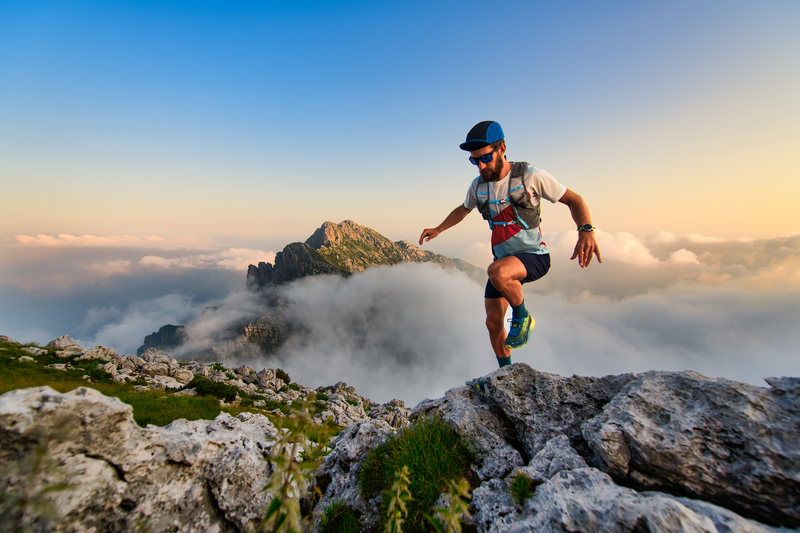
94% of researchers rate our articles as excellent or good
Learn more about the work of our research integrity team to safeguard the quality of each article we publish.
Find out more
ORIGINAL RESEARCH article
Front. Cell. Infect. Microbiol.
Sec. Oral Microbes and Host
Volume 15 - 2025 | doi: 10.3389/fcimb.2025.1506382
The final, formatted version of the article will be published soon.
You have multiple emails registered with Frontiers:
Please enter your email address:
If you already have an account, please login
You don't have a Frontiers account ? You can register here
The circulating microbiome is an emerging concept in biomedical research, offering the potential for predictive biomarkers and pathogen screening. Evidence suggests that the translocation of predominant oral microbes shapes the composition of the blood microbiome in the circulatory system. Herein, we applied this concept to characterize the blood microbiome signature of the healthy controls and patients with myocardial infarction (MI) compared to the oral microbiome signature. We enrolled 20 participants, including 10 healthy controls and 10 patients with MI. Blood and Saliva samples were collected from each participant to analyse the association between blood and oral microbiome in MI patients using 16S rRNA gene sequencing. Our findings revealed that, compared to the oral microbiome, the blood microbiome exhibited significantly higher alpha diversity (p<0.05), while no statistical differences were observed in beta diversity metrics. The blood microbiome of MI patients was predominantly composed of the phyla Firmicutes, Bacteroidota, Actinobacteriota, genus Bacteroides, and depleted levels of Proteobacteria. While Firmicutes, Bacteroidota, Veillonella, and Prevotella_7 dominated the oral microbiome profile. LEfSe analysis found two distinct bacterial taxa in the blood, such as Actinobacteria in MI and Enterobacterales in the control group. At the same time, the oral microbiota was distinctly abundant to Rothia, Micrococcaceae, and Micrococcales in the healthy group, with no distinct taxa found in MI patients. Furthermore, the KEGG Annotated pathway showed significant alterations in the blood and oral microbiome functional prediction pathways between both groups. Moreover, the blood and oral microbiome signatures revealed a substantial correlation with demographic and clinical markers. Our study confirms that the blood contains a distinct microbiome with specific taxa and functional pathways rather than simply reflecting oral translocation. These findings highlight the potential role of the circulating microbiome in MI pathology and its link to clinical markers.
Keywords: Myocardial Infarction, Blood, Oral, microbiome, biomarkers
Received: 05 Oct 2024; Accepted: 17 Mar 2025.
Copyright: © 2025 Lei, Li, Zhou, Zhu, Zhang, Xie, Zhang, Khan and Chen. This is an open-access article distributed under the terms of the Creative Commons Attribution License (CC BY). The use, distribution or reproduction in other forums is permitted, provided the original author(s) or licensor are credited and that the original publication in this journal is cited, in accordance with accepted academic practice. No use, distribution or reproduction is permitted which does not comply with these terms.
* Correspondence:
Ikram Khan, Department of Genetics, School of Basic Medical Sciences, Lanzhou University, Lanzhou, China
Disclaimer: All claims expressed in this article are solely those of the authors and do not necessarily represent those of their affiliated organizations, or those of the publisher, the editors and the reviewers. Any product that may be evaluated in this article or claim that may be made by its manufacturer is not guaranteed or endorsed by the publisher.
Research integrity at Frontiers
Learn more about the work of our research integrity team to safeguard the quality of each article we publish.