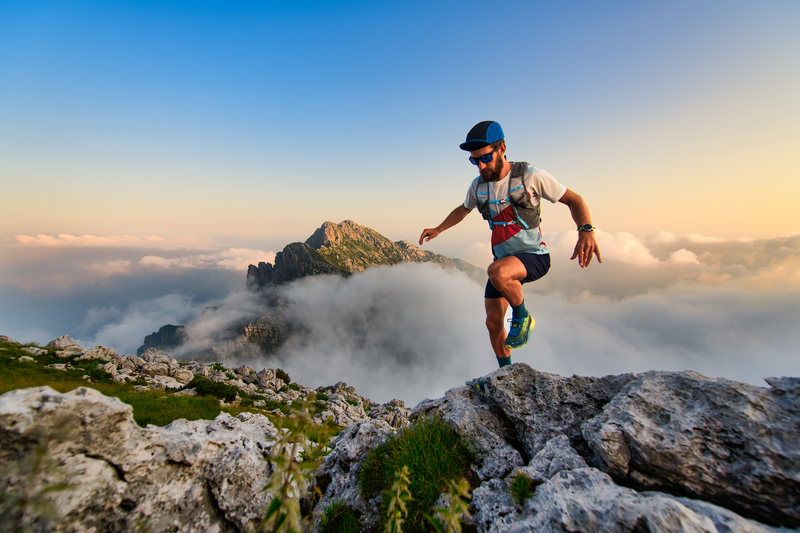
94% of researchers rate our articles as excellent or good
Learn more about the work of our research integrity team to safeguard the quality of each article we publish.
Find out more
ORIGINAL RESEARCH article
Front. Cell. Infect. Microbiol.
Sec. Bacteria and Host
Volume 15 - 2025 | doi: 10.3389/fcimb.2025.1531732
This article is part of the Research Topic Recent Advancements in the Research Models of Infectious Diseases View all 6 articles
The final, formatted version of the article will be published soon.
You have multiple emails registered with Frontiers:
Please enter your email address:
If you already have an account, please login
You don't have a Frontiers account ? You can register here
Purpose: The aim of this study was to develop a valuable clinical prediction model for pneumoniaassociated bloodstream infections (PABSIs). Patients and methods: The study retrospectively collected clinical data of pneumonia patients at the First Medical Centre of the Chinese People's Liberation Army General Hospital from 2019 to 2024. Patients who met the definition of pneumonia-associated bloodstream infections (PABSIs) were selected as the main research subjects. A prediction model for the probability of bloodstream infections (BSIs) in pneumonia patients was constructed using a combination of LASSO regression and logistic regression. The performance of the model was verified using several indicators, including receiver operating characteristic (ROC) curve, calibration curve, decision curve analysis (DCA) and cross validation. Results: A total of 423 patients with confirmed pneumonia were included in the study, in accordance with the Inclusion Criteria and Exclusion Criteria. Of the patients included in the study, 73 developed a related bloodstream infection (BSI). A prediction model was constructed based on six predictors: long-term antibiotic use, invasive mechanical ventilation, glucocorticoids, urinary catheterization, vasoactive drugs, and central venous catheter placement. The areas under the curve (AUC) of the training set and validation set were 0.83 and 0.80, respectively, and the calibration curve demonstrated satisfactory agreement between the two.
Keywords: Pneumonia, bloodstream infections, Bacteremia, risk factor, early diagnosis, Prediction model
Received: 20 Nov 2024; Accepted: 03 Mar 2025.
Copyright: © 2025 Zhou, Liu, Qu, Wei, Song and Guan. This is an open-access article distributed under the terms of the Creative Commons Attribution License (CC BY). The use, distribution or reproduction in other forums is permitted, provided the original author(s) or licensor are credited and that the original publication in this journal is cited, in accordance with accepted academic practice. No use, distribution or reproduction is permitted which does not comply with these terms.
* Correspondence:
Xizhou Guan, Department of Pulmonary and Critical Care Medicine, Eighth Medical Center of the General Hospital of the Chinese People's Liberation Army, Beijing, Beijing Municipality, China
Disclaimer: All claims expressed in this article are solely those of the authors and do not necessarily represent those of their affiliated organizations, or those of the publisher, the editors and the reviewers. Any product that may be evaluated in this article or claim that may be made by its manufacturer is not guaranteed or endorsed by the publisher.
Research integrity at Frontiers
Learn more about the work of our research integrity team to safeguard the quality of each article we publish.