- 1Ocean BioGeoscience, National Oceanography Centre, Southampton, United Kingdom
- 2Plankton Analytics Ltd., Plymouth, United Kingdom
- 3Marine Biological Association, Plymouth, United Kingdom
- 4School of Biological and Marine Sciences, University of Plymouth, Plymouth, United Kingdom
- 5Centre for Environment Fisheries and Aquatic Sciences (Cefas), Lowestoft, United Kingdom
Zooplankton are fundamental to aquatic ecosystem services such as carbon and nutrient cycling. Therefore, a robust evidence base of how zooplankton respond to changes in anthropogenic pressures, such as climate change and nutrient loading, is key to implementing effective policy-making and management measures. Currently, the data on which to base this evidence, such as long time-series and large-scale datasets of zooplankton distribution and community composition, are too sparse owing to practical limitations in traditional collection and analysis methods. The advance of in situ imaging technologies that can be deployed at large scales on autonomous platforms, coupled with artificial intelligence and machine learning (AI/ML) for image analysis, promises a solution. However, whether imaging could reasonably replace physical samples, and whether AI/ML can achieve a taxonomic resolution that scientists trust, is currently unclear. We here develop a roadmap for imaging and AI/ML for future zooplankton monitoring and research based on community consensus. To do so, we determined current perceptions of the zooplankton community with a focus on their experience and trust in the new technologies. Our survey revealed a clear consensus that traditional net sampling and taxonomy must be retained, yet imaging will play an important part in the future of zooplankton monitoring and research. A period of overlapping use of imaging and physical sampling systems is needed before imaging can reasonably replace physical sampling for widespread time-series zooplankton monitoring. In addition, comprehensive improvements in AI/ML and close collaboration between zooplankton researchers and AI developers are needed for AI-based taxonomy to be trusted and fully adopted. Encouragingly, the adoption of cutting-edge technologies for zooplankton research may provide a solution to maintaining the critical taxonomic and ecological knowledge needed for future zooplankton monitoring and robust evidence-based policy decision-making.
Introduction
Zooplankton biodiversity contributes to multiple ecosystem services such as carbon and nutrient cycling, as well as the role of plankton in the marine food web. Understanding how plankton communities respond to changes in anthropogenic pressures, such as climate change and nutrient loading, is key to implementing effective management measures. The new generation of policy initiatives explicitly recognises the role that plankton biodiversity plays in delivering a variety of ecosystem services. These legislations, such as the United Nations Sustainable Development Goals (UN General Assembly, 2015), the Convention on Biological Diversity Aichi Targets (Convention on Biological Diversity, 2011), and the upcoming Post-2020 Global Biodiversity Framework (Convention on Biological Diversity, 2021), focus on a holistic view of biodiversity including the value of zooplankton. In Europe, for example, the Marine Strategy Framework Directive (Directive (EC) 2008/56, 2008) aims to achieve Good Environmental Status of marine waters, with plankton representing pelagic habitats in the legislation and implementation (European Commission, 2008; OSPAR, 2017; Bedford et al., 2018; McQuatters-Gollop et al., 2019; McQuatters-Gollop et al., 2022). European Union Member States are therefore required to monitor and assess the state of plankton, and, if needed, to implement management measures to achieve Good Environmental Status for pelagic habitats. Consequently, a comprehensive understanding of plankton communities is critically needed to inform a robust evidence base for supporting decision-making for marine management. Establishing a robust understanding of the relationships between anthropogenic pressures and zooplankton, however, depends on consistent time-series datasets, which are limited in number and spatial scale (McQuatters-Gollop et al., 2015; Zingone et al., 2015; McQuatters-Gollop et al., 2017). These gaps mean that policymakers have limited evidence on which to base decisions about enacting management measures related to plankton and the ecosystem services they provide.
Even though plankton in European waters are better sampled than those in many other parts of the world (O’Brien et al., 2017), gaps in this evidence base exist due to both lack of sampling and lack of knowledge of plankton dynamics and pressure-state relationships (McQuatters-Gollop et al., 2022). Zooplankton sampling is historically more limited than phytoplankton sampling, resulting in more numerous knowledge gaps around changes in zooplankton communities and the consequent effects on the marine food web and ecosystem services (McQuatters-Gollop et al., 2015). The UK’s fixed-point monitoring programme, for example, has 11 phytoplankton sampling stations but only four of these also sample zooplankton; these stations are supplemented by phytoplankton sampling by the Environment Agency, but to date there has been virtually no inshore zooplankton sampling (Bedford et al., 2020). For larger spatial coverage, the Continuous Plankton Recorder [CPR, a towed net system (Batten et al., 2003)] provides a wealth of taxonomic data for both zooplankton and phytoplankton, particularly in UK and northern European waters, as well as parts of the North Atlantic, Pacific basins, Southern Ocean, and Australian waters (Figure 1). Yet, coverage for zooplankton data is still highly inconsistent, and wide expanses of coastlines and oceans are not covered at all (Figure 1).
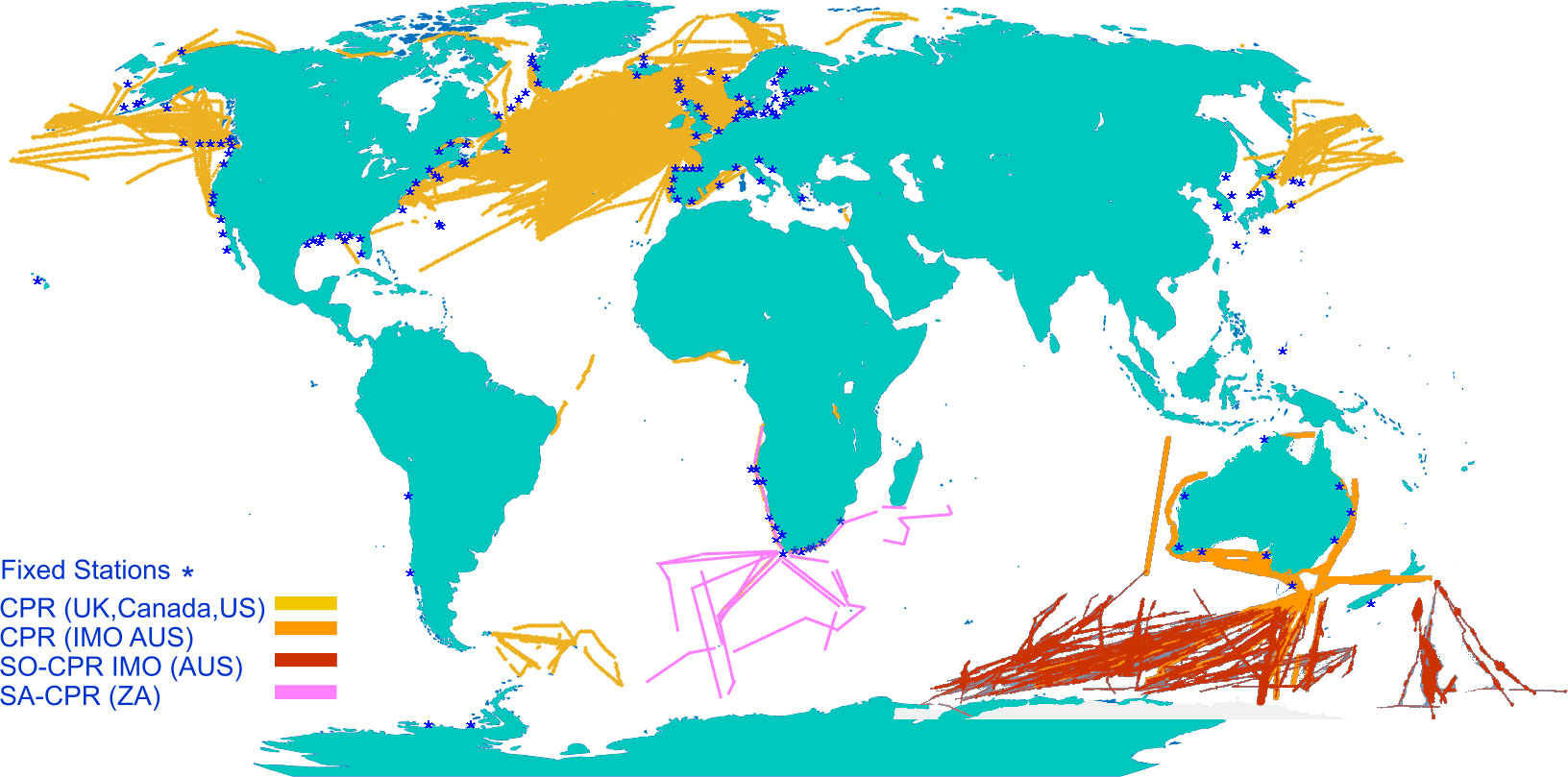
Figure 1 World map overlaid with fixed net sampling stations and Continuous Plankton Recorder tracks (adapted from source: MBA CPR map 1958-2020 (Batten et al., 2019), with data from NOAA Copepod database (O’Brian and Oakes, 2020), Australian IMOS database 1993-2021 (Re3Data.org, 2021) and South African CPR 2005-2021 (Huggett, pers. comm.).
A promising way to fill these gaps in spatial coverage is through the rapid advance of automated sampling systems and plankton imaging capabilities. Numerous commercial and custom-built plankton imaging systems are available (see reviews by Lombard et al., 2019; Giering et al., 2020a), and global roll-outs of zooplankton imaging platforms to match the Argo float global network for physical ocean parameters are starting (Lombard et al., 2019; Picheral et al., 2021).
While the technical abilities now exist to collect data continuously and at fine resolution (Lombard et al., 2019), a major bottleneck is - besides image storage and access - the processing and interpretation, specifically the taxonomic classification of zooplankton images (MacLeod et al., 2010; Orenstein et al., 2022). An obvious avenue to tackle the growing number of plankton images is the use of artificial intelligence (AI) and machine learning tools (ML) for the taxonomic classification of plankton. To date, AI/ML for plankton has been used primarily to aid human-based classification by presorting the images, because their ‘predicted’ taxonomic classifications can be highly variable (Gorsky et al., 2010). Tools available to the community that facilitate such AI/ML-augmented manual classification exist, such as EcoTaxa (Picheral et al., 2017) and MorphoCluster (Schröder et al., 2020). Yet, the reliance on human verification limits the speed with which plankton images can be used for science.
The move to a global plankton-monitoring network hence heavily depends on the automation of taxonomic classification. But challenges with the needed fully-automated taxonomy (because of the large amounts of data) exist, such as questions about whether AI/ML can achieve a taxonomic resolution that scientists trust. We here develop a roadmap for future zooplankton monitoring for policy and management, specifically for the role of imaging and AI/ML, based on community consensus. To do so, we determined current perceptions by the zooplankton research and monitoring community about the use of imaging and AI/ML for zooplankton monitoring, with particular focus on their experience and trust in imaging and AI/ML to produce reliable taxonomic data. Specifically, we assessed the questions:
• Do zooplankton scientists think that images can ever replace physical samples to generate monitoring data?
• Do zooplankton scientists think that an AI can ever replace a human taxonomist in the role of identifying zooplankton?
We recommend the next steps for obtaining robust zooplankton data for large-scale ecosystem assessment and policy decision-making.
We use the term artificial intelligence (AI) to mean the use of computer algorithms to make decisions. In context, AI typically performs data analysis tasks done by humans such as identifying organisms from images. Machine learning (ML) denotes the method of training AI whereby the algorithm improves (‘learns’) based on experience and use of data. In context, ML may be carried out on images already labelled by humans (‘training data’).
Community survey on future of zooplankton monitoring
To obtain a broad sample of responses, we developed a questionnaire in English using JotForm (Supplementary Material). The survey was distributed between November 2021 and January 2022 using social media and through the authors’ professional and personal networks, resulting in 179 complete responses. The final survey used a mixed-methods approach of 34 closed-answer questions. The first part of the survey used classification questions designed to provide an overview of the respondents’ background (age, gender identity, location, education). The remainder of the questionnaire was designed to profile respondents’ experience with zooplankton taxonomy, plankton imaging and AI/ML (qualifications, training, level of expertise, etc.), and their perceptions and trust in plankton imaging and AI/ML for zooplankton taxonomy. The latter was assessed using a series of 5-point Likert scale questions.
All respondents completed the survey themselves and gave their permission to use the results. Individuals were not identifiable from the data provided. All participants were 18 years of age or older. The survey described in this paper was reviewed and approved by the Ethics Committee of the National Oceanography Centre, UK.
Survey analysis
Quantitative data were analysed in R v4.0.2 (R Core Team, 2018). The level of expertise for zooplankton taxonomy, zooplankton imaging, and AI/ML was calculated as the sum of three questions (years of experience, skill level self-assessment, and frequency of training). Likert data were analysed using the ‘Likert’ function from the Likert package in R. Correlations were explored using simple linear regression. The general bias towards or against imaging and AI/ML was calculated as follows. Each question was scored from 1 (‘strongly disagree’) to 5 (‘strongly agree’) on positive questions, and 1 (‘strongly agree’) to 5 (‘strongly disagree’) on reverse questions. If a participant was neutral, they would have scored 18 for imaging or AI/ML (6 questions all answered with neutral = 3). Consequently, a trust score of >18 indicates a favourable disposition towards the technology, while a score of < 18 indicates a negative disposition.
Participant demographic
We collected 179 complete responses. The participant gender distribution showed a near-equal gender balance in the field (55% male and 43% female) with the majority of respondents between 30-39 and 40-49 years old (31 and 26%, respectively) (Figure 2A). Globally, the survey reached participants working in 42 countries. The highest number of participants were from the UK and United States (33 and 25 participants, respectively), followed by Japan (11), Australia (10), Germany (9) and Canada (8) (Figure 2C). This distribution likely reflects funding support and activities in zooplankton monitoring and research as well as network connections both within the community and with the authors, and the use of language (English only).
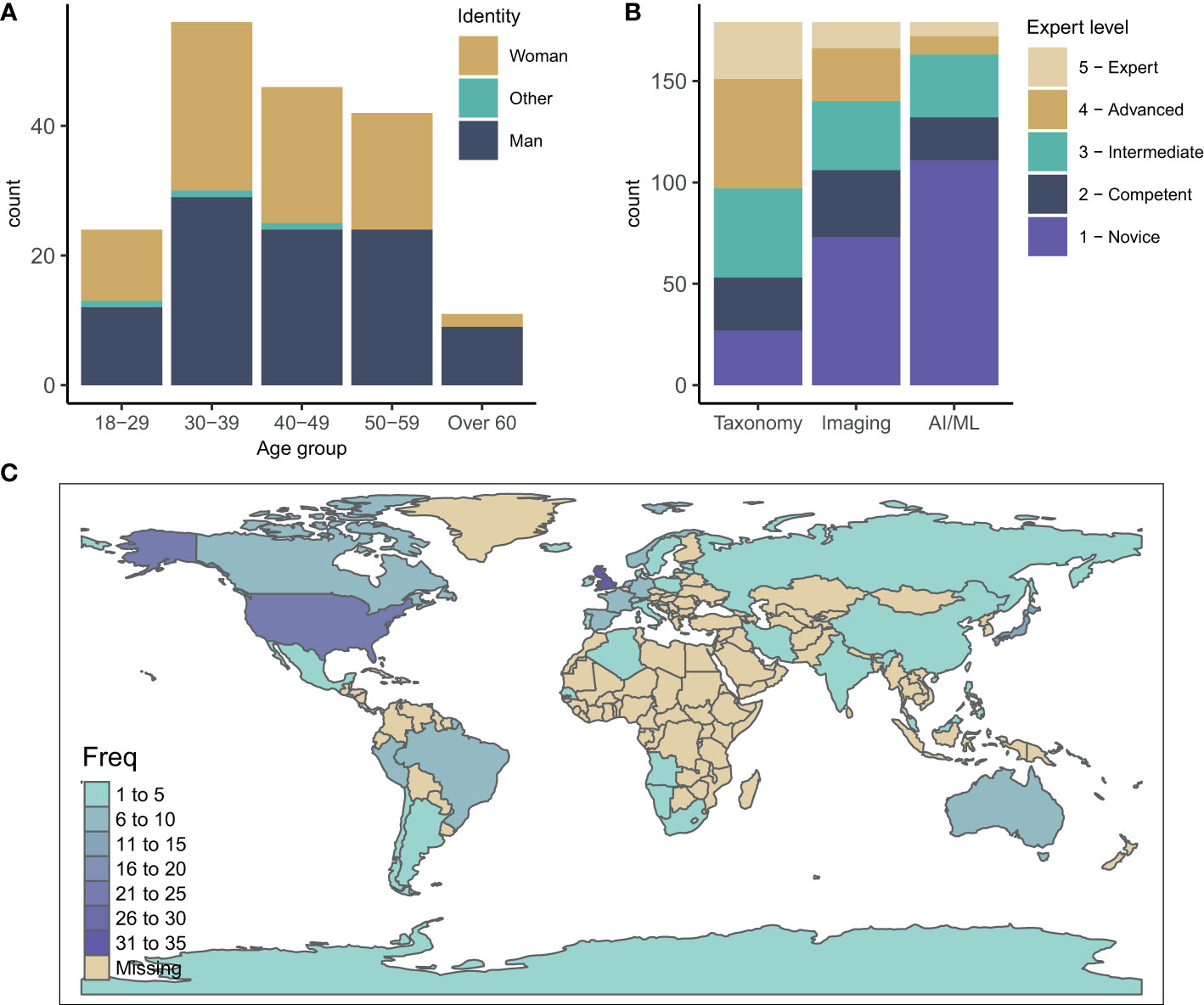
Figure 2 (A) Age and gender distribution of survey participants. (B) Expertise of participants in the three fields of zooplankton taxonomy, zooplankton imaging and AI/ML for zooplankton monitoring and research. (C) Geographic distribution of survey participants.
The participants’ expertise in zooplankton taxonomy was well spread with a slight bias towards intermediate and advanced (median of 3.2 on a scale from 1-Novice to 5-Expert) (Figure 2B). Overall, the participants had less expertise in zooplankton imaging (median 2.3), and least experience in AI/ML (median 1.8) with the majority identifying themselves as novices in this field (Figure 2B). This spread of expertise likely reflects that the field of AI/ML for zooplankton monitoring and research is relatively young and emerging compared to the field of zooplankton taxonomy.
Community consensus
Imaging for zooplankton monitoring and research
When asked about their perceptions on the use of imaging for zooplankton monitoring and research, the participants showed strong consensus that images can provide meaningful information (80%) and have clear advantages over net samples (68%) (Figure 3A). Conversely, participants agreed that images cannot provide the same level of information as physical samples (70%) and physical samples will always be required (72%) (Figure 3). No clear consensus emerged on whether physical samples are preferable (39% neither agreed nor disagreed). Finally, the survey suggested a consensus that time series can be continued with image samples once the technology has evolved sufficiently (62%) (Figure 3A). Overall, the majority of participants was optimistic about the use of images for plankton monitoring: 53% of participants responded positively towards images (with 12% being neutral and 35% having a negative disposition) (Figure 4A).
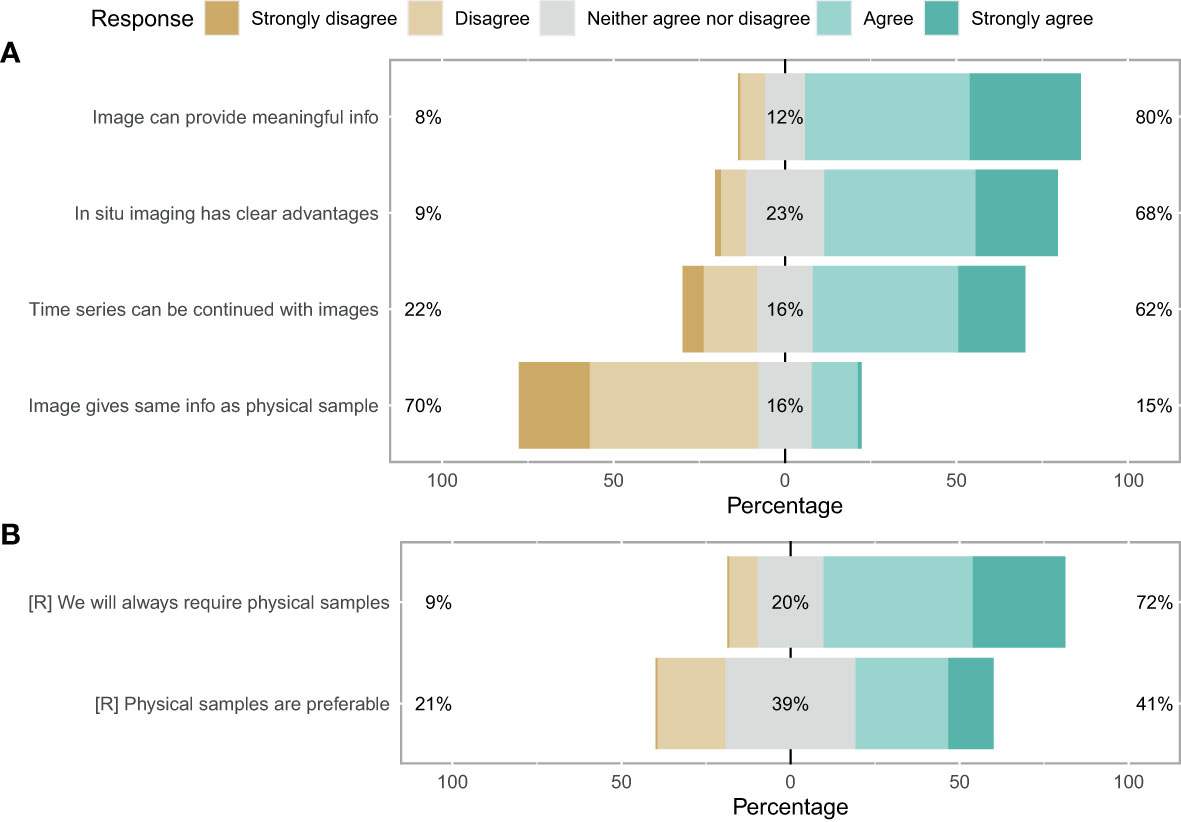
Figure 3 Likert plot for image sufficiency. (A) Darker green shows consensus favours replacement of physical samples with images. Darker brown means a preference for keeping the system as it is. An equal spread likely indicates no clear consensus. (B) Reverse as for (A).
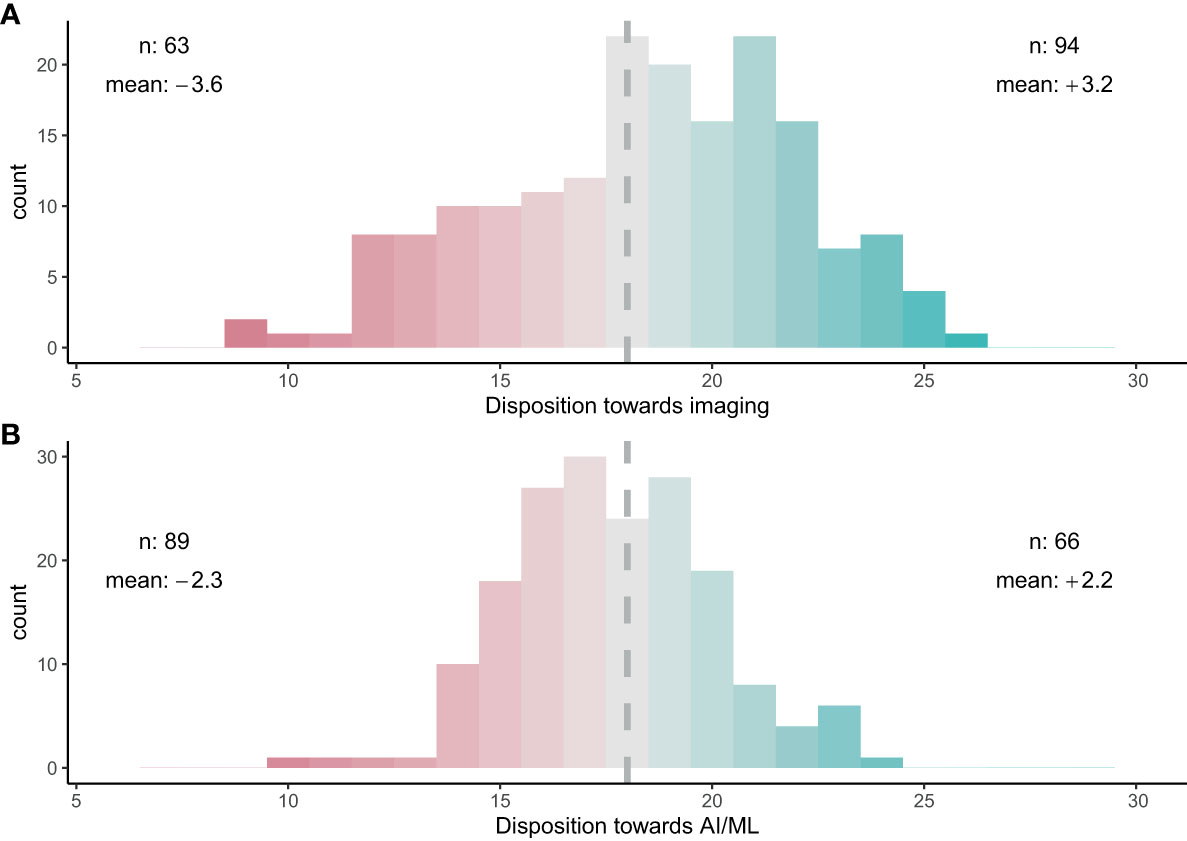
Figure 4 Overall trust in (A) imaging for zooplankton taxonomy and (B) AI/ML for zooplankton taxonomy. A score of 18 indicates a neutral stance towards imaging or AI/ML. A trust score of >18 (green) indicates a favourable disposition towards the technology, while a score of < 18 (red) indicates a negative disposition. ‘n’ shows the number of participants on either side of 18. ‘mean’ shows the average score above or below 18, with a higher absolute number indicating a stronger community bias.
AI/ML for zooplankton taxonomy
When questioned about the potential of AI/ML for zooplankton taxonomy, respondents showed a strong consensus that AI/ML can help to analyse zooplankton data faster than current methods (79%; Figure 5A). However, a strong consensus that AI/ML is limited in its abilities and will always require human guidance and quality control was also evident (83%). When asked whether AI/ML will ever be as good as human taxonomists, which we assessed using both a positive and a reverse statement, no clear consensus was evident. Participants disagreed with the statement that AI/ML would be unbiased and more reliable than humans in identifying images (41%; Figure 5A). Finally, the participants strongly disagreed with the statement that human taxonomists will not be required in future once AI/ML has been trained sufficiently (84%). Indeed, the consensus on this statement was strongest when compared across all 12 questions. Overall, trust in AI/ML for correct taxonomic classification was low: 50% of the participants responded negatively towards AI/ML (with 13% being neutral and 37% having a positive disposition) (Figure 4B).
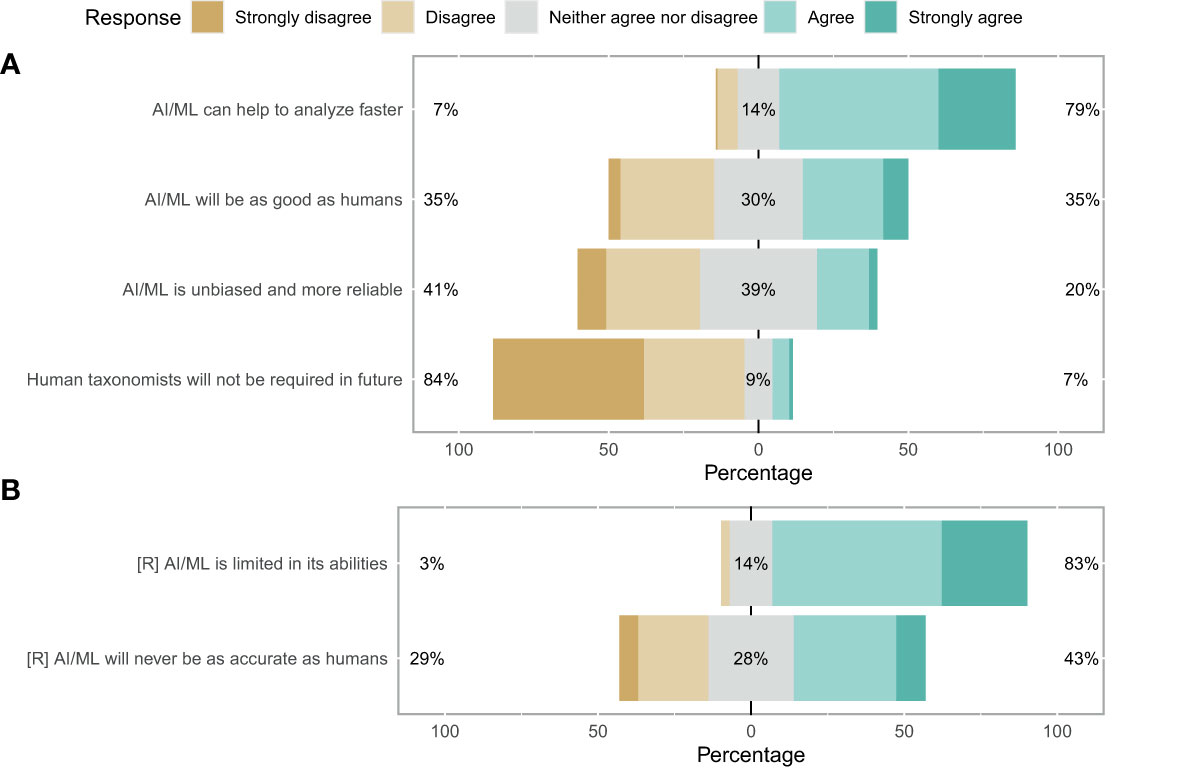
Figure 5 Likert plot for taxonomy sufficiency. (A) More green means consensus favours the replacement of human taxonomists with AI. More brown means a preference for keeping the system as it is. An equal spread likely indicates no clear consensus. (B) Reverse as for a.
Perceived trustworthiness of AI for zooplankton taxonomy
A scientist’s perception is likely influenced by their experience, and we observed clear patterns of this dependency in our survey results: The more respondents were experienced in zooplankton taxonomy, the less they trusted the use of zooplankton images and AI/ML for accurate taxonomy (Figures 6A, D); a significant negative trend was evident between taxonomy expertise and trust in images (p < 0.001, R2 = 0.10, n = 179) and AI/ML (p < 0.001, R2 = 0.10, n = 179). The expertise level in zooplankton imaging had no significant influence on perception of imaging and AI/ML for zooplankton monitoring (for both: p > 0.13, R2 < 0.1, n = 179). Across all imaging expertise levels, respondents were marginally positive towards imaging (median trust scores ≥ 18; Figure 6B). Conversely, the participants were marginally negative towards AI/ML (median trust scores ≤ 18; Figure 6C). Finally, very few of the survey participants were experienced in AI/ML (Figures 6C, D). While novices in this field were undecided on the usefulness of images and AI/ML, the experts tended to be optimistic about the use of zooplankton images (Figure 6C) though less optimistic about the use of AI/ML (Figure 6F). These trends were heavily influenced by single opinions because of the small number of participants who identified as advanced and expert users in the field of AI/ML for zooplankton research.
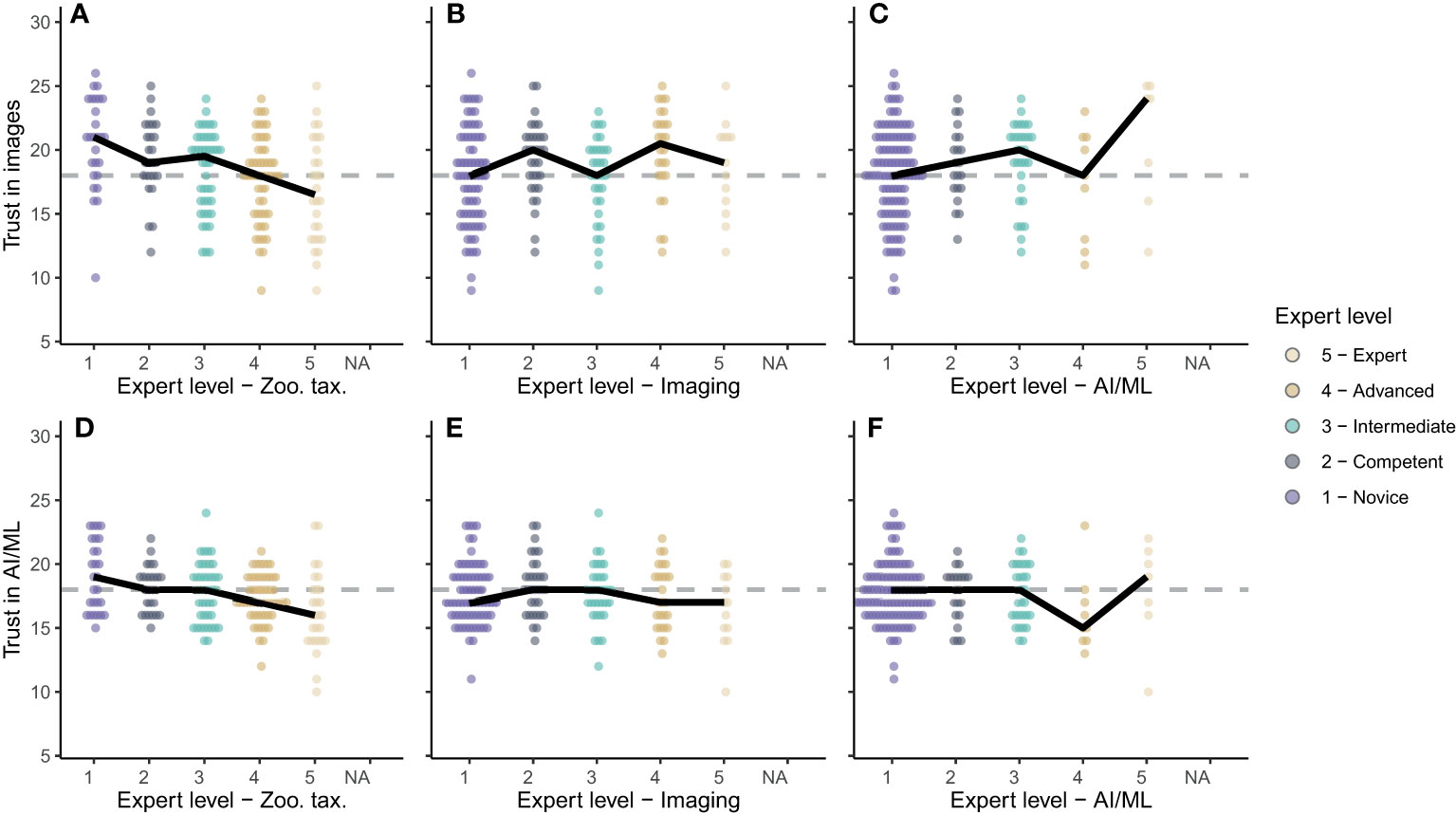
Figure 6 (A–C) Trust in images for zooplankton monitoring and (D–F) trust in AI/ML for zooplankton classification based on expertise in zooplankton taxonomy (A, D), zooplankton imaging (B, E) and AI/ML (C, F). Expert levels range from 1-Novice to 5-Expert as shown in the legend. Dots show the individual scores for each survey participant. Solid black lines connect the medians for each expert level.
The survey participants perceived humans to be good taxonomists with 70% of the participants judging humans to identify >80% of the zooplankton specimens accurately. Sixty-six percent of the participants who rated both AI/ML and human accuracy in identifying zooplankton (103 out of 159) rated AI/ML skill lower than human skill. Only 21% of the participants thought they were similar, and 13% thought that AI/ML was more accurate than humans (Figure 7). Overall, the accuracy of humans was perceived to be significantly better (average rating of 80-90%) than that of AI/ML (average rating 70-80%; paired Wilcoxon test: p < 0.001, n = 156).
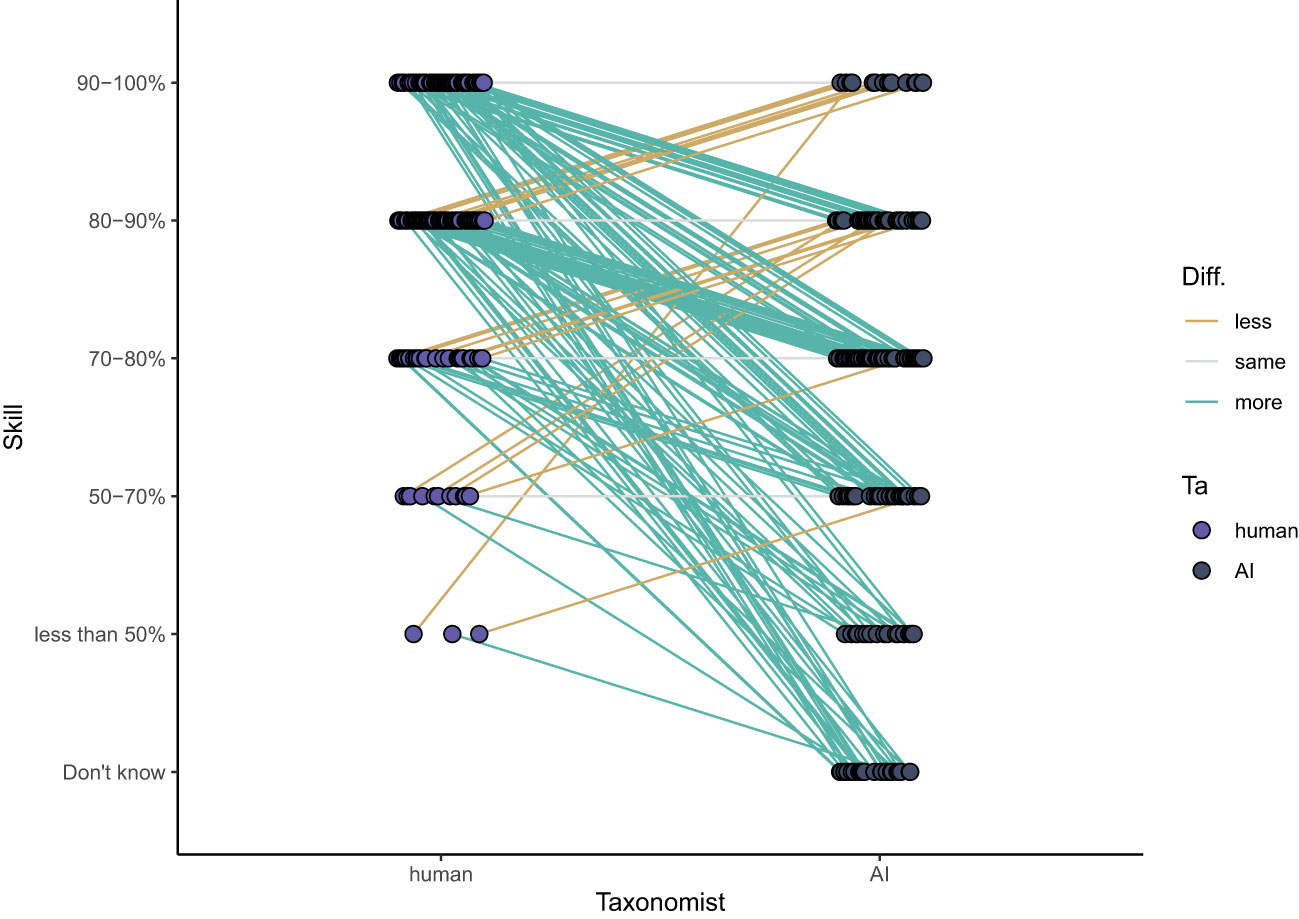
Figure 7 Opinions on the level that humans and AI can correctly identify zooplankton. Lines indicate whether a participant thinks humans are more accurate than AI (green), equally accurate (grey) or less accurate (yellow) than AI.
The participants believed that AI/ML could reasonably identify a copepod to family (28% of participants) or genus level (33% of participants) Figure 8A. For gelatinous zooplankton, the consensus appeared to be that AI/ML could reasonably identify gelatinous zooplankton to family level (33% of participants) (Figure 8B). For both questions (identifying copepods and gelatinous zooplankton), we also asked the participants whether their opinion was mostly influenced by their understanding of the image quality, the capability of AI/ML, or both in equal measures. The participants based their predictions primarily on their understanding of image quality alone or equally both on their understanding of image quality and AI/ML.
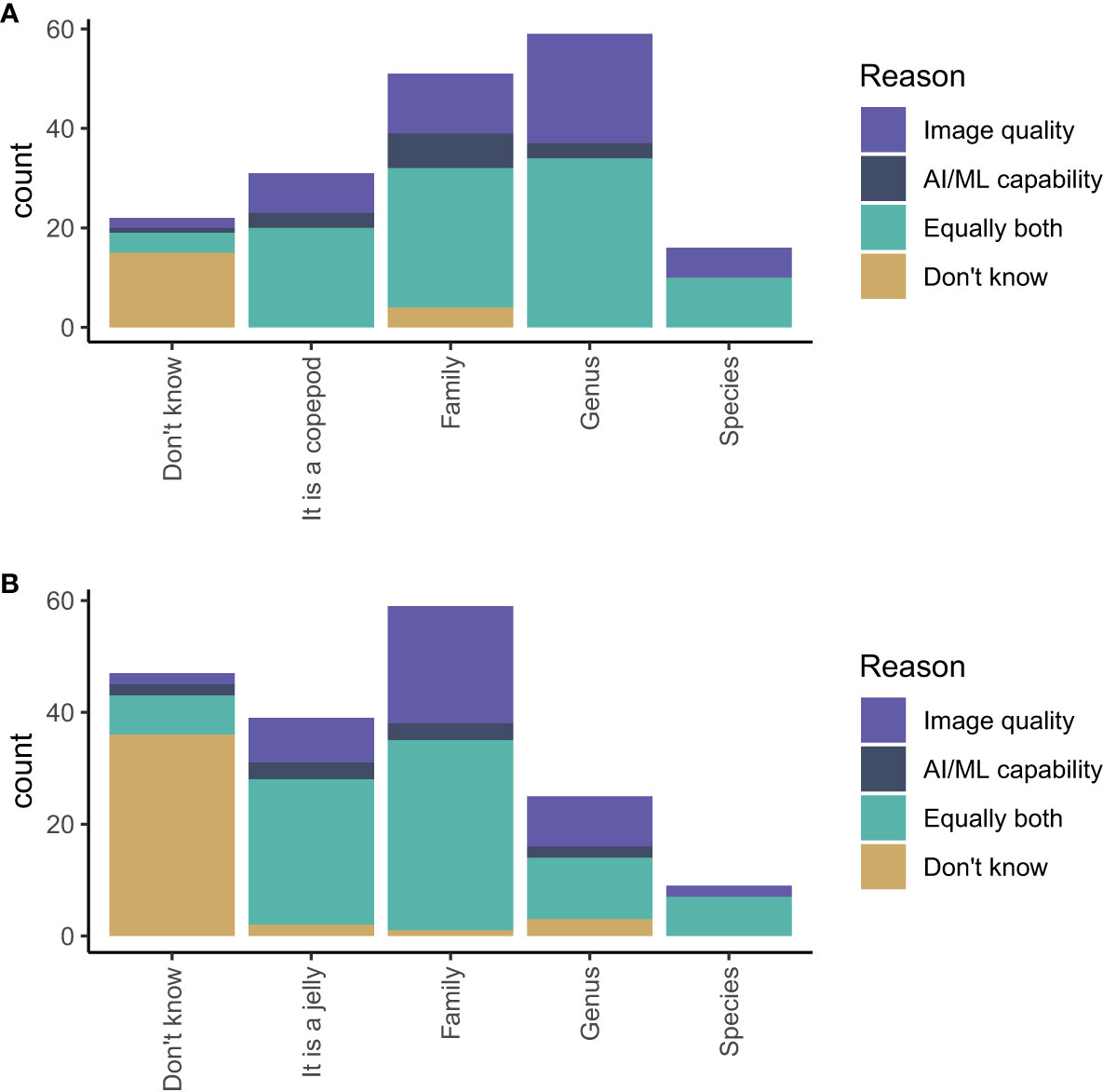
Figure 8 Estimates of the highest taxonomic level an AI/ML algorithm can positively identify (A) a copepod and (B) a gelatinous zooplankton using images generated from an appropriate imaging system. The colours show whether a participant’s answer was most influenced by their understanding of image quality (purple), the capability of AI/ML (dark blue), equally both (turquoise), or unknown (yellow).
Towards a road map
Are images the future?
Our survey results indicated a strong community consensus that images (i.e. digital samples) are a valuable tool for plankton monitoring with clear advantages over physical net samples (Figure 3A), likely reflecting the financial and logistical constraints associated with net sampling. Traditional nets require human-centric, platform-based deployments (usually off a ship) and are hence very limited in their spatiotemporal resolution. The physical samples are stored, often in hazardous chemical preservatives, and shipped to a laboratory for analysis, leading to logistical challenges and considerable delays between sample collection and data availability. Image samples, in contrast, are stored digitally, which offers - amongst other advantages - the ability to share images easily for, e.g., quality control and additional taxonomic classifications by other researchers. Our survey supports the notion that moving towards automated routine image-based sampling combined with image analysis is key to increasing the quantity of zooplankton data to obtain the spatiotemporal coverage required for robust decision-making.
Yet, the survey results also revealed a strong community consensus that, despite the logistical constraints of collecting physical samples, physical samples cannot be replaced by images entirely and will always be required (Figure 3). The reason for the ongoing need for physical samples is likely twofold. First, deeper taxonomic analyses still require physical samples as, at this stage, microscopes offer the often required higher resolution and, importantly, allow the user to investigate each specimen in multiple dimensions and with different exposures. For example, species of the same genus may be morphologically almost indistinguishable bar minute differences in body structures (Fleminger and Hulsemann, 1977; Frost, 1989; Wilson et al., 2015). Considering current technology, such detailed taxonomic information is unlikely from in situ images in the foreseeable future. This notion is also reflected in our survey, where participants revealed low confidence that image quality is sufficient to resolve copepod and gelatinous zooplankton at the species level (Figure 8). Second, physical samples are required for information that cannot be obtained from images, such as biochemical and molecular analyses, which have the potential to greatly advance our understanding of zooplankton biodiversity, ecology and connectivity (Lenz et al., 2021).
While both types of samples (physical and digital) have their advantages, the biggest gain can likely be made when both are used strategically in conjunction (Figure 9). We could leverage the existing monitoring strategies and enhance these through imaging. Ships Of Opportunity have been used by the CPR survey since 1931 to collect physical plankton samples (Batten et al., 2003). Initiatives are now in progress to fit CPRs with holographic camera systems, allowing the simultaneous match-up of in situ imaging data with CPR physical samples (Johns, pers. comm.). In addition, when the physical CPR samples are analysed under the microscope in the laboratory, taxonomists are asked to take an image of each specimen (Johns, pers. comm.). For physical samples, specimens can be imaged before being analysed, e.g., for biochemical composition (Giering et al., 2019). Bench-top instruments for net sample imaging include ZooScan (Grosjean et al., 2004; Picheral et al., 2010) and FlowCam (Detmer et al., 2019). Hence, all physical samples could also be imaged, potentially providing high-quality taxonomic training datasets and additional information on how to translate images into biochemical parameters.
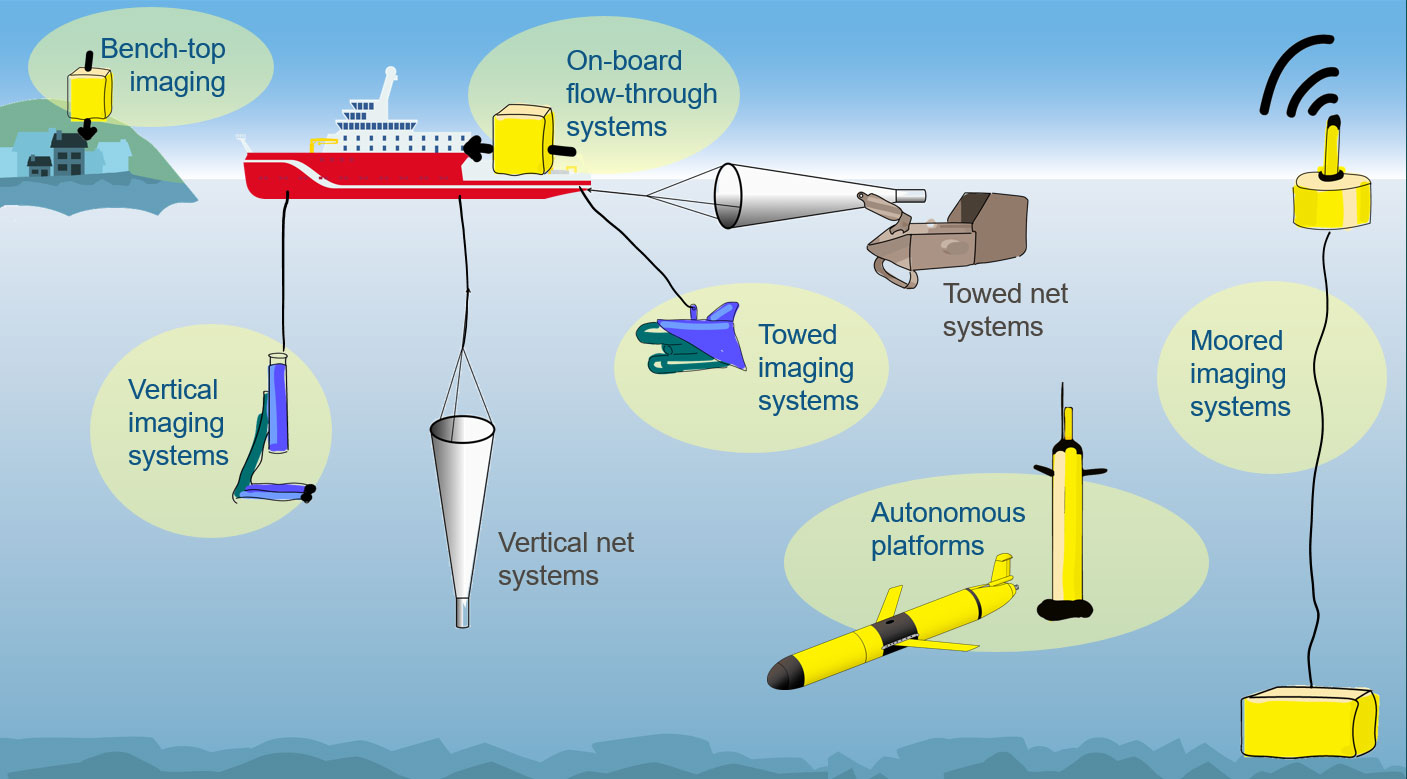
Figure 9 Future zooplankton sampling network. The biggest leverage can be made when both physical sampling, such as vertical and towed net systems, and imaging (highlighted by yellow backgrounds) are used strategically in conjunction. Imaging systems fitted to autonomous and moored platforms allow global coverage with reduced reliance on ships.
On a broader scale, an extension of the current imaging network is the next logical step, and international initiatives to facilitate such networks have commenced (Lombard et al., 2019; de Vargas et al., 2022). Coverage of CPR lines, ideally coupled with imaging, should be expanded to regions with currently poor coverage such as the South Atlantic and Central and South Pacific (Figure 1). As the CPR instrument has to be lowered into the sea and towed behind, it is not suitable for use on all ships. An alternative method is the FerryBox concept, which uses the ship’s pumped water supply (Petersen and Colijn, 2017). While some imaging systems have already been integrated into FerryBoxes (Gannon, 1975), major problems remain with their operation, reliability, size range (too small for large zooplankton), and the development of efficient image processing and classification [https://www.ferrybox.org/]. The Plankton Imager (Pitois et al., 2018; Pitois et al., 2021; Scott et al., 2021) can use the same water source as FerryBox, allowing images of the mesozooplankton to be collected at high speed and moderate volume (34 L min-1) offering similar sampling volume to the CPR (300 L [nautical mile]-1) (John et al., 2002). For research vessels, camera systems such as the Underwater Vision Profiler (Picheral et al., 2010) could be integrated with water sampling rosettes as standard to improve vertically resolved information on zooplankton. Finally, miniaturised camera systems can be fitted on autonomous vehicles, such as floats and gliders (Picheral et al., 2021).
Zooplankton cover a wide range of diversity of organisms in terms of size, shape, and behaviour. As a result, no plankton sampling system - whether collecting digital or physical samples - can estimate the abundance for all components of the plankton at any given time, and any system will likely be biased towards a specific component of the plankton (Owens et al., 2013). Combining datasets from different plankton sampling systems is hence non-trivial. The selection of a sampler and associated sampling design will determine sampling efficiency and selectivity (Pitois et al., 2016; Pitois et al., 2018). Practical issues associated with the collection of physical zooplankton samples (Sameoto et al., 2000) include: active and passive avoidance of the net (Fleminger and Clutter, 1965; Clutter and Anraku, 1968), net clogging, and plankton patchiness (Wiebe and Benfield, 2003; Skjoldal et al., 2013). Imaging devices will not have to cater for all issues associated with nets, but their efficiency will also be dependent on system avoidance, potential damage to fragile organisms particularly when a pumped system is used (albeit typically less problematic compared to net sampling), and camera performance (Pitois et al., 2018). Comparisons between imaging systems and net samples indicated that sampling caveats affect nets and imaging systems in similar proportions (e.g. (Finlay and Roff, 2004; Nogueira et al., 2004; Basedow et al., 2013; Pitois et al., 2018)). In addition, different image processing routines (Giering et al., 2020b) specific to each instrument can result in images that are not directly comparable. A very important step going forward is hence the inter-calibration of all instruments so that all datasets can be combined (Lombard et al., 2019).
Our survey revealed a consensus that time series can be continued with image samples once the technology has evolved sufficiently, indicating a general optimism about the future of imaging for zooplankton monitoring. As we did not investigate what the participants deemed as ‘sufficient’, two aspects need to be considered when evaluating this statement: (1) scientific sufficiency of state-of-the-art technologies, and (2) perceived sufficiency. While recent reviews suggest that further technological and methodological developments are needed to meet the scientific needs (e.g. Lombard et al., 2019; Giering et al., 2020b), this survey suggests that the zooplankton research community is generally willing to adopt these technologies and methodologies. Yet, a period of overlapping use of imaging systems and physical sampling systems, as well as thorough intercalibration between technologies [e.g. (Lombard et al., 2019; Giering et al., 2020a)], will be needed to establish a statistical correlation between the methods before imaging can reasonably replace physical sampling.
Are future taxonomists human?
Our survey results indicated that the community is less favourable towards AI/ML for zooplankton research than towards imaging (Figure 4), likely reflecting the challenges that accurate zooplankton taxonomy poses. Taxonomists learn from concepts, examples and experience, and apply context metadata knowledge to each classification task. Zooplankton taxonomy has a well-established framework with an extensive base of taxonomic literature, most of which is text-based with hand drawings of the organisms’ key features. Reference sheets (for example, the ICES leaflets for marine zooplankton) hold expert keys and drawings that are both distillations and translations of the physical properties of organisms as seen under visual examination (Figure 10). The expert will perform the visual mapping from the hand-drawn “type specimen” to interpret the taxonomic features of the collected specimen, and supplement these using the textual notes on taxonomic descriptions and context metadata (such as size, species distribution, and life history). Together, these information constrain the identification of the collected specimen. The desired confidence in classification often requires a serial search through such taxonomic guides and the call for a second expert opinion. If a taxonomist is not confident in their classification, the specimen is assigned the highest taxonomic level the expert is confident in, or the most probable identification (Choquet et al., 2018). As such, accurate classification of zooplankton is a complex task. This complexity likely explains why researchers with more expertise in zooplankton taxonomy mistrust the use of AI/ML for zooplankton research (Figures 6A, D).
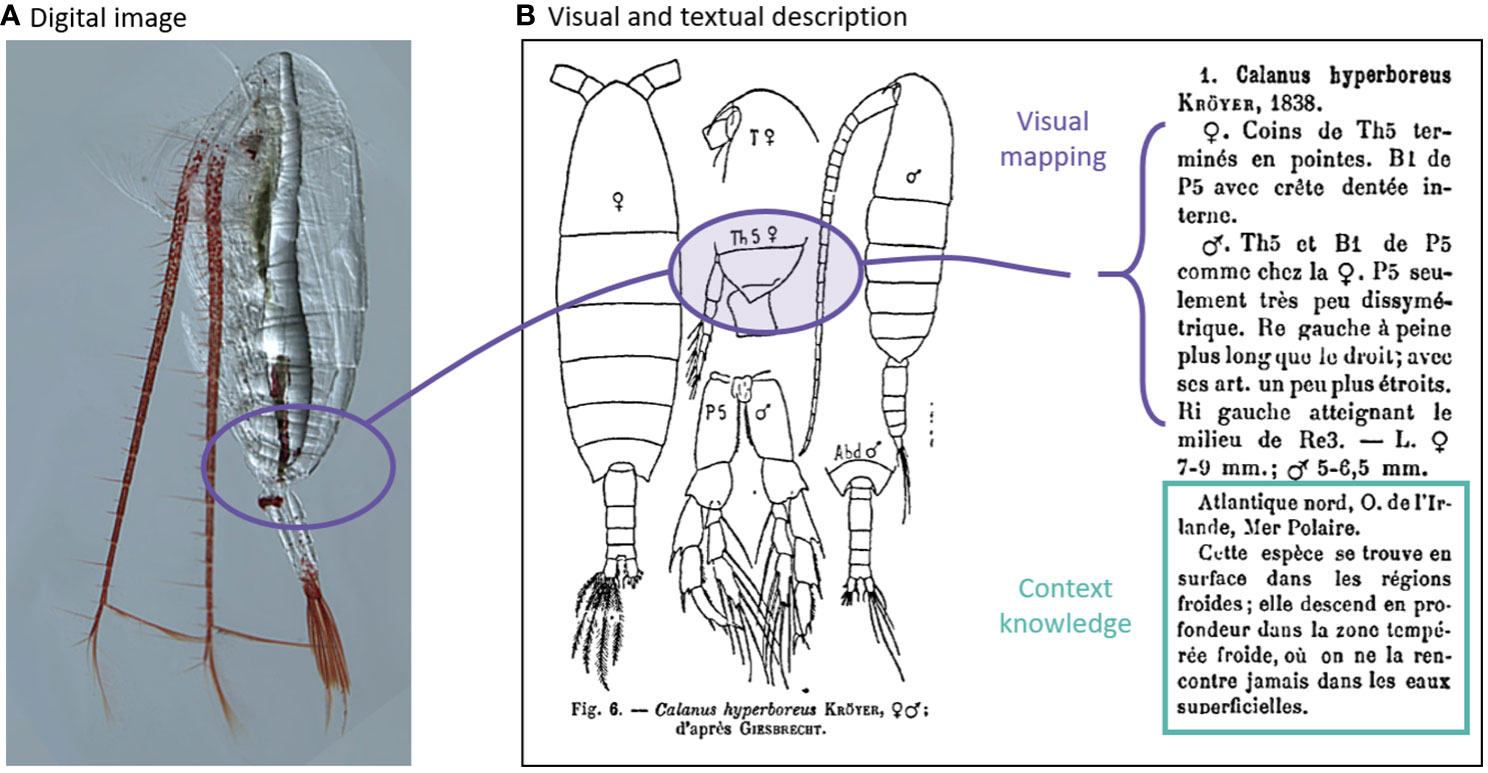
Figure 10 Multimodal learning uses both image and text data. (A) Image of Calanus hyperboreus (Source: Hopcroft at arcodiv.org). (B) Taxonomic description of Calanus hyperboreus (Rose, 1933).
Currently, AI is typically trained on image training datasets produced specifically for the target study, including region and instrument, annotated by the study’s primary researchers. With image quality sometimes low (Lombard et al., 2019; Giering et al., 2020a) and identification frequently carried out by non-specialists (Irisson et al., 2022), confidence in human-led annotation can be low. Sixty percent of the survey participants (that answered the question with a rating) have only a moderate level of trust in current zooplankton training datasets. As ML relies on training data quality (‘garbage in, garbage out’), low-confidence training datasets pose a problem. Hence, we propose that a sufficiently rigorous process, including consensus of training data classification by multiple experts (akin to ‘quality in, quality out’), is needed to facilitate reliable automated classifications that are trusted by the scientific community.
One option is to programme AI to use the same cross-referencing and matching through both visual and textual descriptions as the taxonomy texts (Figure 10). Multimodal approaches, which use both text and image, are now widely applied across a variety of tasks [see reviews by (Baltrušaitis et al., 2019; Chen et al., 2021; Uppal et al., 2022)]. In addition, AI could apply context metadata knowledge to each classification task in a similar way as humans do. For seafloor mapping, for example, the assumption that images captured close to each other are more similar than those taken further apart improves image classification by a factor of two (Yamada et al., 2021). For zooplankton images, the inclusion of context metadata (geometric, hydrographic and geo-temporal information) significantly improves classification accuracy (Ellen et al., 2019).
The survey participants did not agree with the statement that AI/ML is unbiased and more reliable than humans in identifying images (41%), suggesting that human taxonomists are considered reasonably reliable. Yet, expert cognitive biases can contribute to inconsistent performance when manually labelling physical specimens, with inconsistencies in both counting and classification (Culverhouse et al., 2014). For example, repeat analyses of physical net samples, by the same analyst, using microscopy revealed that human-generated repeat counts differed on average by 8%, and taxonomic classification consistency (into 10 broad categories) was on average 75% (Culverhouse et al., 2014). Moreover, differences in counts varied as much as an order of magnitude for the same sample when analysed by different taxonomists, likely owing to psychological factors such as boredom, fatigue and prior expectations (Culverhouse et al., 2014). As such, human-led taxonomy results in non-repeatable outputs, where the same taxonomist at the same location using the same methodology is unlikely to arrive at the same result when repeating sample analysis. Machine learning, in contrast, allows repeatability of analysis results as long as the pre-trained model and weights are used [though the implementation of repeatability needs to be checked prior to model deployment, particularly for deep learning models (Alahmari et al., 2020)]. Yet, self-consistency (i.e. the same person coming to the same conclusion every time) and peer-consistency (i.e. several experts arrive at the same conclusion) (Culverhouse et al., 2003) have received relatively little attention in AI/ML for zooplankton research (Culverhouse et al., 2014).
As current AI-based classifications may be too inaccurate to be used directly for many zooplankton research questions (Irisson et al., 2022), a common practice is to use AI/ML to presort images into classes and then manually verify each AI-based classification; and several commercial and open-source platforms have been designed specifically for this purpose, such as EcoTaxa (Picheral et al., 2017). Alternative strategies are being developed, where unsupervised and supervised classifications alternate to reduce the number of images that a human has to manually verify (e.g. Schröder et al., 2020). The benefit of such workflows is widely accepted by the community, as indicated by our survey results (79% of survey participants agreed that AI/ML can help to analyse images faster; Figure 5A).
AI/ML is still in its infancy and formalised assessment of bias is largely unexplored, which partly explains our survey results that the community is currently undecided whether AI/ML can be as or more accurate as humans for the classification of plankton images (Figures 5, 6D–F). Even if AI will someday be as accurate as human taxonomists, our survey shows a strong community consensus that taxonomists will still be needed in future (84% of participants; Figure 5). While we did not ask specifically why this is the case, several reasons for this judgement are possible. First, the purpose of AI-led classification is to help researchers address scientific questions. Thus, an aspect of scientific quality control will always be required, where a taxonomically literate researcher may perform spot checks and affirm the overall classification as appropriate for the scientific endeavour on hand. Taxonomic experts may further oversee the expansion of current classification algorithms to include newly discovered species or similar amendments to reflect the current scientific knowledge accurately. Second, zooplankton research extends far beyond simply identifying images. Hence, physical samples will continue to play a major role in environmental research (e.g. for biogeochemical analysis or experimental work) and their handling will require expert human taxonomists.
Building human trust in AI/ML for zooplankton research
The survey showed an overall mistrust in the use of AI/ML for zooplankton research, which agrees with reported general attitudes towards AI (Schepman and Rodway, 2020). In their study, Schepman and Rodway (2020) found that participants were positive towards AI and felt comfortable with its use when the application helped humans carry out tasks but did not replace humans or gain autonomy. Conversely, negative feelings were associated with AI applications that involved aspects of human judgement, skill, social understanding or empathy (Schepman and Rodway, 2020). These conclusions can explain some of the trends we observed in our survey, suggesting that researchers consider accurate taxonomy as a difficult skill often relying on judgement based on abstraction and context understanding. While the survey participants felt comfortable with using AI/ML to aid taxonomy (e.g. by presorting images), the replacement of humans with AI was met with scepticism even though research has documented the inaccuracies in human-based taxonomy (Culverhouse et al., 2014).
The reason for the apparent negative perception of AI/ML for zooplankton research is likely founded on a combination of aspects. For those who have not had successful experiences with AI-based classifications and required further taxonomic verification by human taxonomists, trust in automated classification may be weak. Such experiences could explain why 66% of the survey participants that answered the question thought that humans can achieve higher levels of taxonomic accuracy than AI/ML (Figure 7). Yet, ring trials using microscopy on physical samples (community-driven comparison of taxonomic classification across different zooplankton laboratories) show that even highly trained professional zooplankton taxonomists often achieve an identification accuracy of only ~80%, with the identification of copepods to species level posing the biggest challenge (Wootton and Johns, 2019). In contrast, plankton classifiers with an accuracy of >90% have already been developed (Dai et al., 2016; Wang et al., 2018; Ellen et al., 2019; Kerr et al., 2020); though it is seldomly reported whether these classifiers successfully identify key and indicator species, which may be rare (Xue et al., 2018). Another aspect that will influence the trust in AI/ML is previous experience with this technology. Even though we tried to distribute our survey widely, 62% of the participants rated themselves as ‘novice’ in AI/ML for zooplankton image identification and only 9% rated themselves as ‘advanced’ or ‘expert’, reflecting that AI/ML is young in this field and has had limited uptake by the community.
Trust, experience and expertise in AI within the zooplankton research community need to increase for AI-based taxonomy to become fully adopted. Trust is influenced by both the perception of the technology’s competence and emotional factors, and actions to facilitate the adoption of new complex technology, such as AI, need to address both (Hoff and Bashir, 2015; Glikson and Woolley, 2020). Experiments suggested that interaction with AI can significantly increase trust in the observed AI and in future uses (Ullman and Malle, 2017). A visual presence of the AI (rather than an embedded, ‘black box’ feature) also builds trust (Glikson and Woolley, 2020). Visual presence could include visual interfaces as well as visual representations of the results, such as group collages that can be explored by the researcher. In addition, generating the perception of a ‘persona’, the use of human-like behaviour, and personalization to the user’s needs and preferences can help to build emotional trust (Glikson and Woolley, 2020).
In addition, investing in a good reputation and transparency of how the algorithm works also increases trust in the AI’s competence (Glikson and Woolley, 2020). A key step in this process is an increased effort in the development of explainable AI (often referred to as XAI), which provides explanations for the algorithm’s decisions and outputs that are understandable for non-AI experts (in this case, a zooplankton researcher). Keystones for explainability include (1) transparency of how the algorithm works, (2) explanation of the underlying rules for the decision (‘causality’), (3) quantification of bias that could have originated from shortcomings of the training data or choice in algorithm, and (4) confidence in the reliability of the predictions (Hagras, 2018). XAI has gained attention only in the past decade (Carvalho et al., 2019) but is now considered critical for the widespread adoption of AI (e.g. UK Parliament, 2017). However, how exactly XAI for plankton classification could be implemented to maximize trust and confidence by zooplankton researchers is yet unclear, and an appropriate framework needs to be developed through close collaboration between zooplankton taxonomists and researchers (‘users’) and AI developers. Finally, matching users’ expectations and AI performance by providing clear explanations about the AI’s functionality both in terms of how the algorithms work and why they should be used (compared to alternatives) is important. Possible avenues to build cognitive trust thus include demonstration and quantification of reliability of the AI, development of XAI, and close collaboration and dedicated workshops for zooplankton researchers and AI developers.
A new face for zooplankton taxonomy
The exciting developments in cutting-edge information technology for zooplankton research further offer the opportunity of a ‘face-lift’ for the field of taxonomy. The number of taxonomists has declined worldwide (MacLeod et al., 2010; Culverhouse, 2015; McQuatters-Gollop et al., 2017), and fewer trained taxonomists and plankton analysts are recruited each year to replace the previous generation as it retires (McQuatters-Gollop et al., 2017). One solution to this ‘brain drain’ in plankton taxonomy could be to engage traditional taxonomists in training in AL/ML. Such engagement would build trust in the new techniques and also enhance the field of taxonomy with innovative, cutting-edge engineering and informatics technologies. The added interdisciplinary flavour could increase interest in the field of plankton taxonomy because the skills used to collect and analyse in situ imaging data are globally in demand and widely transferable across many non-scientific sectors such as business, economics, and computing. A starting point for merging taxonomy with engineering and computer sciences could be the development of courses that teach the combined skills of AI/imaging/plankton taxonomy at universities. By teaching these skills together, students may start to recognise the links between taxonomy and technology, helping to rebrand zooplankton taxonomy as ‘exciting and relevant’ rather than a career ‘dead end’. This ‘new face’ for zooplankton taxonomy and research may provide a solution to securing, into the future, critical taxonomic and ecological knowledge needed for future zooplankton monitoring and robust evidence-based decision-making and policy.
Robust evidence base for decision-making
To enable policymakers to best make informed decisions about enacting management measures, we require a robust evidence base founded on consistent time-series datasets and broad global coverage. Currently, understanding of plankton dynamics, particularly in response to climate change and direct anthropogenic pressures, is limited due to gaps in data coverage or taxonomic mismatches between time-series with different methodologies. The result is a lack of confidence in the evidence base underpinning decision making (McQuatters-Gollop et al., 2015; McQuatters-Gollop et al., 2017). A big challenge here is how to combine different datasets of varying taxonomic levels. An example of how merged datasets can work is the UK’s and OSPAR’s approach to assessing pelagic habitats in the Northeast Atlantic and the North Sea, which uses flexible indicators that work with a variety of plankton datasets, regardless of differences in sampling method or taxonomic resolution (McQuatters-Gollop et al., 2017; Rombouts et al., 2019; Bedford et al., 2020; McQuatters-Gollop et al., 2022). For example, the “Change in Plankton Communities” indicator applies a plankton lifeform indicator approach that uses functional traits to group plankton taxa into ecologically-relevant lifeform pairs where changes in relative abundance indicate an alteration in ecosystem functioning (McQuatters-Gollop et al., 2019; McQuatters-Gollop et al., 2022). This approach uses taxonomic phytoplankton and zooplankton data that do not need to be refined to the species level. Rather, because of the aggregative nature of lifeforms, data at the order, family, and genus levels can still inform the indicator. Similarly, the “Change in Plankton Biomass and Abundance” indicator is partially informed by data on copepod abundance (OSPAR, 2017). For these indicators, sufficient information is hence broad zooplankton lifeforms identification (e.g. large and small copepods, meroplankton) and abundance. Zooplankton image data therefore has great potential to contribute to these indicators as our survey results indicated a community consensus that images can reasonably inform on the family and genus level (Figure 8).
To retrieve such information from the growing amount of image data swiftly, however, we will have to come up with a strategy to extract relevant taxonomic and abundance information from the images in an automated way. In the foreseeable future, AI will likely be able to classify and count from image datasets with limited input from human taxonomists. Image data could hence be used at a coarse taxonomic level to provide information on lifeforms, or other easily identifiable zooplankton groups, over large spatial scales, akin to Argo data (Roemmich et al., 2019). Thus, the combined use of imaging and automated classification will likely be appropriate to answer questions that require taxonomic resolution that is consistent with the accuracy of the available AI/ML. With AI automation, data can be analysed on-board, for example on the ship during a survey or on a platform, and sent via satellite to provide near-real-time information.
Clear guidelines on quality assurance are required for such a workflow. Algorithms have to follow the FAIR principles (Findability, Accessibility, Interoperability, Reusability) (Hartley and Olsson, 2020). Taxonomic classifications need to link image labels to machine-readable taxonomic trees, such as WoRMS. Data should contain all sources and contributors, including information on both the human and AI who carried out the classifications (i.e. a ‘taxonomist ID’). Finally, the accuracy and certainty of all classifications should be clearly documented (e.g. how sure is the algorithm/human about the identification).
Conclusion and roadmap
The ultimate goal is a cost-effective global zooplankton monitoring programme with comprehensive spatiotemporal coverage that can answer scientific questions and contribute to the robust evidence base required to inform decision making for environmental management. Our survey revealed a clear community consensus that net sampling and traditional taxonomy must be retained in future, yet imaging will play an increasingly important part in the future of zooplankton monitoring and research. For imaging, challenges to address will include, besides technical hurdles such as the transfer of large data and image processing speed, the integration of the outputs from both physical and digital sampling methods. A period of overlapping use of imaging systems and physical sampling systems will be needed before imaging can reasonably replace physical sampling for widespread time-series zooplankton monitoring. In addition, improvements in AI/ML are needed for these to be trusted and fully adopted by zooplankton researchers, particularly taxonomists. The key step forward is parallel programmes that complement each other, while efforts are focussed on bringing imaging technologies on par with traditional taxonomy. This long-term goal will no doubt mean overcoming several challenges, and only then can nets for routine monitoring become a thing of the past.
Based on our discussion above, we recommend the following roadmap:
1. Evidence-based science for decision-making: Use all available plankton datasets to form a robust evidence base for decision-making. Collated and curated datasets will offer unprecedented opportunities to explore differences between collecting instruments. Moreover, large-scale intercomparable datasets can already be used to explore important ecological questions.
2. Technical validation: Enable long-term overlap of imaging and traditional techniques to secure continuity and quality control for high-quality continuous zooplankton monitoring and research.
3. Quality assurance: High-quality robust science demands high levels of self-consistency and peer-consistency. Routines to ensure consistency by humans and AI/ML need to be developed, and the adoption of XAI is required.
4. Interdisciplinary expertise: Invest in training in modern techniques for traditional taxonomists. Support workshops and collaboration between AI/ML and human taxonomists to offer (1) a way of exposing taxonomic experts to AI/ML data and (2) feedback from zooplankton researchers to instrument and AI/ML developers.
5. Capacity building: Invest in retaining taxonomists in the scientific community. Teach combined imaging/AI/taxonomy in university (currently taught independently and traditionally).
Data availability statement
The datasets presented in this article are not readily available because of confidentiality agreements. Requests to access the datasets should be directed to Sarah L. C. Giering (s.giering@noc.ac.uk).
Ethics statement
The studies involving human participants were reviewed and approved by the Ethics Committee of the National Oceanography Centre, UK. The patients/participants provided their written informed consent to participate in this study.
Author contributions
SG: survey design, data analysis and visualization, writing. PC: manuscript writing. DJ: manuscript writing. AM-G: survey design, writing. SP: manuscript writing. All authors contributed to the article and approved the submitted version.
Funding
SG was supported through the ANTICS project, receiving funding from the European Research Council (ERC) under the European Union’s Horizon 2020 research and innovation programme (Grant Agreement No 950212), and through the European Union’s Horizon 2020 research and innovation programme under Grant Agreement No 101000858 (TechOceanS). AM-G is supported by the UK Natural Environment Research Council (NE/R002738/1) Knowledge Exchange fellowship scheme.
Acknowledgments
We would like to thank the editor and two reviewers for their valuable and constructive feedback.
Conflict of interest
PC is a director of Plankton Analytics Ltd., which supplied a Pi-10 plankton imager to CEFAS for evaluation.
The remaining authors declare that the research was conducted in the absence of any commercial or financial relationships that could be construed as a potential conflict of interest
Publisher’s note
All claims expressed in this article are solely those of the authors and do not necessarily represent those of their affiliated organizations, or those of the publisher, the editors and the reviewers. Any product that may be evaluated in this article, or claim that may be made by its manufacturer, is not guaranteed or endorsed by the publisher.
Author disclaimer
This output reflects only the author’s view and the Research Executive Agency (REA) cannot be held responsible for any use that may be made of the information contained therein.
Supplementary material
The Supplementary Material for this article can be found online at: https://www.frontiersin.org/articles/10.3389/fmars.2022.986206/full#supplementary-material
SUPPLEMENTARY DATA SHEET 1 | Questionnaire on future of zooplankton monitoring.
References
Alahmari S. S., Goldgof D. B., Mouton P. R., Hall L. O. (2020). Challenges for the repeatability of deep learning models. IEEE Access 8, 211860–211868. doi: 10.1109/ACCESS.2020.3039833
Baltrušaitis T., Ahuja C., Morency L.-P. (2019). Multimodal machine learning: A survey and taxonomy. IEEE Trans. Pattern Anal. Mach. Intell. 41, 423–443. doi: 10.1109/TPAMI.2018.2798607
Basedow S. L., Tande K. S., Norrbin M. F., Kristiansen S. A. (2013). Capturing quantitative zooplankton information in the sea: Performance test of laser optical plankton counter and video plankton recorder in a calanus finmarchicus dominated summer situation. Prog. Oceanography 108, 72–80. doi: 10.1016/j.pocean.2012.10.005
Batten S. D., Clark R., Flinkman J., Hays G., John E., John A. W. G., et al. (2003). CPR Sampling: the technical background, materials and methods, consistency and comparability. Prog. Oceanography 58, 193–215. doi: 10.1016/j.pocean.2003.08.004
Batten S.D., Abu-Alhaija R., Chiba S., Edwards M., Graham G., Jyothibabu R., et al (2019). A Global Plankton Diversity Monitoring Program. Frontiers in Marine Science 6. doi: 10.3389/fmars.2019.00321
Bedford J., Johns D., Greenstreet S., McQuatters-Gollop A. (2018). Plankton as prevailing conditions: A surveillance role for plankton indicators within the marine strategy framework directive. Mar. Policy 89, 109–115. doi: 10.1016/j.marpol.2017.12.021
Bedford J., Johns D. G., McQuatters-Gollop A. (2020). Implications of taxon-level variation in climate change response for interpreting plankton lifeform biodiversity indicators. ICES J. Mar. Sci. 77, 3006–3015. doi: 10.1093/icesjms/fsaa183
Carvalho D. V., Pereira E. M., Cardoso J. S. (2019). Machine learning interpretability: A survey on methods and metrics. Electronics 8, 832. doi: 10.3390/electronics8080832
Chen W., Wang W., Liu L., Lew M. S. (2021). New ideas and trends in deep multimodal content understanding: A review. Neurocomputing 426, 195–215. doi: 10.1016/j.neucom.2020.10.042
Choquet M., Kosobokova K., Kwaśniewski S., Hatlebakk M., Dhanasiri A. K. S., Melle W., et al. (2018). Can morphology reliably distinguish between the copepods calanus finmarchicus and c. glacialis, or is DNA the only way? Limnology Oceanography: Methods 16, 237–252. doi: 10.1002/lom3.10240
Clutter R. I., Anraku M. (1968). “Avoidance of samplers in zooplankton sampling,” in UNESCO Zooplankton sampling, part i. reviews on zooplankton sampling methods monographs on oceanographic methodology 2 (Paris: UNESCO), 57–76.
Convention on Biological Diversity (2011) Strategic plan for biodiversity 2011-2020, including aichi biodiversity targets. secretariat of the convention on biological diversity. Available at: https://www.cbd.int/sp/ (Accessed September 20, 2022).
Convention on Biological Diversity (2021) First draft of the post-2020 global biodiversity. secretariat of the convention on biological diversity. Available at: https://www.cbd.int/doc/c/abb5/591f/2e46096d3f0330b08ce87a45/wg2020-03-03-en.pdf (Accessed September 20, 2022).
Culverhouse P. F. (2015). Biological oceanography needs new tools to automate sample analysis. J. Mar. Biol. Aquaculture 1, 0–0. doi: 10.15436/2381-0750.15.e002
Culverhouse P. F., Macleod N., Williams R., Benfield M. C., Lopes R. M., Picheral M. (2014). An empirical assessment of the consistency of taxonomic identifications. Mar. Biol. Res. 10, 73–84. doi: 10.1080/17451000.2013.810762
Culverhouse P., Williams R., Reguera B., Herry V., González-Gil S. (2003). Do experts make mistakes? a comparison of human and machine identification of dinoflagellates. Mar. Ecol. Prog. Ser. 247, 17–25. doi: 10.3354/meps247017
Dai J., Wang R., Zheng H., Ji G., Qiao X. (2016). ZooplanktoNet: Deep convolutional network for zooplankton classification, in: OCEANS 2016 - Shanghai. Presented at the OCEANS 2016 - Shanghai, 1–6. doi: 10.1109/OCEANSAP.2016.7485680
Detmer T. M., Broadway K. J., Potter C. G., Collins S. F., Parkos J. J., Wahl D. H. (2019). Comparison of microscopy to a semi-automated method (FlowCAM®) for characterization of individual-, population-, and community-level measurements of zooplankton. Hydrobiologia 838, 99–110. doi: 10.1007/s10750-019-03980-w
de Vargas C., Le Bescot N., Pollina T., Henry N., Romac S., Colin S., et al. (2022). Plankton planet: A frugal, cooperative measure of aquatic life at the planetary scale. Front. Mar. Sci. 9. doi: 10.3389/fmars.2022.936972
Ellen J. S., Graff C. A., Ohman M. D. (2019). Improving plankton image classification using context metadata. Limnology Oceanography: Methods 17, 439–461. doi: 10.1002/lom3.10324
European Commission (2008) Marine strategy framework directive 2008/56/EC — European environment agency. official journal of the European union. Available at: https://www.eea.europa.eu/policy-documents/2008-56-ec (Accessed February 9, 2022).
Finlay K., Roff J. (2004). Radiotracer determination of the diet of calanoid copepod nauplii and copepodites in a temperate estuary. ICES J. Mar. Sci. 61, 552–562. doi: 10.1016/j.icesjms.2004.03.010
Fleminger A., Clutter R. I. (1965). Avoidance of towed nets by zooplankton. Limnology Oceanography 10, 96–104. doi: 10.4319/lo.1965.10.1.0096
Fleminger A., Hulsemann K. (1977). Geographical range and taxonomic divergence in north Atlantic calanus (C. helgolandicus, c. finmarchicus and c. glacialis). Mar. Biol. 40, 233–248. doi: 10.1007/BF00390879
Frost B. W. (1989). A taxonomy of the marine calanoid copepod genus pseudocalanus. Can. J. Zool. 67, 525–551. doi: 10.1139/z89-077
Gannon J. E. (1975). Horizontal distribution of crustacean zooplankton along a cross-lake transect in lake Michigan. J. Great Lakes Res. 1, 79–91. doi: 10.1016/S0380-1330(75)72336-5
Giering S. L. C., Cavan E. L., Basedow S. L., Briggs N., Burd A. B., Darroch L. J., et al. (2020a). Sinking organic particles in the ocean–flux estimates from in situ optical devices. Front. Mar. Sci. 6. doi: 10.3389/fmars.2019.00834
Giering S. L. C., Hosking B., Briggs N., Iversen M. H. (2020b). The interpretation of particle size, shape, and carbon flux of marine particle images is strongly affected by the choice of particle detection algorithm. Front. Mar. Sci. 7. doi: 10.3389/fmars.2020.00564
Giering S. L. C., Wells S. R., Mayers K. M. J., Schuster H., Cornwell L., Fileman E. S., et al. (2019). Seasonal variation of zooplankton community structure and trophic position in the celtic Sea: A stable isotope and biovolume spectrum approach. Prog. Oceanography 177, 101943–101943. doi: 10.1016/j.pocean.2018.03.012
Glikson E., Woolley A. W. (2020). Human trust in artificial intelligence: Review of empirical research. Acad. Manage. Annals. 14 (2): 627–660. doi: 10.5465/annals.2018.0057
Gorsky G., Ohman M. D., Picheral M., Gasparini S., Stemmann L., Romagnan J.-B., et al. (2010). Digital zooplankton image analysis using the ZooScan integrated system. J. Plankton Res. 32, 285–303. doi: 10.1093/plankt/fbp124
Grosjean P., Picheral M., Warembourg C., Gorsky G. (2004). Enumeration, measurement, and identification of net zooplankton samples using the ZOOSCAN digital imaging system. ICES J. Mar. Sci. 61, 518–525. doi: 10.1016/j.icesjms.2004.03.012
Hagras H. (2018). Toward human-understandable, explainable AI. Computer 51, 28–36. doi: 10.1109/MC.2018.3620965
Hartley M., Olsson T. S. G. (2020). dtoolAI: Reproducibility for deep learning. Patterns 1, 100073. doi: 10.1016/j.patter.2020.100073
Hoff K. A., Bashir M. (2015). Trust in automation: Integrating empirical evidence on factors that influence trust. Human Factors 57, 407–434. doi: 10.1177/0018720814547570
Irisson J.-O., Ayata S.-D., Lindsay D. J., Karp-Boss L., Stemmann L. (2022). Machine learning for the study of plankton and marine snow from images. Annu. Rev. Mar. Sci. 14, 277–301. doi: 10.1146/annurev-marine-041921-013023
John E. H., Batten S. D., Stevens D., Walne A. W., Jonas T. J., Hays G. C. (2002). Continuous plankton records stand the test of time: Evaluation of flow rates, clogging and the continuity of the CPR time-series. J. Plankton Res. 24, 941–946. doi: 10.1093/plankt/24.9.941
Kerr T., Clark J. R., Fileman E. S., Widdicombe C. E., Pugeault N. (2020). Collaborative Deep Learning Models to Handle Class Imbalance in FlowCam Plankton Imagery IEEE, 170013–170032. doi: 10.1109/ACCESS.2020.3022242
Lenz P. H., Lieberman B., Cieslak M. C., Roncalli V., Hartline D. K. (2021). Transcriptomics and metatranscriptomics in zooplankton: Wave of the future? J. Plankton Res. 43, 3–9. doi: 10.1093/plankt/fbaa058
Lombard F., Boss E., Waite A. M., Vogt M., Uitz J., Stemmann L., et al. (2019). Globally consistent quantitative observations of planktonic ecosystems. Front. Mar. Sci. 6. doi: 10.3389/fmars.2019.00196
MacLeod N., Benfield M., Culverhouse P. (2010). Time to automate identification. Nature 467, 154–155. doi: 10.1038/467154a
McQuatters-Gollop A., Atkinson A., Aubert A., Bedford J., Best M., Bresnan E., et al. (2019). Plankton lifeforms as a biodiversity indicator for regional-scale assessment of pelagic habitats for policy. Ecol. Indic. 101, 913–925. doi: 10.1016/j.ecolind.2019.02.010
McQuatters-Gollop A., Edwards M., Helaouët P., Johns D. G., Owens N. J. P., Raitsos D. E., et al. (2015). The continuous plankton recorder survey: How can long-term phytoplankton datasets contribute to the assessment of good environmental status? Estuarine Coast. Shelf Sci. 162, 88–97. doi: 10.1016/j.ecss.2015.05.010
McQuatters-Gollop A., Guérin L., Arroyo N. L., Aubert A., Artigas L. F., Bedford J., et al. (2022). Assessing the state of marine biodiversity in the northeast Atlantic. Ecol. Indic. 141, 109148. doi: 10.1016/j.ecolind.2022.109148
McQuatters-Gollop A., Johns D. G., Bresnan E., Skinner J., Rombouts I., Stern R., et al. (2017). From microscope to management: The critical value of plankton taxonomy to marine policy and biodiversity conservation. Mar. Policy 83, 1–10. doi: 10.1016/j.marpol.2017.05.022
Nogueira E., González-Nuevo G., Bode A., Varela M., Morán X. A. G., Valdés L. (2004). Comparison of biomass and size spectra derived from optical plankton counter data and net samples: application to the assessment of mesoplankton distribution along the Northwest and north Iberian shelf. ICES J. Mar. Sci. 61, 508–517. doi: 10.1016/j.icesjms.2004.03.018
O’Brien T. D., Lorenzoni L., Isensee K., Valdés L. (2017). “What are marine ecological time series telling us about the ocean?,” in A status report (Paris, France: IOC-UNESCO).
O’Brien T.D., Oakes S.A., 2020. Visualizing and Exploring Zooplankton Spatio-Temporal Variability, in: Zooplankton Ecology. (Boca Raton: CRC Press) 292.
Orenstein E. C., Ayata S. D., Maps F., Becker É. C., Benedetti F., Biard T., et al (2022). Machine learning techniques to characterize functional traits of plankton from image data Limnol Oceanogr, 67, 1647–1669. doi: 10.1002/lno.12101
OSPAR (2017) Changes in phytoplankton biomass and zooplankton, intermediate assessment 2017. Available at: https://oap.ospar.org/en/ospar-assessments/intermediate-assessment-2017/biodiversity-status/habitats/plankton-biomass/ (Accessed March 4, 2022).
Owens N. J. P., Hosie G. W., Batten S. D., Edwards M., Johns D. G., Beaugrand G. (2013). All plankton sampling systems underestimate abundance: Response to “Continuous plankton recorder underestimates zooplankton abundance” by J.W. dippner and m. Krause. J. Mar. Syst. 128, 240–242. doi: 10.1016/j.jmarsys.2013.05.003
Picheral M., Catalano C., Brousseau D., Claustre H., Coppola L., Leymarie E., et al. (2021). The underwater vision profiler 6: an imaging sensor of particle size spectra and plankton, for autonomous and cabled platforms. Limnology Oceanography: Methods. 20, 115–129 doi: 10.1002/lom3.10475
Picheral M., Colin S., Irisson J.-O. (2017) EcoTaxa, a tool for the taxonomic classification of images. Available at: http://ecotaxa.obs-vlfr.fr.
Picheral M., Guidi L., Stemmann L., Karl D. M., Iddaoud G., Gorsky G. (2010). The underwater vision profiler 5: An advanced instrument for high spatial resolution studies of particle size spectra and zooplankton. Limnology Oceanography: Methods 8, 462–473. doi: 10.4319/lom.2010.8.462
Pitois S. G., Bouch P., Creach V., van der Kooij J. (2016). Comparison of zooplankton data collected by a continuous semi-automatic sampler (CALPS) and a traditional vertical ring net. J. Plankton Res. 38, 931–943. doi: 10.1093/plankt/fbw044
Pitois S. G., Graves C. A., Close H., Lynam C., Scott J., Tilbury J., et al. (2021). A first approach to build and test the copepod mean size and total abundance (CMSTA) ecological indicator using in-situ size measurements from the plankton imager (PI). Ecol. Indic. 123, 107307. doi: 10.1016/j.ecolind.2020.107307
Pitois S. G., Tilbury J., Bouch P., Close H., Barnett S., Culverhouse P. F. (2018). Comparison of a cost-effective integrated plankton sampling and imaging instrument with traditional systems for mesozooplankton sampling in the celtic Sea. Front. Mar. Sci. 5. doi: 10.3389/fmars.2018.00005
Re3Data.org (2021). re3data.org: Integrated Marine Observing System Ocean Data Portal; editing status 2021-11-10; re3data.org - Registry of Research Data Repositories. doi: 10.17616/R35057
R Core Team (2018). R: A language and environment for statistical computing (R Foundation for Statistical Computing: R Foundation for Statistical Computing). Available at: https://www.r-project.org/.
Roemmich D., Alford M. H., Claustre H., Johnson K., King B., Moum J., et al. (2019). On the future of argo: A global, full-depth, multi-disciplinary array. Front. Mar. Sci. 6. doi: 10.3389/fmars.2019.00439
Rombouts I., Simon N., Aubert A., Cariou T., Feunteun E., Guérin L., et al. (2019). Changes in marine phytoplankton diversity: Assessment under the marine strategy framework directive. Ecol. Indic. 102, 265–277. doi: 10.1016/j.ecolind.2019.02.009
Sameoto D., Wiebe P., Runge J., Postel L., Dunn J., Miller C., et al. (2000). “3 - collecting zooplankton,” in ICES zooplankton methodology manual. Eds. Harris R., Wiebe P., Lenz J., Skjoldal H. R., Huntley M. (London: Academic Press), 55–81. doi: 10.1016/B978-012327645-2/50004-9
Schepman A., Rodway P. (2020). Initial validation of the general attitudes towards artificial intelligence scale. Comput. Hum. Behav. Rep. 1, 100014. doi: 10.1016/j.chbr.2020.100014
Schröder S.-M., Kiko R., Koch R. (2020) MorphoCluster: Efficient annotation of plankton images by clustering. arXiv:2005.01595 [cs]. Available at: http://arxiv.org/abs/2005.01595 (Accessed December 9, 2021).
Scott J., Pitois S., Close H., Almeida N., Culverhouse P., Tilbury J., et al. (2021). In situ automated imaging, using the plankton imager, captures temporal variations in mesozooplankton using the celtic Sea as a case study. J. Plankton Res. 43, 300–313. doi: 10.1093/plankt/fbab018
Skjoldal H. R., Wiebe P. H., Postel L., Knutsen T., Kaartvedt S., Sameoto D. D. (2013). Intercomparison of zooplankton (net) sampling systems: Results from the ICES/GLOBEC sea-going workshop. Prog. Oceanography 108, 1–42. doi: 10.1016/j.pocean.2012.10.006
UK Parliament, (House of Lords) Artificial Intelligence Committee (2017) AI In the UK: Ready, willing and able? Available at: https://publications.parliament.uk/pa/ld201719/ldselect/ldai/100/10002.htm (Accessed June 15, 2022).
Ullman D., Malle B. F. (2017). “Human-robot trust: Just a button press away,” in Proceedings of the Companion of the 2017 ACM/IEEE International Conference on Human-Robot Interaction HRI ‘17. Association for Computing Machinery 309–310 (New York, NY, USA: Association for Computing Machinery). doi: 10.1145/3029798.3038423
UN General Assembly (2015) Transforming our world : the 2030 agenda for sustainable development. Available at: http://bit.ly/TransformAgendaSDG-pdf (Accessed September 20, 2022).
Uppal S., Bhagat S., Hazarika D., Majumder N., Poria S., Zimmermann R., et al. (2022). Multimodal research in vision and language: A review of current and emerging trends. Inf. Fusion 77, 149–171. doi: 10.1016/j.inffus.2021.07.009
Wang C., Zheng X., Guo C., Yu Z., Yu J., Zheng H., et al (2018). Transferred Parallel Convolutional Neural Network for Large Imbalanced Plankton Database Classification, in: 2018 OCEANS - MTS/IEEE Kobe Techno-Oceans (OTO). Presented at the 2018 OCEANS - MTS/IEEE Kobe Techno-Oceans (OTO), 1–5. doi: 10.1109/OCEANSKOBE.2018.8558836
Wiebe P. H., Benfield M. C. (2003). From the hensen net toward four-dimensional biological oceanography. Prog. Oceanography 56, 7–136. doi: 10.1016/S0079-6611(02)00140-4
Wilson R. J., Speirs D. C., Heath M. R. (2015). On the surprising lack of differences between two congeneric calanoid copepod species, calanus finmarchicus and c. helgolandicus. Prog. Oceanography 134, 413–431. doi: 10.1016/j.pocean.2014.12.008
Wootton M., Johns D. (2019) Zooplankton ring test 2018/2019. Plymouth: Marine biological analytical quality control scheme. Available at: http://www.nmbaqcs.org/scheme-components/zooplankton/reports/.
Xue Y., Chen H., Yang J. R., Liu M., Huang B., Yang J. (2018). Distinct patterns and processes of abundant and rare eukaryotic plankton communities following a reservoir cyanobacterial bloom. ISME J. 12, 2263–2277. doi: 10.1038/s41396-018-0159-0
Yamada T., Prügel-Bennett A., Thornton B. (2021). Learning features from georeferenced seafloor imagery with location guided autoencoders. J. Field Robotics 38, 52–67. doi: 10.1002/rob.21961
Keywords: in situ imaging, artificial intelligence/machine learning, taxonomy, digital samples, ecosystem assessment, long-term monitoring, zooplankton
Citation: Giering SLC, Culverhouse PF, Johns DG, McQuatters-Gollop A and Pitois SG (2022) Are plankton nets a thing of the past? An assessment of in situ imaging of zooplankton for large-scale ecosystem assessment and policy decision-making. Front. Mar. Sci. 9:986206. doi: 10.3389/fmars.2022.986206
Received: 04 July 2022; Accepted: 26 September 2022;
Published: 16 November 2022.
Edited by:
Tim Wilhelm Nattkemper, Bielefeld University, GermanyReviewed by:
Bank Beszteri, Universität Duisburg-Essen, GermanyJason Stockwell, University of Vermont, United States
Copyright © 2022 Giering, Culverhouse, Johns, McQuatters-Gollop and Pitois. This is an open-access article distributed under the terms of the Creative Commons Attribution License (CC BY). The use, distribution or reproduction in other forums is permitted, provided the original author(s) and the copyright owner(s) are credited and that the original publication in this journal is cited, in accordance with accepted academic practice. No use, distribution or reproduction is permitted which does not comply with these terms.
*Correspondence: Sarah L. C. Giering, cy5naWVyaW5nQG5vYy5hYy51aw==
†ORCID: Sarah L. C. Giering, orcid.org/0000-0002-3090-1876
Phil F. Culverhouse, orcid.org/0000-0002-7586-6496
Abigail McQuatters-Gollop, orcid.org/0000-0002-6043-9563
Sophie G. Pitois, orcid.org/0000-0003-3955-1451