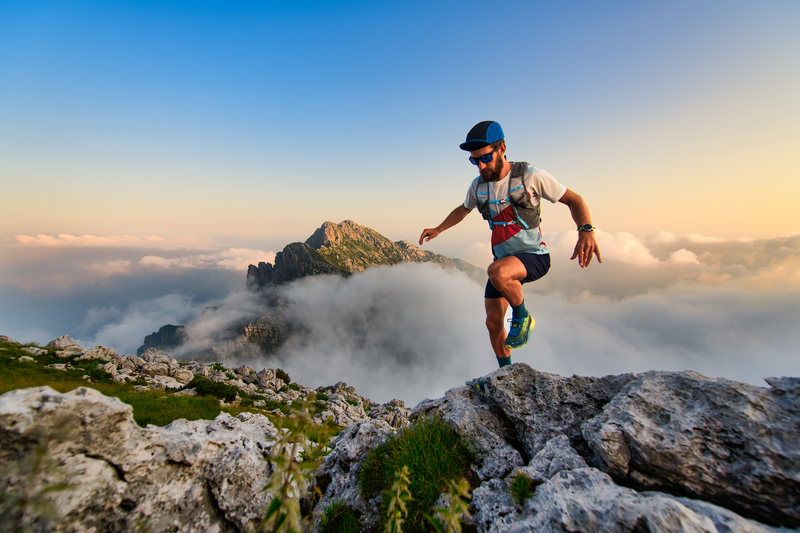
95% of researchers rate our articles as excellent or good
Learn more about the work of our research integrity team to safeguard the quality of each article we publish.
Find out more
PERSPECTIVE article
Front. Educ. , 13 March 2025
Sec. Digital Education
Volume 10 - 2025 | https://doi.org/10.3389/feduc.2025.1548900
The study aims at gaining insights into relationships between perceived institutional support and students’ perceptions of AI-supported learning. It also investigates the mediating role perceived learning outcomes and moderating effect of technology self-efficacy within this context. Research model was developed and validated based on Social Cognitive Theory (SCT) and the learning outcomes of students. Using quantitative research design and convenience sampling technique, 204 students from higher education institutions were included in the analysis. Data were analyzed using structural equation modeling (SEM) to test the hypothesized relationships. The results revealed that perceived institutional support significantly impacts students’ perceptions of AI-supported learning (β = 0.200, C.R. = 2.291, p = 0.022), technology self-efficacy (β = 0.492, C.R. = 9.671, p < 0.001), and learning outcomes. Additionally, technology self-efficacy was found negative moderating effect (β = −0.146, CR = −2.507, p = 0.012) the relationship between perceived institutional support and AI-supported learning perceptions. Perceived learning outcome partial mediated the relationship between perceived institutional support and students’ perceptions of AI-supported learning, with a direct effect of (β = 0.155, p < 0.001) and an indirect effect of (β = 0.539, p < 0.001), as evidenced by the confidence interval [0.235, 0.549]. These findings highlight the significant interplay of perceived institutional support, technology self-efficacy, and perceived learning outcomes in shaping students’ perceptions of AI in higher education, underscoring the importance of fostering supportive academic environments for effective AI integration. The theoretical and practical implications of the study are discussed.
The integration of Artificial Intelligence (AI) in higher education is revolutionizing the learning landscape by offering personalized, adaptive, and efficient learning experiences (Luckin and Holmes, 2016). As AI technologies become increasingly prominent, understanding students’ perceptions of AI-supported learning is critical to ensuring successful adoption and maximizing learning outcomes. Perceived institutional support is critical in encouraging the adoption and effective use of AI tools like ChatGPT in higher education. AI-supported learning refers to the integration of artificial intelligence technologies into educational processes to enhance teaching and learning experiences (Ouyang et al., 2023; Rerhaye et al., 2021; Wang et al., 2023). This approach leverages AI tools to provide personalized learning experiences, automate administrative tasks, and improve the accessibility of educational resources (Alam, 2023; Sajja et al., 2023). Common applications of AI in learning include adaptive learning platforms, virtual tutors, language translation tools, and intelligent feedback systems like ChatGPT, which can provide instant support and interactive content for learners (Alqahtani et al., 2023; Graefen and Fazal, 2024; Zhao et al., 2024).
Institutions that provide robust support in the form of training, resources, and guidance foster an environment where students feel confident and capable of leveraging AI technologies. Such support helps students overcome the initial barriers to adoption, such as lack of knowledge or apprehension about AI tools, and encourages their integration into learning routines. Research suggests that perceived institutional support positively influences technology adoption behaviors by reducing perceived complexity and increasing perceived usefulness (Al-Rahmi et al., 2022; Teo et al., 2019). For instance, students who receive institutional backing, such as workshops or access to online tutorials, are more likely to use AI tools for research, assignment preparation, and skill development, thereby enhancing their overall learning experience. Moreover, institutional support plays a pivotal role in aligning AI technologies with pedagogical objectives. Tools like ChatGPT have the potential to personalize learning experiences, improve critical thinking, and enhance academic performance (Abas et al., 2023; Bettayeb et al., 2024). However, their effective use depends largely on the guidance and support provided by educational institutions. When institutions proactively address ethical concerns, provide guidelines for appropriate use, and encourage feedback, they create an ecosystem that maximizes the potential of AI tools (Abulibdeh et al., 2024; Adel et al., 2024).
Perceived institutional support is a critical factor that directly influences students’ perceptions of AI-supported learning. When students perceive strong institutional backing, such as access to resources, guidance, and a conducive learning environment, their confidence in engaging with technology increases significantly. This perceived institutional support nurtures higher technology self-efficacy, which enables students to interact with AI tools like ChatGPT more effectively. Technology self-efficacy, as a core component of Social Cognitive Theory (SCT), enhances students’ ability to navigate digital platforms, fostering positive perceptions of their utility and relevance in academic contexts (Abubakar, 2024; Wang et al., 2022). These perceptions of AI-supported learning are pivotal in shaping students’ academic outcomes. Research has found that students who perceive AI tools as beneficial are more likely to utilize them for academic purposes, leading to improved critical thinking and enhanced academic performance (Hwang et al., 2020). Thus, the study established these objectives (1) To investigate the influence of perceived institutional support on students’ perceptions of AI-supported learning in higher education. (2) To examine the mediating and moderating roles of technology self-efficacy and perceived learning outcomes in the relationship between institutional support and students’ perceptions of AI-supported learning.
Social Cognitive Theory is a cognitive formulation of social learning theory that explains human behavior as a dynamic interaction between personal factors, environmental influences, and behavior (Glanz, 2001). It integrates concepts from cognitive, behavioristic, and emotional models of behavior change and emphasizes the importance of observational learning, reinforcement, self-control, and self-efficacy in influencing behavior. Social Cognitive Theory (SCT) is among the most commonly utilized frameworks for understanding health behavior (Baranowski et al., 2002). SCT suggests that there is a mutually influential relationship between the individual, their environment, and behavior. These three components interact dynamically with one another, shaping behavior and providing a foundation for potential interventions to alter those behaviors (Bandura, 2001). Bandura’s SCT emphasizes the importance of the environment, personal factors, and behavior in learning and technology adoption. Perceived institutional support acts as an environmental factor that shapes students’ perceptions and behaviors toward AI-supported learning by creating an enabling atmosphere that facilitates the effective use of technology. This support, such as training and access to resources, boosts students’ confidence in AI tools, thus improving their perception of its utility (Bandura, 1986). SCT highlights the interaction between personal, behavioral, and environmental factors, in the context of this study: personal factors (technology self – efficacy), environmental factor (perceived institutional support), Behavioral outcomes (Students’ perceptions of AI-supported learning).
Perceived institutional support plays a crucial role in undergraduate students’ willingness to use AI tools in their academic pursuits. Research indicates that when universities actively endorse and facilitate the use of AI, students are more likely to perceive these tools as beneficial and easy to use, which enhances their intention to adopt them in learning environments. Research indicates that perceived organizational support positively influences students’ intention to use AI language models through mediating factors like perceived usefulness and ease of use (Hoi et al., 2023). Students generally show a favorable perception toward AI tools for autonomous learning, recognizing their potential while acknowledging challenges (Quinde et al., 2024; Zhou et al., 2024). Students who perceive benefits and compatibility with chatbots express stronger intentions to use them academically. Interestingly, some studies found no direct relationship between perceived usefulness, ease of use, and behavioral intention, suggesting other influential factors in AI adoption for educational purposes (Ayanwale and Molefi, 2024). The implementation of AI chatbots in educational settings has improved student support services, providing timely assistance for academic and administrative queries (Abdul Razak et al., 2024). It provides the resources, infrastructure, and training required to help students and educators embrace AI technologies. Universities that prioritize perceived institutional support create an environment conducive to learning innovation, reducing apprehensions about adopting new technologies (Hwang et al., 2020). Students generally view AI positively, seeing it as a tool to enhance learning experiences and increase access to educational resources (Herawati et al., 2024). Perceived usefulness and ease of communication are key factors in the adoption of AI teaching assistants (Kim et al., 2020). AI applications show promise in supporting self-regulated learning, particularly for metacognitive, cognitive, and behavioral regulation (Jin et al., 2023). Students appreciate AI’s potential for personalized assistance, adaptive learning, and immediate feedback, particularly in programming and writing contexts (Sumakul et al., 2022 Keshtkar et al., 2024). Overall, students recognize AI’s benefits in education but acknowledge potential drawbacks, emphasizing the need for balanced integration to maximize advantages while minimizing negative impacts (Maulana et al., 2023). Institutional backing not only facilitates the implementation of AI tools but also addresses potential resistance, thereby encouraging students to explore the benefits of AI-assisted learning systems. However, the study proposes that perceived institutional support can enhance students’ academic performance with AI – supported learning.
Perceived institutional support and technology self-efficacy are critical factors influencing the adoption and effectiveness of AI-supported learning among students. Recent studies have explored the impact of AI tools like ChatGPT on students’ self-efficacy and perceived learning outcomes in higher education. Chatbot support has been found to significantly enhance college students’ self-efficacy (Bation, 2024). ChatGPT aids in writing, research assistance, and idea generation, which can bolster students’ academic performance (Wang et al., 2023). A significant majority of students (70.3%) believe universities should permit AI use, indicating a desire for support in their academic endeavors (Bikanga Ada, 2024). AI tools can improve language comprehension and data collection abilities, contributing positively to students’ research capabilities (Aithal and Aithal, 2023). Critics argue that reliance on AI may diminish critical thinking and problem-solving skills, as students might lean on AI for answers rather than developing their own ideas (Brorsson, 2024). Students generally oppose using ChatGPT for entire assignments but support tools like Grammarly (Johnston et al., 2024). Perceived organizational support plays a crucial role in influencing students’ intention to use AI language models for course learning, mediated by perceived usefulness and ease of use (Hoi et al., 2023). These findings suggest that AI tools can positively impact students’ self-efficacy and learning experiences when integrated thoughtfully into educational practices. Therefore, the study posit that perceived institutional support can increase students’ self- efficacy of AI technology use.
Self-efficacy refers to an individual’s belief in their ability to succeed in specific situations or accomplish a task (Heslin and Klehe, 2006; Schunk, 1984). It plays a crucial role in how goals, tasks, and challenges are approached. Higher technology self-efficacy can lead to improved learning outcomes and better engagement with digital tools (Binti Mohd Nasir, 2023). Students’ self-directed learning correlates with the frequency of digital tool use for learning, and those in technical fields tend to have more favorable attitudes toward digital learning (Popa and Topala, 2018). Students’ confidence in their ability to utilize AI tools significantly influences their learning experiences and outcomes. This self-efficacy is shaped by various factors, including perceived usefulness, ease of use, and trust in AI technologies. Students with higher technology self-efficacy tend to have better technological proficiency and more positive attitudes toward technology use (Dahri et al., 2024).
Self-efficacy mediates the relationship between perceived teacher autonomy support and students’ deep learning (Zhao and Qin, 2021). Technology self-efficacy, in particular, strengthens the positive relationship between online learning environment and student engagement (Owusu-Agyeman, 2021). In the context of AI-supported learning, ICT self-efficacy positively influences students’ perceived ease of use of AI chatbots, while self-directed learning with technology affects both intention and actual use (Wu et al., 2024). Teacher support plays a crucial role in moderating the effects of student expertise on needs satisfaction and intrinsic motivation to learn with AI technologies, particularly in satisfying the need for relatedness (Chiu et al., 2024). Therefore, it’s hypothesized that technology self – efficacy moderates the relationship between perceived institutional support and students’ perception AI – supported learning.
AI tools, such as ChatGPT, improve student satisfaction and perceived learning outcomes by enhancing perceived usefulness and ease of use (Boubker, 2024). AI and mobile learning positively influence perceived learning outcomes, with self-competence acting as a crucial mediator (Priamono et al., 2024). User perceptions of AI-enabled e-learning are shaped by personal learning environments, which affect perceived ease of use and usefulness (Kashive et al., 2021). Intrinsic motivation mediates the relationship between perceived AI learning and computational thinking, emphasizing the importance of student engagement (Martín-Núñez et al., 2023). Intrinsic motivation may also play a significant role in mediating the relationship between AI learning and student outcomes, indicating a complex interplay between external support and internal motivation Furthermore, AI integration in education systems is enhanced through smart learning, which acts as a mediator in improving academic outcomes (Akour, 2024). Hence, learning outcome mediates the relationship between perceived institutional support and students’ perception AI – supported learning (Figure 1).
Based on the above discussion, the study tested the following hypotheses:
H1: Perceived institutional support significantly impacts students’ perceptions of AI-supported learning.
H2: Perceived institutional support significantly impacts technology self – efficacy.
H3: Students’ technology self-efficacy positively moderates the relationship between perceived institutional support and perceptions of AI-supported learning.
H4: Perceived learning outcome mediates the relationship between perceived institutional support and Students’ perceptions of AI-supported learning.
The study employed a quantitative research design to explore student perceptions of AI-supported learning in higher education, focusing on the roles of perceived institutional support, technology self-efficacy, and perceived learning outcomes. The target population of the study are undergraduate students from various institutions in Mogadishu – Somalia. Participants were selected using a convenience sampling technique based on their availability and willingness to participate. Inclusion criteria required participants to have prior experience with AI technologies such as ChatGPT in their educational activities. The structured questionnaire was adapted from previously validated instruments in existing literature. For instance students’ perception of AI - supported learning adapted by Davis (1989) and Khan et al. (2019), perceived institutional support adapted by Lee and Seomun (2016), student technology self – efficacy adapted by Compeau and Higgins (1995), perceived learning outcomes (OECD, 2013). Appendix A list all questionnaire items that were measured with five Likert scale. The survey instrument comprised a structured questionnaire based on a Likert scale, enabling respondents to express their agreement or disagreement with statements related to perceived institutional support, technology self-efficacy, perceptions of AI-supported learning, and perceived learning outcomes. This scale was chosen for its ease of use and ability to capture varying levels of agreement across a wide range of respondents. The study relies on self-reported data collected through participant surveys. While self-reported data is a widely used and convenient method for gathering individual perceptions and experiences, it has inherent limitations that must be acknowledged such as social desirability bias, response consistency pressure and generalizability of the results to other populations (Presser and Stinson, 1998). To mitigate common biases in Likert scale, the survey items were carefully designed to include both positively and negatively worded statements to reduce acquiescence bias. Responses were collected anonymously to encourage honest feedback and reduce social desirability bias (Leong et al., 2019). Moreover, respondents were provided with clear instructions on how to interpret the Likert scale, emphasizing that they should answer based on their true perceptions rather than choosing neutral or socially desirable responses.
G*Power was used to determine the minimum sample size. With the settings of 0.05 for margin errors, 0.95 for statistical power, 0.15 for effect size, and 3 predictors, the minimum sample size calculated to be 119 responses. Before deployment, the Likert scale items were pre-tested for validity and reliability through a pilot study involving 30 respondents. This process ensured the questions were contextually relevant and interpreted consistently by participants. Cronbach’s alpha values were computed for internal consistency, with all constructs exceeding the acceptable threshold of 0.70, ensuring reliability and validity. Data was collected via online kobo toolbox, distributed through WhatsApp groups. Written permission was obtained from Deans and Heads of Departments (HODs) to ensure ethical compliance. The descriptive statistics of the 204 respondents were reported in the Table 1.
The data were analyzed using Structural Equation Modeling (SEM) in SPSS - AMOS to test hypotheses related to direct, moderation, and mediation effects. Descriptive statistics summarized participant demographics, while inferential techniques evaluated relationships between perceived institutional support, technology self-efficacy, perceived learning outcomes, and student perceptions. Goodness-of-fit indices and bootstrapping sampling of 5,000 were applied to validate the SEM model and mediation effects, ensuring a comprehensive understanding of the factors influencing student perceptions of AI-supported learning.
The demographic profile reveals a slightly male-dominated sample, with 58.8% of participants being male (120 participants) and 41.2% female (84 participants). In terms of age distribution, the majority of participants (61.3%) fall within the 18–22 age group, followed by 25.0% in the 23–27 age group, 8.3% in the 28–32 age group, and only 5.4% aged 33 or older. This indicates that the sample is predominantly younger, likely composed of undergraduate students. Regarding the fields of study, Economics is the most represented discipline, comprising 53.4% of the sample (109 participants). Other notable fields include Computer Science (13.7%), Engineering (12.7%), and Public Administration (5.4%). Fields such as Education and Humanities (3.4%), Sharia and Law (2.5%), and Medicine and Surgery (2.0%) are less represented, with the remaining 6.9% categorized as “Other.” This distribution highlights the prominence of Economics and technical disciplines like Computer Science and Engineering within the sample, reflecting the participant recruitment strategy or the general enrollment trends in the institution.
This section presents the findings of the study, analyzed using Structural Equation Modeling (SEM) in SPSS-AMOS. The results are organized as common method bias, inspecting the inner structural model, measurement model, structure model, bootstrapping analysis and evaluation of the model fit.
Common Method Bias (CMB) refers to systematic errors in measurement that arise when data are collected from the same source, potentially inflating or deflating observed relationships among variables. Given that independent and dependent variables were collected at a single point in time and from the same source, Common Method Bias (CMB) may be potential risk in the research (Podsakoff et al., 2012). Addressing this concern is essential to ensure the validity of the findings. Harman’s Single Factor Test was conducted to assess the possible risk of common method bias (CMB) (Ooi et al., 2018). Therefore, the study asserted that a single component accounted for just 43.7% overall variation. Given that the result is below 50% there is concern of CMB in the dataset.
The collinearity statistics for the independent variables were evaluated using Tolerance and Variance Inflation Factor (VIF) to check for potentail multicollinearity issues. The findings show that all variables have Tolerance values greater than 0.1 and VIF values under 5, indicating that the level of collinearity in the model is acceptable (Kim, 2019). Perceived Institutional Support (ISP) (Tolerance = 0.590, VIF =1.696), Technology Self-Efficacy (TSF) (Tolerance = 0.319, VIF = 3.131), and perceived Learning Outcomes (LO) (Tolerance = 0.310, VIF = 3.229) demonstrate no significant multicollinearity concerns. Table 2 presented the result.
The measurement model confirms the reliability and validity of the constructs through factor loadings, square multiple correlations (SMC), Cronbach’s alpha, composite reliability (CR), and average variance extracted (AVE). All factor loadings exceed 0.6. These values exceed the recommended threshold of 0.6, ensuring convergent validity (Gefen and Straub, 2005; Hair et al., 2019). The items SPA1, SPA5, ISP4 were removed from the analysis due to low factor loading. SMC values range between 0.416 and 0.626. All squared multiple correlations (R-square) must be at least 0.40 (Bollen, 1989). And Cronbach’s alpha values are above 0.7, ensuring internal consistency and convergent validity. Composite reliability values (0.760–0.858) exceed the 0.7 threshold (Hair et al., 2012), and AVE values (0.514–0.547) meet the minimum requirement of 0.5 (Fornell and Larcker, 1981a), demonstrating sufficient reliability and validity. Additionally, inter-construct correlations are below the square root of AVE, confirming discriminant validity (Fornell and Larcker, 1981b). These results establish the measurement model’s robustness in supporting the constructs (See Tables 3, 4).
The structural model shows the correlations (paths) between the constructs on the proposed study model. H1 evaluates whether perceived institutional support significantly impacts students’ perceptions of AI-supported learning. The results showed that institutional support positively and significantly influences students’ perceptions of AI-supported learning (β = 0.200, C.R. = 2.291, p = 0.022). This indicates that institutional efforts, such as providing training and resources, are essential for shaping students’ positive perceptions. H2 evaluates whether perceived institutional support is positively related to technology self – efficacy. The findings revealed that perceived institutional support has significance impact on students’ technology self – efficacy (β = 0.492, C.R. = 9.671, p < 0.001). This suggests that higher levels of perceived institutional support associated with increased technology self – efficacy. Moreover, students who feel confident in their technological abilities are more likely to perceive AI-supported learning positively. This finding aligns with previous studies emphasizing the role of institutional support in fostering self-efficacy. For example, research by (Lent et al., 2000) highlighted that institutional resources, guidance, and encouragement contribute positively to individuals’ confidence in their technological skills. The result presented in Table 5.
The study examined the moderating role of technology self-efficacy in the relationship between institutional support and students’ perceptions of AI-supported learning, revealing a negative and significant moderating effect. H3 evaluates whether Students’ technology self-efficacy positively moderates the relationship between perceived institutional support and perceptions of AI-supported learning. The interaction effect between institutional support and technology self-efficacy (β = −0.146, C.R. = −2.507, p = 0.012) revealed a significant negative moderation. This suggests that for students with low technology self-efficacy, institutional support has a stronger impact on their perceptions, as indicated by the steeper slope in the graph (y = 0.692x + 1.507). In contrast, for students with high self-efficacy, institutional support has a weaker influence, as these students already hold positive perceptions irrespective of the level of support (y = 0.108x + 3.293; Figure 2; Table 6).
A mediation analysis was conducted to further assess the dynamic interactions among constructs. H4 evaluates whether Perceived learning outcome mediates the relationship between perceived institutional support and Students’ perceptions of AI-supported learning. The findings indicate that institutional support has both a significant direct effect on perceived learning outcomes (β = 0.155, p < 0.001) and a stronger indirect effect on students’ perceptions of AI-supported learning mediated through perceived learning outcomes [β =0.539, 95% CI (0.235, 0.549), p < 0.001]. This result confirms the statistical significance of the mediation pathway, showing that institutional support significantly enhances students’ perceptions of AI-supported learning through its impact on perceived learning outcomes. This emphasizes the importance of designing institutional support mechanisms that not only promote AI integration but also improve tangible perceived learning outcomes, thereby fostering positive perceptions among students (Tables 7, 8).
The structural equation model (SEM) presented in the figure demonstrates the relationships between constructs, supported by model fit indices indicating an overall good fit. The Chi-Square value (χ2 = 196.370, DF = 113, p = 0.000) is significant, which may reflect sensitivity to sample size rather than poor fit. The relative Chi-Square (χ2/DF = 1.738) falls below the acceptable threshold of 3, suggesting a good fit between the model and the data (Kline, 2023). The Goodness-of-Fit Index (GFI = 0.901) and Comparative Fit Index (CFI = 0.950) exceed the recommended cutoff of 0.9, indicating excellent fit (Bollen, 1989). Similarly, the Incremental Fit Index (IFI = 0.950) and Tucker-Lewis Index (TLI = 0.940) also meet the criteria for a good fit. The Adjusted Goodness-of-Fit Index (AGFI = 0.866) and Normed Fit Index (NFI = 0.891) are slightly below.9 but still suggest an acceptable fit. Lastly, the Root Mean Square Error of Approximation (RMSEA = 0.060) is below the threshold of.08, further supporting good model fit (Steiger, 1990). Taken together, these indices confirm that the model adequately represents the observed data, with only minor deviations in AGFI and NFI (Figure 3).
This study aimed to explore student perceptions of AI-supported learning in higher education, specifically examining the roles of perceived institutional support, technology self-efficacy, and their influence on perceived learning outcomes. The findings contribute to the growing body of literature on AI integration in educational settings by shedding light on key factors that influence student engagement and learning.
The study revealed that student perceptions of AI-supported learning were positively associated with both perceived institutional support and technology self-efficacy. This finding aligns with prior research by Hu (2022) and Wang et al. (2024), who highlighted that institutional readiness and robust technology infrastructure significantly influence how students perceive AI tools in education. Institutions that invest in adequate resources, technical support, and AI-related training create a conducive environment for technology adoption. Students’ positive perceptions were influenced by their belief in the institution’s capacity to provide adequate resources and training, consistent with Deng and Benckendorff (2022), who emphasized the need for a supportive environment for technology adoption in higher education.
Perceived institutional support was found to significantly impact perceived learning outcomes. This finding is consistent with the works of Chatterjee and Bhattacharjee (2020), Mohd Rahim et al. (2022), and Nagy et al. (2024) who demonstrated that institutional efforts such as faculty development programs, accessible IT support, and tailored student services enhance satisfaction and academic performance. These results underscore the importance of institutions addressing barriers to technology use, including limited training or technical challenges, as part of a broader strategy to integrate AI into higher education curricula.
Technology self-efficacy emerged as a critical factor influencing both student perceptions of AI-supported learning and perceived learning outcomes. Students with higher self-efficacy demonstrated greater confidence in utilizing AI tools, resulting in enhanced learning experiences. This aligns with the findings of Luckin and Holmes (2016) and Yavuzalp and Bahcivan (2020), who emphasized the predictive power of self-efficacy in determining students’ adaptability to emerging technologies. Additionally, the current results align with the findings of Wang and Li (2024), who reported that self-efficacy positively mediates the relationship between technology adoption and academic success. These findings highlight the need for educational institutions to foster students’ confidence in leveraging AI tools through workshops, hands-on training, and interactive AI-based platforms.
Furthermore, the analysis revealed perceived learning outcomes mediates the relationship between perceived institutional support and student perceptions of AI-supported learning. This finding is consistent with Xu (2024) who identified that integrating AI tools, such as adaptive learning systems, enhances students’ engagement, problem-solving skills, and overall academic performance. The study further confirms the role of institutional and self-efficacy factors in facilitating positive perceived learning outcomes, echoing the conclusions of Delita et al. (2022), who emphasized the combined influence of technological infrastructure and student confidence on learning achievements.
Theoretically, the study contributes to the growing body of literature on AI-supported learning in higher education by examining the interplay between perceived institutional support, technology self-efficacy, and students’ perceptions. First, perceived institutional support significantly impacts on students’ perceptions of AI-supported learning highlights the importance of institutional frameworks in shaping how students engage with AI technologies. Second, the significant relationship between perceived institutional support and technology self-efficacy, enriches self-efficacy theory by demonstrating how organizational support can enhance individuals’ confidence in using AI tools. Moreover, the moderating role of technology self-efficacy on the relationship between perceived institutional support and perceptions of AI-supported learning provides insights into the interaction between personal and environmental factors, which aligns with the Social Cognitive Theory (SCT). Lastly, the mediating role of perceived learning outcomes in the relationship between perceived institutional support and students’ perceptions adds to the understanding of how outcomes act as a conduit for institutional influence in the educational technology adoption process. From a practical perspective, this study offers actionable insights for higher education institutions aiming to enhance the adoption and efficacy of AI-supported learning. First, universities should focus on reinforcing perceived institutional support mechanisms, such as providing adequate resources, AI-related training, and technical support, to positively influence students’ perceptions and engagement. This includes creating an enabling environment that facilitates access to AI tools and aligns them with the learning goals of students. Second, fostering technology self-efficacy among students is crucial. Institutions can achieve this through workshops, hands-on training, and integration of AI applications into the curriculum, ensuring students feel competent and confident in leveraging these technologies. Third, the significant moderation by technology self-efficacy implies that tailored interventions may be required for students with varying levels of technological proficiency. Programs designed to build self-efficacy among low-tech-experienced students may yield greater equity in AI adoption outcomes. Finally, the mediating role of learning outcomes highlights the necessity of aligning AI-supported learning tools with tangible educational objectives. Institutions should assess the impact of AI tools on academic performance and ensure these technologies add measurable value to students’ learning journeys. These insights collectively provide a roadmap for higher education stakeholders to integrate AI technologies effectively while enhancing students’ educational experiences.
This study highlights the importance of perceived institutional support and technology self-efficacy in shaping students’ perceptions and outcomes in AI-supported learning environments. The findings suggest that higher education institutions should prioritize providing adequate resources, training, and guidance to foster positive student experiences with AI tools. Additionally, enhancing students’ self-efficacy can further facilitate the successful adoption of AI technologies, leading to improved learning outcomes. These insights offer practical implications for institutions seeking to implement AI in their educational systems while contributing to the theoretical understanding of technology integration in higher education.
The study has limitations that provide opportunities for future research. The focus on higher education institutions in Somalia may limit the generalizability of findings to other contexts, suggesting the need for cross-cultural and multi-regional studies. The cross-sectional design captures perceptions at a single point in time, calling for longitudinal studies to understand changes over time. Self – reported data has limitations, future research could complement self-reported data with objective measures, such as system usage logs, academic performance records, or third-party observations, to enhance the robustness of findings. While technology self-efficacy and learning outcomes were considered as moderating and mediating factors, variables such as digital literacy, faculty attitudes, accessibility challenges, and ethical concerns remain unaddressed. Addressing these gaps can enrich theoretical understanding and inform practical applications in AI-supported education.
The original contributions presented in the study are included in the article/supplementary material, further inquiries can be directed to the corresponding author/s.
The studies involving humans were approved by Mogadishu University - Postgraduate Program and Research. The studies were conducted in accordance with the local legislation and institutional requirements. The participants provided their written informed consent to participate in this study.
AJ: Data curation, Methodology, Supervision, Writing – original draft, Writing – review & editing. SA: Supervision, Project administration, Investigation, Data curation, Conceptualization, Formal analysis, Writing – original draft, Writing – review & editing.
The author(s) declare that no financial support was received for the research, authorship, and/or publication of this article.
The authors declare that the research was conducted in the absence of any commercial or financial relationships that could be construed as a potential conflict of interest.
The authors declare that Generative AI was used in the creation of this manuscript. ChatGPT was used for language refinement, improving clarity, and suggesting alternative phrasing. However, all conceptual ideas, data analysis, and interpretations remain the authors’ original work.
All claims expressed in this article are solely those of the authors and do not necessarily represent those of their affiliated organizations, or those of the publisher, the editors and the reviewers. Any product that may be evaluated in this article, or claim that may be made by its manufacturer, is not guaranteed or endorsed by the publisher.
Abas, M. A., Arumugam, S. E., Yunus, M. M., and Rafiq, M. (2023). ChatGPT and personalized learning: opportunities and challenges in higher education. Int. J. Acad. Res. Bus. Soc. Sci. 13, 3536–3545. doi: 10.6007/ijarbss/v13-i12/20240
Abdul Razak, M. S., Manoj Kumar, M. V., Nirmala, C. R., Naseer, R., Prashanth, B. S., and Sneha, H. R. (2024). Enhancing student support with AI: a college assistance Chatbot using NLP and ANN. 2nd IEEE international conference on networks, multimedia and information technology, NMITCON 2024, pp. 1–8.
Abubakar, U. (2024). The influence of technology-integrated curriculum resources on student engagement and academic achievement in higher education. Adv. Mob. Learn. Educ. Res. 4, 1208–1223. doi: 10.25082/AMLER.2024.02.014
Abulibdeh, A., Zaidan, E., and Abulibdeh, R. (2024). Navigating the confluence of artificial intelligence and education for sustainable development in the era of industry 4.0: challenges, opportunities, and ethical dimensions. J. Clean. Prod. 437:140527. doi: 10.1016/j.jclepro.2023.140527
Adel, A., Ahsan, A., and Davison, C. (2024). ChatGPT promises and challenges in education: computational and ethical perspectives. Educ. Sci. 1, 14. doi: 10.3390/educsci14080814
Aithal, P. S., and Aithal, S. (2023). Application of ChatGPT in higher education and research – a futuristic analysis. Int. J. Appl. Eng. Manag. Lett. 7, 168–194. doi: 10.47992/ijaeml.2581.7000.0193
Alam, A. (2023). “Harnessing the power of AI to create intelligent tutoring Systems for Enhanced Classroom Experience and Improved Learning Outcomes” in Lecture notes on data engineering and communications technologies. eds. G. Rajakumar, K.-L. Du, and Á. Rocha, vol. 171 (Singapore: Springer Nature), 571–591.
Alqahtani, T., Badreldin, H. A., Alrashed, M., Alshaya, A. I., Alghamdi, S. S., Bin Saleh, K., et al. (2023). The emergent role of artificial intelligence, natural learning processing, and large language models in higher education and research. Res. Soc. Adm. Pharm. 19, 1236–1242. doi: 10.1016/j.sapharm.2023.05.016
Al-Rahmi, W. M., Uddin, M., Alkhalaf, S., Al-Dhlan, K. A., Cifuentes-Faura, J., Al-Rahmi, A. M., et al. (2022). Validation of an integrated IS success model in the study of E-government. Mob. Inf. Syst. 2022, 1–16. doi: 10.1155/2022/8909724
Ayanwale, M. A., and Molefi, R. R. (2024). Exploring intention of undergraduate students to embrace chatbots: from the vantage point of Lesotho. Int. J. Educ. Technol. High. Educ. 21:451. doi: 10.1186/s41239-024-00451-8
Bandura, A. (1986). Social foundations of thought and action a Social Cognitive Theory. Available at: http://ereserve.library.utah.edu/Annual/PSY/3960/Gelfand/social1.pdf
Bandura, A. (2001). Social cognitive theory: an agentic perspective. Annu. Rev. Psychol. 52, 1–26. doi: 10.1146/annurev.psych.52.1.1
Baranowski, T., Perry, C., and Parcel, G. (2002). “How individuals, environments, and health behavior interact” in Health Behavior and Health Education. eds. K. Glanz, B. K. Rimer, and K. Viswanath (San Francisco, CA: Jossey-Bass), 165–184.
Bation, D. N. (2024). The perceived value of Chatbot support in enhancing college student self-efficacy. Int. J. Soc. Sci. Hum. Res. 7, 356–360. doi: 10.47191/ijsshr/v7-i01-47
Bettayeb, A. M., Abu Talib, M., Sobhe Altayasinah, A. Z., and Dakalbab, F. (2024). Exploring the impact of ChatGPT: conversational AI in education. Front. Educ. 9:796. doi: 10.3389/feduc.2024.1379796
Bikanga Ada, M. (2024). It helps with crap lecturers and their low effort: investigating computer science students’ perceptions of using ChatGPT for learning. Educ. Sci. 14:1106. doi: 10.3390/educsci14101106
Binti Mohd Nasir, P. N. S. (2023). University students’ perception of digital Technology in Self-Directed English Learning: types and effectiveness. Int. J. Acad. Res. Prog. Educ. Dev. 12, 2118–2131. doi: 10.6007/ijarped/v12-i2/17486 Nesamany, S. S.
Bollen, K. A. (1989). A new incremental fit index for general structural equation models. Sociol. Methods Res. 17, 303–316. doi: 10.1177/0049124189017003004
Boubker, O. (2024). From chatting to self-educating: can AI tools boost student learning outcomes? Expert Syst. Appl. 238:121820. doi: 10.1016/j.eswa.2023.121820
Brorsson, N. A. J. (2024). Generative AI in higher education: educators’ perspectives on academic learning and integrity. Eur. Conf. E Learn. 23:406:414. doi: 10.34190/ecel.23.1.3090
Chatterjee, S., and Bhattacharjee, K. K. (2020). Adoption of artificial intelligence in higher education: a quantitative analysis using structural equation modelling. Educ. Inf. Technol. 25, 3443–3463. doi: 10.1007/s10639-020-10159-7
Chiu, T. K. F., Moorhouse, B. L., Chai, C. S., and Ismailov, M. (2024). Teacher support and student motivation to learn with Artificial Intelligence (AI) based chatbot. Interactive Learning Environments, 32, 3240–3256. doi: 10.1080/10494820.2023.2172044
Compeau, D. R., and Higgins, C. A. (1995). Computer self-efficacy: development of a measure and initial test. MIS Q. 19, 189–210. doi: 10.2307/249688
Dahri, N. A., Yahaya, N., Al-Rahmi, W. M., Aldraiweesh, A., Alturki, U., Almutairy, S., et al. (2024). Extended TAM based acceptance of AI-Powered ChatGPT for supporting metacognitive self-regulated learning in education: A mixed-methods study. Heliyon, 10, e29317. doi: 10.1016/j.heliyon.2024.e29317
Davis, F. D. (1989). Perceived usefulness, perceived ease of use, and user acceptance of information technology. MIS Q. 13, 319–339. doi: 10.2307/249008
Delita, F., Berutu, N., and Nofrion, N. (2022). Online learning: the effects of using E-modules on self-efficacy, motivation and learning outcomes. Turk. Online J. Dist. Educ. 23, 93–107. doi: 10.17718/tojde.1182760
Deng, R., and Benckendorff, P. (2022). “Technology-enabled learning” in Handbook of e-tourism. eds. Z. Xiang, M. Fuchs, U. Gretzel, and W. Höpken (Berlin: Springer International Publishing), 1687–1713.
Fornell, C., and Larcker, D. F. (1981a). Evaluating structural equation models with unobservable variables and measurement error. J. Mark. Res. 18:39. doi: 10.2307/3151312
Fornell, C., and Larcker, D. F. (1981b). Evaluating structural equation models with unobservable variables and measurement error. J. Mark. Res. 18, 39–50. doi: 10.1177/002224378101800104
Gefen, D., and Straub, D. (2005). A practical guide to factorial validity using PLS-graph: tutorial and annotated example. Commun. Assoc. Inf. Syst. 16:1605. doi: 10.17705/1cais.01605
Glanz, K. (2001). Current theoretical bases for nutrition intervention and their uses. In A. M. Coulston and C. L. Rock, Monsen, D. Nutrition in the prevention and treatment of disease. (pp. 83–93). Cambridge, MA: Academic Press.
Graefen, B., and Fazal, N. (2024). Chat bots to virtual tutors: an overview of chat GPT's role in the future of education. Arch. Pharm. Pract. 15, 43–52. doi: 10.51847/touppjedsx
Hair, J. F., Risher, J. J., Sarstedt, M., and Ringle, C. M. (2019). When to use and how to report the results of PLS-SEM. Eur. Bus. Rev. 31, 2–24. doi: 10.1108/EBR-11-2018-0203
Hair, J. F., Sarstedt, M., Ringle, C. M., and Mena, J. A. (2012). An assessment of the use of partial least squares structural equation modeling in marketing research. J. Acad. Mark. Sci. 40, 414–433. doi: 10.1007/s11747-011-0261-6
Herawati, A. A., Yusuf, S., Ilfiandra, I., Taufik, A., and Ya Habibi, A. S. (2024). Exploring the role of artificial intelligence in education, students preferences and perceptions. AL-ISHLAH 16, 1029–1040. doi: 10.35445/alishlah.v16i2.4784
Heslin, P. A., and Klehe, U. C. (2006). Self-efficacy how self-efficacy affects performance and well-being. Encyclopedia of Industrial/Organizational Psychology, no. 2, 705–708. Available online at: http://ssrn.com/abstract=1150858
Hoi, S., Yu, Y., and Ye, L. (2023). How perceived organizational support influences university Students45 intention to use AI language models in course learning: an exploratory study based on the technology acceptance model. ACM International Conference Proceeding Series, pp. 781–786.
Hu, Y.-H. (2022). Effects and acceptance of precision education in an AI-supported smart learning environment. Educ. Inf. Technol. 27, 2013–2037. doi: 10.1007/s10639-021-10664-3
Hwang, G. J., Xie, H., Wah, B. W., and Gašević, D. (2020). Vision, challenges, roles and research issues of artificial intelligence in education. Comput. Educ. 1, 100001–100005. doi: 10.1016/j.caeai.2020.100001
Jin, S. H., Im, K., Yoo, M., Roll, I., and Seo, K. (2023). Supporting students’ self-regulated learning in online learning using artificial intelligence applications. Int. J. Educ. Technol. High. Educ. 20:406. doi: 10.1186/s41239-023-00406-5
Johnston, H., Wells, R. F., Shanks, E. M., Boey, T., and Parsons, B. N. (2024). Student perspectives on the use of generative artificial intelligence technologies in higher education. Int. J. Educ. Integr. 20, 1–21. doi: 10.1007/s40979-024-00149-4
Kashive, N., Powale, L., and Kashive, K. (2021). Understanding user perception toward artificial intelligence (AI) enabled e-learning. Int. J. Inf. Learn. Technol. 38, 1–19. doi: 10.1108/IJILT-05-2020-0090
Keshtkar, F., Rastogi, N., Chalarca, S., and Bukhari, S. A. C. (2024). AI Tutor: Student’s Perceptions and Expectations of AI-Driven Tutoring Systems: A Survey-Based Investigation. Proceedings of the International Florida Artificial Intelligence Research Society Conference, FLAIRS, 37. doi: 10.32473/flairs.37.1.135314
Khan, S. Q., Al-Shahrani, M., Khabeer, A., Farooqi, F. A., Alshamrani, A., Alabduljabbar, A. M., et al. (2019). Medical students’ perception of their educational environment at imam Abdulrahman Bin Faisal University, Kingdom of Saudi Arabia. J. Fam. Community Med. 26, 45–50. doi: 10.4103/jfcm.JFCM_12_18
Kim, J. H. (2019). Multicollinearity and misleading statistical results. Korean J. Anesthesiol. 72, 558–569. doi: 10.4097/kja.19087
Kim, J., Merrill, K., Xu, K., and Sellnow, D. D. (2020). My teacher is a machine: understanding students’ perceptions of AI teaching assistants in online education. Int. J. Hum. Comput. Interact. 36, 1902–1911. doi: 10.1080/10447318.2020.1801227
Lee, Y., and Seomun, G. A. (2016). Development and validation of an instrument to measure nurses’ compassion competence. Appl. Nurs. Res. 30, 76–82. doi: 10.1016/j.apnr.2015.09.007
Lent, R. W., Brown, S. D., and Hackett, G. (2000). Contextual supports and barriers to career choice: a social cognitive analysis. J. Couns. Psychol. 47, 36–49. doi: 10.1037/0022-0167.47.1.36
Leong, L.-Y., Hew, T.-S., Ooi, K.-B., and Tan, G. W.-H. (2019). Predicting actual spending in online group buying – an artificial neural network approach. Electron. Commer. Res. Appl. 38:100898. doi: 10.1016/j.elerap.2019.100898
Luckin, R., and Holmes, W. (2016). Intelligence unleashed: An argument for AI in education. In UCL Knowledge Lab: London, UK. Available online at: https://www.pearson.com/content/dam/corporate/global/pearson-dot-com/files/innovation/Intelligence-Unleashed-Publication.pdf.
Martín-Núñez, J. L., Ar, A. Y., Fernández, R. P., Abbas, A., and Radovanović, D. (2023). Does intrinsic motivation mediate perceived artificial intelligence (AI) learning and computational thinking of students during the COVID-19 pandemic? Computers and education. Artif. Intell. 4:128. doi: 10.1016/j.caeai.2023.100128
Maulana, A., Noviandy, T. R., Suhendra, R., Earlia, N., Bulqiah, M., Idroes, G. M., et al. (2023). Evaluation of atopic dermatitis severity using artificial intelligence. Narra J 3:e511. doi: 10.52225/narra.v3i3.511
Mohd Rahim, N. I., Iahad, A. N., Yusof, A. F., and Al-Sharafi, M. A. (2022). AI-based Chatbots adoption model for higher-education institutions: a hybrid PLS-SEM-neural network modelling approach. Sustainability (Switzerland) 14:2726. doi: 10.3390/su141912726
Nagy, A. S., Tumiwa, J. R., Arie, F. V., and Erdey, L. (2024). An exploratory study of artificial intelligence adoption in higher education. Cogent Educ. 11:892. doi: 10.1080/2331186X.2024.2386892
OECD. (2013). PISA 2012 results: excellence through equity: giving every student the chance to succeed (volume II). In: Compare: A journal of comparative and international education, No. 35.
Ooi, K.-B., Lee, V.-H., Tan, G. W.-H., Hew, T.-S., and Hew, J.-J. (2018). Cloud computing in manufacturing: the next industrial revolution in Malaysia? Expert Syst. Appl. 93, 376–394. doi: 10.1016/j.eswa.2017.10.009
Ouyang, F., Wu, M., Zhang, L., Xu, W., Zheng, L., and Cukurova, M. (2023). Making strides towards AI-supported regulation of learning in collaborative knowledge construction. Comput. Hum. Behav. 142:107650. doi: 10.1016/j.chb.2023.107650
Owusu-Agyeman, Y., and Amoakohene, G. (2021). Student engagement and perceived gains in transnational education in Ghana. International Journal of Comparative Education and Development, 23, 297–316. doi: 10.1108/IJCED-11-2020-0085
Podsakoff, P. M., MacKenzie, S. B., and Podsakoff, N. P. (2012). Sources of method bias in social science research and recommendations on how to control it. Annu. Rev. Psychol. 63, 539–569. doi: 10.1146/annurev-psych-120710-100452
Popa, D., and Topala, I. R. (2018). Students’ digital competencies, related attitudes and self directed learning. 14th International Conference ELearning and Software for Education, no. 3, pp. 90–95.
Presser, S., and Stinson, L. (1998). Data collection mode and social desirability Bias in self-reported religious attendance author (s): Stanley Presser and Linda Stinson source: American sociological review, published by: American Sociolo. Am. Sociol. Rev. 63, 137–145. doi: 10.2307/2657486
Priamono, G. H., Hakim, A. R., and Daryono, R. W. (2024). The influence of artificial intelligence (AI) and Mobile learning on learning outcomes in higher education: did the mediation of self-competence matter? J. Penelitian Pengkajian Ilmu Pendidikan 8, 241–259. doi: 10.36312/esaintika.v8i2.1902
Quinde, G. A. L., Muñoz, M. Y. T., Suárez, J. M. R., Villarreal, R. E. P., Vélez, W. A. Z., and Laínez, A. A. D. (2024). Perception of university students on the use of artificial intelligence (AI) tools for the development of autonomous learning. Rev. Gestão Soc. Ambiental 18:e06170. doi: 10.24857/rgsa.v18n2-136
Rerhaye, L., Altun, D., Krauss, C., and Müller, C. (2021). “Evaluation methods for an AI-supported learning management system: quantifying and qualifying added values for teaching and learning BT - adaptive instructional systems” in Design and evaluation. eds. R. A. Sottilare and J. Schwarz (Berlin: Springer International Publishing), 394–411.
Sajja, R., Sermet, Y., Cikmaz, M., Cwiertny, D., and Demir, I. (2023). Artificial intelligence-enabled intelligent assistant for personalized and adaptive learning in higher education. Informations 15:596. doi: 10.3390/info15100596
Schunk, D. H. (1984). Self-efficacy perspective on achievement behavior. Educ. Psychol. 19, 48–58. doi: 10.1080/00461528409529281
Steiger, J. H. (1990). Structural model evaluation and modification: an interval estimation approach. Multivar. Behav. Res. 25, 173–180. doi: 10.1207/s15327906mbr2502_4
Sumakul, D. T. Y. G., Hamied, F. A., and Sukyadi, D. (2022). Students’ Perceptions of the Use of AI in a Writing Class. Proceedings of the 67th TEFLIN International Virtual Conference & the 9th ICOELT 2021 (TEFLIN ICOELT 2021), 624, 52–57. doi: 10.2991/assehr.k.220201.009
Teo, T., Zhou, M., Fan, A. C. W., and Huang, F. (2019). Factors that influence university students’ intention to use Moodle: a study in Macau. Educ. Technol. Res. Dev. 67, 749–766. doi: 10.1007/s11423-019-09650-x
Wang, Y., Cao, Y., Gong, S., Wang, Z., Li, N., and Ai, L. (2022). Interaction and learning engagement in online learning: the mediating roles of online learning self-efficacy and academic emotions. Learn. Individ. Differ. 94:102128. doi: 10.1016/j.lindif.2022.102128
Wang, X., and Li, P. (2024). Assessment of the relationship between music students’ self-efficacy, academic performance and their artificial intelligence readiness. Eur. J. Educ. 59:761. doi: 10.1111/ejed.12761
Wang, X., Liu, Q., Pang, H., Tan, S. C., Lei, J., Wallace, M. P., et al. (2023). What matters in AI-supported learning: a study of human-AI interactions in language learning using cluster analysis and epistemic network analysis. Comput. Educ. 194:104703. doi: 10.1016/j.compedu.2022.104703
Wang, X., Pang, H., Wallace, M. P., Wang, Q., and Chen, W. (2024). Learners’ perceived AI presences in AI-supported language learning: a study of AI as a humanized agent from community of inquiry. Comput. Assist. Lang. Learn. 37, 814–840. doi: 10.1080/09588221.2022.2056203
Wang, S., Sun, Z., and Chen, Y. (2023). Effects of higher education institutes’ artificial intelligence capability on students’ self-efficacy, creativity and learning performance. Educ. Inf. Technol. 28, 4919–4939. doi: 10.1007/s10639-022-11338-4
Wu, D., Zhang, S., Ma, Z., Yue, X.-G., and Dong, R. K. (2024). Unlocking potential: key factors shaping undergraduate self-directed learning in AI-enhanced educational environments. Systems 12:332. doi: 10.3390/systems12090332
Xu, Z. (2024). AI in education: enhancing learning experiences and student outcomes. Appl. Comput. Eng. 51, 104–111. doi: 10.54254/2755-2721/51/20241187
Yavuzalp, N., and Bahcivan, E. (2020). The online learning self-efficacy scale: its adaptation into Turkish and interpretation according to various variables. Turk. Online J. Dist. Educ. 21, 31–44. doi: 10.17718/TOJDE.674388
Zhao, W., Huang, S., and Yan, L. (2024). ChatGPT and the future of translators: overview of the application of interactive AI in English translation teaching. 2024 4th international conference on computer communication and artificial intelligence (CCAI), pp. 303–307.
Zhao, J., and Qin, Y. (2021). Perceived teacher autonomy support and students’ deep learning: the mediating role of self-efficacy and the moderating role of perceived peer support. Front. Psychol. 12, 1–11. doi: 10.3389/fpsyg.2021.652796
Zhou, X., Zhang, J., and Chan, C. (2024). Unveiling students’ experiences and perceptions of artificial intelligence usage in higher education. Journal of university teaching and learning. Practice 21:23. doi: 10.53761/xzjprb23
Gender: Male [] Female [] Age: 18–22, 23–27, 28–32, 33+; Field: Economics; Computer Science, public Admin, Education and Humanities, Sharia and Law, Medicine and Surgery, Engineering and others.
Keywords: perceived institutional support, AI-supported learning, technology self-efficacy, perceived learning outcomes, higher education justified
Citation: Jeilani A and Abubakar S (2025) Perceived institutional support and its effects on student perceptions of AI learning in higher education: the role of mediating perceived learning outcomes and moderating technology self-efficacy. Front. Educ. 10:1548900. doi: 10.3389/feduc.2025.1548900
Received: 20 December 2024; Accepted: 17 February 2025;
Published: 13 March 2025.
Edited by:
Musa Adekunle Ayanwale, University of Johannesburg, South AfricaReviewed by:
Hui Luan, National Taiwan Normal University, Taipei, TaiwanCopyright © 2025 Jeilani and Abubakar. This is an open-access article distributed under the terms of the Creative Commons Attribution License (CC BY). The use, distribution or reproduction in other forums is permitted, provided the original author(s) and the copyright owner(s) are credited and that the original publication in this journal is cited, in accordance with accepted academic practice. No use, distribution or reproduction is permitted which does not comply with these terms.
*Correspondence: Abdulkadir Jeilani, YS5qZWlsYW5pQG11LmVkdS5zbw==
Disclaimer: All claims expressed in this article are solely those of the authors and do not necessarily represent those of their affiliated organizations, or those of the publisher, the editors and the reviewers. Any product that may be evaluated in this article or claim that may be made by its manufacturer is not guaranteed or endorsed by the publisher.
Research integrity at Frontiers
Learn more about the work of our research integrity team to safeguard the quality of each article we publish.