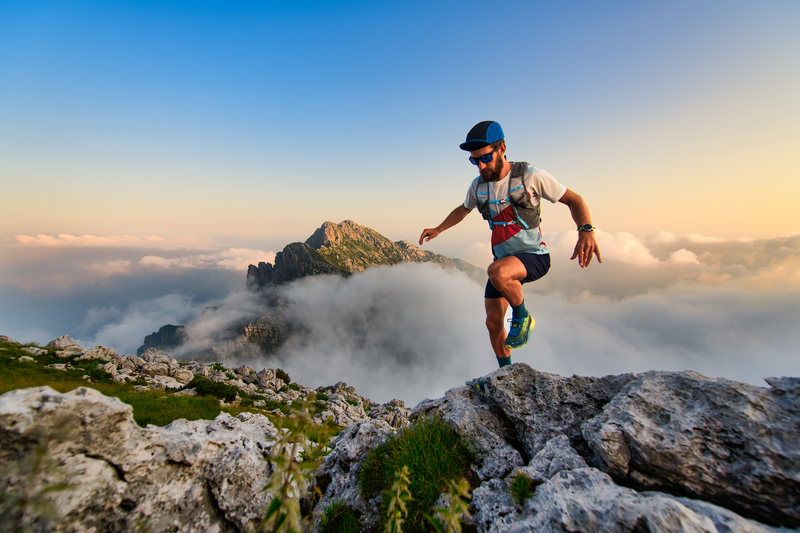
94% of researchers rate our articles as excellent or good
Learn more about the work of our research integrity team to safeguard the quality of each article we publish.
Find out more
EDITORIAL article
Front. Mar. Sci. , 26 January 2023
Sec. Ocean Observation
Volume 10 - 2023 | https://doi.org/10.3389/fmars.2023.1100998
This article is part of the Research Topic Technological Advances for Measuring Planktonic Components of the Pelagic Ecosystem: An Integrated Approach to Data Collection and Analysis View all 14 articles
Editorial on the Research Topic
Technological advances for measuring planktonic components of the pelagic ecosystem: An integrated approach to data collection and analysis
The traditional collection of plankton samples, often using nets followed by visual sorting and taxonomic analysis is a labour intensive, time-consuming, and ultimately expensive process. The increasing demand for pelagic data combined with ever reducing budgets for monitoring and the general problem of the taxonomic impediment have driven the development of new tools and techniques for the sampling and analysis of this key ecosystem component.
Technological advances have allowed monitoring of the pelagic environment to evolve towards a more integrated approach to data collection at a finer scale. Nowadays, there is a plethora of methods including for example molecular, optical, remote sensing, and automated techniques that help further our understanding of biodiversity and species interactions within the pelagic ecosystem. Yet, there is still a need for morphological identification of species to enable the verification of the new sensor modalities.
Ongoing and new challenges need to be addressed, for example those associated with taxonomic resolution from image analysis methods; or where the rate of collecting data exceeds that of processing it, a situation arising from collection of huge amounts of fine-resolution data. Furthermore, there is a large variety of data formats including for example, images, acoustics, taxonomy, remote sensing etc … collected at different spatial and temporal scales. There is therefore a need to: (1) improve on how these emerging technologies, collecting multi-modal data, work together; (2) develop new methods embedding technological advances in data collection and data analytics, for a fully integrated understanding of pelagic processes; and (3) utilise open access databases that can handle diverse data sets.
Articles presented in this topic have a strong focus on:
(1) imaging instruments both for collected samples (e.g., PlanktoScope (Mériguet et al.; Pollina et al.), FlowCam (Mériguet et al.), and in-situ deployment (e.g., Underwater Vision Profiler (Barth and Stone; Drago et al.), Plankton Imager (PI) (Giering et al.), In Situ Ichthyoplankton Imaging System (ISIIS) (Panaïotis et al.), Scripps Plankton Camera (SPC) (Le et al.), Imaging FlowCytobot (Kraft et al.); Video Plankton Recorder (VPR) (Plonus et al.) and the use of machine learning tools to automatically classify the collected images (e.g., Weldrick).
(2) molecular tools (DNA barcoding (de Vargas et al.), COI metabarcoding (Bucklin et al.) to explore the taxonomic and ecological diversity.
These tools have their own characteristics, with molecular based techniques focussing on taxonomic resolution and imaging instruments on quantifying plankton abundance, biomass, and morphology, but at a lower taxonomic resolution. Both have played an increasing role in the collection of plankton information, and the articles in this collection suggest that this will continue to be the case as technologies are refined and become more affordable. As affordability and ease of use in technologies increases, along with the advances and reliability of machine learning algorithms, it will become possible to move towards consistent and long-term measurements of plankton abundance/biomass and diversity.
Globally, plankton time-series have provided essential information about how planktonic assemblages and constituent taxa respond to climate change. However, more data are needed to inform these powerful tools. Ships of opportunity and citizen science have an increasing role to play, by deploying easy to use instruments and offering an opportunity to collect cost-effective plankton data on a global scale (Pollina et al.; de Vargas et al.).
Imaging systems and traditional nets collect data on scales and from within volumes that are very different. Consequently, comparisons of data collected from imaging systems and traditional methods need further attention if we are to inter-calibrate their measurements (Le et al.). Further improvements to the performance of automated image analysis for taxonomic identification are also needed. This will help to reconcile findings with traditional net-based methods (Barth and Stone), their complementary use, and build trust in Artificial Intelligence (AI) based taxonomy (Giering et al.).
All the methods using novel technologies described in this collection of papers allow for plankton information to be collected at a much higher rate than previously possible using traditional deployment of nets followed by microscopic analysis. The increasing use of digital imaging and molecular tools will enable plankton abundances and biomass distributions, as well as community composition and taxonomic diversity to be mapped at global scales in a much shorter time frame (Drago et al.). Used in combination, they offer new opportunities to monitor and study plankton ecosystems at levels of detail never possible before.
A key element of an ever-increasing amount of data is the development of automated and efficient data processing techniques for seamless data flow. Ship time remains an expensive commodity and it is important to be able to capitalise on information collected at sea in real-time. Analysing continuous streams of high-frequency data calls for development and deployment of novel computer vision and machine learning systems (Panaïotis et al.; Le et al.), while cloud platform and high-performance computing are needed for processing the huge datasets collected. Together they can open new horizons for testing core hypotheses on plankton communities in aquatic ecosystems. Seamless data pipeline and emerging data analytics based on AI further offer the opportunity to move towards real time monitoring of plankton ecosystems (Kraft et al.).
Cost-effective global monitoring will enable us to obtain a complete picture of plankton composition, biogeography and biogeochemistry, opening the way to a plankton observatory network on a planetary scale (Mériguet et al.; Pollina et al.; Drago et al.; de Vargas et al.).
All authors listed have made a substantial, direct, and intellectual contribution to the work and approved it for publication.
FL recieved funding from the European Union’s Horizon 2020 research and innovation programme “Atlantic Ecosystems Assessment, Forecasting and Sustainability” (AtlantECO) under grant agreement No 862923 and the JERICO-S3 project under grant agreement No 871153. EF was funded through the UK Natural Environment Research Council's National Capability Long-term Single Centre Science Programme, Climate Linked Atlantic Sector Science, grant number NE/R015953/1, a contribution to Theme 1.3–Biological Dynamics.
The authors declare that the research was conducted in the absence of any commercial or financial relationships that could be construed as a potential conflict of interest.
All claims expressed in this article are solely those of the authors and do not necessarily represent those of their affiliated organizations, or those of the publisher, the editors and the reviewers. Any product that may be evaluated in this article, or claim that may be made by its manufacturer, is not guaranteed or endorsed by the publisher.
Keywords: imaging, molecular tools, global monitoring, AI based taxonomy, computer vision, data pipeline, real-time monitoring, plankton observatory network
Citation: Pitois SG, Fileman ES, Benfield MC, Wiebe PH and Lombard F (2023) Editorial: Technological advances for measuring planktonic components of the pelagic ecosystem: An integrated approach to data collection and analysis. Front. Mar. Sci. 10:1100998. doi: 10.3389/fmars.2023.1100998
Received: 17 November 2022; Accepted: 16 January 2023;
Published: 26 January 2023.
Edited and Reviewed by:
Won Sang Lee, Korea Polar Research Institute, Republic of KoreaCopyright © 2023 Pitois, Fileman, Benfield, Wiebe and Lombard. This is an open-access article distributed under the terms of the Creative Commons Attribution License (CC BY). The use, distribution or reproduction in other forums is permitted, provided the original author(s) and the copyright owner(s) are credited and that the original publication in this journal is cited, in accordance with accepted academic practice. No use, distribution or reproduction is permitted which does not comply with these terms.
*Correspondence: Sophie G. Pitois, c29waGllLnBpdG9pc0BjZWZhcy5nb3YudWs=
Disclaimer: All claims expressed in this article are solely those of the authors and do not necessarily represent those of their affiliated organizations, or those of the publisher, the editors and the reviewers. Any product that may be evaluated in this article or claim that may be made by its manufacturer is not guaranteed or endorsed by the publisher.
Research integrity at Frontiers
Learn more about the work of our research integrity team to safeguard the quality of each article we publish.