- 1HUN-REN-ELTE Theoretical Physics Research Group, Budapest, Hungary
- 2MTA Atomki Lendület Quantum Correlations Research Group, HUN-REN Institute for Nuclear Research, Debrecen, Hungary
- 3HUN-REN Institute of Earth Physics and Space Science (EPSS), Sopron, Hungary
- 4Department of Theoretical Physics, Eötvös University, Budapest, Hungary
- 5Max Planck Institute for the Physics of Complex Systems, Dresden, Germany
- 6Department of Water and Environmental Policy, Faculty of Water Sciences, University of Public Service, Budapest, Hungary
We address the hypothetical question of whether an increasing total solar irradiance (TSI) trend, without anthropogenic contributions, could be sufficient to explain the ongoing global warming. To this end, the intermediate-complexity climate model PlaSim is used. To consider the total internal variability, we present a set of ensemble simulations, with different forcing histories in TSI and CO2 concentration, that have converged sufficiently tightly to the relevant probability distributions to provide a satisfactory bound on any spurious trend possibly arising from a sampling bias; similar bounds on any other unforced contributions to ensemble mean trends are also estimated. A key point is the consideration, among the forcing histories, the steepest increasing trend in TSI that is still consistent with observations according to a recent study; thereby, we essentially revisit corresponding TSI reconstructions, more than 20 years after their last modeling-based evaluation, by improving the analysis through taking care of all possible sources of error or uncertainty and incorporating data that have become available since then. Without any change in CO2 concentration, our TSI trend (i.e., and upper bound on actual TSI trends) is found to be insufficient to produce outcomes compatible with the observational record in global mean surface temperature (GMST) with a nonnegligible probability. We formalize our statement for quantifiers of GMST trends through evaluating their distributions over the ensemble, and we speculate that the hypothesis about the exclusive role of an increasing TSI remains implausible even beyond our particular model setup. At the same time, if we consider a constant TSI, and the observational record in CO2 concentration is applied as forcing, the simulation results and the recorded GMST match well. While we currently need to leave the question of a precise attribution open, we conclude by pointing out that an attribution of the ongoing global warming to an increasing TSI alone could be made plausible only if a bias in the set of land-based instrumental temperature measurements were increasing more rapidly than commonly estimated; an assessment of the latter possibility is out of the scope of our study, as well as addressing solar forcing mechanisms beyond the effect of TSI.
1 Introduction
In a recent study, Connolly et al. (2021) revisited the idea that the current warming trend in the Northern Hemisphere might be driven by changes in solar irradiance. They even suggested that the role of anthropogenic forcing could be negligible, aligning with several previous publications (Soon et al., 1999; Alexander and Bailey, 2007; Scafetta, 2012; Soon and Legates, 2013), although their possible conclusions cover a fairly wide spectrum, encompassing all possibilities from no influence of the Sun to no influence of human activity (Connolly et al., 2021). It is worth noting that the latter possibility has been demonstrated to be a result of an erroneous use of statistics (Richardson and Benestad, 2022), although a modified analysis performed in a subsequent publication by Connolly et al. (2023) appears to confirm the same possibility. Irrespective of the final conclusion in this respect, Connolly et al. (2021) highlighted the unexpected inconsistency in estimates of the total solar irradiance (TSI), previously known as the solar constant (Kopp, 2014; Dudok de Wit et al., 2017; Gueymard, 2018; Roy, 2018; Connolly et al., 2021; Montillet et al., 2022). While measurements of the CO2 concentration worldwide exhibit a firm consistency (Scripps Institute, 2023), the spread in the estimated changes of TSI justifies a dedicated investigation into the corresponding possible impacts on climate.
The decomposition of various forces driving the time evolution of climate system variables (i.e., attribution of observed changes to specific forcings) is usually attempted by two general classes of methodologies. The first is time series analysis, where the traditional approach involves multivariate auto-regressive fits and their variants (see, e.g., Qian et al., 2021, and references therein). The second class is based on extensive numerical simulations using global climate models (e.g., Stott et al., 2000; Folland et al., 2018; Hegerl et al., 2019). Both methods aim to reproduce measured variables (primarily annual global mean surface temperature over continents and oceans, GMST). The basic difference between the two approaches is that while time series analysis is entirely based on the target and input variables and relies on a proper weighting of different forcing components via a parameter fit, numerical simulations attempt to use the physical equations and relations, along with a tuning of parameterizations to represent reality, for the reproduction. In the former approach, it is possible to try to precisely reproduce the observed signal by taking into account internal processes (e.g., ENSO, AMO, AO) beyond external forcing as drivers of changes, while in the latter one, only purely external forcing factors can be used for the reproduction (e.g., TSI, CO2 concentration, aerosol concentration related to air pollution and volcano eruptions) with some “room” left for internal variability, which may mask the forced features of the signal and thus make a direct comparison difficult. This kind of uncertainty is often quantified through sampling internal variability in preindustrial control runs (e.g., Hegerl and Zwiers, 2011), which may be a good first approximation, but internal variability itself responds to forcing, which means that it is changing during a forced climate change (e.g., Herein et al., 2016). For this reason, a better methodology is desirable, which is provided by an ensemble of simulations differing in their initial conditions. This approach to represent internal variability in comparison with the observed signal at any time instant is used, mostly among other approaches, in Stott et al. (2000); Tett et al. (2002); Meehl et al. (2003); Hegerl et al. (2007); Jones et al. (2013); Bindoff et al. (2013); Gillett et al. (2021); Eyring et al. (2021) [see, in particular, Figure 1 of FAQ 9.2 in Hegerl et al. (2007), Figure 1 of FAQ 10.1, together with Figure 10.7, in Bindoff et al. (2013) and Figure 1 of FAQ 3.1, together with Figure 3.9, in Eyring et al. (2021)]. Our study applies the same kind of methodology, and our aim is to revisit a hypothesized exclusive role of an increasing TSI in the observed global warming such that possible pitfalls, to be detailed below, are avoided and confidence in the conclusion is improved.
Such an exclusive role could also be ruled out by a statistically significant detection of a contribution from anthropogenic greenhouse gases. This is widely believed to already have been performed by (variants of) optimal fingerprinting (Allen and Tett, 1999; Allen and Stott, 2003; Huntingford et al., 2006; Ribes et al., 2013), which have become the dominating tool for model-based detection and attribution in the last decades (e.g., Tett et al., 1999; Hegerl et al., 2019; Gillett et al., 2021; Eyring et al., 2021, and see references therein). As an appealing feature, this approach to detection permits an arbitrary rescaling of any ensemble mean model response or any forcing signal. Under suitable processing choices (such as temporal averaging in windows nearly covering the 11-year solar cycle), it may be expected to be unaffected by the reported uncertainties in the magnitude of some long-term increasing trend in TSI; however, it has turned out to be sensitive to the details of the TSI time series used (Stott et al., 2003). Notwithstanding, this is not the main reason why we do not fully trust this methodology and seek independent confirmation. Instead, we detail our concerns as follows.
First of all, optimal fingerprinting relies on a relatively accurate model representation of the spatial pattern of the climate system’s response to each significant forcing factor; a relaxation has been proposed but associating rather idealized properties with the model errors (Huntingford et al., 2006; Hannart et al., 2014). (The “pattern” can also be extended or, in principle, even fully transferred to the time domain, in which case, however, the forcing signals will also be concerned.) A number of other assumptions are also made. One is the additivity of responses of climatic means to different forcing factors. Existing studies (Meehl et al., 2003; Shiogama et al., 2013; Marvel et al., 2015) suggest that additivity is approximately fulfilled for the long-term GMST response, but the statistical power of the tests is not discussed; more importantly, additivity is found to break down on smaller spatial scales. Most of the assumptions are, however, related to the spatial and possibly also temporal covariance structure of internal variability.
Even after transforming severe criticism from McKitrick (2022) into a tractable form by Chen et al. (2024) (but see McKitrick, 2024, as well), some of these latter assumptions remain unconfirmed and possibly necessarily invalid, and the effect of a deviation from them remains unquantified. From a heuristic point of view, we highlight that the covariance matrix corresponding to the actual internal variability (arising under the influence of all forcing factors) needs to be estimated. This estimation is based on preindustrial control runs in most cases (Hegerl et al., 2019); sometimes data are pooled from different models and even from numerical experiments with different forcing as well as from different ensemble members (Gillett et al., 2021). Stationarity of internal variability is almost always assumed; an exception is Tett et al. (2002), where “intraensemble variances” are computed in forced numerical experiments, suitable for the representation of a nonstationary covariance structure (Tél et al., 2020, Section 10.1). We find even the latter approach unsatisfactory, since is unclear how the covariance structure corresponding to the combination of all forcing factors relates to those arising in single-forcing numerical experiments (e.g., some scaling factors could have to be introduced to form a linear combination even in a minimalist description). Although a so-called residual consistency test has been introduced as early as in Allen and Tett (1999) to check whether the covariance estimation is appropriate, its statistical power is unknown at present even with a sufficiently large sample size for the test to be valid (Chen et al., 2024). In fact, the test might not be sufficiently powerful under realistic circumstances, as Li et al. (2021) report that the traditionally computed confidence intervals associated with the final results can easily underestimate the true uncertainty due to an imperfect estimation of the covariance structure of internal variability. Attempts in Hannart et al. (2014); Hannart (2016); Katzfuss et al. (2017); Cummins et al. (2022) to circumvent potential problems either keep some original or introduce some new assumptions that may not be fully justified. While bootstrap methods may appear promising (DelSole et al., 2019), they may also fail for accessible sample sizes (Li et al., 2021).
In view of these circumstances, we prefer not to rely on optimal fingerprinting (whether from existing studies or in our own) but turn to the direct comparison approach utilized in Stott et al. (2000); Tett et al. (2002); Meehl et al. (2003); Hegerl et al. (2007); Jones et al. (2013); Bindoff et al. (2013); Gillett et al. (2021); Eyring et al. (2021) at the price of having to fix the amplitudes of the forcing and also of the response. In the context of our aim, this has an important implication for how to choose the TSI forcing history, as we will discuss soon. It also suggests us to perform a primary analysis assuming a perfect model response amplitude (but only in the variable under consideration, which is GMST in our case, without any assumption on spatial patterns; this analysis will be rather rigorous except for an assessment of TSI forcing histories of a generic shape) and to address model imperfection afterwards (necessarily with less rigor as well).
Among the cited analyses, Hegerl et al. (2007); Jones et al. (2013); Bindoff et al. (2013); Gillett et al. (2021); Eyring et al. (2021) actually utilize multiple models [taking each simulation from the CMIP collection (e.g., Eyring et al., 2016)]. While this should be an advantage and one approach to address model imperfection, the results are commonly processed in a suboptimal way: the aggregation of data from different models makes it impossible to decide whether the instrumental record is within the range of internal variability (represented by the ensemble spread) for any of the individual models, and precludes any probabilistic interpretation. Ideally, each model should be evaluated separately, as in Jones et al. (2013), from which it turns out that the statistics is relatively poor (there are few ensemble members only) for CMIP3 and CMIP5 simulations subjected to natural forcing factors only. Notwithstanding, it appears clear that the instrumental records are out of the range of such simulations. There are, however, two issues about this claim.
One is that the time evolution of the natural forcing factors is not known precisely. In particular, as mentioned, estimates of changes in TSI are surprisingly incoherent. While the simulations presented in Jones et al. (2013); Bindoff et al. (2013); Gillett et al. (2021); Eyring et al. (2021) were subjected, according to the relevant CMIP protocols, to some of the most plausible TSI histories according to available data (e.g., Matthes et al., 2017), these reconstructions feature rather small temporal variations beyond the 11-year solar cycle among all available reconstructions [as pointed out by Connolly et al. (2021) and subsequent work (Soon et al., 2023)]. However, excluding the possibility that the observed global warming could be attributed solely to an increasing TSI, which we shall refer to as the “solar hypothesis”, must take into account the strongest possible increase that is still consistent with measurements (and should not depend on short-scale, e.g., decadal, details of the time evolution of TSI). A few studies from more than 20 years ago (Tett et al., 2002; Meehl et al., 2003) are suitable from this point of view for investigating the question posed, attending to the fact that the TSI reconstructions available at that time (Hoyt and Schatten, 2024; Lean et al., 1995) are among the ones with the strongest increase (cf. Figures 1–3 in Connolly et al., 2021); notwithstanding, we believe that it is worth revisiting their conclusions in view of what we learned about models and reality since then, utilizing larger ensembles and, first and foremost, making sure that the statistics are reliable.
In particular, the other issue concerns whether the ensemble of the trajectories simulated according to a given single model under a given forcing history provides a faithful representation of the time evolution of the relevant (possibly conditional; see Section 3.2) probability distribution. While there is no CMIP protocol for selecting initial conditions for the different ensemble members, Jones et al. (2013) specifies that they were obtained in CMIP3 and CMIP5 by sampling the preindustrial control run, and this was done so for Tett et al. (2002); Meehl et al. (2003) as well. Even if the preindustrial control run were not drifting, it would still be unknown how much the ensemble members would be affected by a sampling bias (even where the selection is performed according to the ocean’s state). In the presence of a bias in the sampling of the relevant distribution at initialization, spurious effects, such as spurious trends (a kind of a drift), may appear in the evolution of the ensemble until it forgets its initial conditions and thus converges to the relevant probability distribution (Drótos et al., 2017). Moreover, the preindustrial control runs are drifting in all relevant CMIP phases (Gupta et al., 2012; Gupta et al., 2013; Irving et al., 2021). While the drift in the GMST has been shown by Jones et al. (2013) to be negligible during a few centuries in CMIP3 and CMIP5, the preindustrial control runs from which the different ensemble members were branched off are typically much longer, so that it may well happen that the initialization procedure does not sample a single well-defined probability distribution, and convergence to one will not take place within the duration of the attribution experiments (or perhaps ever). In fact, whether these problems have a significant effect on the results presented in Stott et al. (2000); Tett et al. (2002); Meehl et al. (2003); Hegerl et al. (2007); Jones et al. (2013); Bindoff et al. (2013); Gillett et al. (2021); Eyring et al. (2021) could be decided by dividing the single-model ensembles into two parts according to the initial GMST values and assessing their convergence to each other. However, this appears to be possible only in CMIP6 due to the very low number of ensemble members in earlier CMIP phases; in any case, such an investigation has not yet been carried out to the best of our knowledge.
In our study, which addresses the strongest observation-consistent TSI increase by new ensemble simulations, we avoid the drift-related initialization issue by generating our ensembles by small perturbations applied to a single model state (which is obtained after a long spin-up; any unforced contribution to the ensemble mean trend carried over from the spin-up simulation will be estimated to leave our conclusions unaffected). What remains after such an initialization is to wait for a practically complete convergence to the relevant probability distribution (thereby forgetting the extreme form of sampling bias associated with the concentrated initial conditions); we will dedicate an individual subsection in our paper to determine the corresponding convergence time.
Ensuring complete convergence is the essence of the so-called snapshot/pullback framework (Romeiras et al., 1990; Ghil et al., 2008; Chekroun et al., 2011; Drótos et al., 2015; Herein et al., 2016; Tél et al., 2020; Drótos and Bódai, 2022). Being a more careful variant of the large ensemble approach (see, e.g., Deser, 2020; Maher et al., 2021; Rodgers et al., 2021), it relies on running simulations with different initial conditions under given forcing histories in a given model. It allows drawing the full ranges of possible variable values permitted by internal variability under each forcing history, and it supports a probabilistic interpretation which we shall rely on in our analysis. The probabilistic interpretation is due to the ensemble members being distributed according to a unique but time-dependent probability distribution, that corresponding to the so-called [possibly conditional (Drótos and Bódai, 2022)] natural probability measure of the snapshot/pullback attractor of the dynamics [the “climate attractor”; we argued that the unique probability probability distribution supported by this attractor is the relevant one for climate (Drótos et al., 2015, 2017; Tél et al., 2020; Drótos and Bódai, 2022)]. Besides producing statistically reliable climate projections, this framework helps to understand statistical features of changing climates in the past, including, e.g., teleconnections (see, e.g., Herein et al., 2017; Haszpra et al., 2020; Bódai et al., 2021), and is unavoidable in properly interpreting certain turbulence-related experiments (Vincze et al., 2017). As explained in Drótos et al. (2015); Herein et al. (2016); Tél et al. (2020); Drótos and Bódai (2022), this framework enables one to construct true probability distributions for model variables, which is one step beyond the approach of Stott et al. (2000) and which we will take advantage of to investigate the compatibility of the observed GMST increase with modeled ones.
In particular, we will confirm that changes in TSI alone cannot explain the observed global warming according to a “reasonably credible” climate model. We note that we restrict our investigation to the integrated direct radiative effect of the Sun’s activity and disregard potentially relevant mechanisms that are more complicated [such as a higher variability in the UV spectral band captured in the stratosphere or an increased flux of charged particles; see Gray et al. (2010) for a review]. [Note that the consistency of results from standard CMIP5 models with observations regarding the 11-year solar cycle does not suggest such effects to have a high relevance (Amdur et al., 2021). Furthermore, no indications have been found so far for this either in CMIP6 climate models that have become sufficiently complex to be able to capture them, even under unrealistically strong variations in solar activity (Myhre et al., 2022). Cf., however, Scafetta (2023).] In principle, arguments for the same conclusion could be based on existing simulations in the linear forcing-feedback framework (Gregory et al., 2004; Gregory and Forster, 2008); we nonetheless believe that addressing the issue by dedicated simulations has some added value in comparison with such a simplified approach.
We carry out the numerical experiments, each corresponding to a 40-member ensemble and a different forcing history, using an intermediate-complexity climate model, the Planet Simulator (PlaSim; Fraedrich et al., 2005). PlaSim has been developed based on the most basic physical principles relevant to climate dynamics, and in previous studies (e.g., Lucarini et al., 2010; Herein et al., 2017; Kilic et al., 2018; Vincze et al., 2021; Mehling et al., 2023), it proved to be an appropriate numerical tool for investigating the climate system on global scales. Our main result is that even the maximum realistic increase in TSI alone, with all other forcing agents kept fixed, cannot result in a growing GMST comparable to observations [which we represent by HadCRUT5 data (Morice et al., 2021)]. However, when we feed PlaSim with the time series of the observed CO2 concentrations at a fixed TSI value, the instrumentally recorded GMST signal is found to be in surprisingly good harmony with the bundle of the simulated time series.
We emphasize that our primary objective is to determine whether we can exclude the attribution of the ongoing global warming to an increasing TSI alone (i.e., the “solar hypothesis”). This also means that we do not aim to attribute the time evolution of GMST to specific forcing mechanisms, so that we do not need to address the influence of all significant forcing agents. In particular, aerosols are not represented in PlaSim. Considering this, our CO2-driven forcing histories will only provide a “soft” indication of the role of this greenhouse gas.
2 Instrumental records
We make use of the HadCRUT5 data set (Morice et al., 2021), which extends coverage in data-sparse regions and presents improvements in observed regions in comparison with HadCRUT4. The data set combines near-surface air temperature measurements from weather stations over land and sea surface temperature measurements over the ocean from ships and buoys. These measurements are converted to a so-called analysis product of surface temperature anomalies, relative to the 1961–1990 period, of global coverage. We consider the annual global mean surface temperature analysis between 1920 and 2021 from version 5.0.2.0.
One needs to take into account that the HadCRUT5 data are subject to uncertainty, which is discussed by Morice et al. (2021) in detail. In order to take spatiotemporal correlations of uncertainty into account, uncertainty from all sources, with the exception of one, is represented by a 200-member ensemble of time series; the exception is uncertainty arising from a limited spatial coverage of the observations, for which a time series of standard deviations is provided in association with the global mean value of surface temperature. Beyond these representations, a “best estimate” time series, corresponding to the ensemble mean, and lower (2.5%) and upper (97.5%) confidence limit time series (taking into account all sources of uncertainty, including limited spatial coverage) are also readily available.
For our purposes, which will be explained later, we also construct ensembles representing the full uncertainty of the data, including coverage uncertainty. On the one hand, we do so by adding a random value to each ensemble member’s GMST in each year according to a Gaussian distribution with zero mean and a standard deviation corresponding to the coverage uncertainty associated with the given year. Since coverage uncertainty is not considered to be correlated with the rest of the uncertainty and Gaussianity is also assumed by Morice et al. (2021), the resulting ensemble provides a reasonable representation of the full uncertainty in each individual year. Furthermore, if we take the increment (signed difference) of GMST between two (sufficiently separated) years in each ensemble member, the distribution of these increments over the ensemble will still serve as a reasonable representation of the full uncertainty of the increment. Then the “best estimate” increment will be the ensemble mean increment (i.e., the ensemble mean of the increment values obtained for the individual ensemble members), and the lower and upper confidence limits can be obtained as the 2.5 and 97.5 percentiles in the ensemble, respectively (with a precision that the ensemble size makes accessible). It is, however, important that the two selected years must be separated by a sufficient time, e.g., by several decades, such that any correlation in coverage uncertainty decays between them.
On the other hand, if we are interested in the slope corresponding to a least-squares linear fit to the GMST time series, a rather conservative estimate on its full uncertainty can be represented as follows. To begin with, we select the first year of the time interval under consideration, and add a random value to each ensemble member’s GMST in the same way as described earlier. We then do the same for the last year of the given time interval. However, we register the two particular random values for each ensemble member, and proceed by adding a value to each intermediate year’s GMST within the given ensemble member such that it is obtained by linearly interpolating between the two registered values corresponding to the endpoints. This procedure assumes that the endpoints are sufficiently far away from each other to be uncorrelated in terms of coverage uncertainty, but within this, it tries to (although formally does not necessarily) maximize the impact of coverage uncertainty on the slope of a line fitted to the GMST time series of the given ensemble member. The representation of the rest of the uncertainty remains intact, so that the distribution of these slopes over the ensemble will, as anticipated, represent a rather conservative estimate on the full uncertainty of the slope in question. The “best estimate” and the confidence limits of the slope can then be derived similarly to those of the increment.
We must emphasize at this point that the ensembles representing uncertainty in the HadCRUT5 data set are conceptually very different from those representing internal variability in the PlaSim simulations. In the former case, a variable of interest has a true value, it is just our knowledge about it that is limited, but it could be improved in principle, thus reducing the width of the associated probability distribution, which is expressed by the ensemble spread. In contrast, internal variability is an inherent property of the dynamics, and any given realization is just as realistic as any other. The relevant probability distribution describing internal variability has a well-defined width; the ensemble spread must faithfully reflect this width and, consequently, cannot be reduced.
Since the HadCRUT5 data set concerns solely anomalies with respect to the 1961–1990 temporal mean, we utilize the CRU climatology (Jones et al., 1999), version v5, to determine the GMST averaged for this period, and we add this value to the HadCRUT5 GMST analysis where it becomes relevant. However, our main analyses are independent of such an offset. For this reason, we do not take the uncertainty of the CRU climatology into account.
Finally, we make a note about an effect called urbanization bias, which results from the evolution of urban heat islands [see Wang and Yan (2016) for a review]. When constructing the HadCRUT5 analysis as described by Morice et al. (2021), it has been taken into account attending to relevant literature (Brohan et al., 2006; Parker, 2010; Efthymiadis and Jones, 2010; Morice et al., 2012, and further references therein). At the same time, Connolly et al. (2021), as well as Soon et al. (2023), partially with reference to earlier literature, argue that the urbanization bias may have been much stronger than commonly estimated; for an apparently more robust assessment of any land-related bias with a similar conclusion, see also Scafetta (2021). We prefer not to address this issue, since its comprehensive examination surpasses the purview of this article.
3 Modeling strategy
3.1 PlaSim setup
For our purposes, we choose the same atmospheric setup for PlaSim as in Lucarini et al. (2010), i.e., the horizontal resolution of the simulations is T21, corresponding to a grid of approximately 5.6° × 5.6°. The dynamics of the atmosphere is described by the primitive equations. The model accounts for numerous unresolved processes through parameterizations. As a non-standard aspect of our model setup, the PlaSim atmosphere is coupled to a large-scale geostrophic (LSG) ocean, to a sea ice module, as well as to a dynamic global vegetation model, called SimBA, and the radiation module has been adapted to describe time dependence.
LSG ocean: We use the Hamburg Large Scale Geostrophic Ocean General Circulation Model (Cycle 1) (Maier-Reimer and Mikolajewicz, 1991). It is based on the fact that for ocean models designed for climate studies, the relevant spatial scales are large compared with the internal Rossby radius. The characteristic time scales are large compared with the periods of gravity and barotropic Rossby wave modes. This LSG model was originally proposed by Hasselmann (Hasselmann, 1982) and was described more fully in Maier-Reimer et al. (1993). It was used in a number of climate and paleoclimate studies (e.g., Maier-Reimer et al., 1990; Mikolajewicz et al., 1990; Maier-Reimer et al., 1993; Mikolajewicz et al., 1993; Drijfhout et al., 1996), more recently as the ocean component of PlaSim (e.g., Dallmeyer et al., 2015; Mehling et al., 2023).
Sea ice: The module is based on the zero layer model of Semtner (1976). It computes the thickness of the sea ice from the thermodynamic balance at the top and the bottom. The temperature gradient in the ice is assumed to be linear, and the capacity of the ice to store heat is neglected. For each marine cell of the grid, sea ice is allowed to form when the surface temperature drops below 271.25 K (−1.90 C°). When a cell is covered by sea ice, snowfall can accumulate on top. Snow is then converted into sea ice if its thickness is sufficient to push the ice/snow interface below the sea level. The typical sea ice thickness for a fully ice-covered sea is around 1 m.
SimBA (Simulator for Biospheric Aspects) is a simple terrestrial dynamic global vegetation model. It provides the following land surface variables for non-glaciated grid cells: surface albedo, roughness length, a surface conductance factor for latent heat, and a “bucket” depth for the soil. The SimBA variables ultimately depend on macroscopic variables: primarily on the soil moisture content, but also on snow depth and vegetative biomass (for details see Fraedrich et al., 2005).
Modified radiation module: Since PlaSim is a modular and flexible model, we developed it to handle continuously time-dependent forcings (beyond the annual time scale; strictly speaking, by “forcing,” we mean the time evolution of some parameter, even if it happens to be constant, after a, possibly hypothetical, initialization on a reference level). Concerning CO2 concentration, we incorporated historical (Lamarque et al., 2010) and observed (Keeling et al., 2001) data. The strength of TSI is changed to be an arbitrary function of time instead of being a constant.
Thanks to these adaptations and the relatively simple and well-understood but realistic physics, PlaSim becomes a reasonable choice for modeling the impact of a changing CO2 concentration or TSI on climate. Credibility of results obtained with our PlaSim configuration is supported by its equilibrium climate sensitivities with respect to CO2 and TSI forcing.
The equilibrium climate sensitivity in terms of the GMST increase that would correspond to a doubled CO2 concentration has been found to be around 4 K near the preindustrial level, which is in the upper half of the relevant CMIP ranges (Andrews et al., 2012; Zelinka et al., 2020). [Angeloni (2022) found very similar values with SimBA switched off and with some modified oceanic parameters; and her overall evaluation of PlaSim is rather favorable.] We must note that the CMIP values of Andrews et al. (2012); Zelinka et al. (2020) originate from a kind of extrapolation from non-equilibrated runs (as opposed to our running of PlaSim to equilibrium), which underestimates the true equilibrium sensitivity of a model corresponding to the given forcing level (Knutti et al., 2017; Rugenstein et al., 2020). At the same time, they were obtained by quadrupling the CO2 concentration and dividing the corresponding result by 2, which is prone to overestimate the response to an actual doubling (Meraner et al., 2013; Rugenstein et al., 2020), whereas our PlaSim estimation did not involve a similarly large alteration of the CO2 concentration. According to Table 1 of Rugenstein et al. (2020), the net effect of these two biases is typically an underestimation but limited to 13% among the simulations where this can be evaluated, which is clearly much smaller than, e.g., the inter-model spread and is not considerable from the point of view of our analyses.
The equilibrium climate sensitivity to TSI forcing is more important for the aim of our study than that to CO2 forcing. It has been determined as 0.12 KW−1m2, or 0.67 and 0.69 KW−1m2 in terms of a radiative forcing obtained by averaging over the sphere and assuming a planetary albedo of 0.3, in two experiments with TSI values increased by 10 Wm−2 and 100 Wm−2, respectively. Both increments in TSI fall beyond the relevant domain and indicate linearity in a wide range of TSI levels. These sensitivity values are just in the middle of the range, 0.3–1 KW−1m2, specified by Lean and Rind (1998) based on model studies. By combining data from Table 2 of Schmidt et al. (2012) and Table 1 of Andrews et al. (2012), the equilibrium climate sensitivity to TSI forcing, expressed in terms of an alteration of TSI (instead of a derived radiative forcing), for four more recent and comprehensive CMIP5 models can be estimated as 0.17 (IPSL-CM5A), 0.11 (MPI-ESM-LR), 0.10 (NorESM) and 0.17 (HadGEM2-ES) KW−1m2. What we obtained for our PlaSim configuration is consistent with these values. We reiterate that the data from Andrews et al. (2012) are somewhat biased; although the combination of CO2 and TSI forcing in Schmidt et al. (2012) might perhaps represent an even more complicated situation, the (long-term, although transient) GMST responses associated with increases in TSI and greenhouse gas concentration are suggested by Shiogama et al. (2013); Marvel et al. (2015) to be additive.
It is crucial to emphasize that CMIP5 models presumably do not fall far from reality in terms of capturing the impact of solar forcing. We base this claim on a recent study by Amdur et al. (2021) about the response to the 11-year solar cycle. The results presented in this study suggest that “typical” CMIP5 models could potentially underestimate the actual sensitivity of the Earth system to this form of forcing by a maximum factor of two. [Note that the amplitude of this solar cycle is rather consistent among the TSI reconstructions compiled by Connolly et al. (2021).] Therefore, regarding PlaSim’s equilibrium climate sensitivity to TSI forcing as “typical” in comparison with CMIP5 models, it appears to be appropriate to generate data by our PlaSim configuration as a starting point if we are intending to see if the high-end estimates of the warming attributable to TSI forcing can reach what has been observed instrumentally; more elaborate considerations will be presented in Section 5.2, after presenting our numerical results.
We have so far discussed the realism of our PlaSim configuration in terms of the globally averaged annual mean surface temperature only. To gain some basic insight on its performance regarding spatial inhomogeneity, we also evaluated spatial standard deviations over the globe. According to this quantifier, we have found that PlaSim somewhat overestimates the global-scale spatial inhomogeneity of the observed absolute temperature field (HadCRUT5 analysis added to the CRU climatology, having a 14.5 K spatial standard deviation; the PlaSim value is roughly 1 K higher); see Supplementary Figure S1. Overestimation occurs also with respect to the observed temperature anomaly field (HadCRUT5 analysis, 0.7 K) but to a lesser extent (by 0.1 K), as Supplementary Figure S2 illustrates. A reason for this overestimation may be related to a cold bias specific to high latitudes, which possibly results from limitations of the low-level cloud parameterization of PlaSim (Haberkorn, 2013). This reminds us that PlaSim, as mentioned before, is merely an intermediate-complexity model, and suggests a certain level of caution when drawing general conclusions from our simulation results.
Notwithstanding, these discrepancies in spatial standard deviations are not major. It is important to point out that the magnitude of internal variability (represented by the ensemble spread in PlaSim and estimated from decadal-scale fluctuations in the observed time series; cf. Section 5.2) appears to be modeled surprisingly well at the same time (about 0.5 K and between 0.2 and 0.3 K, possibly increasing with time, for the spatial standard deviation of the absolute temperature and that of the temperature anomaly, respectively). Furthermore, even if the absolute magnitudes of the PlaSim spatial standard deviations do not coincide with observations, trends in their time evolution [possibly indicative of polar amplification (Holland and Bitz, 2003; Serreze et al., 2009)] appear to follow those observable in the instrumental records, but only when PlaSim is subjected to an increasing CO2 concentration; with TSI forcing only, the recorded trends do not seem to be reproduced. The suspected increase in the internal variability of the spatial standard deviation of the observed temperature anomalies also appears to be discernible under the former but to be absent under the latter type of forcing histories. See once more Supplementary Figures S1, S2 in these regards.
3.2 Design of the numerical experiments
•Choosing the TSI history. Observation data sets for TSI appear to have uncertainties concerning possible temporal trends extending beyond the 11-year solar cycle (so-called secular trends). Although the most common view might be that any such trend has been rather minor over the last centuries (Gulev et al., 2021, see Chapter 2.2.1 and literature therein), a few publications suggest alternative possibilities. An extreme example is given in Willson and Mordvinov (2003) where the slope of an increasing trend is estimated to be 0.05 percent, approximately 0.7 Wm−2 per decade, for two and a half decades of satellite observations. Connolly et al. (2021) have compiled a collection of TSI reconstructions for the last two centuries in their Figures 1–3. Our intention is to assess the possible effect of the strongest TSI increase that is consistent with any of these reconstructions. Instead of considering each reconstruction separately, we concentrate on century-scale variations in TSI. Over 100 years, the strongest increase in TSI approximately amounts to 3 Wm−2 among the collected reconstructions; a similar upper bound on secular trends applies to other collections (Solanki et al., 2013; Chatzistergos et al., 2023; Connolly et al., 2023). According to this property of the reconstructions, we take a linear ramp in TSI with this very slope, 3 Wm−2(100yr)−1, between 1850 and 2021. This upper bound on the TSI trend is in fact very unlikely to be reached by actual trends according to recent analyses (e.g., Lockwood and Ball, 2020; Yeo et al., 2020; Kopp, 2021; Chatzistergos et al., 2023). We also highlight that each of the existing reconstructions suggests a slightly decreasing trend since 1996 (Chatzistergos et al., 2023), which we do not take into account in our study.
•Careful spinup of the ocean. It was shown in Maier-Reimer et al. (1993) that the simulated mean ocean circulation, for appropriately chosen surface forcing fields, adequately reproduces the principal water mass properties. We use the default resolution of 3.5° × 3.5° in 22 non-equidistant vertical layers along with realistic present-day bathymetry. The typical maximum basin depth is 5,500 m. The spinup of the LSG ocean starts from a resting ocean, and reaching a steady state lasts around 10,000 years. To complete the spinup of the ocean and the vegetation to the initial climate state of preindustrial conditions, we use a single run, which is approximately 2000 years long and leads to a steady state; we illustrate in Section 4.1 that any unforced contribution to the ensemble mean GMST trend carried over from the spin-up simulation is negligible for our analyses. By preindustrial conditions, assigned to 1850, we mean a CO2level of 286 ppm (Lamarque et al., 2010; Taylor et al., 2012).
•Initialization by the KICK routine. The KICK routine allows one to use white noise at the very beginning of the simulation as a slight perturbation in the surface pressure (10−4 Pa). It is used to generate different initial conditions for the ensemble members (Fraedrich et al., 2005). We apply the KICK routine to initialize the main ensembles, consisting of 40 members, at the end of the single steady-state run, corresponding to 1850 in their calendar, and also to initialize a 20-member auxiliary ensemble in 1920 to assess convergence (see the next point).
•Convergence to the relevant probability distribution in the atmosphere and the upper ocean. The convergence time in these system components in realistic climate models is considered to be on the order of a few decades [see Drótos and Bódai (2022) for a discussion of the issue with references]. In the particular case of PlaSim without a dynamical ocean or a vegetation component, the convergence time to the unique distribution of the climate attractor was estimated to be 40 years (Drótos et al., 2017). We emphasize that the full convergence time with a deep ocean and vegetation is expected to be much longer. However, we concentrate here on the atmosphere and the upper ocean; therefore, in the spirit of Drótos and Bódai (2022), we stick to small perturbations at initialization (see the previous point), and, as a new result, demonstrate in Section 4.2 that a convergence to a unique probability distribution (which is possibly conditional on the state of the slower system components) becomes sufficiently tight in the course of 40 years, such that the effect of the initial, extreme form of sampling bias becomes sufficiently small. Ensuring convergence in the atmosphere and the upper ocean thus means that we do not use data from the first 40 years after initialization by the KICK routine, i.e., between 1850 and 1890 in the main ensembles. In fact, we will restrict our analysis to the 1920–2021 interval, so that it will also become rather independent of the conditions preceding the initialization of the ensembles in 1850.
• Forcing histories:
•History C. Only CO2 increase: After the single steady-state run, which has relaxed to the preindustrial climate state of 1850, we follow the historical forcing (Lamarque et al., 2010; Taylor et al., 2012) up to 1957. We use the available measured data (Keeling et al., 2001) from 1958 up to 2021.
•Histories T. Only TSI increase. After the single steady-state run, we simulate the influence of the TSI increase in the following ways:
•History Ta: A constant CO2 forcing of 315.8 ppm is prescribed, which is relevant for 1958 (Keeling et al., 2001). At the same time, TSI increases along a linear ramp between years 1850 and 2021, starting from the value of 1,369 Wm−2 with a slope of 3 Wm−2 (100yr)−1.
•History Tb: Similar to Ta but the preindustrial CO2 level (286 ppm) is applied (Lamarque et al., 2010; Taylor et al., 2012).
•History B. Both: It is a combination of forcing histories C and T, both TSI (with the aforementioned linear ramp) and the CO2level are time dependent.
•History N. Neither: Neither the CO2 concentration nor TSI are time dependent. We take a constant CO2 level of 315.8 ppm (valid for the year 1958), and a constant TSI (1,369 Wm−2).
Note that the choice of a constant 315.8 ppm represents a jump in the CO2 forcing in 1850 in forcing histories Ta and N. Since we are intending to interpret these forcing histories as representing a constant CO2 level (a new reference level, defining the absence of CO2 forcing), any relaxation to the elevated value will be regarded as a drift; the total unforced contribution to ensemble mean trends under these forcing histories will be assessed in Section 4.3.
3.3 Initial TSI value
TSI has been directly measured since 1978, but different instruments, as mentioned above, produce non-negligible uncertainties in its value (Dudok de Wit et al., 2017; Kopp, 2021; Chatzistergos et al., 2023). Thus, making a statistically consistent TSI composite (Lockwood and Ball, 2020; Yeo et al., 2020; Marchenko et al., 2022; Chatzistergos et al., 2023) is challenging. The latest available composites, based on satellite data, have been reported, e.g., by Dudok de Wit et al. (2017); Kopp (2021), and Chatzistergos et al. (2023). According to these composites, the mean TSI value is around 1,361 Wm−2 (Kopp and Lean, 2011; Solanki et al., 2013; Dudok de Wit et al., 2017; Gulev et al., 2021; Kopp, 2021; Chatzistergos et al., 2023). Until the mid-2000s, the accepted mean TSI value was around 1,366 Wm−2 based on the data of pioneering satellite missions (Kopp, 2021).
We experimented with PlaSim using constant forcing (measured CO2 concentration for 1958) to determine the best TSI value to reproduce the observed temperature in 1958 (when temporal changes are still rather flat). We found that PlaSim works best with the value of 1,369 Wm−2. Though this value is 0.5% higher than the composite mean, it is equally within the range of the satellite measurements, see Figure 1 in Solanki et al. (2013), or Figures 7 and 8 in Kopp (2021). We repeat that PlaSim does not contain secondary greenhouse agents, such as aerosol, volcano soot, methane, etc., which is one reason why the offset of TSI base value was necessary. It is worth noting already at this point that the full performance of our tailored PlaSim version (see Section 3.1) with the measured CO2 data and this single constant TSI value is astonishingly good, see later. Therefore, we shall use this value as the constant value in forcing histories C and N and the initial value in forcing histories T and B.
4 Estimating bounds on unforced contributions to ensemble mean trends
For a quantitative analysis of model results obtained in an ensemble view, one needs to estimate a bound on any systematic deviation from the relevant probability distribution. Such a deviation may arise from an incomplete convergence (whether in the sense that the attractor has not been reached or that the sampling is biased) or a numerical inaccuracy; it is spurious by definition and may be perceived as a drift (Gupta et al., 2013) in a single time series in certain cases.
Since we are interested in the increase of GMST, we will concentrate on distributions of quantifiers of GMST trends. As a first approximation, we mostly assume that any systematic deviation from the relevant probability distribution can be described as a uniform shift (i.e., the shape of the distribution is not affected); we check the accuracy of this approximation where it appears to be necessary. A uniform shift represents a drift in a more general sense and can be characterized by the shift of the expected value. This shift will be reflected in the ensemble mean value of the given trend quantifier evaluated in the individual ensemble members of a numerical experiment (this is thus a spurious unforced contribution to the ensemble mean trend). Furthermore, if the quantifier in question is a linear function of the values of the corresponding GMST time series, its ensemble mean will be equal to the same quantifier evaluated for the time series of the ensemble mean GMST; we shall utilize this property for our estimation of a bound on the shift.
As a separate issue, the assessment of some response to a forcing, such as an increasing GMST, requires considering any unforced deviation from stationarity appearing in the relevant probability distribution itself. The existence of such a deviation may be possible if some slow modes of unforced variability are excluded from the relevant probability distribution (Drótos and Bódai, 2022). Unlike a drift as introduced above, a corresponding shift in the expected value of the relevant probability distribution of a given trend quantifier is not spurious, and the instrumentally recorded trend may also be subject to such an effect. The difficulty is that the realization of the slow modes in question are generically different in the numerical experiment and the real world. However, it is reasonable to believe that such a shift in the real-world trend cannot be much larger than those in models of sufficient realism.
In fact, drifts may be indistinguishable from manifestations of slow modes of unforced variability. Instead of considering them separately, we will estimate a bound on the total expected trend at the end of the (unforced) spin-up simulation. We believe that it approximates a bound on the corresponding unforced contribution to the mean trend in the forced numerical experiments. The generation of the ensembles at the beginning of the latter introduces the need for an additional step to estimate a bound on the total unforced contribution in them. The case of forcing history Ta needs an alternative consideration, which will be provided by forcing history N (meant to represent the absence of forcing).
4.1 The spin-up simulation
The spin-up simulation follows a single trajectory evolving in the absence of any forcing, as depicted in Figure 1 in terms of GMST. Therefore, trends of an ensemble mean cannot be evaluated. However, the unforced nature of the simulation implies that any trend (well) beyond the time scale of internal variability, which is on the order of a decade according to Figure 1, will represent a deviation of the expected trend of the simulation from zero.
Since the ensembles of the numerical experiments are branched off from the spin-up simulation at its end, any such deviation present at that time will carry over to those ensembles as an unforced contribution to the ensemble mean trend. We suppose that this contribution does not change considerably in the course of the numerical experiments. From the last 500 years of the spin-up simulation, we can safely estimate that the magnitude of any such contribution is well below 0.1 K(100yr)−1.
It is worth mentioning that the value of 0.1 K(100yr)−1 also serves as an approximate bound for the CMIP5 preindustrial control simulations (Gupta et al., 2013; Jones et al., 2013). As far as CMIP5 models are realistic, this confirms that there are no relevant slow modes missing from PlaSim.
4.2 Convergence of the ensembles to the relevant probability distribution
The small magnitude of any trend remaining in the spin-up simulation indicates that the trajectory must be very close to the preindustrial (stationary) attractor of the model. This attractor will continue as a (time-dependent) snapshot attractor after the forcing is switched on, and we are interested to create an ensemble of trajectories distributed according to the (time-dependent) distribution (the “relevant” one) corresponding to the [possibly conditional (Drótos and Bódai, 2022)] natural probability measure of this attractor. However, concentrating initial conditions near a single point on the preindustrial attractor represents an extreme form of sampling bias, which will lead to a drift (a spurious contribution to the ensemble mean trend) right after initialization (note that this is not observable in any single ensemble member).
This sampling bias will nonetheless fade out as the trajectories converge to the probability distribution in question. The process of convergence can be investigated by comparing two ensembles, initialized, e.g., at different times, with each other. The convergence becomes complete when they reach a unique probability distribution (which then turns out to be the relevant one, representing climate, see below). More precisely, any remaining drift will be revealed as a suitably quantified trend in the difference of the ensemble means, provided that the ensemble serving as a reference was initialized sufficiently much time earlier in terms of an approximate e-folding time of the convergence of this difference to zero.
For the purpose of determining the convergence time in our PlaSim configuration associated with reaching a sufficiently small drift, we consider a 20-member auxiliary ensemble initialized by applying the KICK routine to a given member of the main history C ensemble in 1920 and study how it converges, still under history C, to the main ensemble in GMST. We borrow this auxiliary ensemble from the analysis of Supplementary Section S2 of the Supplementary Material of Herein et al. (2023).
The two ensembles are visually compared in Figure 2. By 1960, 40 years after initializing the auxiliary ensemble (displayed in blue), no difference is discernible by the human eye. This is so even though the system possesses a deep ocean and a vegetation component. Our observation suggests that the distribution traced out by an ensemble becomes practically unique 40 years after initialization in the sense that it only depends on the model, on the forcing history, and possibly on the state of the slower system components but not on the details (such as the time) of initialization and thus represents climate (Drótos and Bódai, 2022).
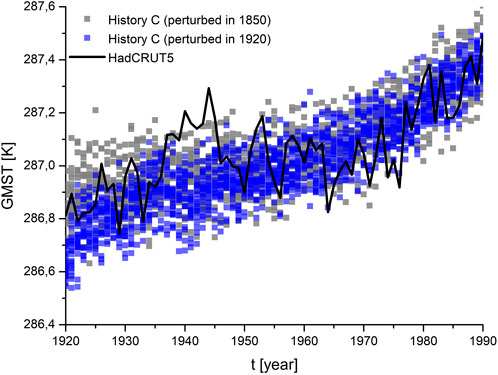
Figure 2. GMST as a function of time in the main history C ensemble (grey) and in the auxiliary ensemble which is subject to the same forcing history but is initialized in 1920 (blue). The simulation results are presented as follows. We draw a square centered on each simulated GMST value with a height of 0.025 K, a width of 1 year, and a hue given in the legend. Overlapping squares then increase the saturation of the color. The “best estimate” from the HadCRUT5 analysis (offset by the CRU climatology) is also included as a solid line.
Note that this is not so before convergence becomes complete. By comparing the “best estimate” time series for GMST from the HadCRUT5 analysis and the auxiliary ensemble in Figure 2, one could erroneously conclude that the model’s climate is rapidly warming in the first two decades after initialization such that it would not really be in a good agreement with the instrumental observations. However, what can be seen like this warming is, in fact, an initial transient of the auxiliary ensemble, a drift that originates from the sampling bias. At those times, the auxiliary ensemble does not represent the model’s climate, as the deviation from the main ensemble (displayed in grey) indicates.
One quantifier of this deviation is the difference between the ensemble means. We utilize this difference to estimate a bound on any GMST drift introduced by the sampling bias. Figure 3 shows a spurious warming in the first 30 years of the auxiliary ensemble, in harmony with what was visually recognized in the first 20 years in Figure 2. However, no definite trend can be seen from 1960 on. Taking into account where the fluctuations are centered and that this must reach zero asymptotically, one concludes that maximally 0.03 K of trend, with a positive sign, can remain in the difference of the ensemble means in the last three decades displayed in Figure 3, corresponding to a slope of 0.1 K(100yr)−1. Since the main history C ensemble was initialized 50 years before the auxiliary ensemble and an approximate e-folding time can be read off from Figure 3 to be a few decades, this slope represents an estimate of a bound on the unforced contribution to the ensemble mean trend arising from the sampling bias after 40 years of convergence. In an additional 30 years, by 1920 in the main numerical experiments, this value must further decrease by a factor of e or so.
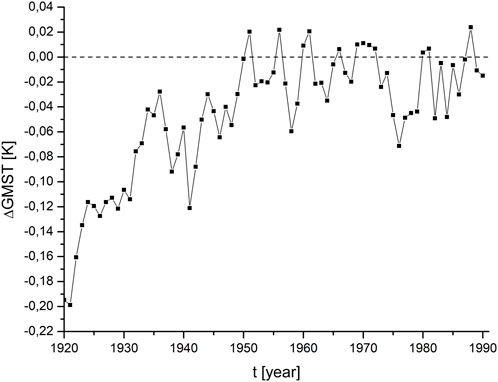
Figure 3. The difference of the ensemble mean GMST of the auxiliary ensemble (blue in Figure 2) from that of the main history C ensemble (grey in Figure 2) as a function of time.
Since the ensembles of our numerical experiments were initialized by small perturbations, we also need to take into account that the widths of the corresponding distributions are much smaller at the beginning than those of the relevant distributions. However, according to Figure 2, the ensemble spread approaches the relevant extension in GMST to a good accuracy (much better than 0.1 K) within 40 years; therefore, our assumption about the shape of the distribution appears to be accurate at a similar level.
4.3 The case of forcing histories Ta and N
The jump in the CO2 level at the beginning of forcing histories Ta and N introduces a relaxation to the new level, the effect of which we are intending to exclude from our analyses.
In fact, since we regard the history N simulations as unforced, the unforced contribution to the ensemble mean trend (including that induced by a sampling bias) will just be equal to its deviation from zero. As opposed to the spin-up simulation, this can be directly evaluated as we actually have an ensemble available in this case.
The ensemble mean GMST time series is displayed in Figure 4 between 1920 and 2021, the interval that will be the subject of our analysis. Any trend remaining in this interval is estimated to be smaller than 0.1 K(100yr)−1 but positive.
As a first approximation, we can assume that this estimate applies to the history Ta experiment as well, in the spirit of the recommendation of Drótos and Bódai (2022) for determining the effect of forcing. Since the unforced contribution has turned out to be positive, the native results corresponding to forcing history Ta will overestimate the effect of the prescribed increase in TSI.
Further related considerations will be presented at the analysis of the numerical results.
5 An increasing TSI and global warming in PlaSim and beyond
5.1 Numerical results from PlaSim
To address the question of whether an increasing TSI can play an exclusive role in global warming, we first visually compare the time evolution of GMST in the HadCRUT5 analysis and in the bundles of individual ensemble members in the different numerical experiments.
According to Figure 5A, the HadCRUT5 analysis fits well to the experiment with forcing history C consisting of the measured CO2 data up to 2021 without any trend in TSI (grey): the black line generally stays within the bounds traced out by the ensemble members and wanders across their range. The only exception is around 1940, which is a period still subject to debate from forcing agents’ and instrumental records’ point of view (Egorova et al., 2018; Folland et al., 2018); note that the 95% confidence band of the HadCRUT5 analysis still overlaps with the simulation bundle in this period. It is worth mentioning that the rapid increase in GMST from 1980 on appears in both data sets in complete harmony.
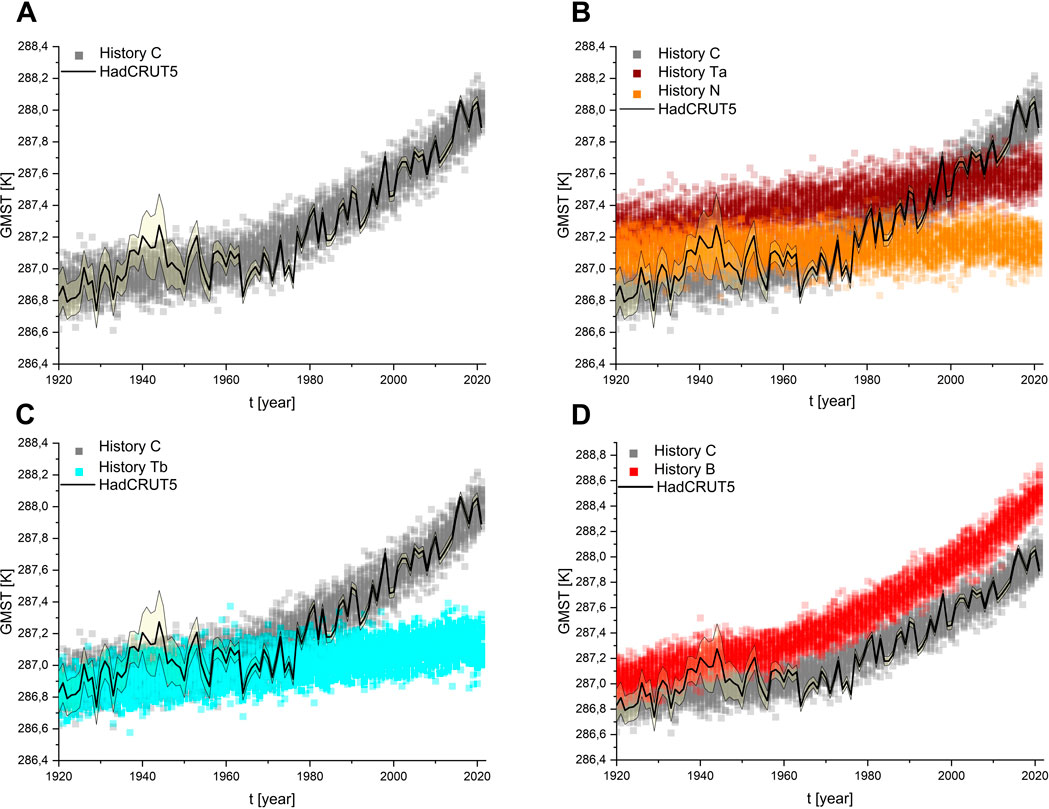
Figure 5. GMST as a function of time in the HadCRUT5 analysis (offset by the CRU climatology) and under different forcing histories. The HadCRUT5 “best estimate” is represented by a thick black line, while the lower (2.5%) and upper (97.5%) confidence limits are marked by thin black lines; the corresponding confidence interval is shaded. The way of presenting the simulation results is the same as in Figure 2. In panel (A), we display history C (grey), in (B) also Ta (claret coloring) and N (orange), (C) Tb (cyan), and (D) B (red). The time span is 1920–2021 in all panels.
In Figure 5B, we can see that the HadCRUT5 confidence band leaves the range traced out by the members of the experiment with forcing history Ta (trend in TSI with the constant 1958 CO2 level, claret coloring) around 2010, even at the relatively high CO2 level of this experiment, causing an opposite bias before 1980. The HadCRUT5 confidence band leaving the ensemble range upwards occurs even though the model obviously reacts to the TSI increase: the corresponding increase in GMST causes all ensemble members to leave the range traced out by the members of the experiment with forcing history N (no time dependence, orange) by 1990.
Not surprisingly, the HadCRUT5 confidence band leaves the ensemble range of the experiment with forcing history Tb (trend in TSI with a preindustrial constant CO2 level, cyan) much earlier than that of the experiment with forcing history Ta, around 1990 in particular, as can be observed in Figure 5C.
As for the experiment with forcing history B (trend in both CO2 and TSI, red; Figure 5D), the HadCRUT5 confidence band exits the range of the ensemble members downwards around 1960.
In total, the only experiment which the HadCRUT5 analysis appears to be compatible with by 2021 is the one with forcing history C. Nevertheless, since this result partially relies on the choice of when and how initial parameter values are prescribed, it appears to be a more careful approach to evaluate and compare trends in the GMST data. Since the linear TSI ramp of forcing histories T and B is a century-scale representation of the evolution featured by certain TSI reconstructions, we need to evaluate GMST trends nearly corresponding to the whole century. The subsequent analysis of such trends, of course, should be based on the statistics of the ensemble.
We first adopt the most common trend quantifier, the slope resulting from a least-squares linear fit. We determine this slope between 1920 and 2021 for each ensemble member of each experiment, make a histogram of these slopes for each experiment separately, and compare the confidence interval (and the “best estimate”) of the HadCRUT5 analysis slope (constructed as described in Section 2) with these histograms.
According to Figure 6, even the lower confidence limit of the HadCRUT5 slope is relatively far from the slopes arising in the numerical experiments with forcing histories T: in terms of probabilities (Drótos et al., 2015; Herein et al., 2016; Tél et al., 2020; Drótos and Bódai, 2022), one can practically exclude that the HadCRUT5 slope originates from one of the corresponding probability distributions. This is an (almost) formal confirmation of the incompatibility of the HadCRUT5 analysis with forcing histories T according to a high confidence. [Our nominal estimate of the confidence level is 97.5%, since the 2.5% confidence limit of the HadCRUT5 slope is out of the support of the histograms in question. This estimate, however, does not take into account that the sample sizes are finite. Notwithstanding, the probability density functions of the true probabilities underlying the histograms, similarly to the histograms themselves, do have a finite support. We believe that applying some generic methodology such as in Vermeesch (2005), with possibly invalid assumptions in our specific situation, to estimate confidence limits on our histogram counts would not be able to meaningfully capture the uncertainty in their tails and especially in the true bounds of their supports. Instead, as we also suggest through the plotting style, we guess that the first bins with zero counts might actually fail to capture some small but relevant probabilities, but the farther empty bins describe truly negligible probabilities. In principle, by increasing the ensemble size, the issue of confidence may be possible to be traced back exclusively to the uncertainty of the HadCRUT5 data.] At the same time, the HadCRUT5 slope is compatible with forcing history C according to the corresponding histogram, and is just on the boundary of compatibility with forcing history B.
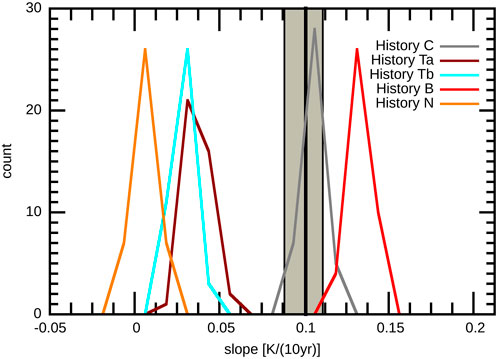
Figure 6. Histograms of the slopes of lines fitted to the GMST time series of the individual ensemble members between 1920 and 2021 in each numerical experiment (see legend). The bin size is 0.0125 K(10yr)−1. The “best estimate” of the HadCRUT5 analysis slope is marked by a thick black vertical line, while the lower (2.5%) and upper (97.5%) confidence limits are marked by thin black vertical lines; the corresponding confidence interval is shaded.
However, these results cannot be interpreted as a falsification of the “solar hypothesis” on their own: we have to emphasize that the incompatibility concerns the particular linear TSI ramp defining forcing histories T. To test whether the conclusion is more universal, we have to adapt to the century-scale nature of our linear representation of the relevant TSI reconstructions, and utilize some quantifier that is more independent, in comparison with a linear fit, of the details of how TSI and the corresponding GMST evolved between the endpoints of the time interval under consideration (i.e., 1920 and 2021).
For this purpose, we turn to an extremely simple quantifier: the GMST increment (signed difference) between the endpoints of a given interval; this may be regarded as a quantifier of the trend associated with that interval. We believe that this quantifier forms a reasonable (although not rigorous) basis for testing the hypothesis that the instrumentally recorded GMST increment between a “generic” year in the early 20th century and one in the early 21st century could be attributed to a TSI time series with a prescribed increment over the same period (as defined by our forcing histories) but having a rather unconstrained shape. Obviously, since each given year is rather “special” than “generic”, the robustness of such a test has to be checked by selecting slightly different interval endpoints; we will use the intervals 1920–2021, 1921–2020, 1925–2016, and 1930–2011. At the same time, the relatively strong manifestation of internal variability in the yearly GMST data is not a problem, since it is fully captured by the simulation ensembles.
We proceed similarly to the case of the slopes: we compute the GMST increment between the endpoints of the selected interval in each ensemble member of each experiment, make a histogram of the increments for each experiment separately, and finally determine the confidence interval (and the “best estimate”) of the HadCRUT5 increment for comparison (again as described in Section 2).
The organization of the data in the different panels of Figure 7, presenting results for the different time intervals specified above, is very similar to each other and also to that in Figure 6. This is an indication for the robustness of the results.
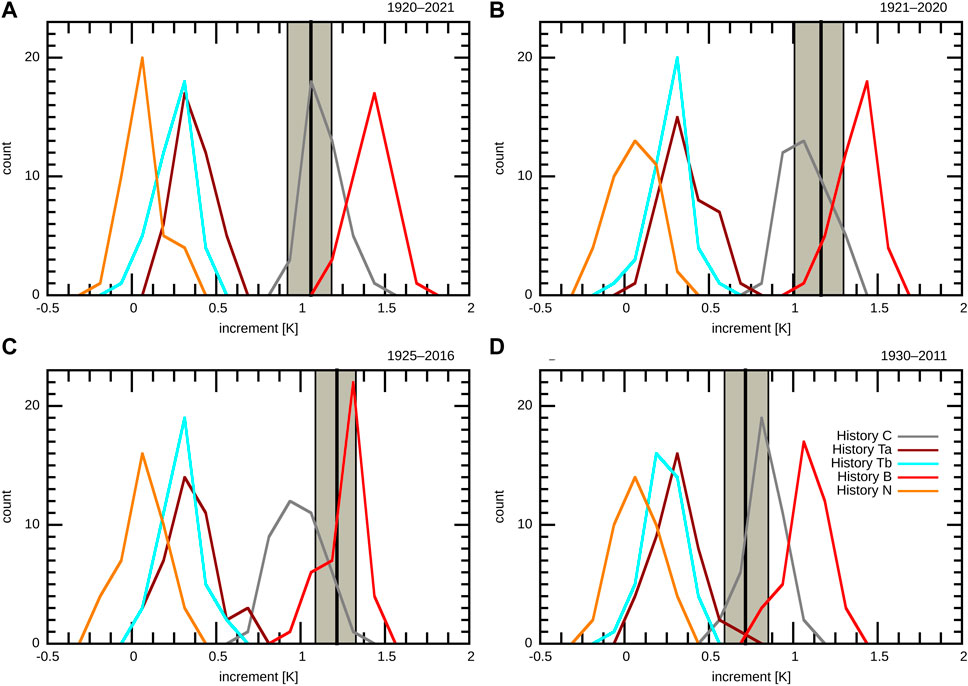
Figure 7. Histograms of the increments in GMST in the individual ensemble members between the endpoints of the indicated time intervals in each numerical experiment (see legend). The bin size is 0.125 K. The “best estimates” of the corresponding HadCRUT5 analysis increments are marked by thick black vertical lines, while the lower (2.5%) and upper (97.5%) confidence limits are marked by thin black vertical lines; the corresponding confidence intervals are shaded.
On a quantitative level, the histograms corresponding to forcing histories C and B are considerably shifting to the left from panel (A) to (D) in Figure 7, i.e., with a shrinking interval length; this is a consequence of the rapid increase in GMST in the last years of the corresponding simulations. Irrespective of this fact, the entire HadCRUT5 confidence interval always falls within the support of the history C histogram, so that the HadCRUT5 analysis appears compatible with a forcing history in which only the CO2 concentration is increasing. Qualitative differences between the panels can mainly be observed in relation to forcing history B: the HadCRUT5 confidence interval happens to lie in the bulk of the corresponding histogram in panel (C), while it is rather in the left tail of this histogram in panel (B), and it is located around the position where this tail reaches zero in panels (A) and (D), although with a considerable overlap with the support of the histogram. While two out of these four cases are not likely to occur, we cannot claim that the HadCRUT5 analysis would be incompatible with a forcing history incorporating both the observed increase in CO2 concentration and a representation of the maximum possible increase in TSI, especially that the different cases might not be fully statistically independent, and some of them might not be representative of a maximum TSI increase of a “generic” shape. The incompatibility observed in Figure 5D may be a consequence of the already existing absolute temperature bias in 1920, which results from the (presumably unrealistic) TSI increase prescribed between 1850 and 1920.
Unlike for forcing history B, Figure 7 clearly shows that the relevant probabilities are very low for forcing histories T: it is only in panel (D) that the HadCRUT5 confidence interval overlaps with the right tail of the histogram corresponding to forcing history Ta; otherwise, the probabilities that the HadCRUT5 increment could arise from forcing histories T are completely negligible according to the considerable distance of the lower HadCRUT5 confidence limit from the support of the corresponding histograms. Based on the above-presented considerations, we can thus rather safely conclude that the instrumental records are practically incompatible, in terms of the GMST increase, with a forcing history in which only TSI is changing and thus with the “solar hypothesis” in our PlaSim configuration.
This safety, and the confidence in the presented conclusions in general, relies on the analyses of Section 4. Note that both the slope of a linear fit and an increment (our trend quantifiers) are linear in the values of the corresponding time series, so that these analyses are applicable. According to the combination of these analyses, the total unforced contribution to the ensemble mean trend remains below 0.1 K(100yr)−1 or so, which means that the histograms of Figures 6, 7 are shifted at most by one bin’s width with respect to ideal histograms which are free from any undesirable effect; the same is true in relation to some slow mode of variability potentially affecting the HadCRUT5 analysis. Deviations from the ideal histogram widths are supposed to be even smaller. Effects of these magnitudes do not have an important impact on our analyses concerning the forcing histories T presented in the current subsection.
Moreover, according to Section 4.3, the histograms corresponding to forcing history Ta are shifted to the right; the ideal histograms would then be farther away from the HadCRUT5 confidence intervals, which would imply that we could, in fact, only overestimate the true chances that an increasing TSI could result in the observed warming in the absence of other forcing factors (unless the HadCRUT5 confidence intervals are shifted even more by some slow mode of variability, which appears to be unlikely).
This is confirmed by a more careful evaluation of the shift under consideration: the histograms corresponding to forcing history N, representing the unforced reference for forcing history Ta, appear roughly symmetric but are always centered to the right of zero. This shift to the right, of a magnitude of approximately 0.1 K (over 100 years) at most, might also explain the relative positions of the Ta and Tb histograms with respect to each other. If this could be verified, it would imply that this shift would mainly describe the relaxation to the elevated CO2 level, so that the total unforced contribution to the ensemble mean trends would remain much smaller than 0.1 K (over 100 years) for the histograms unaffected by such a relaxation.
5.2 Generalization attending to an imperfect model credibility
We now present some considerations regarding a generalization of our conclusions obtained for our particular PlaSim configuration. For this purpose, we return to the issue of PlaSim’s sensitivity to TSI forcing, and consider what the histograms of Figures 6, 7 could look like if our PlaSim configuration had a sensitivity at the very high end of the plausible range; then we check where the lower HadCRUT5 confidence limits could lie with respect to such histograms associated with the relevant forcing histories (Ta and Tb).
One difficulty is that a model’s response to TSI forcing may not be characterized by a single sensitivity value, but the shape of the dynamical (i.e., transient) response may vary from model to model, and linearity with respect to TSI forcing is not guaranteed either (even if the equilibrium response is linear). What we know from Section 3.1 is only that PlaSim has a “typical” equilibrium sensitivity (describing a nearly linear response to TSI forcing) among CMIP5 models, and that the maximum plausible sensitivity of the real Earth system to the 11-year solar cycle is roughly twice as high as that of “typical” CMIP5 models. However, we can add now our observation that the HadCRUT5 analysis and the history C bundle match surprisingly well in Figure 5A, following a shape that is common with numerical experiments forced by the historical increase of greenhouse-gas concentrations in Jones et al. (2013). This softly suggests that the characteristic response time scales of our PlaSim configuration and thus the shape of its dynamical responses in general are realistic and are in harmony with those of CMIP5 models. Taking this into account, it appears reasonable to adopt the maximum factor of two from Amdur et al. (2021) to represent PlaSim’s maximum underestimation of the amplitude of the real Earth system’s dynamical response to TSI forcing. Although a factor of two is a very high estimate in Amdur et al. (2021), we must keep in mind that it is not a formal upper bound, and our argumentation for its adoption is rather speculative.
According to this estimate, the centers of the Ta and Tb histograms could at most be twice as far from zero as in Figures 6, 7. At the same time, we also have to address the realism of the widths of these histograms.
In the absence of an ensemble of real-world realizations, one needs to rely on the time evolution of a given instrumentally recorded (possibly aggregated) variable (such as GMST) to assess the realism of the internal variability (underlying the histogram widths) arising in a given model subjected to a given forcing history (represented by the ensemble spread). Note that assessment is thus inaccessible for the internal variability associated with a single time instant (remember that internal variability itself is changing in time in response to a forcing), unless some assumptions are made about the real-world internal variability. What can be assessed without such assumptions is whether the full time evolution of the modeled internal variability may be realistic or credible in view of the recorded signal.
As formulated in Herein et al. (2023) with reference to earlier literature (e.g., Suarez-Gutierrez et al., 2021), “a necessary consistency condition for a given climate model to be credible is that the observed, measured time series lies within the range spanned by its large ensemble realizations over all times, [such] that it reaches the maxima or minima of the model simulations occasionally,” adding that “the consistency condition holds only for a converged ensemble.” We first note that this is in fact a necessary condition for the joint credibility of the model and the forcing history it is subjected to; the model itself may be credible even if the condition is not fulfilled due to an unrealistic forcing history. Second, as implied by this formulation, credibility of a model as a whole (obviously) includes a credible forced response in terms of the climatic (i.e., ensemble) mean. Internal variability in the model, including its own forced response, can be credible even if the forced response (or simply the absolute value) of the climatic mean is not. This requires the amplitude of the fluctuations in the recorded signal to match the range spanned by the ensemble members in the whole time interval under consideration if the forcing history is also realistic.
This naturally leads to the problem of separating fluctuations from forced changes of the climatic mean within the recorded time series; while this task is theoretically infeasible (without relying on a model already known to be credible), relevant fluctuations are conjectured to have a decadal time scale at most (e.g., Drótos and Bódai, 2022, and references therein). Therefore, identifying all changes occurring within a decade or so as fluctuations perhaps overestimates the magnitude of internal variability, but underestimation is supposed to be excluded. If we make a further assumption of a decadal smoothness in the (temporally changing) magnitude of the real-world internal variability, we can finally formulate a sufficient condition for excluding an underestimation of the magnitude of the real-world internal variability under the actually occurred forcing history at any time instant: the ensemble range in the ensemble of our interest must not be smaller at the given time instant than what we identify as fluctuations in the real-world signal around that time instant.
The condition of Herein et al. (2023) is fulfilled in Figure 5A to a surprisingly high accuracy; furthermore, the widths of the bundles of the ensemble members are similar under all of the forcing histories in Figure 5 and approximately remain constant. This means that our sufficient condition is fulfilled in practically the entire time span under any forcing history, including histories Ta and Tb. Taking into account that the corresponding assumptions about the real-world internal variability can be regarded as plausible, and also that the internal variability of even the spatial inhomogeneity in surface temperature appears to be captured rather accurately as well (perhaps including an increase in its magnitude under plausible forcing histories; see Section 3.1 and Supplementary Figures S1, S2), we speculate that we may indeed exclude that the magnitude of the simulated internal variability in GMST underestimates that of the real-world internal variability under the forcing history that actually occurred. (Note that we are not able to assess whether the simulated internal variability would match that of the real world under hypothetical forcing histories that did not occur in the real world; however, this is not a relevant question if we are to make comparisons with the instrumentally recorded signal.) Therefore, we suppose that the widths of the Ta and Tb histograms in Figures 6, 7 could not be substantially larger in association with a faithful representation of the real-world internal variability.
In total, our most pessimistic estimate about the real Earth system predicts Ta and Tb histograms centered twice as far from zero as in Figures 6, 7 with an essentially unchanged width. This transformation translates to a shift to the right by 0.03 K(10yr)−1 in Figure 6 and 0.3 K in Figure 7. After such a shift, the lower HadCRUT5 confidence limits would lie, with the single exception of Figure 7D, in the right tails of the Ta histograms, around the positions where the right tails of the Tb histograms reach zero. This makes compatibility with the corresponding forcing histories and, more broadly, the “solar hypothesis,” rather implausible.
Note that we have not quantified our estimate on the possible realistic widths, relative to the numerical model results, of the relevant histograms. The reason why our analysis is not as overly sensitive to the accuracy of the estimate of internal variability as optimal fingerprinting is its reliance on the amplitude of the forced response of climatic (i.e., ensemble) mean values (which can be rescaled without any constraint in optimal fingerprinting). Notwithstanding, it has been crucial to correctly represent PlaSim’s internal variability, allowing us to numerically evaluate the absolute widths of the PlaSim histograms in question and also to speculate about their credibility.
However, we reiterate that the assessment of credibility or realism and, consequently, the generalization of our negative PlaSim conclusion about a hypothetical exclusive role of a TSI increase is not really substantiated but remains speculative. We should remember at this point that PlaSim is merely an intermediate-complexity climate model and does not reproduce, e.g., the instrumentally observed spatial inhomogeneity of surface temperature accurately. This underscores the need for further investigations to validate these findings with more rigor.
6 Conclusion
• In ensemble simulations performed by our tailored PlaSim model, we have found with a high confidence that the instrumental record, represented by the HadCRUT5 analysis, is incompatible with our forcing histories in which only TSI is increasing.
• Even in case PlaSim happens to considerably underestimate the Earth system’s real sensitivity to TSI forcing, we speculate that the instrumentally recorded warming remains rather unlikely to arise under these forcing histories. However, this conclusion should ideally be validated by further studies.
Within PlaSim, the real-world increase in CO2 concentration appears to be compatible with the instrumentally recorded global warming both alone and in conjunction with an increase in TSI, although the relevant probability is smaller with the maximum observation-consistent increasing TSI trend. Note that further greenhouse gases and aerosols, along with their considerable and comparable respective warming and cooling effects (see, e.g., Myhre et al., 2013, Figure 8.15 for associated radiative forcing), are missing from PlaSim, so that such a compatibility may be consistent with existing literature. We emphasize that we cannot narrow down constraints on the possible time evolution of TSI based on our results.
Formally, our conclusions concern the very specific forcing histories considered in our numerical experiments. However, we presented an analysis that arguably confirms the validity of these conclusions in relation to forcing histories with rather unconstrained shapes between the early 20th and 21st centuries.
Notably, these conclusions are drawn such that any spurious trends in the simulation results possibly arising from a sampling bias at initialization have been sufficiently bounded by ensuring a sufficient level of convergence of the ensemble members to the relevant probability distribution. Moreover, any other drift or any deviation from stationarity associated with some slow mode of unforced variability has been estimated to leave our conclusions valid.
In principle, the climate of the system and how it responds to a forcing can depend on the state of some slow system components (Drótos and Bódai, 2022). However, such system components are also prone to model drifts, which are not reflected in GMST in a pronounced way (Irving et al., 2021), suggesting that GMST, including its time evolution, is insensitive to the state of such system components.
We could thus conclude that it would be implausible that the ongoing global warming could be attributed to an increasing TSI alone, even in the unlikely case if the strongest observation-consistent TSI trend proved to be correct for the last centuries. It appears to us that the “solar hypothesis” with reference to a TSI increase could hold only if the set of instrumental temperature measurements were subject to a more rapidly increasing bias than commonly estimated, e.g., due to more pronounced urbanization effects (Connolly et al., 2021; Scafetta, 2021; Soon et al., 2023). It is not our aim to discuss this issue in the present study, nor potentially relevant solar forcing mechanisms beyond the effect of TSI (Gray et al., 2010; Scafetta, 2023).
After establishing that human activity has been necessary for the ongoing global warming (which should take all possible natural factors into account), another relevant task will be to provide a lower estimate on the magnitude of the anthropogenic contribution such that the uncertainty in TSI forcing (and, preferably, in any solar forcing in general) is taken into account. Our results suggest this to be considerably large, but a quantitative analysis in a careful ensemble framework is outstanding to the best of our knowledge.
Author’s Note
We have learned after acceptance that the original Hoyt and Schatten (1993) TSI reconstruction has been made basically void by Chatzistergos (2024, Sol. Phys. 299, 21. https://doi.org/10.1007/s11207-024-02262-6) most recently. Its extensions by Scafetta et al. (2019, Remote Sens. 11, 2569. https://doi.org/10.3390/rs11212569) and Scafetta (2023) are also concerned. In fact, a basic assumption behind most of the reconstructions featuring a similar increase in the 20th century had already been falsified long before, and the single family avoiding this problem is implausible for several reasons; see Chatzistergos et al. (2023) and references therein. TSI composites from satellite measurements do not allow for comparable secular increments throughout their time span, even in view of the considerable uncertainty associated with them (Chatzistergos et al., 2023). While such composites might feed speculations about relatively strong long-term secular trends (e.g., Scafetta, 2023), Yeo et al. (2020) have determined a fundamental bound on TSI variation that is exceeded by all available reconstructions underlying our choice of the TSI slope. Notwithstanding, deviation from a crucial assumption for the derivation of this bound "cannot be excluded" according to the authors, even if unlikely. Our approach is to take a conservative viewpoint and address any speculations about “high variability estimates" (Connolly et al., 2021) of TSI evolution.
Data availability statement
The datasets presented in this study can be found in online repositories. The names of the repository/repositories and accession number(s) can be found below: https://doi.org/10.5281/zenodo.6967247, https://doi.org/10.5281/zenodo.6967253, https://doi.org/10.5281/zenodo.6966993, https://doi.org/10.5281/zenodo.6968486.
Author contributions
All authors took part in the design of the modeling strategy. MH implemented and carried out the numerical simulations in PlaSim. All authors jointly interpreted the results. GD devised and performed the statistical analyses of trends. GD and IJ led the writing of the manuscript. GD and MH created the figures. All authors contributed to the article and approved the submitted version.
Funding
This work was supported by the National Research, Development and Innovation Office (NKFIH) under Grants K125171 (all authors), FK135115 (MH) and 2019-2.1.7-ERA-NET-2020-00003 (GD). MH was supported by the János Bolyai Research Scholarship of the Hungarian Academy of Sciences. GD was supported by the EU under the QuantERA eDICT grant. IJ was supported by the Max Planck Institute for the Physics of Complex Systems, Dresden (Visitors Program).
Acknowledgments
Fruitful discussions with Tamás Tél and Miklós Vincze are gratefully acknowledged. Thanks are due to Frank Lunkeit and Zoltán Trócsányi for providing useful suggestions. GD is thankful to Hauke Schmidt for directing him to Schmidt et al. (2012) with regard to the climate sensitivity to TSI forcing inCMIP5 models.
In memoriam
This paper is dedicated to the memory of our Professor and co-author, Imre M. Jánosi (1963–2023). His invaluable guidance, always delivered with a friendly demeanor, often with a touch of humor, and his profound knowledge have greatly influenced our journey in science. His dedication to science was truly inspiring. Through this paper, we aim to honor his legacy and remarkable contributions to the field of climate science.
Conflict of interest
The authors declare that the research was conducted in the absence of any commercial or financial relationships that could be construed as a potential conflict of interest.
Publisher’s note
All claims expressed in this article are solely those of the authors and do not necessarily represent those of their affiliated organizations, or those of the publisher, the editors and the reviewers. Any product that may be evaluated in this article, or claim that may be made by its manufacturer, is not guaranteed or endorsed by the publisher.
Supplementary material
The Supplementary Material for this article can be found online at: https://www.frontiersin.org/articles/10.3389/feart.2024.1240784/full#supplementary-material
References
Alexander, W., and Bailey, F. (2007). Solar activity and climate change - a summary. Energy Environ. 18, 801–804. doi:10.1260/095830507782088749
Allen, M., and Stott, P. (2003). Estimating signal amplitudes in optimal fingerprinting, part I: theory. Clim. Dyn. 21, 477–491. doi:10.1007/s00382-003-0313-9
Allen, M., and Tett, S. (1999). Checking for model consistency in optimal fingerprinting. Clim. Dyn. 15, 419–434. doi:10.1007/s003820050291
Amdur, T., Stine, A. R., and Huybers, P. (2021). Global surface temperature response to 11-yr solar cycle forcing consistent with general circulation model results. J. Clim. 34, 2893–2903. doi:10.1175/JCLI-D-20-0312.1
Andrews, T., Gregory, J. M., Webb, M. J., and Taylor, K. E. (2012). Forcing, feedbacks and climate sensitivity in CMIP5 coupled atmosphere-ocean climate models. Geophys. Res. Lett. 39, L09712. doi:10.1029/2012GL051607
Angeloni, M. (2022). “Climate variability in an Earth system Model of Intermediate Complexity: from interannual to centennial timescales”. Ph.D. thesis (Bologna, Italy: Alma Mater Studiorum Università di Bologna. Dottorato di ricerca in Geofisica), 34. Ciclo. doi:10.48676/unibo/amsdottorato/10152
Bindoff, N., Stott, P., AchutaRao, K., Allen, M., Gillett, N., Gutzler, D., et al. (2013). “Detection and attribution of climate change: from global to regional,” in Climate change 2013: the physical science basis. Contribution of working group I to the fifth assessment report of the Intergovernmental Panel on Climate Change. Editors T. F. Stocker, D. Qin, G.-K. Plattner, M. Tignor, S. K. Allen, J. Boschunget al. (Cambridge, United Kingdom and New York, NY, USA: Cambridge University Press), 867–952.
Bódai, T., Drótos, G., Ha, K-. J., Lee, J-. Y., and Chung, E-. S. (2021). Nonlinear forced change and nonergodicity: the case of ENSO-Indian Monsoon and global precipitation teleconnections. Front. Earth Sci. 8, 1–24. doi:10.3389/feart.2020.599785
Brohan, P., Kennedy, J. J., Harris, I., Tett, S. F. B., and Jones, P. D. (2006). Uncertainty estimates in regional and global observed temperature changes: a new data set from 1850. J. Geophys. Res. Atmos. 111, D12106. doi:10.1029/2005JD006548
Chatzistergos, T., Krivova, N. A., and Yeo, K. L. (2023). Long-term changes in solar activity and irradiance. J. Atmos. Sol-Terr. Phys. 252, 106150. doi:10.1016/j.jastp.2023.106150
Chekroun, M. D., Simonnet, E., and Ghil, M. (2011). Stochastic climate dynamics: random attractors and time-dependent invariant measures. Phys. D. 240, 1685–1700. doi:10.1016/j.physd.2011.06.005
Chen, H., Chen, S. X., and Mu, M. (2024). A statistical review on the optimal fingerprinting approach in climate change studies. Clim. Dyn. 62, 1439–1446. doi:10.1007/s00382-023-06975-5
Connolly, R., Soon, W., Connolly, M., Baliunas, S., Berglund, J., Butler, C. J., et al. (2021). How much has the sun influenced Northern Hemisphere temperature trends? An ongoing debate. Res. Astron. Astrophys. 21, 131. doi:10.1088/1674-4527/21/6/131
Connolly, R., Soon, W., Connolly, M., Baliunas, S., Berglund, J., Butler, C. J., et al. (2023). Challenges in the detection and attribution of Northern Hemisphere surface temperature trends since 1850. Res. Astronomy Astrophysics 23, 105015. doi:10.1088/1674-4527/acf18e
Cummins, D., Stephenson, D., and Stott, P. (2022). Could detection and attribution of climate change trends be spurious regression? Clim. Dyn. 59, 2785–2799. doi:10.1007/s00382-022-06242-z
Dallmeyer, A., Claussen, M., Fischer, N., Haberkorn, K., Wagner, S., Pfeiffer, M., et al. (2015). The evolution of sub-monsoon systems in the Afro-Asian monsoon region during the Holocene– comparison of different transient climate model simulations. Clim. Past 11, 305–326. doi:10.5194/cp-11-305-2015
DelSole, T., Trenary, L., Yan, X., and Tippett, M. K. (2019). Confidence intervals in optimal fingerprinting. Clim. Dyn. 52, 4111–4126. doi:10.1007/s00382-018-4356-3
Deser, C. (2020). Certain uncertainty: the role of internal climate variability in projections of regional climate change and risk management. Earths Future 8, e2020EF001854. doi:10.1029/2020ef001854
Drijfhout, S. S., Maier-Reimer, E., and Mikolajewicz, U. (1996). Tracing the conveyor belt in the Hamburg large-scale geostrophic ocean general circulation model. J. Geophys. Res. 101 (C10), 22563–22575. doi:10.1029/96JC02162
Drótos, G., and Bódai, T. (2022). On defining climate by means of an ensemble. ESS Open Archive, 1–35. doi:10.1002/essoar.10510833.3
Drótos, G., Bódai, T., and Tél, T. (2015). Probabilistic concepts in a changing climate: a snapshot attractor picture. J. Clim. 28, 3275–3288. doi:10.1175/JCLI-D-14-00459.1
Drótos, G., Bódai, T., and Tél, T. (2017). On the importance of the convergence to climate attractors. Eur. Phys. J. Spec. Top. 226, 2031–2038. doi:10.1140/epjst/e2017-70045-7
Dudok de Wit, T., Kopp, G., Fröhlich, C., and Schöll, M. (2017). Methodology to create a new total solar irradiance record: making a composite out of multiple data records. Geophys. Res. Lett. 44, 1196–1203. doi:10.1002/2016GL071866
Efthymiadis, D. A., and Jones, P. D. (2010). Assessment of maximum possible urbanization influences on land temperature data by comparison of land and marine data around coasts. Atmosphere 1, 51–61. doi:10.3390/atmos1010051
Egorova, T., Rozanov, E., Arsenovic, P., Peter, T., and Schmutz, W. (2018). Contributions of natural and anthropogenic forcing agents to the early 20th century warming. Front. Earth Sci. 6, 206. doi:10.3389/feart.2018.00206
Eyring, V., Bony, S., Meehl, G. A., Senior, C. A., Stevens, B., Stouffer, R. J., et al. (2016). Overview of the Coupled Model Intercomparison Project Phase 6 (CMIP6) experimental design and organization. Geosci. Model. Dev. 9, 1937–1958. doi:10.5194/gmd-9-1937-2016
Eyring, V., Gillett, N., Rao, K. A., Barimalala, R., Parrillo, M. B., Bellouin, N., et al. (2021). “Human influence on the climate system,” in Climate change 2021: the physical science basis. Contribution of working group I to the sixth assessment report of the Intergovernmental Panel on Climate Change. Editors V. Masson-Delmotte, P. Zhai, A. Pirani, S. Connors, C. Péan, S. Bergeret al. (Cambridge, United Kingdom and New York, NY, USA: Cambridge University Press), 423–551. doi:10.1017/9781009157896.005
Folland, C. K., Boucher, O., Colman, A., and Parker, D. E. (2018). Causes of irregularities in trends of global mean surface temperature since the late 19th century. Sci. Adv. 4, eaao5297. doi:10.1126/sciadv.aao5297
Fraedrich, K., Jansen, H., Kirk, E., Luksch, U., and Lunkeit, F. (2005). The planet simulator: towards a user friendly model. Meteorol. Z. 14, 299–304. doi:10.1127/0941-2948/2005/0043
Ghil, M., Chekroun, M. D., and Simonnet, E. (2008). Climate dynamics and fluid mechanics: natural variability and related uncertainties. Phys. D. 237, 2111–2126. doi:10.1016/j.physd.2008.03.036
Gillett, N. P., Kirchmeier-Young, M., Ribes, A., Shiogama, H., Hegerl, G. C., Knutti, R., et al. (2021). Constraining human contributions to observed warming since the pre-industrial period. Nat. Clim. Change 11, 207–212. doi:10.1038/s41558-020-00965-9
Gray, L. J., Beer, J., Geller, M., Haigh, J. D., Lockwood, M., Matthes, K., et al. (2010). Solar influences on climate. Rev. Geophys. 48, RG4001. doi:10.1029/2009RG000282
Gregory, J. M., and Forster, P. M. (2008). Transient climate response estimated from radiative forcing and observed temperature change. J. Geophys. Res. Atmos. 113, D23105. doi:10.1029/2008JD010405
Gregory, J. M., Ingram, W. J., Palmer, M. A., Jones, G. S., Stott, P. A., Thorpe, R. B., et al. (2004). A new method for diagnosing radiative forcing and climate sensitivity. Geophys. Res. Lett. 31, L03205. doi:10.1029/2003GL018747
Gueymard, C. A. (2018). A reevaluation of the solar constant based on a 42-year total solar irradiance time series and a reconciliation of spaceborne observations. Sol. Energy 168, 2–9. doi:10.1016/j.solener.2018.04.001
Gulev, S., Thorne, P., Ahn, J., Dentener, F., Domingues, C., Gerland, S., et al. (2021). “Changing state of the climate system,” in Climate change 2021: the physical science basis. Contribution of working group I to the sixth assessment report of the Intergovernmental Panel on Climate Change. Editors V. Masson-Delmotte, P. Zhai, A. Pirani, S. Connors, C. Péan, S. Bergeret al. (Cambridge, United Kingdom and New York, NY, USA: Cambridge University Press), 287–422. doi:10.1017/9781009157896.004
Gupta, A. S., Jourdain, N. C., Brown, J. N., and Monselesan, D. (2013). Climate drift in the CMIP5 models. J. Clim. 26, 8597–8615. doi:10.1175/JCLI-D-12-00521.1
Gupta, A. S., Muir, L. C., Brown, J. N., Phipps, S. J., Durack, P. J., Monselesan, D., et al. (2012). Climate drift in the CMIP3 models. J. Clim. 25, 4621–4640. doi:10.1175/JCLI-D-11-00312.1
Haberkorn, K. (2013). “Reconstruction of the Holocene climate using an atmosphere-ocean-biosphere model and proxy data”. Ph.D. thesis (Hamburg, Germany: University of Hamburg).
Hannart, A. (2016). Integrated optimal fingerprinting: method description and illustration. J. Clim. 29, 1977–1998. doi:10.1175/JCLI-D-14-00124.1
Hannart, A., Ribes, A., and Naveau, P. (2014). Optimal fingerprinting under multiple sources of uncertainty. Geophys. Res. Lett. 41, 1261–1268. doi:10.1002/2013GL058653
Hasselmann, K. (1982). An ocean model for climate variability studies. Prog. Oceanogr. 11, 69–92. doi:10.1016/0079-6611(82)90004-0
Haszpra, T., Herein, M., and Bódai, T. (2020). Investigating ENSO and its teleconnections under climate change in an ensemble view – a new perspective. Earth Syst. Dyn. 11, 267–280. doi:10.5194/esd-11-267-2020
Hegerl, G., and Zwiers, F. (2011). Use of models in detection and attribution of climate change. WIREs Clim. Change 2, 570–591. doi:10.1002/wcc.121
Hegerl, G., Zwiers, F. W., Braconnot, P., Gillett, N., Luo, Y., Orsini, J. M., et al. (2007). “Understanding and attributing climate change,” in Climate change 2007: the physical science basis. Contribution of working group I to the fourth assessment report of the Intergovernmental Panel on Climate Change. Editors S. Solomon, D. Qin, M. Manning, Z. Chen, M. Marquis, K. Averytet al. (Cambridge, United Kingdom and New York, NY, USA: Cambridge University Press), 663–745.
Hegerl, G. C., Brönnimann, S., Cowan, T., Friedman, A. R., Hawkins, E., Iles, C., et al. (2019). Causes of climate change over the historical record. Environ. Res. Lett. 14, 123006. doi:10.1088/1748-9326/ab4557
Herein, M., Drótos, G., Haszpra, T., Márfy, J., and Tél, T. (2017). The theory of parallel climate realizations as a new framework for teleconnection analysis. Sci. Rep. 7, 44529. doi:10.1038/srep44529
Herein, M., Márfy, J., Drótos, G., and Tél, T. (2016). Probabilistic concepts in intermediate-complexity climate models: a snapshot attractor picture. J. Clim. 29, 259–272. doi:10.1175/JCLI-D-15-0353.1
Herein, M., Tél, T., and Haszpra, T. (2023). Where are the coexisting parallel climates? Large ensemble climate projections from the point of view of chaos theory. Chaos Interdiscip. J. Nonlinear Sci. 33, 031104. doi:10.1063/5.0136719
Holland, M. M., and Bitz, C. M. (2003). Polar amplification of climate change in coupled models. Clim. Dyn. 21, 221–232. doi:10.1007/s00382-003-0332-6
Hoyt, D. V., and Schatten, K. H. (2024). TSI reconstruction has been made basically void by Chatzistergos. Sol. Phys 299, 21. doi:10.1007/s11207-024-02262-6
Huntingford, C., Stott, P. A., Allen, M. R., and Lambert, F. H. (2006). Incorporating model uncertainty into attribution of observed temperature change. Geophys. Res. Lett. 33, L05710. doi:10.1029/2005GL024831
Irving, D., Hobbs, W., Church, J., and Zika, J. (2021). A mass and energy conservation analysis of drift in the CMIP6 ensemble. J. Clim. 34, 1–43. doi:10.1175/JCLI-D-20-0281.1
Jones, G. S., Stott, P. A., and Christidis, N. (2013). Attribution of observed historical near–surface temperature variations to anthropogenic and natural causes using CMIP5 simulations. J. Geophys. Res. Atmos. 118, 4001–4024. doi:10.1002/jgrd.50239
Jones, P. D., New, M., Parker, D. E., Martin, S., and Rigor, I. G. (1999). Surface air temperature and its changes over the past 150 years. Rev. Geophys. 37, 173–199. doi:10.1029/1999RG900002
Katzfuss, M., Hammerling, D., and Smith, R. L. (2017). A Bayesian hierarchical model for climate change detection and attribution. Geophys. Res. Lett. 44, 5720–5728. doi:10.1002/2017GL073688
Keeling, C. D., Piper, S. C., Bacastow, R. B., Wahlen, M., Whorf, T. P., Heimann, M., et al. (2001). Exchanges of atmospheric CO2 and 13CO2 with the terrestrial biosphere and oceans from 1978 to 2000. I. Global aspects. SIO Reference Series, No. 01-06. San Diego: Scripps Institution of Oceanography, 1–28.
Kilic, C., Lunkeit, F., Raible, C. C., and Stocker, T. F. (2018). Stable equatorial ice belts at high obliquity in a coupled atmosphere-ocean model. Astrophys. J. 864, 106. doi:10.3847/1538-4357/aad5eb
Knutti, R., Rugenstein, M., and Hegerl, G. (2017). Beyond equilibrium climate sensitivity. Nat. Geosci. 10, 727–736. doi:10.1038/ngeo3017
Kopp, G. (2014). An assessment of the solar irradiance record for climate studies. J. Space Weather Space Clim. 4, A14. doi:10.1051/swsc/2014012
Kopp, G. (2021). Science highlights and final updates from 17 years of Total Solar Irradiance measurements from the Solar Radiation and Climate Experiment/Total Irradiance Monitor (SORCE/TIM). Sol. Phys. 296, 133. doi:10.1007/s11207-021-01853-x
Kopp, G., and Lean, J. L. (2011). A new, lower value of total solar irradiance: evidence and climate significance. Geophys. Res. Lett. 38, L01706. doi:10.1029/2010GL045777
Lamarque, J.-F., Bond, T. C., Eyring, V., Granier, C., Heil, A., Klimont, Z., et al. (2010). Historical (1850-2000) gridded anthropogenic and biomass burning emissions of reactive gases and aerosols: methodology and application. Atmos. Chem. Phys. 10, 7017–7039. doi:10.5194/acp-10-7017-2010
Lean, J., Beer, J., and Bradley, R. (1995). Reconstruction of solar irradiance since 1610: implications for climate change. Geophys. Res. Lett. 22, 3195–3198. doi:10.1029/95GL03093
Lean, J., and Rind, D. (1998). Climate forcing by changing solar radiation. J. Clim. 11, 3069–3094. doi:10.1175/1520-0442(1998)011<3069:cfbcsr>2.0.co;2
Li, Y., Chen, K., Yan, J., and Zhang, X. (2021). Uncertainty in optimal fingerprinting is underestimated. Environ. Res. Lett. 16, 084043. doi:10.1088/1748-9326/ac14ee
Lockwood, M., and Ball, W. T. (2020). Placing limits on long-term variations in quiet-Sun irradiance and their contribution to total solar irradiance and solar radiative forcing of climate. Proc. Roy. Soc. A Math. Phys. Eng. Sci. 476, 20200077. doi:10.1098/rspa.2020.0077
Lucarini, V., Fraedrich, K., and Lunkeit, F. (2010). Thermodynamic analysis of snowball Earth hysteresis experiment: efficiency, entropy production and irreversibility. Q. J. R. Meteorol. Soc. 136, 2–11. doi:10.1002/qj.543
Maher, N., Milinski, S., and Ludwig, R. C. (2021). Large ensemble climate model simulations: introduction, overview, and future prospects for utilising multiple types of large ensemble. Earth Syst. Dyn. 12, 401–418. doi:10.5194/esd-12-401-2021
Maier-Reimer, E., and Mikolajewicz, U. (1991). The Hamburg large scale geostrophic ocean general circulation model (Cycle 1). Tech. Rep./ Dtsch. Klimarechenzentrum 2, 1–34.
Maier-Reimer, E., Mikolajewicz, U., and Crowley, T. J. (1990). Ocean general circulation model sensitivity experiment with an open central American isthmus. Paleoceanography 5, 349–366. doi:10.1029/PA005i003p00349
Maier-Reimer, E., Mikolajewicz, U., and Hasselmann, K. (1993). Mean circulation of the Hamburg LSG OGCM and its sensitivity to the thermohaline surface forcing. J. Phys. Oceanogr. 23, 731–757. doi:10.1175/1520-0485(1993)023⟨0731:MCOTHL⟩2.0.CO;2
Marchenko, S. V., Lean, J. L., and DeLand, M. T. (2022). Relationship between total solar irradiance and magnetic flux during solar minima. Astrophys. J. 936, 158. doi:10.3847/1538-4357/ac8a98
Marvel, K., Schmidt, G. A., Shindell, D., Bonfils, C., LeGrande, A. N., Nazarenko, L., et al. (2015). Do responses to different anthropogenic forcings add linearly in climate models? Environ. Res. Lett. 10, 104010. doi:10.1088/1748-9326/10/10/104010
Matthes, K., Funke, B., Andersson, M. E., Barnard, L., Beer, J., Charbonneau, P., et al. (2017). Solar forcing for CMIP6 (v3.2). Geosci. Model. Dev. 10, 2247–2302. doi:10.5194/gmd-10-2247-2017
McKitrick, R. (2022). Checking for model consistency in optimal fingerprinting: a comment. Clim. Dyn. 58, 405–411. doi:10.1007/s00382-021-05913-7
McKitrick, R. (2024). Total least squares bias in climate fingerprinting regressions with heterogeneous noise variances and correlated explanatory variables. Environmetrics, e2835. doi:10.1002/env.2835
Meehl, G. A., Washington, W. M., Wigley, T. M. L., Arblaster, J. M., and Dai, A. (2003). Solar and greenhouse gas forcing and climate response in the twentieth century. J. Clim. 16, 426–444. doi:10.1175/1520-0442(2003)016⟨0426:SAGGFA⟩2.0.CO;2
Mehling, O., Bellomo, K., Angeloni, M., Pasquero, C., and von Hardenberg, J. (2023). High-latitude precipitation as a driver of multicentennial variability of the AMOC in a climate model of intermediate complexity. Clim. Dyn. 61, 1519–1534. doi:10.1007/s00382-022-06640-3
Meraner, K., Mauritsen, T., and Voigt, A. (2013). Robust increase in equilibrium climate sensitivity under global warming. Geophys. Res. Lett. 40, 5944–5948. doi:10.1002/2013GL058118
Mikolajewicz, U., Maier-Reimer, E., Crowley, T. J., and Kim, K.-Y. (1993). Effect of Drake and Panamanian Gateways on the circulation of an ocean model. Paleoceanography 8, 409–426. doi:10.1029/93PA00893
Mikolajewicz, U., Santer, B. D., and Maier-Reimer, E. (1990). Ocean response to greenhouse warming. Nature 345, 589–593. doi:10.1038/345589a0
Montillet, J.-P., Finsterle, W., Kermarrec, G., Sikonja, R., Haberreiter, M., Schmutz, W., et al. (2022). Data fusion of total solar irradiance composite time series using 41 years of satellite measurements. J. Geophys. Res. Atmos. 127, e2021JD036146. doi:10.1029/2021JD036146
Morice, C. P., Kennedy, J. J., Rayner, N. A., and Jones, P. D. (2012). Quantifying uncertainties in global and regional temperature change using an ensemble of observational estimates: the HadCRUT4 data set. J. Geophys. Res. Atmos. 117, D08101. doi:10.1029/2011JD017187
Morice, C. P., Kennedy, J. J., Rayner, N. A., Winn, J. P., Hogan, E., Killick, R. E., et al. (2021). An updated assessment of near-surface temperature change from 1850: the HadCRUT5 data set. J. Geoph. Res. Atmos. 126, e2019JD032361. doi:10.1029/2019JD032361
Myhre, G., Shindell, D., Bréon, F.-M., Collins, W., Fuglestvedt, J., Huang, J., et al. (2013). “Anthropogenic and natural radiative forcing,” in Climate change 2013: the physical science basis. Contribution of working group I to the fifth assessment report of the Intergovernmental Panel on Climate Change. Editors T. F. Stocker, D. Qin, G.-K. Plattner, M. Tignor, S. K. Allen, J. Boschunget al. (Cambridge, United Kingdom and New York, NY, USA: Cambridge University Press), 659–740.
Myhre, G., Shindell, D., Bréon, F.-M., Collins, W., Sandstad, M., Mohr, C. W., et al. (2022). Scientific data from precipitation driver response model intercomparison project. Sci. Data 9, 123. doi:10.1038/s41597-022-01194-9
Parker, D. E. (2010). Urban heat island effects on estimates of observed climate change. WIREs Clim. Change 1, 123–133. doi:10.1002/wcc.21
Qian, G., Li, Q., Li, C., Li, H., Wang, X. L., Dong, W., et al. (2021). A novel statistical decomposition of the historical change in global mean surface temperature. Environ. Res. Lett. 16, 054057. doi:10.1088/1748-9326/abea34
Ribes, A., Planton, S., and Terray, L. (2013). Application of regularised optimal fingerprinting to attribution. Part I: method, properties and idealised analysis. Clim. Dyn. 41, 2817–2836. doi:10.1007/s00382-013-1735-7
Richardson, M. T., and Benestad, R. E. (2022). Erroneous use of statistics behind claims of a major solar role in recent warming. Res. Astronomy Astrophysics 22, 125008. doi:10.1088/1674-4527/ac981c
Rodgers, K. B., Lee, S.-S., Rosenbloom, N., Timmermann, A., Danabasoglu, G., Deser, C., et al. (2021). Ubiquity of human-induced changes in climate variability. Earth Syst. Dyn. 12, 1393–1411. doi:10.5194/esd-12-1393-2021
Romeiras, F. J., Grebogi, C., and Ott, E. (1990). Multifractal properties of snapshot attractors of random maps. Phys. Rev. A 41, 784–799. doi:10.1103/PhysRevA.41.784
Roy, I. (2018). “Total solar irradiance (TSI): measurements and reconstructions,” in Climate variability and sunspot activity: analysis of the solar influence on climate (Cham: Springer International Publishing), 63–67.
Rugenstein, M., Bloch-Johnson, J., Gregory, J., Andrews, T., Mauritsen, T., Li, C., et al. (2020). Equilibrium climate sensitivity estimated by equilibrating climate models. Geophys. Res. Lett. 47, e2019GL083898. doi:10.1029/2019GL083898
Scafetta, N. (2012). Multi-scale harmonic model for solar and climate cyclical variation throughout the Holocene based on Jupiter-Saturn tidal frequencies plus the 11-year solar dynamo cycle. J. Atmos. Sol.-Terr. Phys. 80, 296–311. doi:10.1016/j.jastp.2012.02.016
Scafetta, N. (2021). Detection of non-climatic biases in land surface temperature records by comparing climatic data and their model simulations. Clim. Dyn. 56, 2959–2982. doi:10.1007/s00382-021-05626-x
Scafetta, N. (2023). Empirical assessment of the role of the Sun in climate change using balanced multi-proxy solar records. Geosci. Front. 14, 101650. doi:10.1016/j.gsf.2023.101650
Schmidt, H., Alterskjær, K., Bou Karam, D., Boucher, O., Jones, A., Kristjánsson, J. E., et al. (2012). Solar irradiance reduction to counteract radiative forcing from a quadrupling of CO2: climate responses simulated by four earth system models. Earth Syst. Dyn. 3, 63–78. doi:10.5194/esd-3-63-2012
Scripps Institute (2023). All global stations CO2 concentration trends. Available at: https://scrippsco2.ucsd.edu/graphics_gallery/other_stations/all_global_stations_co2_trends.html (Accessed June 20, 2023).
Semtner, A. J. J. (1976). A model for the thermodynamic growth of sea ice in numerical investigations of climate. J. Phys. Oceanogr. 6, 379–389. doi:10.1175/1520-0485(1976)006⟨0379:AMFTTG⟩2.0.CO;2
Serreze, M. C., Barrett, A. P., Stroeve, J. C., Kindig, D. N., and Holland, M. M. (2009). The emergence of surface-based Arctic amplification. Cryosphere 3, 11–19. doi:10.5194/tc-3-11-2009
Shiogama, H., Stone, D. A., Nagashima, T., Nozawa, T., and Emori, S. (2013). On the linear additivity of climate forcing-response relationships at global and continental scales. Int. J. Climatol. 33, 2542–2550. doi:10.1002/joc.3607
Solanki, S. K., Krivova, N. A., and Haigh, J. D. (2013). Solar irradiance variability and climate. Annu. Rev. Astron. Astrophys. 51, 311–351. doi:10.1146/annurev-astro-082812-141007
Soon, W., Baliunas, S. L., Robinson, A. B., and Robinson, Z. W. (1999). Environmental effects of increased atmospheric carbon dioxide. Energy Environ. 10, 439–468. doi:10.1260/0958305991499694
Soon, W., Connolly, R., Connolly, M., Akasofu, S.-I., Baliunas, S., Berglund, J., et al. (2023). The detection and attribution of Northern Hemisphere land surface warming (1850–2018) in terms of human and natural factors: challenges of inadequate data. Climate 11, 179. doi:10.3390/cli11090179
Soon, W., and Legates, D. R. (2013). Solar irradiance modulation of Equator-to-Pole (Arctic) temperature gradients: empirical evidence for climate variation on multi-decadal timescales. J. Atmos. Sol.-Terr. Phys. 93, 45–56. doi:10.1016/j.jastp.2012.11.015
Stott, P. A., Jones, G. S., and Mitchell, J. F. B. (2003). Do models underestimate the solar contribution to recent climate change? J. Clim. 16, 4079–4093. doi:10.1175/1520-0442(2003)016⟨4079:DMUTSC⟩2.0.CO;2
Stott, P. A., Tett, S. F. B., Jones, G. S., Allen, M. R., Mitchell, J. F. B., and Jenkins, G. J. (2000). External control of 20th century temperature by natural and anthropogenic forcings. Science 290, 2133–2137. doi:10.1126/science.290.5499.2133
Suarez-Gutierrez, L., Milinski, S., and Maher, N. (2021). Exploiting large ensembles for a better yet simpler climate model evaluation. Clim. Dyn. 57, 2557–2580. doi:10.1007/s00382-021-05821-w
Taylor, K. A., Stouffer, R. J., and Meehl, G. A. (2012). An overview of CMIP5 and the experiment design. Bull. Am. Meteorol. Soc. 93, 485–498. doi:10.1175/BAMS-D-11-00094.1
Tél, T., Bódai, T., Drótos, G., Haszpra, T., Herein, M., Kaszás, B., et al. (2020). The theory of parallel climate realizations. J. Stat. Phys. 179, 1496–1530. doi:10.1007/s10955-019-02445-7
Tett, S. F. B., Jones, G. S., Stott, P. A., Hill, D. C., Mitchell, J. F. B., Allen, M. R., et al. (2002). Estimation of natural and anthropogenic contributions to twentieth century temperature change. J. Geophys. Res. Atmos. 107, ACL 10–1–ACL 10–24. doi:10.1029/2000JD000028
Tett, S. F. B., Stott, P. A., Allen, M. R., Ingram, W. J., and Mitchell, J. F. B. (1999). Causes of twentieth-century temperature change near the Earth’s surface. Nature 399, 569–572. doi:10.1038/21164
Vermeesch, P. (2005). Statistical uncertainty associated with histograms in the Earth sciences. J. Geophys. Res. Solid Earth 110, B02211. doi:10.1029/2004JB003479
Vincze, M., Borcia, I. D., and Harlander, U. (2017). Temperature fluctuations in a changing climate: an ensemble based experimental approach. Sci. Rep. 7, 254. doi:10.1038/s41598-017-00319-0
Vincze, M., Bozóki, T., Herein, M., Borcia, I. D., Harlander, U., Horicsányi, A., et al. (2021). The Drake Passage opening from an experimental fluid dynamics point of view. Sci. Rep. 11, 19951. doi:10.1038/s41598-021-99123-0
Wang, J., and Yan, Z.-W. (2016). Urbanization-related warming in local temperature records: a review. Atmos. Ocean. Sci. Lett. 9, 129–138. doi:10.1080/16742834.2016.1141658
Willson, R. C., and Mordvinov, A. V. (2003). Secular total solar irradiance trend during solar cycles 21-23. Geophys. Res. Lett. 30, 1199–1203. doi:10.1029/2002GL016038
Yeo, K. L., Solanki, S. K., Krivova, N. A., Rempel, M., Anusha, L. S., Shapiro, A. I., et al. (2020). The dimmest state of the Sun. Geophys. Res. Lett. 47, e2020GL090243. doi:10.1029/2020GL090243
Keywords: climate change, total solar irradiance, ensemble simulations, snapshot attractors, internal variability
Citation: Drótos G, Herein M, Haszpra T and Jánosi IM (2024) Converged ensemble simulations of climate: possible trends in total solar irradiance cannot explain global warming alone. Front. Earth Sci. 12:1240784. doi: 10.3389/feart.2024.1240784
Received: 15 June 2023; Accepted: 07 March 2024;
Published: 06 August 2024.
Edited by:
Ismail Gultepe, Ontario Tech University, CanadaReviewed by:
Eduardo Zorita, Helmholtz Centre for Materials and Coastal Research (HZG), GermanyAna G. Elias, Universidad Nacional de Tucumán, Argentina
Copyright © 2024 Drótos, Herein, Haszpra and Jánosi. This is an open-access article distributed under the terms of the Creative Commons Attribution License (CC BY). The use, distribution or reproduction in other forums is permitted, provided the original author(s) and the copyright owner(s) are credited and that the original publication in this journal is cited, in accordance with accepted academic practice. No use, distribution or reproduction is permitted which does not comply with these terms.
*Correspondence: Gábor Drótos, ZHJvdG9zQGdlbmVyYWwuZWx0ZS5odQ==
†These authors have contributed equally to this work
‡Deceased