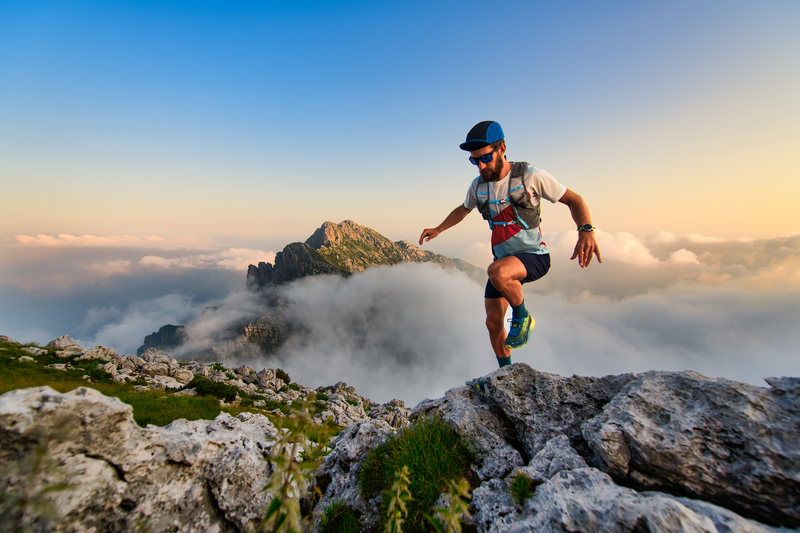
95% of researchers rate our articles as excellent or good
Learn more about the work of our research integrity team to safeguard the quality of each article we publish.
Find out more
HYPOTHESIS AND THEORY article
Front. Sustain. Food Syst. , 26 March 2025
Sec. Agricultural and Food Economics
Volume 9 - 2025 | https://doi.org/10.3389/fsufs.2025.1515188
This article is part of the Research Topic Harnessing Digital Innovation for Sustainable Agricultural Development View all 13 articles
The primary application of blockchain technology in the food supply chain is the development of a food traceability system that enhances transparency and accountability. The adoption of food blockchain traceability systems depends on consumer engagement and support at retail endpoints. This study integrates the Technology Acceptance Model (TAM) and Technology Readiness (TR) to explore consumer adoption behavior. A survey was conducted with 322 supermarket consumers in Shenzhen, and data were analyzed using Structural Equation Modeling (SEM) and hierarchical multiple regression analysis. The findings suggest that the extended TAM framework effectively predicts consumer adoption attitudes and intentions towards blockchain traceability systems. Perceived ease of use and perceived usefulness are significant factors influencing adoption attitude, which in turn strongly predicts adoption intention. Furthermore, positive dimensions of TR, such as optimism and innovativeness, significantly moderate the TAM model, whereas negative dimensions, such as discomfort and insecurity, show no significant moderating effects. These insights provide valuable recommendations for food and retail enterprises to facilitate blockchain adoption and expand its application within the food industry.
Blockchain has gained widespread application in various industries, including agriculture, logistics, and food safety, offering significant improvements in transparency, security, and traceability. This transformation aims to enhance supply chain performance by improving information linkage, promoting efficient collaboration among partners, and enabling intelligent decision-making. As a result, Traceability and transparency are critical components of modern digital supply chains (Centobelli et al., 2022). Blockchain technology provides substantial benefits, including enhanced transparency, trust, smart contract automation, secure encryption, and disintermediation, which collectively address the challenges of traceability and transparency in supply chains.
Currently, global food security faces significant challenges, exacerbated by factors such as climate change, soil degradation, contamination, and the overuse of fertilizers and pesticides (Mc Carthy et al., 2018). Food safety incidents are frequent worldwide, and the widespread presence of counterfeit, substandard food products, coupled with misleading food labeling, has led to growing public concern over food quality and safety (Nagaraj, 2021). In China, high-profile media debates about “Hex technology” and “intense techniques” last year, along with the recent controversy surrounding pre-prepared meals in schools, have further heightened public anxiety regarding food safety. These highlight the urgent need for blockchain technology to enhance traceability within the food supply chain.
The primary application of blockchain in the food industry’s digital transformation involves the development of a more traceable and transparent food supply chain tracking system, which has already been successfully implemented across various food categories. Food blockchain traceability systems offer stakeholders a comprehensive view of a product’s entire lifecycle, allowing food enterprises to trace product origins, optimizing logistics, and improving decision-making processes. Additionally, these systems convey precise food safety information to consumers, fostering trust through an innovative digital format (Nagaraj, 2021; Karamchandani et al., 2020). Therefore, achieving the goals of food blockchain traceability systems and encouraging their widespread adoption requires not only regulatory support and technological investments from companies but also strong acceptance and support from consumers and the general public (Marimuthu et al., 2022; Wirtz et al., 2022).
Despite widespread approval from businesses, the adoption of food blockchain traceability systems are hindered by a lack of consumer participation and low usage rates. Identifying key factors influencing consumer usage is crucial for increasing adoption rate. If consumers face barriers, experience aversion, or have no intention of using food blockchain traceability systems, the full potential of the technology will not be realized. Consequently, increasing consumer adoption intention is essential for improving the efficiency and effectiveness of these systems. Understanding consumers’ inclination toward using these systems, as well as the factors that influence their intention, is critical. While numerous studies in innovation adoption focus on blockchain technology from an enterprise perspective, few examine the influence of individual behavior on the adoption and diffusion of blockchain applications from the consumer standpoint (Qian et al., 2020; Marimuthu et al., 2022).
There are two major research directions in individual innovation adoption: one focuses on identifying attributes influencing perceptions and use of technology, where the Technology Acceptance Model (TAM) is widely recognized as one of the most influential and effective models for predicting innovation adoption (Kamal et al., 2020). TAM has been extensively used in studies assessing user acceptance of innovative technologies. The second direction seeks to explore individual characteristics that explain technology use, such as Technology Readiness (TR), which identifies individual predispositions toward using innovative technologies (Jafari-Sadeghi et al., 2021).
This study shifts from the traditional enterprise-focused perspective on blockchain adoption in the food supply chain to emphasize the consumer viewpoint. Unlike most research that centers on business adoption of blockchain or traceability systems, this study investigates consumer behaviors and adoption intentions in real-world retail environments, specifically targeting supermarket consumers in Shenzhen to explore their experiences and the factors that influence their decisions. The primary research questions guiding this study are as follows:
Q1: What factors influence consumer adoption intentions toward food blockchain traceability systms?
Q2: How do individual traits, such as personal knowledge, experiences, and technology readiness, affect consumers’ propensity to adopt digital technologies in the context of food traceability?
Q3: How can food and retail businesses design digital services that align with consumer expectations and enhance adoption?
Understanding consumer inclinations from a TR perspective is crucial as it provides insights into how food and retail businesses can design digital services that meet consumer expectations and enhance service offerings. This study integrates TAM and TR models to explore consumer tendencies toward food blockchain traceability systems, providing a solid theoretical foundation for understanding the factors that drive adoption. Employing SEM for statistical analysis, this study offers a multifaceted exploration of consumer attitudes and adoption intentions, revealing a comprehensive pattern of technology acceptance behaviors within the context of food blockchain traceability. The findings provide valuable insights for businesses in the food and retail sectors looking to integrate blockchain technology into their services, facilitating more effective implementation and enhanced consumer engagement strategies.
This paper is organized as follows: Section 2 reviews the existing literature, Section 3 presents the theoretical model framework and research hypotheses, Section 4 describes the survey instruments and research methods, Section 5 presents the data analysis and empirical findings, and Section 6 concludes the study with future research directions.
Blockchain is a distributed ledger technology designed to ensures data integrity, transparency, and security. Its ability to create immutable records and enable real-time tracking has made it a promising solution for supply chain management, especially in addressing issues related to food safety and fraud (Gourisetti et al., 2019). Over time, blockchain has evolved, initially applied in digital currencies, and now integrated with technologies such as the Internet of Things (IoT), artificial intelligence (AI), and big data, offering intelligent supply chain solutions (Rijanto, 2021; Siegfried et al., 2022).
In the food industry, blockchain technology is increasingly applied to enhance traceability, quality control, and supply chain collaboration: (i) Food safety and traceability: Blockchain enables end-to-end food traceability, allowing stakeholders to track product origins, processing, and distribution in real time. Empirical studies show that this transparency enhances consumer trust and minimizes risks associated with contamination and fraud (Srivastava and Dashora, 2022; Dehghani et al., 2022). (ii) Quality control and compliance: Blockchain records ensure the accuracy of food quality claims, such as organic certification and geographical indication labeling, reducing fraudulent activities in food labeling (Kshetri, 2019). (iii) Supply chain collaboration and efficiency: Blockchain enables smart contracts and automated data sharing, improving coordination among producers, distributors, and retailers, thereby increasing supply chain efficiency and resilience (Rejeb et al., 2020).
Empirical studies have primarily focused on corporate adoption of blockchain within food supply chains. Research on innovation adoption has established influential theories such as the TAM, Behavioral Reasoning Theory (BRT), Technology-Organization-Environment Framework (TOE), and the Diffusion of Innovation (DOI). Studies employing these theories show that while blockchain traceability solutions enhance food safety, adoption remains limited due to high implementation costs, regulatory complexities, and resistance from supply chain actors (Gourisetti et al., 2019; Karamchandani et al., 2020; Munir et al., 2022; Khan et al., 2023). More recent studies have shifted towards analyzing consumer perceptions, revealing that while consumers generally favor blockchain-based food traceability, their willingness to engage with such systems depends on ease of access, perceived benefits, and trust in the system (Paul et al., 2016; Kumar et al., 2022).
Understanding the key determinants of consumer adoption of food blockchain traceability systems is critical for successful implementation. Empirical research has identified several factors influencing consumer adoption, categorized into perceived benefits, usability, trust and security, and external influences: (i) Perceived benefits and utility: The main driver of consumer adoption is the belief that blockchain traceability enhances food safety, transparency, and authenticity. Studies suggest that when consumers perceive blockchain technology as effective in preventing food fraud and ensuring product quality, their likelihood of adoption significantly increases (Paul et al., 2016; Afzalan et al., 2017). Research further suggests that blockchain-enabled real-time tracking provides verifiable data, which enhances consumer confidence in food products (Dehghani et al., 2022). (ii) Usability and accessibility: Perceived ease of use plays a crucial role in shaping consumer adoption intentions. Blockchain applications that require technical knowledge or additional effort to access traceability information may discourage adoption (Jafari-Sadeghi et al., 2021). Simplified user interfaces, mobile accessibility, and integration with existing consumer apps have been proposed as solutions to enhance adoption rates (Marimuthu et al., 2022). (iii) Despite blockchain’s inherent security features, data privacy concerns and a lack of trust in digital transactions remain key barriers to adoption (Kamal et al., 2020). Consumers worry about the potential misuse of their data and whether blockchain systems are truly tamper-proof. Empirical findings suggest that higher transparency in data-sharing policies and clear consumer education on blockchain security can significantly mitigate trust-related concerns (Sawrikar and Mote, 2022). (iv) External influences: Social norms and regulatory support—such as the endorsement of blockchain traceability by retailers, regulatory bodies, and industry leaders—positively influence consumer attitudes toward adoption (Marimuthu et al., 2022). Social influence, including peer recommendations, expert reviews, or governmental mandates, has been shown to increase consumer trust and willingness to engage with blockchain-based food traceability systems (Wirtz et al., 2022). Empirical studies confirm that these factors collectively shape consumer adoption attitudes and behavioral intentions. For instance, a study conducted in China found that younger, urban consumers exhibit a higher likelihood of adopting blockchain traceability, while older consumers tend to be skeptical due to a lack of familiarity with the technology (Wirtz et al., 2022). The findings underscore the need for targeted consumer education, regulatory backing, and user-friendly system design to enhance adoption rates.
Consumer adoption is crucial for the successful integration and widespread implementation of food blockchain traceability systems. TAM and TR have been employed to examine consumer adoption behavior, particularly. The TAM framework posits that two key factors—perceived ease of use and perceived usefulness—determine an individual’s intention to adopt new technology (Davis et al., 1989). Blockchain traceability must be both user-friendly and beneficial to encourage consumer adoption. TR explains how an individual’s predisposition to accept new technology affects their adoption behavior. Optimism and innovativeness positively influence adoption, whereas discomfort and insecurity act as barriers (Parasuraman, 2000; Cruz-Cárdenas et al., 2021). Since food blockchain traceability systems are user-oriented innovative digital services, with consumers actively participating in the service creation, relying solely on TAM is insufficient to explain consumers’ technology adoption behaviors for digital services. There is a need to incorporate factors that account for individual differences among consumers and understand their psychological processes in evaluating technological value. By integrating TAM with TR, insights can be gained into how individual traits affect perceived ease of use and perceived usefulness, offering a deeper understanding of consumers’ acceptance levels for specific digital service technologies in real retail scenarios (Seong and Hong, 2022). Thus, combining TAM’s system attributes with TR’s individual traits offers a more comprehensive explanation of how personality types influence interactions with and adoption of new technologies (Kolade et al., 2022).
Current studies on the adoption of food blockchain traceability systems primarily focus on evaluating the technological implementation behaviors of food enterprises, with limited attention given to the consumer’s perspective in the retail service domain. This research builds upon the TAM by investigating the influence of its two core elements, perceived ease of use and perceived usefulness, on consumers’ attitudes and intentions to use food blockchain traceability system services. Additionally, four individual trait variables from TR are incorporated as moderating variables. This approach provides a more comprehensive analysis of consumers’ intentions to use food blockchain traceability system in real retail environments. The theoretical model framework is illustrated in Figure 1.
Perceived ease of use refers to the degree to which a user believes that a specific technology is easy to use (Davis et al., 1989). Many information technology products are designed with user-friendly features and reduced technical complexity, enabling users to adopt the technology with minimal learning or training (Venkatesh et al., 2012). Perceived usefulness refers to a user’s belief that using a specific technology will meet their expectations or enhance their performance (Davis et al., 1989). This reflects an individual’s confidence in the effectiveness of a technology, similar to the perceived benefits derived from its use (Yu et al., 2017). If a technology is perceived as easy to use, it enables the user to complete tasks more efficiently, thus improving performance. Based on this reasoning, we propose the following hypothesis:
H1: Perceived ease of use positively affects perceived usefulness.
Adoption attitude represents a user’s overall evaluation and psychological response to a specific technology (Kim and Han, 2010). It typically includes cognitive, emotional, and intentional factors (Davis et al., 1989; Venkatesh et al., 2012). Perceived ease of use enhances a user’s sense of control, implying that the user does not need to exert significant physical or mental effort, thereby increases their intention to use the technology. Thus, perceived ease of use is expected to positively influence adoption attitude. Adoption attitude is shaped by personal beliefs and the perceived outcomes of behavior (Ajzen et al., 2018). When technology meets user objectives effectively, and perceived usefulness positively influences adoption attitude. In other words, adoption attitude arises from individual beliefs, and when users perceive that a technology enhances performance and is easy to use, their attitude toward the technology improves. The positive relationships between perceived ease of use, perceived usefulness, and adoption attitude have been validated in numerous studies. Based on this, the following hypotheses are proposed:
H2: Perceived ease of use positively affects adoption attitude.
H3: Perceived usefulness positively affects adoption attitude.
Behavioral intention refers to the strength of an individual’s intention to perform a specific action (Ajzen et al., 2018; Paul et al., 2016). According to TAM, Theory of Reasoned Action (TRA), Theory of Planned Behavior (TPB), and other theories, attitude is a strong predictor of the intention to adopt innovative behavior. Specifically, the more positively an individual feels about innovative technology, the stronger their intention to use it. A substantial body of research has confirmed that individuals with a positive attitude toward innovative technology are more likely to adopt or continue using it (Jaiswal et al., 2021; Lembcke et al., 2021). Therefore, we propose the following hypothesis:
H4: Adoption attitude positively affects adoption intention.
TR as defined by Parasuraman (2000) refers to individuals’ tendency to accept and use new technologies for daily life or work objectives. TR is categorized into four dimensions: (i) Optimism: A positive attitude toward new technologies, believing they can enhance control, convenience, flexibility, and efficiency; (ii) Innovativeness: A person’s inclination to be a pioneer in new technologies, showing a keen interest in learning and exploring them; (iii) Discomfort: The feeling of being unable to master new technologies, accompanied by a sense of powerlessness and fear; (iv) Insecurity: A lack of confidence in the flawless operation of new technologies, with concerns about data security, device malfunctions, and system errors. Numerous empirical studies indicate that TR influences the intention to use digital service technologies and moderates TAM. Among these dimensions, positive drivers such as optimism and innovativeness positively influence adoption intention, while negative barriers like discomfort and insecurity adversely affect adoption intention (Borrero et al., 2014; Parasuraman and Colby, 2015; Cruz-Cárdenas et al., 2019; Cruz-Cárdenas et al., 2021). Based on this, the following hypotheses are proposed:
H5a: Optimism moderates the relationship between perceived ease of use and adoption attitude.
H5b: Innovativeness moderates the relationship between perceived ease of use and adoption attitude.
H5c: Discomfort moderates the relationship between perceived ease of use and adoption attitude.
H5d: Insecurity moderates the relationship between perceived ease of use and adoption attitude”.
H6a: Optimism moderates the relationship between perceived usefulness and adoption attitude”.
H6b: Innovativeness moderates the relationship between perceived usefulness and adoption attitude.
H6c: Discomfort moderates the relationship between perceived usefulness and adoption attitude.
H6d: Insecurity moderates the relationship between perceived usefulness and adoption attitude.
A questionnaire was administered to consumers aged 18 and above to assess their attitudes and intentions toward adopting the food blockchain traceability system, as well as the factors influencing these attitudes and intentions. The primary target group for the survey was young consumers for two main reasons: (i) Shenzhen has the youngest demographics in China. In 2020, the average age of Shenzhen’s permanent population was 32.5 years, 6.3 years younger than the national average. (ii) Urban young consumers in Shenzhen generally have higher education levels, greater disposable incomes, and higher technological literacy, making them more knowledgeable about food traceability technologies and more inclined to pay a premium for traceable food products. The survey was conducted over a three-week period from April 15 to May 30, 2024, in the food sections of four major supermarkets in Shenzhen: Walmart, Carrefour, Vanguard, and Rainbow. A team of three researchers conducted the survey using non-probability convenience sampling, a method commonly used in exploratory research to capture insights from relevant consumer groups. Participants were randomly approached in the food sections of the selected supermarkets, briefed on the purpose of the study, and screened for prior knowledge and experience of food traceability systems or blockchain technology. Only those who met the inclusion criteria were invited to participate. After careful screening, 400 responses were initially collected. Following the exclusion of invalid responses due to issues such as sequence bias, incomplete answers, and other factors, 322 valid responses were retained for analysis. This sample size was determined based on practical considerations, including resource constraints and the exploratory nature of the study. While it may limit the generalizability of the findings beyond Shenzhen, it provides sufficient data for initial insights into consumer attitudes toward food blockchain traceability. Prior consumer behavior studies suggest that sample sizes between 200 and 400 respondents are generally adequate for SEM analysis, ensuring the robustness of model testing and hypothesis validation (Hair et al., 2019).
Research variables were measured using multi-item scales, with each item rated on a five-point Likert scale (1 = Strongly Disagree, 5 = Strongly Agree). The questionnaire items were adapted from validated theoretical frameworks, ensuring consistency with prior research. To enhance content validity, the questionnaire underwent expert review, incorporating feedback from five industry and academic experts, including two senior food industry executives, one secretary-general of a food industry association, and two scholars specializing in food engineering and supply chain management. The items and their references are summarized in Table 1.
Following data collection and encoding, several statistical methods were employed: (i) Descriptive statistics: Used to summarize demographic distributions and general response patterns. (ii) Reliability and validity analysis: Assessed using Cronbach’s alpha and Composite Reliability (CR) for internal consistency, while Average Variance Extracted (AVE) tested construct validity. (iii) SEM: Applied to examine latent construct relationships and assess the overall model fit. SEM was chosen for its ability to analyze complex interrelationships among multiple variables simultaneously. Model fit indicators such as χ2/df, CFI, NFI, RMSEA, and SRMR were used to validate the robustness of the model (Hair et al., 2019). (iv) Hierarchical regression analysis: Used to examine the incremental explanatory power of different predictor variables by entering them into the regression model in sequential steps. This technique was specifically employed to assess how TR moderates the effects of perceived usefulness and perceived ease of use on adoption attitude.
Table 2 summarizes the demographic distribution of respondents, providing insights into key characteristics such as age, education level, and blockchain awareness. Among the valid responses, females comprised the majority, accounting for 191 respondents (59.32%). Regarding age, the majority of respondents (263 individuals, over 80% of the sample) were aged 19–40 years. In terms of educational background, most respondents had a college or vocational degree (162 respondents, 50.31%), followed by those with a master’s degree (115 respondents, 35.71%). Regarding familiarity with food traceability-related technologies (such as RFID, POS, GPS, EDI, ERP, barcodes, blockchain, etc.), nearly half of the respondents reported having some knowledge, while approximately 40% were moderately familiar. As for the frequency of traceability technology usage over the past month, the most common response was 1–4 experiences (39.44%), followed by 5–10 experiences (30%). However, 20% of respondents reported no usage of such technologies in the last month.
The predominance of younger, highly educated participants aligns with the study’s focus on exploring technology readiness in urban populations. Given that younger consumers tend to have higher digital literacy and are more open to technological innovations, this demographic distribution is particularly relevant for examining blockchain adoption in food traceability. However, an interesting observation arises: despite the high educational attainment of respondents, 47.83% reported only awareness of food traceability technology, and 20% had not used such technology in the past month. This suggests that while education promotes awareness, it does not necessarily lead to frequent usage. Two factors may explain this phenomenon: (i) Perceived relevance: Educated consumers may be aware of food traceability technologies but may not perceive them as directly relevant to their daily lives or purchasing decisions. (ii) Accessibility and availability: The integration of traceability technologies into consumer-facing platforms may be limited, reducing opportunities for consumers to engage with these systems regularly. This demographic bias may limit the generalizability of findings, especially to older or rural consumer groups. Additionally, the discrepancy between awareness and usage highlights the need for further research to understand the barriers to adoption among educated consumers.
Table 3 presents the descriptive statistics for all variables, including mean values, standard deviations, and correlation coefficients. The mean values of the variables range from 2.95 to 3.91, with standard deviations between 0.50 and 0.75, suggesting that consumers exhibit a moderately positive attitude toward the food blockchain traceability system, with some variability in perceptions of ease of use and perceived security. Specifically, Perceived ease of use (PEU) has the lowest mean value of 2.95, indicating that respondents found the system not particularly user-friendly, which suggests potential barriers to adoption. Perceived usefulness (PU) has a high mean of 3.84, indicating that respondents generally agree the system provides expected benefits. The mean values for Adoption attitude (AA) and Adoption intention (AI) are 3.57 and 3.70, respectively, suggesting positive attitudes toward the system and intend to continue using it. Optimism (OP) shows a mean of 3.91, reflecting positive consumer sentiment toward the system, while Insecurity (IS) (mean = 3.76) emphasizes consumer concern over security and privacy protection. Innovativeness (IN) and Discomfort (DC) show mean values of 3.27 and 3.21, indicating that consumers are neither particularly enthusiastic about learning new technologies nor highly resistant to them. Furthermore, the correlation coefficients reveal significant positive relationships between the listed factors, attitude, and intention. However, it is important to note that the correlation between IS and DC is weak (0.11), indicating a modest association between these two variables. This suggests that the relationship between security concerns and discomfort may not be as strong as anticipated. This finding underscores the complexity of consumer attitudes toward the blockchain system, with security concerns and discomfort influencing adoption behavior in different ways.
The measurement model was assessed for reliability and validity to ensure the robustness of the constructs. Table 4 outlines the results. Convergent validity was assessed via confirmatory factor analysis. Among the initial 28 items, two items (OP2 and IS2, with loadings factor of 0.55 and 0.59, respectively) were excluded due to factor loadings below the threshold of 0.7. For discriminant validity, all latent variable had Average Variance Extracted (AVE) values exceeding the 0.5 threshold, indicating good discriminant validity. Regarding reliability, Cronbach’s α and Composite Reliability (CR) were used to assess internal consistency. Cronbach’s α coefficients range from 0.80 and 0.89, all above the standard threshold of 0.50, and CR values ranged from 0.89 to 0.94, surpassing the 0.7 threshold, indicating high reliability. Lastly, multicollinearity was assessed using Variance Inflation Factor (VIF) values, which ranged from 1.91 to 3.17, all below the 5.0 threshold, indicating well-contained multicollinearity. These results confirm that the construct validity aligns with the theoretical framework of the study. Given that the constructs are based on established theories in TAM and TR, this validation strengthens the relevance of the findings in answering research questions related to blockchain adoption.
LISREL was utilized to verify the model’s fit and the hypothesized causal relationships within the research framework. The results show that all fit indices met their respective standards (χ2(40) = 159.28, χ2/df = 3.98, GFI = 0.93, AGFI = 0.90, NFI = 0.94, NNFI = 0.94, CFI = 0.96, RMSEA = 0.042), indicating a strong fit between the data and the model.
The estimated results of the causal relationships among the variables in the structural model are presented in Figure 2. Based on the standardized estimates and t-values, the following results were observed: (i) PEU positively affects PU (H1: β = 0.67, t = 12.82, p < 0.01), suggesting that when consumers perceive the food blockchain traceability system as easy to use, they also find it more useful, thus supporting H1. (ii) PEU positively affects AA (H2: β = 0.49, t = 8.09, p < 0.01), indicating that the easier consumers find the food blockchain traceability system to use, the more positive their attitude toward its use, confirming H2. (iii) PU also positively impacts AA (H3: β = 0.34, t = 5.79, p < 0.01), meaning that the more consumers perceive the system to be useful, the more positive their attitude is toward its use, thereby validating H3. (iv) Lastly, AA positively influences AI (H4: β = 0.88, t = 17.46, p < 0.001), revealing that the more positive consumers’ attitudes are toward the system, the higher their intention to use it, thus substantiating H4.
The validation of TAM confirms that consumers’ PEU and PU significantly influence their AA, which in turn affects their AI. Notably, PEU exhibited a stronger positive effect than PU. These findings suggest that usability plays a more significant role than perceived usefulness in shaping consumers’ adoption intentions. This contrasts with some prior studies on technology adoption, where PU is typically a stronger determinant of behavioral intentions (Venkatesh and Bala, 2008). However, in the context of blockchain food traceability, it appears that consumers prioritize usability and accessibility over the perceived benefits of the technology itself. This highlights the need for user-friendly blockchain interfaces and seamless integration with existing purchasing platforms to encourage widespread adoption. Despite the positive association between PEU and AI, challenges remain. Some consumers may still perceive blockchain as technically complex and difficult to understand, even if the system is designed to be user-friendly. Additionally, PU’s relatively lower impact suggests that consumers may remain uncertain about the tangible benefits of blockchain-based traceability. Studies have shown that perceived security, trust, and regulatory endorsement can further influence blockchain adoption (Kamble et al., 2019; Balasubramanian et al., 2021). Therefore, businesses and policymakers should focus on enhancing consumer trust, increasing awareness of blockchain’s value, and simplifying user interactions to drive adoption.
To examine the influence of individual traits on the relationships between the key variables—PEU, PU, and AA—within the TAM, hierarchical regression analysis was conducted. The significance of the interaction terms between independent variables and moderating variables determines the presence of a moderating effect. Therefore, hierarchical regression was performed with AA as the dependent variable and PEU, PU, and their interaction terms as the independent variables. The results are displayed in Table 5. Model 1 includes only the main effects, consisting of the independent variables PEU and PU. Model 2 introduces eight interaction terms: “OP × PEU,” “IN × PEU,” “DC × PEU,” “IN × PEU,” “OP × PU,” “IN × PU,” “DC × PU,” and “IS × PU” to test hypotheses H5a to H5d and H6a to H6d regarding the moderating effects.
The overall regression models for both Model 1 and Model 2 were statistically significant (F = 194.08 and 44.97, respectively). Additionally, PEU was significant in both Model 1 (β = 0.38, t = 8.76) and Model 2 (β = 0.43, t = 9.61). Similarly, PU was significant in both Model 1 (β = 0.41, t = 9.43) and Model 2 (β = 0.39, t = 8.81), indicating that both PEU and PU positively influence AA. Thus, hypotheses H2 and H3 are supported.
Next, the moderating effects were evaluated based on the significance of the interaction terms in Model 2: (i) The interaction between OP and PEU was significant (β = −0.12, t = −2.06), suggesting that the relationship between PEU and AA is moderated by OP. Specifically, as users become less optimistic about the technology, the relationship between PEU and AA strengthens. This means that when users have negative perceptions of new technology, ease of use makes them more willing to adopt it. Therefore, H5a is supported. (ii) The interaction between OP and PU was also significant (β = 0.12, t = 2.06), implying that as users’ positive feelings toward the technology increase, the relationship between PU and AA becomes stronger. When users view new technology positively, its usefulness becomes a more significant factor in their willingness to use it. Therefore, H6a is supported. (iii) The interaction between IN and PEU was significant (β = 0.22, t = 3.62), indicating that the relationship between PEU and AA is influenced by users’ level of innovative traits. Specifically, as users’ degree of innovativeness increases, the relationship between PEU and AA strengthens. If users are highly receptive to new technologies, and the technology is user-friendly, this boosts their adoption attitude. Therefore, H5b is supported. (iv) The interaction between IN and PU was also significant (β = −0.19, t = −2.94), suggesting that as users’ innovativeness decreases, the relationship between PU and AA strengthens. Therefore, H6b is supported. (v) The interactions between DC and PEU (β = −0.08, t = −1.45) and DC and PU (β = 0.04, t = 0.76) were not significant, meaning H5c and H6c are not supported. (vi) The interactions between IS and PEU (β = 0.05, t = 0.93) and IS and PU (β = −0.06, t = −1.31) were also not significant, indicating that H5d and H6d are not supported.
These findings are essential for understanding how individual traits influence adoption behavior. Specifically, they show that factors such as OP and IN can significantly influence the relationship between PEU/PU and AA. However, traits such as DC and IS seem to have lesser impact, suggesting that consumers’ perceptions of ease of use and usefulness are more strongly influenced by their overall optimism and innovativeness than by concerns related to security or discomfort. High-TR consumers, who are more technologically inclined, are more receptive to blockchain innovations and more willing to integrate traceability systems into their purchasing behavior. In contrast, low-TR consumers exhibit weaker associations between these constructs, potentially due to skepticism, lack of trust, or difficulties in interacting with blockchain-based traceability platforms. However, an overreliance on early adopters and high-TR consumers could lead to market imbalances, as low-TR consumers may be excluded from accessing blockchain-enhanced food traceability systems. Prior studies suggest that technological divides could exacerbate disparities in access to food safety innovations (Balasubramanian et al., 2021). If blockchain traceability remains too complex or is primarily marketed toward digitally literate, high-income consumers, it may fail to achieve widespread adoption. To mitigate these challenges, companies and policymakers should ensure that blockchain-based food traceability systems are designed to be inclusive and user-friendly, offering accessible interfaces, simplified digital literacy requirements, and education programs that bridge the technology readiness gap. A multi-tiered approach, targeting both high-and low-TR consumers with different engagement strategies, could enhance adoption rates across diverse demographic segments.
The food blockchain traceability system harnesses blockchain technology to improve the transparency and security of the food supply chain. By reducing information asymmetry, this system provides consumers with greater access to resources and services, thus elevating the overall service quality in the food and retail sectors. Consequently, enhancing consumer adoption intentions toward the food blockchain traceability system is essential for realizing its full potential within the food supply chain. This study integrates the TAM and TR to examine the factors influencing consumer intentions toward adopting the food blockchain traceability system in real retail environments. The study also explores the moderating effects of consumers’ TR, leading to the following conclusions:
First, TAM effectively predicts consumers’ attitudes and intentions regarding the food blockchain traceability system. The validation results confirm hypotheses H1, H2, H3, and H4: Perceived ease of use significantly positively influences perceived usefulness, perceived usefulness significantly positively impacts adoption attitude, and adoption attitude significantly positively affects adoption intention. These findings align with the core principles of TAM. The positive impact of perceived ease of use on adoption attitude highlights the importance of creating user-friendly system interfaces and environments to enhance consumers’ willingness to adopt the technology. The positive impact of perceived usefulness on adoption attitude reinforces the consumer-oriented nature of the blockchain traceability system, suggesting that its effectiveness and perceived benefits foster positive consumer responses. Furthermore, the strong positive effect of perceived ease of use on perceived usefulness indicates that a relatively low technical complexity increases consumers’ confidence in the technology’s effectiveness and utility. Additionally, the substantial positive effect of adoption attitude on adoption intention confirms that consumers’ acceptance and recognition of the food blockchain traceability system are strong predictors of their intention to use it.
Second, the positive dimensions of TR, such as optimism and innovation, exhibit significant moderating effects on TAM, while the negative dimensions, such as Discomfort and Insecurity, do not. TR, as a measure of individual characteristics, shows that personal differences can moderate the factors influencing the use of food blockchain traceability services and related attitudes. The validation results indicate that the positive dimensions of TR, namely optimism and innovation, moderate the relationships between perceived ease of use, perceived usefulness, and adoption attitude, thus supporting hypotheses H5a, H5b, H6a, and H6b. Conversely, the negative dimensions of TR, including discomfort and insecurity, do not show significant moderating effects on these relationships, leading to the rejection of hypotheses H5c, H5d, H6c, and H6d. The lack of significant moderating effects from discomfort and insecurity may be attributed to the blockchain traceability system’s ability to minimize complex technological barriers and alleviate concerns related to data security.
In the context of the food supply chain ecosystem, the implementation and promotion of blockchain traceability services must prioritize the consumer’s perspective. Focusing on how consumers benefit from the technology, and how their behaviors influence the adoption of innovative technology, will allow us to maximize the potential of blockchain to reshape industry standards and enhance societal welfare.
Theoretical Implications: Unlike previous studies on blockchain adoption that primarily focused on corporate implementation, this study shifts the focus to the retail service domain, evaluating consumer behavior with respect to the food blockchain traceability system. This approach addresses a significant research gap concerning consumer adoption of blockchain application systems. Furthermore, by integrating TR with TAM, the study uncovers the varying moderating effects of different TR dimensions on TAM, thereby refining the theoretical framework.
Practical Implications: For successful blockchain implementation in the food retail sector, it is critical to focus on consumers to optimize services and encourage higher participation rates. First, regarding usability, as usability influences user attitudes through perceived utility, and given that consumer evaluations of the blockchain traceability system’s usability are relatively low, it is essential for food and retail businesses to improve interface design and incorporate engaging content. This will enhance consumer perceptions of both usability and utility, thus maximizing benefits and boosting adoption rates. Second, in terms of utility, food and retail businesses should intensify advocacy for the blockchain traceability system. Various platforms such as social media, online advertisements, and product packaging descriptions can be leveraged to emphasize the system’s ability to address food safety concerns. Marketers should underscore the system’s key features, including identity verification, visibility, data tampering prevention, and traceability. By fostering a positive and innovative attitude among consumers, businesses can build confidence in the benefits and convenience offered by blockchain traceability systems. Lastly, by focusing on consumers’ TR, food and retail businesses should actively project a positive image that highlights their commitment to food safety, technological advancements, and convenience. This strategy can help alleviate negative perceptions related to incompatibility and insecurity within the retail service domain. Additionally, enhancing consumers’ digital literacy and information skills will foster optimism and innovation, building trust in the benefits and convenience of the food blockchain traceability system. This, in turn, can increase consumers’ willingness to pay a premium for traceable food products.
This study has several limitations that should be acknowledged and addressed in future research. First, this research is grounded in the TAM and TR theories, which limits the scope of factors considered. Future research could integrate theories, such as BRT, TOE, DOI, and UTAUT (Unified Theory of Acceptance and Use of Technology), to encompass a broader range of influencing factors. This would further refine our understanding of consumer behavior toward food blockchain traceability systems. Second, the research sample was drawn from a single urban setting (Shenzhen), which may limit the generalizability of the findings to other geographic or cultural contexts. Shenzhen is a highly developed, technology-oriented city, and consumer attitudes toward blockchain adoption may differ in less urbanized regions or international markets where food safety concerns, regulatory environments, and technological literacy levels vary. Future research should extend the scope to cross-regional and cross-cultural studies, comparing consumer adoption patterns in different cities, rural areas, and global markets to offer a more comprehensive perspective. Third, this study is based on cross-sectional data, capturing consumer attitudes at a single point in time. However, blockchain technology and food traceability systems are evolving rapidly, and consumer perceptions may change as these technologies become more widespread and accessible. Future research should consider longitudinal studies that track changes in consumer adoption intentions over time, particularly in response to technological advancements, regulatory shifts, and increasing public awareness of food safety. These studies would provide a more dynamic understanding of adoption behaviors and help anticipate long-term trends in blockchain integration within the food industry. Finally, although this study focuses on blockchain adoption specifically within food traceability, the technology has broader potential applications. Future research could explore how blockchain contributes to wider food supply chain management aspects, such as sustainability tracking, carbon footprint measurement, and food waste reduction. Investigating these areas would provide a more comprehensive understanding of blockchain’s transformative role in the food industry, aligning with global efforts toward sustainable and transparent food systems. By addressing these limitations, future studies can build upon the findings of this research, offering a more holistic and nuanced perspective on blockchain adoption in food systems. Ultimately, this will contribute to both academic knowledge and industry practice.
The original contributions presented in the study are included in the article/supplementary material, further inquiries can be directed to the corresponding author.
WL: Conceptualization, Investigation, Methodology, Writing – original draft, Writing – review & editing. RS: Formal analysis, Methodology, Visualization, Writing – original draft. KY: Conceptualization, Investigation, Project administration, Resources, Writing – review & editing.
The author(s) declare that financial support was received for the research and/or publication of this article. This work has been fully financed by the Major Program of Fujian Province Social Science Foundation (No. FJ2023A008).
The authors declare that the research was conducted in the absence of any commercial or financial relationships that could be construed as a potential conflict of interest.
The author(s) declare that no Generative AI was used in the creation of this manuscript.
All claims expressed in this article are solely those of the authors and do not necessarily represent those of their affiliated organizations, or those of the publisher, the editors and the reviewers. Any product that may be evaluated in this article, or claim that may be made by its manufacturer, is not guaranteed or endorsed by the publisher.
Afzalan, N., Sanchez, T. W., and Evans-Cowley, J. (2017). Creating smarter cities: considerations for selecting online participatory tools. Cities 67, 21–30. doi: 10.1016/j.cities.2017.04.002
Ajzen, I., Fishbein, M., Lohmann, S., and Albarracín, D. (2018). “The influence of attitudes on behavior” in The handbook of attitudes, volume 1: Basic principles. eds. D. Albarracin and B. T. Johnson. 2nd ed (New York: Routledge), 197–255.
Balasubramanian, S., Shukla, V., Sethi, J. S., Islam, N., and Saloum, R. (2021). A readiness assessment framework for Blockchain adoption: a healthcare case study. Technol. Forecast. Soc. Chang. 165:120536. doi: 10.1016/j.techfore.2020.120536
Borrero, J. D., Yousafzai, S. Y., Javed, U., and Page, K. L. (2014). Expressive participation in internet social movements: testing the moderating effect of technology readiness and sex on student SNS use. Comput. Hum. Behav. 30, 39–49. doi: 10.1016/j.chb.2013.07.032
Centobelli, P., Cerchione, R., Del Vecchio, P., Oropallo, E., and Secundo, G. (2022). Blockchain technology for bridging trust, traceability and transparency in circular supply chain. Inf. Manag. 59:103508. doi: 10.1016/j.im.2021.103508
Cruz-Cárdenas, J., Guadalupe-Lanas, J., Ramos-Galarza, C., and Palacio-Fierro, A. (2021). Drivers of technology readiness and motivations for consumption in explaining the tendency of consumers to use technology-based services. J. Bus. Res. 122, 217–225. doi: 10.1016/j.jbusres.2020.08.054
Cruz-Cárdenas, J., Zabelina, E., Deyneka, O., Guadalupe-Lanas, J., and Velín-Fárez, M. (2019). Role of demographic factors, attitudes toward technology, and cultural values in the prediction of technology-based consumer behaviors: a study in developing and emerging countries. Technol. Forecast. Soc. Chang. 149:119768. doi: 10.1016/j.techfore.2019.119768
Davis, F. D., Bagozzi, R. P., and Warshaw, P. R. (1989). User acceptance of computer technology: a comparison of two theoretical models. Manag. Sci. 35, 982–1003. doi: 10.1287/mnsc.35.8.982
Dehghani, M., Popova, A., and Gheitanchi, S. (2022). Factors impacting digital transformations of the food industry by adoption of blockchain technology. J. Bus. Ind. Mark. 37, 1818–1834. doi: 10.1108/JBIM-12-2020-0540
Gourisetti, S. N. G., Mylrea, M., and Patangia, H. (2019). Evaluation and demonstration of blockchain applicability framework. IEEE Trans. Eng. Manag. 67, 1142–1156. doi: 10.1109/TEM.2019.2928280
Hair, J. F., Risher, J. J., Sarstedt, M., and Ringle, C. M. (2019). When to use and how to report the results of PLS-SEM. Eur. Bus. Rev. 31, 2–24. doi: 10.1108/EBR-11-2018-0203
Jafari-Sadeghi, V., Garcia-Perez, A., Candelo, E., and Couturier, J. (2021). Exploring the impact of digital transformation on technology entrepreneurship and technological market expansion: the role of technology readiness, exploration and exploitation. J. Bus. Res. 124, 100–111. doi: 10.1016/j.jbusres.2020.11.020
Jaiswal, D., Kaushal, V., Kant, R., and Singh, P. K. (2021). Consumer adoption intention for electric vehicles: insights and evidence from Indian sustainable transportation. Technol. Forecast. Soc. Chang. 173:121089. doi: 10.1016/j.techfore.2021.121089
Kamal, S. A., Shafiq, M., and Kakria, P. (2020). Investigating acceptance of telemedicine services through an extended technology acceptance model (TAM). Technol. Soc. 60:101212. doi: 10.1016/j.techsoc.2019.101212
Kamble, S., Gunasekaran, A., and Arha, H. (2019). Understanding the Blockchain technology adoption in supply chains-Indian context. Int. J. Prod. Res. 57, 2009–2033. doi: 10.1080/00207543.2018.1518610
Karamchandani, A., Srivastava, S. K., and Srivastava, R. K. (2020). Perception-based model for analyzing the impact of enterprise blockchain adoption on SCM in the Indian service industry. Int. J. Inf. Manag. 52:102019. doi: 10.1016/j.ijinfomgt.2019.10.004
Khan, S., Kaushik, M. K., Kumar, R., and Khan, W. (2023). Investigating the barriers of blockchain technology integrated food supply chain: a BWM approach. BIJ 30, 713–735. doi: 10.1108/BIJ-08-2021-0489
Kim, Y., and Han, H. (2010). Intention to pay conventional-hotel prices at a green hotel–a modification of the theory of planned behavior. J. Sustain. Tour. 18, 997–1014. doi: 10.1080/09669582.2010.490300
Kolade, O., Odumuyiwa, V., Abolfathi, S., Schröder, P., Wakunuma, K., Akanmu, I., et al. (2022). Technology acceptance and readiness of stakeholders for transitioning to a circular plastic economy in Africa. Technol. Forecast. Soc. Chang. 183:121954. doi: 10.1016/j.techfore.2022.121954
Kshetri, N. (2019). Blockchain and the economics of food safety. IT Prof. 21, 63–66. doi: 10.1109/MITP.2019.2906761
Kumar, N., Upreti, K., and Mohan, D. (2022). Blockchain adoption for provenance and traceability in the retail food supply chain: a consumer perspective. Int. J. E-Bus. Res. 18, 1–17. doi: 10.4018/IJEBR.294110
Lembcke, T. B., Herrenkind, B., Nastjuk, I., and Brendel, A. B. (2021). Promoting business trip ridesharing with green information systems: a blended environment perspective. Transp. Res. Part D: Transp. Environ. 94:102795. doi: 10.1016/j.trd.2021.102795
Marimuthu, M., D'Souza, C., and Shukla, Y. (2022). Integrating community value into the adoption framework: a systematic review of conceptual research on participatory smart city applications. Technol. Forecast. Soc. Chang. 181:121779. doi: 10.1016/j.techfore.2022.121779
Mc Carthy, U., Uysal, I., Badia-Melis, R., Mercier, S., O’Donnell, C., and Ktenioudaki, A. (2018). Global food security–issues, challenges and technological solutions. Trends Food Sci. Technol. 77, 11–20. doi: 10.1016/j.tifs.2018.05.002
Munir, M. A., Habib, M. S., Hussain, A., Shahbaz, M. A., Qamar, A., Masood, T., et al. (2022). Blockchain adoption for sustainable supply chain management: economic, environmental, and social perspectives. Front. Energy Res. 10:899632. doi: 10.3389/fenrg.2022.899632
Nagaraj, S. (2021). Role of consumer health consciousness, food safety & attitude on organic food purchase in emerging market: a serial mediation model. J. Retail. Consum. Serv. 59:102423. doi: 10.1016/j.jretconser.2020.102423
Parasuraman, A. (2000). Technology readiness index (TRI) a multiple-item scale to measure readiness to embrace new technologies. J. Serv. Res. 2, 307–320. doi: 10.1177/109467050024001
Parasuraman, A., and Colby, C. L. (2015). An updated and streamlined technology readiness index: TRI 2.0. J. Serv. Res. 18, 59–74. doi: 10.1177/1094670514539730
Paul, J., Modi, A., and Patel, J. (2016). Predicting green product consumption using theory of planned behavior and reasoned action. J. Retail. Consum. Serv. 29, 123–134. doi: 10.1016/j.jretconser.2015.11.006
Qian, J., Ruiz-Garcia, L., Fan, B., Villalba, J. I. R., Mc Carthy, U., Zhang, B., et al. (2020). Food traceability system from governmental, corporate, and consumer perspectives in the European Union and China: a comparative review. Trends Food Sci. Technol. 99, 402–412. doi: 10.1016/j.tifs.2020.03.025
Rejeb, A., Keogh, J. G., Zailani, S., Treiblmaier, H., and Rejeb, K. (2020). Blockchain technology in the food industry: a review of potentials, challenges and future research directions. Logistics 4:27. doi: 10.3390/logistics4040027
Rijanto, A. (2021). Business financing and blockchain technology adoption in agroindustry. J. Sci. Technol. Policy Manag. 12, 215–235. doi: 10.1108/JSTPM-03-2020-0065
Sawrikar, V., and Mote, K. (2022). Technology acceptance and trust: overlooked considerations in young people’s use of digital mental health interventions. Health Policy Technol. 11:100686. doi: 10.1016/j.hlpt.2022.100686
Seong, B. H., and Hong, C. Y. (2022). Corroborating the effect of positive technology readiness on the intention to use the virtual reality sports game “screen golf”: focusing on the technology readiness and acceptance model. Inf. Process. Manag. 59:102994. doi: 10.1016/j.ipm.2022.102994
Siegfried, N., Rosenthal, T., and Benlian, A. (2022). Blockchain and the industrial internet of things: a requirement taxonomy and systematic fit analysis. J. Enterp. Inf. Manag. 35, 1454–1476. doi: 10.1108/JEIM-06-2018-0140
Srivastava, A., and Dashora, K. (2022). Application of blockchain technology for agrifood supply chain management: a systematic literature review on benefits and challenges. BIJ 29, 3426–3442. doi: 10.1108/BIJ-08-2021-0495
Venkatesh, V., and Bala, H. (2008). Technology acceptance model 3 and a research agenda on interventions. Decis. Sci. 39, 273–315. doi: 10.1111/j.1540-5915.2008.00192.x
Venkatesh, V., Thong, J. Y., and Xu, X. (2012). Consumer acceptance and use of information technology: extending the unified theory of acceptance and use of technology. MIS Q. 36, 157–178. doi: 10.2307/41410412
Wirtz, B. W., Becker, M., and Schmidt, F. W. (2022). Smart city services: an empirical analysis of citizen preferences. Public Organ. Rev. 22, 1063–1080. doi: 10.1007/s11115-021-00562-0
Keywords: blockchain, food traceability system, technology acceptance model (TAM), technology readiness (TR), innovation adoption
Citation: Li W, Song R and Yu K (2025) Consumer adoption of food blockchain traceability: insights from integrating TAM and TR models. Front. Sustain. Food Syst. 9:1515188. doi: 10.3389/fsufs.2025.1515188
Received: 22 October 2024; Accepted: 12 March 2025;
Published: 26 March 2025.
Edited by:
Siphe Zantsi, Agricultural Research Council of South Africa (ARC-SA), South AfricaReviewed by:
Zlati Monica Laura, Dunarea de Jos University, RomaniaCopyright © 2025 Li, Song and Yu. This is an open-access article distributed under the terms of the Creative Commons Attribution License (CC BY). The use, distribution or reproduction in other forums is permitted, provided the original author(s) and the copyright owner(s) are credited and that the original publication in this journal is cited, in accordance with accepted academic practice. No use, distribution or reproduction is permitted which does not comply with these terms.
*Correspondence: Wei Li, bGVld2VpQHN6bnBpcy5jb20=
Disclaimer: All claims expressed in this article are solely those of the authors and do not necessarily represent those of their affiliated organizations, or those of the publisher, the editors and the reviewers. Any product that may be evaluated in this article or claim that may be made by its manufacturer is not guaranteed or endorsed by the publisher.
Research integrity at Frontiers
Learn more about the work of our research integrity team to safeguard the quality of each article we publish.