the characterization of the uncertainties in each (75).
Data assimilation is one of the most viable ways to optimally integrate EO and models. Through model state update and/or parameter estimation, data assimilation allows geophysical quantities to be modeled more accurately and enables the improvement of initial conditions for subsequent hydrometeorological forecasts (72, 74). However, the robustness of any data assimilation system depends on an optimal representation of the observation and forecast error covariances. In this context, the observation error must account not only for instrument errors but also errors in the observation operator and in the interpolation of the observations, generally defined as representativeness errors (76). Because the error characterization relies on high-quality benchmark data from ground observations, its correct representation either in satellite data or models is not always guaranteed. To circumvent this problem, many researchers have proposed error characterization strategies that compare multiple datasets of the same variable provided that these datasets satisfy certain mathematical requirements, such as the independence of their errors (77–80). Therefore, temporally and spatially collocated model- and EO-based time series can provide a robust error characterization for use in data assimilation experiments (23, 81, 82).
The treatment of bias is another important aspect, considering that most data assimilation methods assume that sources of information are unbiased (72, 76). Bias arises when the underlying assumptions of retrieval models are different from those used by hydrological and land surface models. Researchers have reported many attempts to correct this bias, most notably by matching the cumulative distribution function (CDF) between assimilated observations and model simulations (73, 83). To better deal with the bias with respect to the model state, spectral signatures of solar and Earth-emitted radiation can be assimilated by directly equipping models with calibrated forward operator components (e.g., based on inverse radiative transfer models or machine learning) (47, 67, 84, 85).
Information and communication technology
Data latency (i.e., the total time elapsed between data acquisition by a sensor and its availability) is an important limitation of EO-based datasets for some applications. For instance, the <1 hour latency required for early warning systems in developed countries is hardly achievable from remote-sensing observations. In contrast, the longer latency (≥1 day) required in developing countries, due to technological limitations in data collection and sharing, is achievable, and hence the potential of EO-based datasets should be exploited in these regions. Moreover, the exponential growth of data volumes, combined with the possible integration and fusion of cross-satellite missions, are driving users to request long time series. This is likely to increase even more in the future, with a substantial impact on both access and computational performances. Indeed, in some regions the need for increasingly data-intensive analyses is likely to cause problems with access to the necessary computational resources and performance levels.
A new paradigm aims to overcome the limits of computational capacity, and thus exploit the full potential of EO data, by providing centralized data access and a cloud environment to facilitate the implementation of scalable processing services. This paradigm involves providing access to large spatiotemporal data stored in data archives—both public [e.g., Data and Information Service (DIAS), European Weather Cloud (EWC), and European Open Science Cloud (EOSC)] and commercial [e.g., Amazon Web Services (AWS), Google Earth Engine, Microsoft Azure, CloudFerro, Earth Observation Data Centre (EODC), openEO Platform, and the Advanced geospatial Data Management (ADAM) platform]. In addition, several different platforms have been developed to simplify the use of these infrastructures and data access.
In parallel to this technology evolution at the infrastructure and platform level, data providers and space industries are focused on the generation of analysis ready data (ARD) and the evolution of data exploitation service components (e.g., Cloud Optimized GeoTIFF; datacube services) to better support and improve the implementation of algorithms by the science community.
However, an efficient, global, near-real time processing environment that could continuously provide higher-level data products on top of the acquired satellite data might require a specifically designed IT infrastructure optimized for the data acquisition, ARD, and product processing and data distribution. A first practical example for global near-real time emergency support from EO data has been published recently within the Copernicus Emergency Management Service. The GloFAS Global Flood Monitoring (GFM) service provides a worldwide flood monitoring service by immediately processing and analyzing all incoming Copernicus Sentinel-1 Synthetic Aperture Radar (SAR) satellite data (https://www.globalfloods.eu/technical-information/glofas-gfm/).
Role of AI/machine learning techniques
Recent years have seen a continuous and rapid development of new artificial intelligence (AI) and machine learning techniques. These are expected to improve the outcomes of the DTE Hydrology project and will be tested and improved in future developments (14). Beyond the standard applications of AI/machine learning in the hydrological sciences (e.g., in parameter retrieval, data assimilation and fusion, downscaling, and anomaly detection), a few opportunities are particularly relevant in the context of the DTE:
● Improved parameter estimates. AI/machine learning can help improve retrievals and make them faster as well as aid in deploying forward operators in data assimilation schemes, where complex interactions between the state of the land surface and observations cannot yet be physically modeled. Data acquisition schemes are closely related to data-model integration and fusion; a wealth of simulated data available through physical models such as radiative transfer models (RTMs) is becoming increasingly available, and blending real and simulated data will help avoid overfitting in observation-scarce regimes while being consistent and allowing for extrapolation (86).
● Toward robust predictions. In addition to fitting observations, AI/machine learning can also help elucidate poorly understood processes. The paradigm of hybrid machine learning (13, 86) aims to combine well-established governing laws and principles with the flexibility of data-driven machine learning approaches, thereby allowing latent functions and driving forces, and their parameters, to be determined. Physics-aware machine learning thus leads to enhanced computational efficiency and constitutes a stepping stone toward more interpretable and robust machine learning models (87).
● Emulating costly models. Another obvious form of integration of AI in a DTE is through developing emulators (88). Emulators have become important tools for researchers dealing with computationally heavy models in many fields and in the remote-sensing and geoscience communities (89). Emulators are essentially machine learning algorithms that provide fast approximations to complex physical (radiative transfer, Earth, or climate) models—an approach with a long history in statistics. These surrogate models or metamodels are generally orders of magnitude faster than the original models and hence can be substituted for these, opening the door to more advanced biophysical parameter estimation methods.
● Explainability and counterfactuals. An interesting future possibility of AI is to answer questions about the systems—questions not related to the “what?” but to the “why?” and the “what if?” Answering the “why?” question requires explainable AI (XAI) modules, i.e., tools that can interpret what the AI models learned, and a certain interaction with the users (87). Beyond interpreting AI models, one may want to imagine scenarios and assess risks. XAI can help to formulate hypotheses that go beyond human insights and so indirectly improve the DTE implementation. This is intrinsically related to the challenging concepts of causal inference, causal impact quantification and assessment, and counterfactuals, where some statistical learning and AI techniques promise advances (90, 91). Being able to run on-demand models and what-if scenarios to simulate the sensitivity of some hydrological parameters and/or variables to atypical and hypothesized conditions might potentially be a paradigm shift. These what-if games with data and models could enable users to assess the impact of factors such as climate change, pollution, or severe droughts.
Future satellite missions and concepts
In the longer term, new satellite observations will further extend our ability to reproduce the terrestrial water cycle at high resolution. Specifically, the following missions/products are expected:
● L-band (and C-band) future missions. By integrating Sentinel-1 with the upcoming missions like the National Aeronautics and Space Administration-Indian Space Research Organisation (NASA-ISRO) Synthetic Aperture Radar (NISAR) mission and the Radar Observation System for Europe at L-band (ROSE-L) synthetic aperture radar (SAR) mission, the spatiotemporal sampling and accuracy of soil moisture, water extent, and other hydrological data products (e.g., flooded areas) could be significantly improved. This would directly lead to major improvements in level 3 and 4 products for rainfall and root zone soil moisture. The integration will be additionally guaranteed by the future launch of Sentinel-1C and Sentinel-1D satellites, which will extend the Sentinel-1 mission at least until 2030, making available a long-term backscatter dataset that will be useful for several hydrological applications.
● High-resolution thermal missions. Within the Copernicus program, the Land Surface Temperature Mission (LSTM) will complement Sentinel observation capabilities with high spatiotemporal resolution thermal infrared observations over land and coastal regions in support of agriculture management services and possibly other applications and services. The primary objective is to enable monitoring of the evaporation rates at European field scales by capturing high spatial and temporal fluctuations in land surface temperature. Notably in this context, the high-resolution Thermal Imaging Satellite for High-resolution Natural resource Assessment (TRISHNA) mission led by the French Centre National d’Études Spatiales (CNES) and the Indian Space Research Organisation (ISRO) is scheduled for launch in 2025 (92).
● Global Navigation Satellite System (GNSS) reflectometry. GNSS reflectometry is increasingly seen as a valuable alternative to SMOS and SMAP to collect L-band data for the monitoring of soil moisture, water bodies, freeze/thaw status, and flooded areas. For example, the ESA recently approved the Scout Mission HydroGNSS (93). However, the development of robust data products will probably be more challenging for this bi-static active measurement technique than for both passive (SMOS, SMAP) and mono-static active (ASCAT, Sentinel-1, etc.) sensing approaches.
● Terrestrial water storage and groundwater dynamic. The next-generation gravity missions (currently named Mass change and Geoscience International Constellation; MAGIC) (94) are expected to significantly improve the temporal and spatial resolution of water storage measurements from space (weekly, <100 km), thus providing new observations for hydrological modeling and prediction in medium scale basins.
● Geosynchronous radar missions. Geosynchronous radar missions would have a huge potential to study dynamic hydrological processes at sub-daily time intervals. Unfortunately, after the deselection of the Hydroterra mission (one of the candidate missions for ESA’s 10th Earth Explorer) there are no concrete plans for such a mission in Europe. However, a Chinese mission is under development (95).
● High-resolution optical platforms. Further advances in the field of global terrestrial evaporation monitoring may involve developments in high-resolution optical platforms (96) and ongoing and future thermal missions such as ECOSTRESS (60) and TRISHNA (97). Moreover, the use of CubeSat data, e.g., from the Planet constellation (98), has already demonstrated a high potential for monitoring evaporation at agricultural field scales (99). Whether current evaporation models (such as e.g., GLEAM) are suited to extract the intrinsic value of these high-resolution observations, or whether models specifically dedicated to estimating evaporation over agricultural fields are more adequate for this task, remains to be answered.
● Snow cover and snow depth. The Sentinel-3b satellite will soon provide snow coverage at 300 m spatial resolution and daily frequency. This is a step change compared to the revisit time of Sentinel-2, which is between 3 and 6 days. Moreover, the assimilation of C-SNOW data (from Sentinel-1) into hydrological modeling has shown the potential of snow depth for the retrieval of snow water equivalent. C-SNOW is not operational, but such products are extremely valuable for next-generation mountain hydrology.
● Meteosat Third Generation (MTG) and EUMETSAT Polar System-Second Generation (EPS-SG) missions. The MTG mission, launched in December 2022, and the EPS-SG mission, planned for launch in early 2025, will deliver novel data with significant potential to advance weather, climate, and Earth system research as well as enhance operational forecasting. Providing next-generation precipitation, snow, and soil moisture products at improved resolution (<5 km), these missions will be highly valuable in the development of the DTE for the terrestrial water cycle.
● The Surface Water and Ocean Topography satellite (SWOT) mission. The SWOT mission launched in December 2022, will enable scientists to measure and track the elevation, extent, and movement of water across the planet in ground-breaking detail (100). This will provide a wealth of data for testing and validating the results of the DTE Hydrology modeling systems.
Toward a fully operational Digital Twin Earth of the terrestrial water cycle
Although high-resolution hydrological modeling and observations offer valuable opportunities both for future research and operational applications, substantial challenges remain to be addressed. The most important high-level challenges are as follows:
1. In situ observations and satellite-based datasets (e.g., precipitation, evaporation, soil moisture, river discharge, and snow) must be available not only at the high spatial and temporal resolution but also with sufficient accuracy and appropriate uncertainty characterization.
2. The representation of physical processes (e.g., infiltration, surface and subsurface runoff, plant-atmosphere interaction, and groundwater dynamic) at high resolution is different from processes at coarse spatial resolution (20 km), as currently modeled by continental and global scale land surface and hydrological models. The hydrological community, and specifically the experimental hydrology field, has considerable expertise in the representation of physical processes at local and small scales. Experimentalist hydrologists and regional/global modelers must now collaborate to develop a modeling system that is reliable across multiple spatial scales.
3. Human impacts on the water cycle (e.g., through irrigation, reservoir management, flood protections, and river water diversion), occurring at very high-resolution, challenge current attempts to reproduce a digital replica of the Earth. EO will be crucial in providing information on the human impact on the water cycle, and such data therefore need to be optimally and efficiently integrated into the modeling system.
4. An information and communications technology infrastructure allowing users (scientists, stakeholders, and citizens) to easily interact with data and models needs to be properly designed, scaled, and implemented. This infrastructure should be an interoperable, modular, and seamless cloud-based web service (34, 101) embodying an open data approach based on the FAIR (Findability, Accessibility, Interoperability, and Reusability) principles in order to allow its maximal use and benefit. Crucially, current computational capabilities are far from being sufficient to develop high-resolution hydrological systems at a continental or a global scale, necessitating investment in suitable infrastructures and expertise.
Conclusion
Advancing toward a DTE for the terrestrial water cycle, and specifically extending DTE Hydrology across the Mediterranean area and then throughout Europe, will require a significant effort to address current knowledge gaps and technological challenges. We must develop both the technological infrastructures and scientific expertise that will allow us to create robust systems that can reliably predict the water cycle and extreme events. The DTE Hydrology project and other ESA and NASA initiatives are making significant progress in developing the first high-resolution EO-based products for soil moisture, precipitation, evaporation, snow water equivalent, and river discharge over large regions. The datasets are now freely available in the DTE Hydrology datacube (https://viewer.earthsystemdatalab.net/?dataset=hydrology), allowing scientists to further advance the knowledge of the water cycle toward a full DTE for this critical system. Specifically, in the DTE Hydrology project, new case studies in southern Italy (Medicane Apollo) and over the full Mediterranean Basin have been developed and made openly available in the DTE Hydrology Platform: https://explorer.dte-hydro.adamplatform.eu/.
Related content
Video 1 | An overview of the Digital Twin Earth (DTE) Hydrology Platform. This openly available digital twin of the terrestrial water cycle reconstructs and simulates hydrological processes and human interactions at unprecedented resolution and accuracy. The video showcases four case studies and what-if scenarios available on the platform, for flood and landslide risk assessment and water resources management in the Mediterranean Basin. Accessible at: https://www.youtube.com/watch?v=EiGm_vAV9YE.
Statements
Author contributions
LB: Writing – original draft, Writing – review & editing, Conceptualization, Data curation, Funding acquisition, Project administration, Visualization. SB: Writing – review & editing, Methodology. SC: Writing – original draft, Writing – review & editing, Methodology. LC: Writing – review & editing, Data curation. JD: Writing – original draft, Writing – review & editing, Data curation, Validation. PF: Writing – review & editing, Data curation, Validation. CM: Writing – review & editing, Data curation, Funding acquisition. SM: Writing – review & editing, Data curation, Resources, Validation. AT: Writing – review & editing, Data curation. BB: Writing – review & editing, Methodology. HM: Writing – review & editing, Validation. WW: Writing – original draft, Writing – review & editing, Data curation. MV: Writing – review & editing, Data curation, Validation. RQ: Writing – original draft, Writing – review & editing, Data curation, Validation. LA: Writing – original draft, Writing – review & editing, Methodology. SG: Writing – review & editing, Methodology. FA: Writing – review & editing, Methodology, Validation. DR: Writing – review & editing, Data curation, Validation. DM: Writing – original draft, Writing – review & editing. SM: Writing – review & editing. CB: Writing – review & editing, Project administration, Resources. AD: Writing – review & editing, Methodology. AJ: Writing – review & editing, Data curation. MC: Writing – review & editing, Data curation, Validation. GC-V: Writing – original draft, Writing – review & editing. EV: Writing – review & editing, Supervision. DF: Writing – review & editing, Supervision.
Data availability statement
The raw data supporting the conclusions of this article will be made available by the authors, without undue reservation.
Funding
The author(s) declare that financial support was received for the research, authorship, and/or publication of this article. We would like to acknowledge the support of the ESA projects DTE Hydrology (Contract no. ESA 4000129870/20/I-NB - CCN N. 1), DTE Hydrology Evolution (Contract no. ESA 4000136272/21/I-EF - CCN N. 1), 4D MED Hydrology (Contract no. ESA 4000136272/21/I-EF), and Irrigation+ (Contract no. ESA 4000129870/20/I-NB).
Conflict of interest
The author SM is employed by Meteorological Environmental Earth Observation s.r.l. The author CB is employed by Earth Observation Data Centre for Water Resources Monitoring GmbH. These companies had no involvement in the study and did not provide funding.
The funders listed under “Funding” had the following involvement with the study: supervision of the research activities, editing of the manuscript, discussion and interpretation of the results. The Frontiers Editorial Office assisted in the conceptualization, interpretation, and design of the figures in this article.
The authors LB, SC, LC, JD, CM, SM, AT, MV, LA, DR, DM declared that they were an editorial board member of Frontiers, at the time of submission. This had no impact on the peer review process and the final decision.
Publisher’s note
All claims expressed in this article are solely those of the authors and do not necessarily represent those of their affiliated organizations, or those of the publisher, the editors and the reviewers. Any product that may be evaluated in this article, or claim that may be made by its manufacturer, is not guaranteed or endorsed by the publisher.
References
1. Douville H, Raghavan K, Renwick J, Allan RP, Arias PA, Barlow M, et al. Water cycle changes. In: Masson-Delmotte V, Zhai P, Pirani A, Connors SL, Péan C, Berger S, Caud N, Chen Y, Goldfarb L, Gomis MI, Huang M, Leitzell K, Lonnoy E, Matthews JBR, Maycock TK, Waterfield T, Yelekçi O, Yu R, Zhou B, editors. Climate Change 2021: The Physical Science Basis. Contribution of Working Group I to the Sixth Assessment Report of the Intergovernmental Panel on Climate Change. Cambridge, United Kingdom and New York, NY, USA: Cambridge University Press (2021) 1055–210. doi: 10.1017/9781009157896.010
2. Caretta MA, Mukherji A, Arfanuzzaman M, Betts RA, Gelfan A, Hirabayashi Y, et al. Water. In: Pörtner H-O, Roberts DC, Tignor M, Poloczanska ES, Mintenbeck K, Alegría A, Craig M, Langsdorf S, Löschke S, Möller V, Okem A, Rama B, editors. Climate change 2022: impacts, adaptation and vulnerability. contribution of working group II to the Sixth Assessment Report of the Intergovernmental Panel on Climate Change. Cambridge, UK: Cambridge University Press (2022) 551–712. doi: 10.1017/9781009325844.006
3. Wood EF, Roundy JK, Troy TJ, van Beek LPH, Bierkens MFP, Blyth E, et al. Hyperresolution global land surface modeling: meeting a grand challenge for monitoring earth’s terrestrial water. Water Resour Res (2011) 47(5). doi: 10.1029/2010WR010090
4. Beven KJ, Cloke HL. Comment on “Hyperresolution global land surface modeling: meeting a grand challenge for monitoring Earth’s terrestrial water” by Eric F. Wood et al. Water Resour Res (2012) 48(1):W01801. doi: 10.1029/2011WR010982
5. Bierkens MFP, Bell VA, Burek P, Chaney N, Condon LE, David CH, et al. Hyper-resolution global hydrological modelling: what is next? Hydrol Process (2015) 29(2):310–20. doi: 10.1002/hyp.10391
6. Wada Y, Bierkens MF, Roo A Wada Y, Bierkens MFP, de Roo A, Dirmeyer PA, et al. Human–water interface in hydrological modelling: current status and future directions. Hydrol Earth Syst Sci (2017) 21(8):4169–93. doi: 10.5194/hess-21-4169-2017
7. Nguyen-Quang T, Polcher J, Ducharne A, Arsouze T, Zhou X, Schneider A, et al. ORCHIDEE-routing: revising the river routing scheme using a high-resolution hydrological database. Geosci Model Dev (2018) 11(12):4965–85. doi: 10.5194/gmd-11-4965-2018
8. Fisher RA, Koven CD. Perspectives on the future of land surface models and the challenges of representing complex terrestrial systems. J Adv Model Earth Syst (2020) 12(4):MS001453:e2018. doi: 10.1029/2018MS001453
9. Beck HE, van Dijk AIJM, de Roo A, Dutra E, Fink G, Orth R, et al. Global evaluation of runoff from 10 state-of-the-art hydrological models. Hydrol Earth Syst Sci (2017) 21(6):2881–903. doi: 10.5194/hess-21-2881-2017
10. Telteu CE, Müller Schmied H, Thiery W, Leng G, Burek P, Liu X, et al. Understanding each other’s models: an introduction and a standard representation of 16 global water models to support intercomparison, improvement, and communication. Geosci Model Dev (2021) 14(6):3843–78. doi: 10.5194/gmd-14-3843-2021
11. Hoch JM, Sutanudjaja EH, Wanders N, Van Beek RLPH, Bierkens MFP. Hyper-resolution PCR-GLOBWB: opportunities and challenges from refining model spatial resolution to 1 km over the European continent. Hydrol Earth Syst Sci (2023) 27(6):1383–401. doi: 10.5194/hess-27-1383-2023
12. Naz BS, Sharples W, Ma Y, Goergen K, Kollet S. Continental-scale evaluation of a fully distributed coupled land surface and groundwater model, ParFlow-CLM (v3.6.0), over Europe. Geosci Model Dev (2023) 16(6):1617–39. doi: 10.5194/gmd-16-1617-2023
13. Reichstein M, Camps-Valls G, Stevens B, Jung M, Denzler J, Carvalhais N, et al. Deep learning and process understanding for data-driven Earth system science. Nature (2019) 566(7743):195–204. doi: 10.1038/s41586-019-0912-1
14. Schneider R, Bonavita M, Geer A, Arcucci R, Dueben P, Vitolo C, et al. ESA-ECMWF Report on recent progress and research directions in machine learning for Earth System observation and prediction. NPJ Clim Atmos Sci (2022) 5(1):51. doi: 10.1038/s41612-022-00269-z
15. Kraft B, Jung M, Körner M, Koirala S, Reichstein M. Towards hybrid modeling of the global hydrological cycle. Hydrol Earth Syst Sci (2022) 26(6):1579–614. doi: 10.5194/hess-26-1579-2022
16. Koppa A, Rains D, Hulsman P, Poyatos R, Miralles DG. A deep learning-based hybrid model of global terrestrial evaporation. Nat Commun (2022) 13(1):1912. doi: 10.1038/s41467-022-29543-7
17. El Hajj M, Baghdadi N, Zribi M, Bazzi H. Synergic use of Sentinel-1 and Sentinel-2 images for operational soil moisture mapping at high spatial resolution over agricultural areas. Remote Sens (2017) 9(12):1292. doi: 10.3390/rs9121292
18. Bauer-Marschallinger B, Freeman V, Cao S, Paulik C, Schaufler S, Stachl T, et al. Toward global soil moisture monitoring with Sentinel-1: harnessing assets and overcoming obstacles. IEEE Trans Geosci Remote Sens (2019) 57(1):520–39. doi: 10.1109/TGRS.2018.2858004
19. Greifeneder F, Khamala E, Sendabo D, Wagner W, Zebisch M, Farah H, et al. Detection of soil moisture anomalies based on Sentinel-1. Phys Chem Earth Parts A B C (2019) 112:75–82. doi: 10.1016/j.pce.2018.11.009
20. Balenzano A, Mattia F, Satalino G, Lovergine FP, Palmisano D, Peng J, et al. Sentinel-1 soil moisture at 1 km resolution: a validation study. Remote Sens Environ (2021) 263:112554. doi: 10.1016/j.rse.2021.112554
21. Peng J, Albergel C, Balenzano A, Brocca L, Cartus O, Cosh MH, et al. A roadmap for high-resolution satellite soil moisture applications – confronting product characteristics with user requirements. Remote Sens Environ (2021) 252:112162. doi: 10.1016/j.rse.2020.112162
22. Castelli M, Asam S, Jacob A, Zebisch M, Notarnicola C. Monitoring daily evapotranspiration in the Alps exploiting Sentinel-2 and meteorological data. In: Remote Sensing and Hydrology Symposium (RSHS) 2018 (2018).
23. Martens B, De Jeu RA, Verhoest NE, Schuurmans H, Kleijer J, Miralles DG. Towards estimating land evaporation at field scales using GLEAM. Remote Sens (2018) 10(11):1720. doi: 10.3390/rs10111720
24. Guzinski R, Nieto H, Sandholt I, Karamitilios G. Modelling high-resolution actual evapotranspiration through Sentinel-2 and Sentinel-3 Data Fusion. Remote Sens (2020) 12(9):1433. doi: 10.3390/rs12091433
25. Karger DN, Wilson AM, Mahony C, Zimmermann NE, Jetz W. Global daily 1 km land surface precipitation based on cloud cover-informed downscaling. Sci Data (2021) 8(1):307. doi: 10.1038/s41597-021-01084-6
26. Filippucci P, Brocca L, Quast R, Ciabatta L, Saltalippi C, Wagner W, et al. High-resolution (1 km) satellite rainfall estimation from SM2RAIN applied to Sentinel-1: Po River basin as a case study. Hydrol Earth Syst Sci (2022) 26(9):2481–97. doi: 10.5194/hess-26-2481-2022
27. He K, Zhao W, Brocca L, Quintana-Seguí P. SMPD: A soil moisture-based precipitation downscaling method for high-resolution daily satellite precipitation estimation. Hydrol Earth Syst Sci (2022) 27(1):169–90. doi: 10.5194/hess-27-169-2023
28. Lievens H, Demuzere M, Marshall HP, Reichle RH, Brucker L, Brangers I, et al. Snow depth variability in the Northern Hemisphere mountains observed from space. Nat Commun (2019) 10(1):4629. doi: 10.1038/s41467-019-12566-y
29. Shi Z, Chen Y, Liu Q, Huang C. Discharge estimation using harmonized landsat and Sentinel-2 product: case studies in the Murray Darling Basin. Remote Sens (2020) 12(17):2810. doi: 10.3390/rs12172810
30. Tarpanelli A, Camici S, Nielsen K, Brocca L, Moramarco T, Benveniste J. Potentials and limitations of Sentinel-3 for river discharge assessment. Adv Space Res (2021) 68(2):593–606. doi: 10.1016/j.asr.2019.08.005
31. Filippucci P, Brocca L, Bonafoni S, Saltalippi C, Wagner W, Tarpanelli A. Sentinel-2 high-resolution data for river discharge monitoring. Remote Sens Environ (2022) 281:113255. doi: 10.1016/j.rse.2022.113255
32. Jaafar H, Mourad R, Schull M. A global 30-m ET model (HSEB) using harmonized Landsat and Sentinel-2, MODIS and VIIRS: comparison to ECOSTRESS ET and LST. Remote Sens Environ (2022) 274:112995. doi: 10.1016/j.rse.2022.112995
33. Riggs RM, Allen GH, David CH, Lin P, Pan M, Yang X, et al. RODEO: an algorithm and Google Earth Engine application for river discharge retrieval from Landsat. Environ Modell Softw (2022) 148:105254. doi: 10.1016/j.envsoft.2021.105254
34. Rigon R, Formetta G, Bancheri M, Tubini N, D’Amato C, David O, et al. HESS Opinions: Participatory Digital eARth Twin Hydrology systems (DARTHs) for everyone – a blueprint for hydrologists. Hydrol Earth Syst Sci (2022) 26:4773–800. doi: 10.5194/hess-26-4773-2022
35. Alfieri L, Avanzi F, Delogu F, Gabellani S, Bruno G, Campo L, et al. High-resolution satellite products improve hydrological modeling in northern Italy. Hydrol Earth Syst Sci (2022) 26(14):3921–39. doi: 10.5194/hess-26-3921-2022
36. Tarpanelli A, Bonaccorsi B, Sinagra M, Domeneghetti A, Brocca L, Barbetta S. Flooding in the Digital Twin Earth: the case study of the Enza river levee breach in December 2017. Water (2023) 15(9):1644. doi: 10.3390/w15091644
37. Quast R, Albergel C, Calvet JC, Wagner W. A generic first-order radiative transfer modelling approach for the inversion of soil and vegetation parameters from scatterometer observations. Remote Sens (2019) 11(3):285. doi: 10.3390/rs11030285
38. Muñoz-Sabater J, Dutra E, Agustí-Panareda A, Albergel C, Arduini G, Balsamo G, et al. ERA5-Land: a state-of-the-art global reanalysis dataset for land applications. Earth Syst Sci Data (2021) 13(9):4349–83. doi: 10.5194/essd-13-4349-2021
39. Quast R, Wagner W, Bauer-Marschallinger B, Vreugdenhil M. Soil moisture retrieval from Sentinel-1 using a first-order radiative transfer model—A case-study over the Po-Valley. Remote Sens Environ (2023) 295:113651. doi: 10.1016/j.rse.2023.113651
40. Brocca L, Filippucci P, Hahn S, Ciabatta L, Massari C, Camici S, et al. SM2RAIN-ASCAT (2007-2018): global daily satellite rainfall data from ASCAT soil moisture observations. Earth Syst Sci Data (2019) 11(4):1583–601. doi: 10.5194/essd-11-1583-2019
41. Brocca L, Moramarco T, Melone F, Wagner W. A new method for rainfall estimation through soil moisture observations. Geophys Res Lett (2013) 40(5):853–8. doi: 10.1002/grl.50173
42. Brocca L, Ciabatta L, Massari C, Moramarco T, Hahn S, Hasenauer S, et al. Soil as a natural rain gauge: estimating global rainfall from satellite soil moisture data. JGR Atmospheres (2014) 119(9):5128–41. doi: 10.1002/2014JD021489
43. Miralles DG, Holmes TRH, De Jeu RAM, Gash JH, Meesters AGCA, Dolman AJ. Global land-surface evaporation estimated from satellite-based observations. Hydrol Earth Syst Sci (2011) 15(2):453–69. doi: 10.5194/hess-15-453-2011
44. Di Miceli C, Carroll M, Sohlberg R, Kim D, Kelly M, Townshend J. MOD44B MODIS/Terra Vegetation Continuous Fields Yearly L3 Global 250m SIN Grid V006., distributed by NASA EOSDIS Land Processes DAAC. (2015). doi: 10.5067/MODIS/MOD44B.006
45. Tóth B, Weynants M, Pásztor L, Hengl T. 3D soil hydraulic database of Europe at 250 m resolution. Hydrol Processes (2017) 31(14):2662–6. doi: 10.1002/hyp.11203
46. Rains D, Trigo I, Dutra E, Ermida S, Ghent D, Hulsman P, et al. High-resolution all-sky land surface temperature and net radiation over Europe. Earth System Science Data (ESSD (2022).
47. Rains D, Lievens H, De Lannoy GJM, McCabe MF, de Jeu RAM, Miralles DG. Sentinel-1 backscatter assimilation using support vector regression or the water cloud model at European soil moisture sites. IEEE Geosci Remote Sens Lett (2021) 19:1–5. doi: 10.1109/LGRS.2021.3073484
48. Lievens H, Brangers I, Marshall H-P, Jonas T, Olefs M, De Lannoy G. Sentinel-1 snow depth retrieval at sub-kilometer resolution over the European Alps. Cryosphere (2022) 16(1):159–77. doi: 10.5194/tc-16-159-2022
49. Avanzi F, Gabellani S, Delogu F, Silvestro F, Cremonese E, Morra di Cella U, et al. Snow Multidata Mapping and Modeling (S3M) 5.1: a distributed cryospheric model with dry and wet snow, data assimilation, glacier mass balance, and debris-driven melt. Geosci Model Dev (2022) 15(12):4853–79. doi: 10.5194/gmd-15-4853-2022
50. Silvestro F, Gabellani S, Delogu F, Rudari R, Boni G. Exploiting remote sensing land surface temperature in distributed hydrological modelling: the example of the Continuum model. Hydrol Earth Syst Sci (2013) 17(1):39–62. doi: 10.5194/hess-17-39-2013
51. Brocca L, Melone F, Moramarco T. Distributed rainfall-runoff modelling for flood frequency estimation and flood forecasting. Hydrol Processes (2011) 25(18):2801–13. doi: 10.1002/hyp.8042
52. Camici S, Massari C, Ciabatta L, Marchesini I, Brocca L. Which rainfall score is more informative about the flood simulation performance? A comprehensive assessment on 1318 basins over Europe. Hydrol Earth Syst Sci (2020) 24(10):4869–85. doi: 10.5194/hess-24-4869-2020
53. De Lavenne A, Andréassian V, Thirel G, Ramos M-H, Perrin C. A regularization approach to improve the sequential calibration of a semidistributed hydrological model. Water Resour Res (2019) 55(11):8821–39. doi: 10.1029/2018WR024266
54. Brocca L, Tarpanelli A, Filippucci P, Dorigo W, Zaussinger F, Gruber A, et al. How much water is used for irrigation? A new approach exploiting coarse resolution satellite soil moisture products. Int J Earth Obs Geoinf (2018) 73:752–66. doi: 10.1016/j.jag.2018.08.023
55. Dari J, Brocca L, Quintana-Seguí P, Escorihuela MJ, Stefan V, Morbidelli R. Exploiting high-resolution remote sensing soil moisture to estimate irrigation water amounts over a Mediterranean region. Remote Sens (2020) 12(16):2593. doi: 10.3390/rs12162593
56. Dari J, Quintana-Seguí P, Morbidelli R, Saltalippi C, Flammini A, Giugliarelli E, et al. Irrigation estimates from space: implementation of different approaches to model the evapotranspiration contribution within a soil-moisture-based inversion algorithm. Agric Water Manag (2022) 265:107537. doi: 10.1016/j.agwat.2022.107537
57. Dari J, Brocca L, Modanesi S, Massari C, Tarpanelli A, Barbetta S, et al. Regional data sets of high-resolution (1 and 6 km) irrigation estimates from space. Earth Syst Sci Data (2023) 15(4):1555–75. doi: 10.5194/essd-15-1555-2023
58. Brocca L, Camici S, Massari M, Tarpanelli A, Ciabatta L, Dari J, et al. (EURAC). Digital Twin Earth Hydrology Evolution [final report]. Zenodo (2023). doi: 10.5281/zenodo.8089045
59. Bazzi H, Baghdadi N, El Hajj M, Zribi M, Belhouchette H. A comparison of two soil moisture products S 2 MP and copernicus-SSM over Southern France. IEEE J Sel Top. Appl Earth Obs Remote Sens (2019) 12(9):3366–75. doi: 10.1109/JSTARS.2019.2927430
60. Fisher JB, Lee B, Purdy AJ, Halverson GH, Dohlen MB, Cawse-Nicholson K, et al. ECOSTRESS: NASA’s next generation mission to measure evapotranspiration from the international space station. Water Resour Res (2020) 56(4). doi: 10.1029/2019WR026058
61. Crow WT, Chen F, Colliander A. Benchmarking downscaled satellite-based soil moisture products using sparse, point-scale ground observations. Remote Sens Environ (2022) 283:113300. doi: 10.1016/j.rse.2022.113300
62. Massari C, Modanesi S, Dari J, Gruber A, De Lannoy GJM, Girotto M, et al. A review of irrigation information retrievals from space and their utility for users. Remote Sens (2021) 13(20):4112. doi: 10.3390/rs13204112
63. Dari J, Brocca L, Quintana-Seguí P, Casadei S, Escorihuela MJ, Stefan V, et al. Double-scale analysis on the detectability of irrigation signals from remote sensing soil moisture over an area with complex topography in central Italy. Adv Water Resour (2022) 161:104130. doi: 10.1016/j.advwatRes2022.104130
64. Zappa L, Schlaffer S, Brocca L, Vreugdenhil M, Nendel C, Dorigo W. How accurately can we retrieve irrigation timing and water amounts from (satellite) soil moisture? Int J Appl Earth Obs Geoinf (2022) 113:102979. doi: 10.1016/j.jag.2022.102979
65. Robichaud PR. Fire effects on infiltration rates after prescribed fire in Northern Rocky Mountain forests, USA. J Hydrol (2000) 231–232:220–9. doi: 10.1016/S0022-1694(00)00196-7
66. Gruber A, De Lannoy G, Albergel C, Al-Yaari A, Brocca L, Calvet J-C, et al. Validation practices for satellite soil moisture retrievals: what are (the) errors? Remote Sens Environ (2020) 244:111806. doi: 10.1016/j.rse.2020.111806
67. Modanesi S, Massari C, Gruber A, Lievens H, Tarpanelli A, Morbidelli R, et al. Optimizing a backscatter forward operator using Sentinel-1 data over irrigated land. Hydrol Earth Syst Sci (2021) 25(12):6283–307. doi: 10.5194/hess-25-6283-2021
68. Modanesi S, Massari C, Bechtold M, Lievens H, Tarpanelli A, Brocca L, et al. Challenges and benefits of quantifying irrigation through the assimilation of Sentinel-1 backscatter observations into Noah-MP. Hydrol Earth Syst Sci (2022) 26(18):4685–706. doi: 10.5194/hess-26-4685-2022
69. Wagner W, Bauer-Marschallinger B, Navacchi C, Reuß F, Cao S, Reimer C, et al. A Sentinel-1 backscatter datacube for global land monitoring applications. Remote Sens (2021) 13(22):4622. doi: 10.3390/rs13224622
70. Frantz D. FORCE. landsat+ Sentinel-2 analysis ready data and beyond. Remote Sens (2019) 11(9):1124. doi: 10.3390/rs11091124
71. Kumar SV, Dong J, Peters-Lidard CD, Mocko D, Gómez B. Role of forcing uncertainty and background model error characterization in snow data assimilation. Hydrol Earth Syst Sci (2017) 21(6):2637–47. doi: 10.5194/hess-21-2637-2017
72. De Lannoy GJM, Bechtold M, Albergel C, Brocca L, Calvet J, Carrassi A, et al. Perspective on satellite-based land data assimilation to estimate water cycle components in an era of advanced data availability and model sophistication. Front Water (2022) 4:981745. doi: 10.3389/frwa.2022.981745
73. Kumar SV, Reichle RH, Harrison KW, Peters-Lidard CD, Yatheendradas S, Santanello JA. A comparison of methods for a priori bias correction in soil moisture data assimilation. Water Resour Res (2012) 48(3):W03515. doi: 10.1029/2010WR010261
74. De Lannoy GJM, de Rosnay P, Reichle RH. Soil moisture data assimilation. In: Duan Q, Pappenberger F, Wood A, Cloke H, Schaake J, editors. Handbook of hydrometeorological ensemble forecasting. Berlin, Heidelberg: Springer (2019). doi: 10.1007/978-3-642-39925-1_32
75. Camporese M, Girotto M. Recent advances and opportunities in data assimilation for physics-based hydrological modeling. Front Water (2022) 4:948832. doi: 10.3389/frwa.2022.948832
76. Reichle RH. Data assimilation methods in the earth sciences. Adv Water Resour (2008) 31(11):1411–8. doi: 10.1016/j.advwatRes2008.01.001
77. Stoffelen A. Toward the true near-surface wind speed: error modeling and calibration using triple collocation. J Geophys Res (1998) 103(C4):7755–66. doi: 10.1029/97JC03180
78. Scipal K, Holmes T, de Jeu R, Naeimi V, Wagner W. A possible solution for the problem of estimating the error structure of global soil moisture data sets. Geophys Res Lett (2008) 35(24):L24403. doi: 10.1029/2008GL035599
79. McColl KA, Vogelzang J, Konings AG, Entekhabi D, Piles M, Stoffelen A. Extended triple collocation: estimating errors and correlation coefficients with respect to an unknown target. Geophys Res Lett (2014) 41(17):6229–36. doi: 10.1002/2014GL061322
80. Massari C, Crow W, Brocca L. An assessment of the performance of global rainfall estimates without ground-based observations. Hydrol Earth Syst Sci (2017) 21(9):4347–61. doi: 10.5194/hess-21-4347-2017
81. Alvarez-Garreton C, Ryu D, Western AW, Crow WT, Su C-H, Robertson DR. Dual assimilation of satellite soil moisture to improve streamflow prediction in data-scarce catchments. Water Resour Res (2016) 52(7):5357–75. doi: 10.1002/2015WR018429
82. Massari C, Camici S, Ciabatta L, Brocca L. Exploiting satellite-based surface soil moisture for flood forecasting in the Mediterranean area: state update versus rainfall correction. Remote Sens (2018) 10(2):292. doi: 10.3390/rs10020292
83. Reichle RH, Koster RD. Bias reduction in short records of satellite soil moisture. Geophys Res Lett (2004) 31(19):L19501. doi: 10.1029/2004GL020938
84. De Lannoy GJM, Reichle RH. Assimilation of SMOS brightness temperatures or soil moisture retrievals into a land surface model. Hydrol Earth Syst Sci (2016) 20(12):4895–911. doi: 10.5194/hess-20-4895-2016
85. Lievens H, Reichle RH, Liu Q, De Lannoy GJM, Dunbar RS, Kim SB, et al. Joint Sentinel-1 and SMAP data assimilation to improve soil moisture estimates. Geophys Res Lett (2017) 44(12):6145–53. doi: 10.1002/2017GL073904
86. Camps-Valls G, Svendsen DH, Cortés-Andrés J, Mareno-Martínez A, Pérez-Suay A, Adsuara J, et al. Physics-aware machine learning for geosciences and remote sensing. IEEE Int Geosci Remote Sens Symp IGARSS (2021) 2021:2086–9. doi: 10.1109/IGARSS47720.2021.9554521
87. Tuia D, Roscher R, Wegner JD, Jacobs N, Zhu X, Camps-Valls G. Toward a collective agenda on ai for earth science data analysis. IEEE Geosci Remote Sens Mag (2021) 9(2):88–104. doi: 10.1109/MGRS.2020.3043504
88. Bauer P, Stevens B, Hazeleger W. A Digital Twin of Earth for the green transition. Nat. Clim Change (2021) 11(2):80–3. doi: 10.1038/s41558-021-00986-y
89. Svendsen DH, Martino L, Camps-Valls G. Active emulation of computer codes with Gaussian processes–Application to remote sensing. Pattern Recognit (2020) 100:107103. doi: 10.1016/j.patcog.2019.107103
90. Runge J, Bathiany S, Bollt E, Camps-Valls G, Coumou D, Deyle E, et al. Inferring causation from time series in Earth system sciences. Nat Commun (2019) 10(1):1–13. doi: 10.1038/s41467-019-10105-3
91. Camps-Valls G, Gerhardus A, Ninad U, Varando G, Martius G, Balaguer-Ballester E, et al Discovering causal relations and equations from data. Phys Rep (2023) 1044:1–68. doi: 10.1016/j.physrep.2023.10.005
92. Lagouarde J-P, Bhattacharya BK, Crébassol P, Gamet P, Adlakha D, Murthy CS, et al. Indo-French high-resolution thermal infrared space mission for earth natural resources assessment and monitoring -Concept and definition of TRISHNA. Int Arch. Photogramm Remote Sens Spatial Inf Sci XLII–3 (2019) XLII–3/W6:403–7. doi: 10.5194/isprs-archives-XLII-3-W6-403-2019
93. Unwin MJ, Pierdicca N, Cardellach E, Rautiainen K, Foti G, Blunt P, et al. An introduction to the HydroGNSS GNSS reflectometry remote sensing mission. IEEE J Sel Top Appl Earth Obs Remote Sens (2021) 14:6987–99. doi: 10.1109/JSTARS.2021.3089550
94. Massotti L, Siemes C, March G, Haagmans R, Silvestrin P. Next generation gravity mission elements of the mass change and geoscience international constellation: from orbit selection to instrument and mission design. Remote Sens (2021) 13(19):3935. doi: 10.3390/rs13193935
95. Hu C, Chen Z, Li Y, Dong X, Hobbs S. Research progress on geosynchronous synthetic aperture radar. Fundam Res (2021) 1(3):346–63. doi: 10.1016/j.fmre.2021.04.008
96. McCabe MF, Rodell M, Alsdorf DE, Miralles DG, Uijlenhoet R, Wagner W, et al. The future of earth observation in hydrology. Hydrol Earth Syst Sci (2017) 21(7):3879–914. doi: 10.5194/hess-21-3879-2017
97. Lagouarde J-P, Bhattacharya BK, Crebassol P, Gamet P, Babu SS, Boulet G, et al. (2018). The Indian-French Trishna mission: earth observation in the thermal infrared with high spatio-temporal resolution, in: Proceedings of the IGARSS 2018 2018, Valencia, Spain, 22–27 July 2018. pp. 4078–81. doi: 10.1109/IGARSS.2018.8518720
98. Aragon B, Ziliani MG, Houborg R, Franz TE, McCabe MF. CubeSats deliver new insights into agricultural water use at daily and 3 m resolutions. Sci Rep (2021) 11(1):12131. doi: 10.1038/s41598-021-91646-w
99. McCabe MF, Aragon B, Houborg R, Mascaro J. CubeSats in hydrology: ultrahigh-resolution insights into vegetation dynamics and terrestrial evaporation. Water Resour Res (2017) 53(12):10017–24. doi: 10.1002/2017WR022240
100. Tollefson J. Billion-dollar NASA satellite launches to track Earth’s water. Nature (2022). doi: 10.1038/d41586-022-04455-0
Keywords: hydrology, remote sensing, flood, drought, water resources management, Digital Twin Earth
Citation: Brocca L, Barbetta S, Camici S, Ciabatta L, Dari J, Filippucci P, Massari C, Modanesi S, Tarpanelli A, Bonaccorsi B, Mosaffa H, Wagner W, Vreugdenhil M, Quast R, Alfieri L, Gabellani S, Avanzi F, Rains D, Miralles DG, Mantovani S, Briese C, Domeneghetti A, Jacob A, Castelli M, Camps-Valls G, Volden E and Fernandez D. A Digital Twin of the terrestrial water cycle: a glimpse into the future through high-resolution Earth observations. Front Sci (2024) 1:1190191. doi: 10.3389/fsci.2023.1190191
Received: 20 March 2023; Accepted: 18 December 2023;
Published: 05 March 2024.
Edited by:
Justin Sheffield, University of Southampton, United KingdomCopyright © 2024 Brocca, Barbetta, Camici, Ciabatta, Dari, Filippucci, Massari, Modanesi, Tarpanelli, Bonaccorsi, Mosaffa, Wagner, Vreugdenhil, Quast, Alfieri, Gabellani, Avanzi, Rains, Miralles, Mantovani, Briese, Domeneghetti, Jacob, Castelli, Camps-Valls, Volden and Fernandez. This is an open-access article distributed under the terms of the Creative Commons Attribution License (CC BY). The use, distribution or reproduction in other forums is permitted, provided the original author(s) and the copyright owner(s) are credited and that the original publication in this journal is cited, in accordance with accepted academic practice. No use, distribution or reproduction is permitted which does not comply with these terms.
*Correspondence: Luca Brocca, bHVjYS5icm9jY2FAaXJwaS5jbnIuaXQ=