- 1LaMOS Research Unit, Faculty of Technology, University of Bejaia, Bejaia, Algeria
- 2LaMOS Research Unit, Faculty of Exact Sciences, University of Bejaia, Bejaia, Algeria
- 3Department of Bioscience and Technology for Food, Agriculture and Environment, University of Teramo, Teramo, Italy
- 4INRAE, AgroParisTech, Paris-Saclay Applied Economics, Paris-Saclay University, Palaiseau, France
Today, there is a significant “digital divide” in the agricultural sector between developing and developed countries. Such a digital disparity has negative consequences on the international competitiveness of these countries and their ability to comply with Food Satefy Standards. We propose a theoretical model to analyze the role of smart agriculture in the ability of countries to comply with international food safety regulations, specifically the Maximum Residue Limits (MRLs) for contaminants such as aflatoxins, pesticides,and heavy metals. Firstly, we show that reducing the digital divide will always improve international food safety and food security (availability of a global supply). However, it can lead to more intense international competition, potentially causing a perverse effect: underinvestment in good agricultural practices by more digitally advanced countries. Furthermore, the digital catch-up of less advanced countries cannot sufficiently reduce health risks in international markets unless accompanied by strengthening official food control systems. Finally, we show that such digital catch-up encourages lowering MRLs rather than relaxing them, contrary to what producers or authorities might hope.
1 Introduction
The introduction of Information Technologies and IoT (Internet of Things) tools in agriculture is revolutionizing production methods and the performance of food systems. Colossal progress is being made not only in improving land yields and the productivity of agricultural workers, but also in the precision of soil treatment and pest control practices (Farooq et al., 2020). The development of smart agriculture1 is likely to facilitate the advent of sustainable and rational agriculture, midway between increasingly criticized intensive agriculture and organic farming, which struggles to establish itself sustainably due to production constraints, low yields, and difficulties in access to consumption by consumers with low revenues (Vishnoi and Goel, 2024; Jararweh et al., 2023; De Gennaro and Forleo, 2019). The use of ICT (Information and Communications Technology) and connected objects is becoming tools for rationalizing agricultural practices, contributing not only to cost savings and yield increases, but also to better results in environmental protection and plant protection against pests (Rejeb et al., 2022; El Bilali and Allahyari, 2018). Advanced technologies such as sensors, drones, and satellite monitoring systems can indeed significantly contribute to the sanitary and phytosanitary quality of agricultural products. They particularly help in the early detection of plant diseases and pest infestations. By enabling rapid intervention, they reduce the use of pesticides (Zhang and Kovacs, 2012; Paraforos et al., 2016) and make it possible to apply precise quantity of necessary fertilizers and phytosanitary products. This prevents overuse and contamination of products (Gebbers and Adamchuk, 2010). Moreover, the increase in production yields enabled by the use of digital tools can also, indirectly, promote the sanitary and phytosanitary quality of products. Fabregas et al. (2019) indeed show how increased yields reduce the need for intensive use of inputs and ultimately create incentives to better comply with phytosanitary legislation.
Pest and pest control is regulated by national, regional, and multilateral legislations, such as European regulations, and within the framework of sanitary and phytosanitary agreements (SPS), where reference standards and guides to good agricultural practices are proposed (see Hammoudi et al., 2009). Since the health incidents of the 90s (mad cow disease crisis, dioxin chicken, melamine milk…), access to international markets has increasingly been conditioned by the obligation to comply with numerous and increasingly demanding public norms and private standards (Hammoudi et al., 2015). At the European level, food safety systems rely partly on the imposition of maximum residue limits (MRL) for biological or chemical residues. This regulatory tool specifies the maximum allowable thresholds of contaminants in a product, such as aflatoxin, dioxin, heavy metals, etc.2 All regulations like MRL aim to reduce consumption risks related to food and protect consumer health.3 However, these thresholds imply obligations of results: producers must determine the means to be deployed in farms to ensure that their products comply with the imposed thresholds.4 Producers must determine by themselves the appropriate good practices or rely on the guides to good practices recommended by regional or multilateral organizations (WHO, FAO, European Union).
The implementation of such means is generally quite costly. However, since the introduction of digitization, farmers have a valuable tool to manage health risks in addition to or as a substitute for on-site production means. Digitization has indeed become a particularly effective facilitator in the implementation of good agricultural practices while providing unprecedented precision in risk assessment in farms. Connected objects (and more generally, IoT), an example of a booming technology, play an important role in improving farm efficiency, reducing losses, and preventing contamination risks, thus ensuring better sanitary quality of finished products (Huo et al., 2024). Specifically, environmental sensors,5 smart irrigation systems, cameras and drones, and portable analyzers6 represent a valuable asset for achieving both food safety objectives (increased yields and availability of supply) and food safety objectives (Morchid et al., 2024).
Having these tools can undoubtedly be an asset for better competitiveness of producers. It can also constitute, in an open economy context where compliance with health regulations becomes a condition for market access, an additional means for producers to improve their control of food risk (see for example Dabbene et al., 2014; Melo et al., 2014). However, today, there is a real “digital divide” between less developed and developed countries (see for example Acılar, 2011; Hennessy et al., 2016). Such a disparity in digital resources and know-how can have negative consequences on the competitiveness of these countries at the international level, further widening the existing gap between the “North” and the “South” in this area. Moreover, is not this disparity likely to thwart the hopes placed on digital agriculture by institutional and professional actors, agriculture considered as a lever for drastically improving food risk control?
There are relatively few studies that provide economic analyses on the impact of smart agriculture on international competition.7 An important branch of literature has focused on presenting IoT innovations by demonstrating their utility through experiments or case studies in various agricultural fields.8 Senyolo et al. (2018) demonstrate how the adoption of smart agricultural technologies is crucial for improving sustainability as well as international competitiveness. The work of Dragomir et al. (2019) explores how the Common Agricultural Policy (CAP) of the European Union for 2021–2027 promotes the adoption of smart agriculture to enhance the competitiveness of the European agricultural sector. Drawing from successful implementation examples of smart agricultural solutions, such as in Slovakia, Dragomir et al. (2019) highlight the importance of transnational cooperation in this field for knowledge transfer and technological innovation. A significant number of studies have focused on highlighting the potentially positive link between the use of digital tools and the increase in agricultural yields and labor productivity (Swinton and Lowenberg-DeBoer, 1998; Griffin et al., 2005; Schimmelpfennig, 2016; Bullock et al., 2020; Schimmelpfennig and Ebel, 2016).
Another branch of literature, more critical, has focused on highlighting the risks associated with the digitalization of agriculture, including: (i) risks related to the sociological and cultural aspects of digital transformation, such as more precarious agricultural work resulting from the development of robotics and other IoT tools (Burton and Riley, 2018; Miles et al., 2019; Rotz et al., 2019), (ii) risks related to the emergence of inequalities and new dominant (technological) powers in the supply chains and an inequitable distribution of benefits resulting from the use of digital technologies, (iii) risks related to the exclusion of producers from the activity due to the small size of their farms or their lack of skills (see the literature review proposed by Rejeb et al., 2022).
There are no analytical, theoretical or quantitative studies that have explicitly addressed the role of the digital transition of agriculture and its relation to producers’ behaviors and health food risk, particularly in the context of agri-food competition.9 To fill this gap, we propose a theoretical analysis to answer some important questions: how does digital capability affect food quality, the level of available supply, and therefore the market price? What is the role of international heterogeneity of digital capability in agriculture on the safety of the international food market? Our model considers competition between producers from various countries characterized by heterogeneous digital capabilities. This heterogeneity indeed leads to unequal capacities to comply with food safety regulations.
The model builds upon several theoretical works that formalize price formation and sanitary risk on markets by leveraging tools from industrial economics, particularly within the framework of interactions between producers and public authorities10 (Hammoudi et al., 2009; Nait Mohand et al., 2017). Our model extends the work of Nait Mohand et al. (2017). We introduce three elements of differentiation: an international trade context, the incorporation of smart agriculture into the model, and heterogeneity among countries in digital capabilities.
We show that under the assumption of a competitive international market, it is not systematic that a digital catch-up by less advanced countries leads to better safety of products circulating in the market. This somewhat counter-intuitive result can be nuanced based on a key variable that, as we show, plays an important role in the level of risk obtained after a digital catch-up by less advanced countries. It concerns the food control systems that authorities put in place to verify the conformity of marketed products to regulatory MRLs.
Specifically, while narrowing the digital divide will certainly lead to an improvement in food safety and, in parallel, food security (availability of global supply), it induces more severe international competition which, in the end, can lead to a perverse effect consisting of under-investment in production means specifically dedicated to the quality of production practices by the most digitally advanced countries.11 This under-investment, results in a paradoxical outcome: a greater share of contaminated products exceeding regulatory MRL thresholds will come from these advanced countries. This counter-intuitive result at first glance is mainly explained by the market competitiveness hypothesis, the response of producers from advanced countries to increased competition and price evolution, making the physical means invested on-site and dedicated to quality practices become adjustment variables in the face of intense competition.
We show that the quality of official food controls can be used by authorities to correct this undesirable effect associated with the positive evolution of digital capabilities. In particular, if health risk always decreases with the improvement of digital capabilities, unreliable controls can reduce the expected health benefits of improving these capabilities. More specifically, health risk decreases less strongly in the case of unreliable controls than in the case of reliable controls. Moreover, unreliable control does not allow producers, regardless of their country of origin, to benefit from the improvement of digital capabilities (the profit of all types of producers decreases with the digital catch-up of less advanced countries).
Finally, another very important question is addressed in the last part of this study: can the emergence of smart agriculture eventually allow a relaxation of MRLs? This question is at the intersection of two contradictory societal demands: a demand from European producers who generally find that health quality standards are too strict and a demand from consumers and their representatives who find it important, to better protect consumer health, to reinforce standards and in particular, to lower MRLs. Our model shows that a positive evolution of digitization through a catch-up by less advanced countries is not sufficient to allow a relaxation of MRLs unless one is willing to accommodate a higher health risk in markets. On the contrary, we highlight the need to accompany any dynamic of digital progress by reinforcing MRL thresholds.
The rest of the article is organized as follows. In section 2, we present the model. In section 3, we analyze the effects of an international catch-up on health risk (food sanitary quality) and on available market quantities (food security in the quantitative sense). In section 4, we focus on the effects of digital agriculture progress on the income evolution of trade partners (profits of producers from less advanced countries and profits of producers from digitally advanced countries). We conclude the analysis with a conclusion.
2 Model
We consider an international market for a food product represented by a demand given by:
The parameter , ( ) represents the size of the market, and is the market price, which is determined by equalizing supply and demand. The inverse demand is , where is the ultimate potential supply. We assume, for all the rest of the paper, that .
We assume also that the production system is composed of ( ) countries and each country is represented by a producer. All producers have the same production capacity or farm size The maximum supply that the international production system can offer is therefore . The countries (and then producers) are assumed to be heterogeneous in the development of digital tools for agriculture. It is more simply assumed that there are two categories of countries or producers: an number of countries with a Lowly Digital Capabilities (LDC) and a second category of countries with a High Digital Capabilities (HDC)12 (see Figure 1). For convenience, we will take in all the following pair. We assume that producers export to an integrated market representative of a global spot market or a representative importing region that we will call simply “importing region.”
First, we assume that the product sold by producers must comply with a safety regulation based on an obligation of results (Starbird, 2005; Grazia et al., 2012). More specifically, the import country’s supranational authority control the sanitary or phytosanitary risk through the imposition of a permitted maximum threshold ( of a microbiological or microchemical contaminant in each product unit (eg, an aflatoxin or pesticide residue). Random official health inspections are carried out at the importing region’s borders before the product enters the market and is sold to the consumer to ensure that the products comply with the regulation. It is assumed that the control system is flawed in the sense that contaminated products may not be detected and are sold on the market. A contaminated sample is identified as contaminated and therefore rejected after inspection with probability , ( The imperfection of the control system may be attributable in practice to multiple causes, such as the lack of human and material resources allocated to the control posts (number of inspectors, analytical laboratories, etc.), but also, for example, to considerations related to the training of controllers or the operations management on-site.13 Producers observe the threshold but must determine the investment in means and Good Agricultural Practices (GAP) that they must agree on production site for their products to meet these regulations. The upstream/downstream production and marketing structure is described in Figure 1.
We denote , the level of investment in the quality of the production practices of a producer and , , the total cost of bringing the farm into compliance:
is the unit cost of production ( ).
Each producer first observes the standard imposed by the importing region and chooses the optimal amount of investment in good practices on its production site.
It is assumed that country, with high digital capability, can increase a producer’s ability to comply with the standard by increasing the “yield” of the investment in good production practices (process). Formally, we will note , with the digital capability of a producer of the country .
When the indicator increases, the digital capability increases (the lowest level being 0 and the maximum level 1). We assume simply that the producers with LDC have a digital capability 1 and the producers with HDC have a maximum digital capability equal to 1. When a producer invests to comply with the observed standard , it is assumed that his investment is less efficient as his digital capability is low.14 At fixed , we assume that the producer integrates the level of efficiency (or inefficiency) due to his digital capability in order to anticipate the probability that his product will pass the inspection. More precisely, it is supposed that he can evaluate the probability that a unit of its product conforms to the norm when the official controls are perfect and this probability is expressed through a function increasing in , and .
In addition, since control is imperfect, producers can hope that some non-compliant production will not be detected by inspection. Let be the probability that a unit of good produced with practice is declared compliant by the border control system. The function is deduced easily from the function (see Expression 8):
Each producer , can anticipate, (i) the individual quantities, noted , that “pass” the inspection (or declared compliant), (ii) those who pass the inspection and are actually conform ( , (iii) those that will be rejected because they do not conform ( ) and; (iv) those that will be declared compliant while they are contaminated ( . These quantities, or more precisely expected quantities, are expressed as follows:
It is assumed that these rejected quantities generate costs: each unit of rejected product generates a marginal cost of rejection noted for the incriminated producer.
Throughout the rest of the paper, we will specify the function as follows:
This function is increasing in , and . In addition, it verifies some other more specific properties15 that are not relevant from the point of view of the qualitative results of the model.
We define the game as follow: at the first stage, the supranational authority of the importing region decides a threshold (MRL) and a level of food control . At the second step, the producers observe the decisions made at the first step, the market price in force in the spot market and choose simultaneously their investment level on GAP. At the third step, the producers sell their produced quantities in the importing region. This quantity is controlled before marketing in the region. The “contaminated” quantity (whose contamination threshold exceeds threshold ) is rejected and the rest is sold on the market. The law of supply and demand determines the equilibrium price in the country that ultimately defines the actual levels of investment and the profits of the producers.
We start from the fact that the definition of a level of controls and MRL thresholds by a supranational authority of regional or international type (European Commission, FAO/WTO for example) aims above all to reduce risk. However, it cannot completely ignore the economic consequences (profits of commercial partners, prices and supply available for consumption, i.e., consumer surplus in the region concerned).16 We will assume in all the following that the supranational authority has to ensure food safety in the spot market, but it has also to consider the evolution of producers (their profits), the interests of consumers that is say the “economic” consumers ‘surplus according to the total quantity consumed (the food supply or food security criteria). In other words, the supranational authority must ensure a reduction in health risk on the global market while guaranteeing a reasonable level of incentives to produce and to participate to international trade.
We assume that the health risk on the global market comes essentially from the contaminated quantities that circulate in the market and are consumed by consumers. It will simply be defined as a “contamination rate,” the ratio of the total contaminated quantity and, the total quantity ( ) offered and consumed on the importing region. We note this ratio .
The total offer and the health risk in the global spot market are given, respectively, by:
In the following sections, we analyze the impact of the digital transformation of agriculture on the food safety of products marketed on international markets, considering the heterogeneity of countries in terms of digital capability. For this, we will determine the outcome of competition on the final market and evaluate on the one hand, the role of this heterogeneity in the formation of prices and on the other hand, the evolution of good agricultural practices at the resulting from the introduction of digital tools.
3 Digital capabilities, food safety and supply availability
We assume that the maximum authorized threshold , and the official control of reliability level are given (first step of the game defined previously). The authority gives producers of exporting countries the freedom to determine their investments in good practices.
For fixed and , and for an observed price , each producer determines in the second step of the game, the level of investment that maximizes its profit (see Expression 12):
Given the Expressions 2, 4, 6, the profit of producer is given by:
The resolution of the program given in Expression 11 reveals that, the first and the second order conditions being verified, the optimal investment levels of producers with LDC (noted ) and producers with HDC (noted ) verify:
We can observe that, knowing that , .
Given the Expressions 1, 9, 13, the market price that emerges is the solution of the equation given as follow:
Then the market price at equilibrium is given by:
Thus, the price on the spot market depends on the digital capability of the least developed countries. It can be easily shown that the price decreases with respect to the parameter : . Thus, the gradual introduction of digital tools into agriculture in the least developed countries will increase the international competition by gradually lowering market price and which will lead to an increasingly large available supply. However, the variation of the market price according to the level of control reliability is not monotonous. Indeed, we can easily verify that and such that if and only if and . Moreover, we can easily verify that and .
Thus, in a weak area of controls ( , when the quality of control improves, the equilibrium price increases. On the other hand, a sufficiently effective control system ( and a relatively high level of producer efficiency ( will generate a decrease in the equilibrium price. Such a decrease in price is mechanically induced by the increase of the total supply (quantities that pass inspection): . Such a positive evolution of the total supply is due to the improvement of the probability of individual compliance which is the result of the consequent increase in the investment of each type of producer ( ).
If we consider both the decrease in price in relation to and its decrease in relation to in the control zones [0, ] and [ , 1], we can draw an important lesson in relation to the evolution of international competition. Indeed, in a context of positive and progressive digital transformation of the least advanced countries, we can expect a gradual drop in prices but this drop will be (i) slowed down if it is accompanied by a gradual improvement of initially weak controls and (ii) will be accentuated if the controls are initially relatively effective.
In addition, the threshold is decreasing in ( ). This means that when digital progress is made by the least developed countries, the equilibrium price will decrease in a wider area of controls, in other words, including for less reliable controls.
By replacing the Expression 14 in the Relation 13, the equilibrium investment level of producers is given by17 and with:
Given the Expression 15, we can verify easily that:
Thus, knowing that and , the decrease of with respect to shows that the positive impact of digitalization on LDC producers outweighs the negative effect on HDC producers’ investments.
Proposition 1: When the control level improves, there exits such that the HDC producers’ investment increases if and only if
We can verify easily that: if and only if . The expression of is given in the Appendix.
Thus, at a given , an increasing of the level of controls in [0, increases the investment of producers with HDC (and those with LDC). Thus, strengthening controls may be a solution to mitigate the relative disinvestment of HDC producers following the development of the digital capabilities of LDC producers. After this control threshold , the control becomes relatively reliable, and producers’ investment decreases as it strengthens. Thus, beyond a certain threshold of control reliability, the incentives to comply with the MRL decrease because the cost of compliance increases while the market price drops due to an oversupply. Producers then begin to disinvest, increasingly relying on the relative imperfection of the control system to pass non-compliant quantities.
Starting from these variations of the equilibrium investments and from the variation of the health risk function defined in Equation 10, we can state the following result.
Proposition 2: When the digital capability of the producers with LDC improves, (i) the health risk decreases and the total available supply increases, and, (ii) the producers with HDC are responsible for a greater proportion of non-compliant quantities consumed on the market.
The proof of the proposition is obvious. Indeed, we can easily verify that:
i. and ,
ii. and .
The first result stated in the proposition stipulates that the objectives of risk reduction and availability of supply (food safety and food security) are compatible when we move from a less digitalized agriculture to a more digitalized agriculture. The progress made in the LDC country in this area leads to more food safety and more food security (in the sense of availability of supply). However, in the total supply, which increases when we increase the level of digitalization, there is a proportion of contaminated products exceeding the threshold , which is more attributable to producers from HDC countries (assertion ii) due to “mechanical” disinvestment arising from market incentives resulting from such an evolution of the digital capabilities of LDC producers.
When the digital capability of producers improves, the increase (respectively the decrease) in the quantities offered (respectively the contaminated quantities) by the producers with LDC is greater than the decrease (respectively the increase) in the quantities of producers with HDC. Producers (with LDC), who benefit from the digital capability building, will increase their level of investment. Thus, the compliance probability increases ( and ) and leads to the increase of their offer and the reduction of contaminated quantities.
The interesting results (i) and (ii) of Proposition 2 highlights a “perverse” effect due to international competition: As the level of producers with LDC improves, these producers increase their efforts in production practices by betting on a larger portion of compliant quantities passing the inspection. When the digital capability of the producers with LDC improves, producers with HDC respond by underinvesting in GAP. This result comes from the evolution of the equilibrium market price under the hypothesis that producers are price takers on the spot market. When the differential of the digital capability of the two types of producers decreases the equilibrium price decreases due to the increase in total supply, producers with HDC will be encouraged to seek better cost competitiveness by lowering their investment in good practice. As the digital capabilities of countries become more homogeneous with the digital catching up of the least developed countries, we will observe an improvement in the good practices of these countries but a relative disinvestment of the most advanced countries. When digital tools develop in the least developed countries, investments in good practices at the international level will tend to gradually become closer with more investment in the least developed countries and less in the most advanced countries. This effect, mainly due to the hypothesis of a competitive international market, has no impact on the evolution of health risk (which decreases with the digitalization of agriculture) but (because of the investments of HDC producers) does not lead to a reduction in risk in proportion to the progress of digitalization.
Such an effect could be seen as a factor slowing down to a certain extent the benefit that could be expected from the digitalization of agriculture in terms of food safety. How can supranational authorities correct such an effect? The following corollary shows how control can be a strategic tool for achieving such an objective.
The Figures 2 illustrate the results of the Proposition 2. The first graphic Figure 2A shows that the available supply does not always decrease with respect to control. The initial intuition, which suggests that strengthening controls should systematically lead to a decrease in quantities traded, is contradicted by this result. This initial intuition would be validated if there were no strategic response from producers to the evolution of controls through adjustments in on-site production methods. When the efficiency level of the control system is relatively low and improves below , producers’ investment are not sufficiently high and the quantities detected non-compliant by the control system increases. Thus, the total supply decreases, the price increases and consequently, the consumer surplus decreases. When the control is more reliable and improves above , the efforts in good production practices are significant enough to, sufficiently, reduce the quantities rejected. Thus, the individual supply and the total supply increase and finally, the consumer surplus increases despite the price drop.
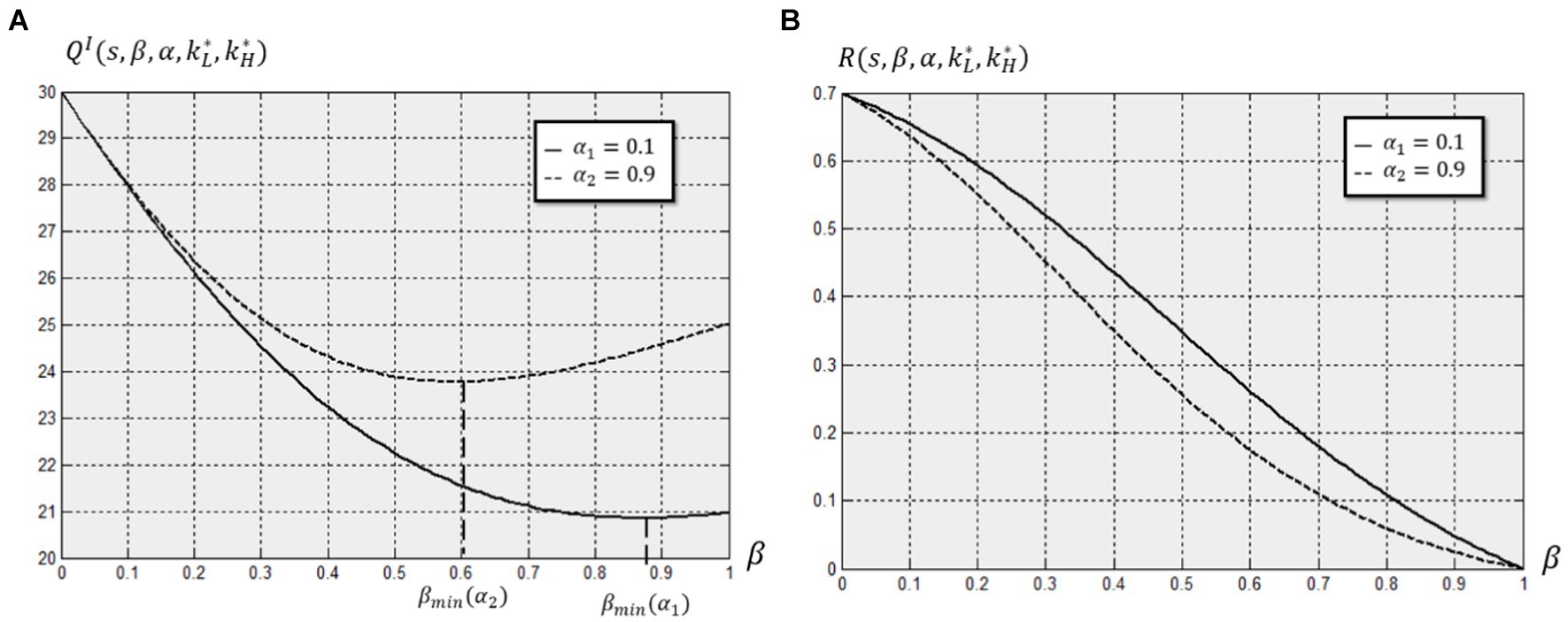
Figure 2. Variation of available supply (Curves A) and health risk (Curves B) in relation to the control and the digital capability of producers.
We have previously highlighted how an increasing digitalization of agriculture in the least developed countries can generate a reduction in health risk on international markets. However, we have also shown that progress on food safety may be less than expected due to the insufficient effectiveness of official controls.
In the next section, we will analyze the collateral economic effects associated with a risk reduction policy, particularly on the profits of trading partners. In particular, we will assess whether the increase in digital capacity in the least developed countries benefits producers, especially those belonging to these countries. This indirectly amounts to measuring whether there are incentives for countries that are initially less advanced in the digitalization of agriculture to engage in a dynamic of development of this digital tool. The progress of digitalization reduces the health risk even if such a decrease would benefit from being reinforced by an improvement in controls, do the profits of the actors go in the same direction?
4 Digital capabilities and trading partner profits
The supranational authority must reduce the health risk while preferably not reducing the quantity of food supply available for consumption as well as the income of participants in international trade.
The variation in profits of the two types of producers is given in the following proposition.
Proposition 3: When the digital capability of the producers with LDC improves, the profit of producers with HDC decreases whatever the control level and the profit of producers with LDC increases if and only if , and .
The proof of the proposition is obvious and is obtained simply from the derivative of profits.
and 0,1[such that if and only if , and .
The expression of , and are given in the Appendix.
Thus, the profit of producers with LDC can only grow with the development of digitalization if, first of all, the progress of digitalization is relatively moderate [with a maximum level . Furthermore, it is necessary (and sufficient) that the total potential supply does not exceed and finally that the control is sufficiently reliable (beyond the level ). It must, therefore, be deduced from the Proposition 3 that an advance in digitalization in the least advanced countries can possibly benefit producers in these countries if official controls in the region of import are sufficiently reliable and digitization progress is not too significant.
Let us stop at this last condition. For LDC producers to see digitalization benefit them in terms of international competition, it is therefore necessary that the progress made in the digitalization of agriculture is within an “eligible” range. [0, ] (under the assumption ). We can verify that all things being equal, is decreasing in [ see Appendix]. This means that reinforced control tends to restrict the possibility that LDC producers can benefit from the digital development of their agriculture (the eligible interval [0, ] becomes smaller).
Corollary 1: When the digital capability of producers with LDC improves and the control is insufficiently reliable the equilibrium profit of all producers always decreases.
Thus, too great an imperfection of controls in the importing region can prevent producers with LDC producers in the least developed countries from benefiting from the improvement of their digital capacities.
The strategic behaviors of both types of producers and their effects on competition explain these results. When the level of digital capability of the producers with LDC improves in a zone of low control levels ( ), producers with HDC under-invest in quality practices and as the level of control is low, the quantities offered passing the inspection increases ( ). However, the price falls, the investment effort of the producers with LDC increases ( ), their cost of compliance also ( ) and therefore their profit decreases. Moreover, since the underinvestment of producers with HDC is less important compared to the improvement of the digital capability , their cost of compliance is not compensated, which reduces their profit.
The proposition gives us an interesting lesson with regard to the issue of fairness in North–South trade relations. Catching up in know-how or digital capability of the less developed exporting countries may not benefit them, especially when the importing region in the North does not improve the reliability of its controls. Indeed, when the digital capability goes from to ( ) and the level of control is relatively low (zone 1), the producers will disinvest by betting on the imperfection of the controls to pass their products. This strategy is rational and makes it possible not to deteriorate their profit due to the fall in the market price. The drop-in price is obviously due to the passage of a relatively large quantity of products (induced by an improvement in the country’s digital capability).
In a relatively high control zone ( ) (zone 2), the improvement in the digital capability of producers with LDC induces an increase in their supply ( ) and a decrease in the supply of their competitors ( ). Thus, the producers with HDC under-invest in quality of production practices. Since control is relatively efficient, the total supply tends to decrease ( ) leading to an increase in the market price. The profit of producers with LDC increases and that of producers with HDC decreases. In other words, the restoration of a certain fairness in trade via the improvement of the digital capability of producers with LDC (from to ) will only be effective if at least the level of control is relatively reliable (zone 2). Since the efficiency level of control, is high, producers with HDC will to improve their level of investment in production practices even if the market price decreases. This will increase the supply (consistent quantities) and reduce the rejects (see Figures 2, 3).18 It can be noted (Figures 2, 3) that on the one hand, there are levels of control, which ensure the maximum profit for the producers, and on the other hand, these levels differ according to whether the producers have low digital capability or high digital capability. The existence of the strict concavity of the profit functions is due to the combination of two factors: (i) the effect of the increase of the control reliability on the price (which increases up to a certain level and then decreases) and, (ii) its effect on the compliance cost (which increases with the need to invest in good practices). The initial increase in price with control reliability comes from contraction of supply due to increased releases. Its decrease in a second time comes from better investments of the producers, which make decrease the rejects and increase the marketed total offer.
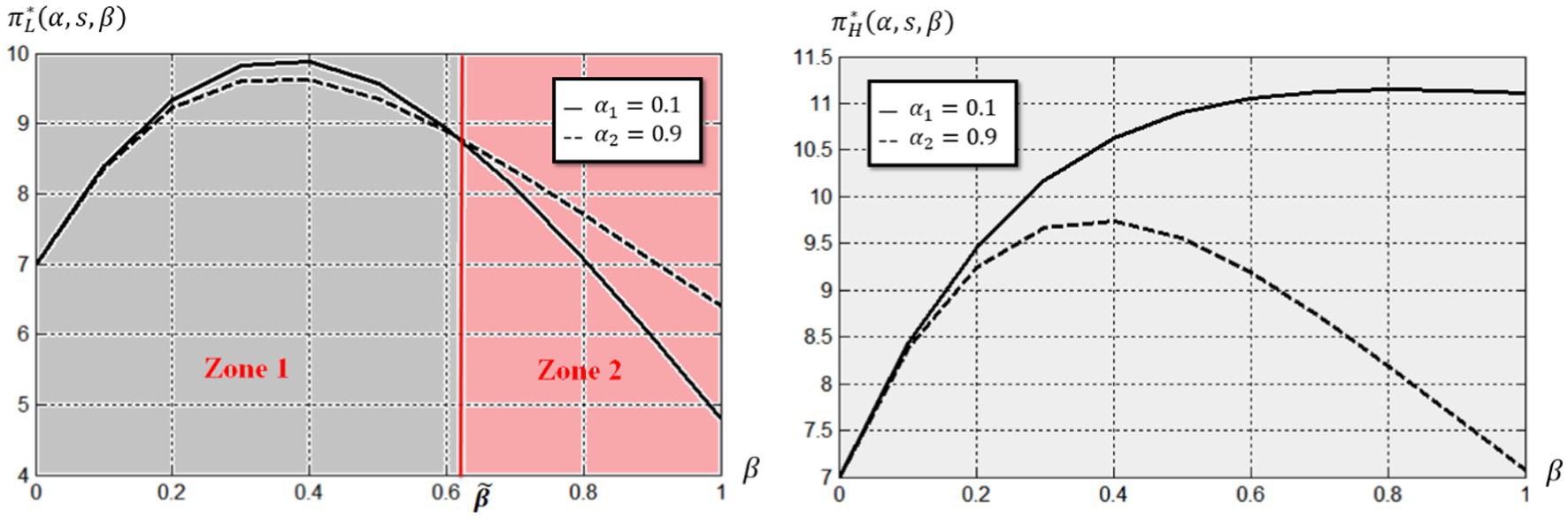
Figure 3. Variation of the profit of producers with LDC and HDC according to control and digital capability of producers with LDC.
5 MRLs as a tool for risk reduction and economic regulation
The MRL thresholds are set gradually as scientific advances concerning the toxicity of biological and chemical contaminants contained in foods consumed by consumers. Alongside this strictly health motivation, setting a threshold is not neutral from the point of view of strictly economic effects. The previous sections show that the level of economic variables resulting from international competition (price, available supply, profits, consumer surplus) are a function of the level of the MRLs chosen by the supranational authority. A multi-criteria approach to health regulation requires reconciling, when possible, health objectives and economic objectives.
In this section, we will ask ourselves the following question: Can the development of digital agriculture facilitate such a multi-criteria approach? In particular, can the development of such agriculture allow a relaxation of MRLs without compromising the objective of reducing health risk and availability of supply?19
In other words, what will interest us from the point of view of the health benefit of consumers is to know if the level of health risk can be maintained or reduced when we move from an initial situation to a situation with < .
Consider , The risk resulting from quantities contaminated with respect to , that is to say, exceeding residue level, when the threshold is in effect (the official threshold set by the authority).
At a fixed and , we will consider that the health of consumers is not deteriorated when we go from the maximum authorized threshold to maximum authorized threshold if these two conditions are verified:
i. The quantities in circulation on the market exceeding the threshold when is the authorized threshold are lower than the quantities exceeding the threshold when the authorized threshold is
ii. The quantities in circulation on the market exceeding the threshold when is the authorized threshold are lower than the quantities exceeding the threshold when the authorized threshold is
Formally, these two conditions can be written as follows:
To carry out the analysis, we will rely on curves with respect to , drawn with different values20 of , , with , .
Figures 4A,B respectively represent the health risk curve for relatively low control and for relatively reliable control. Figures 5A,B represent the curves of the quantities available for consumption for relatively low control, and for relatively reliable control.
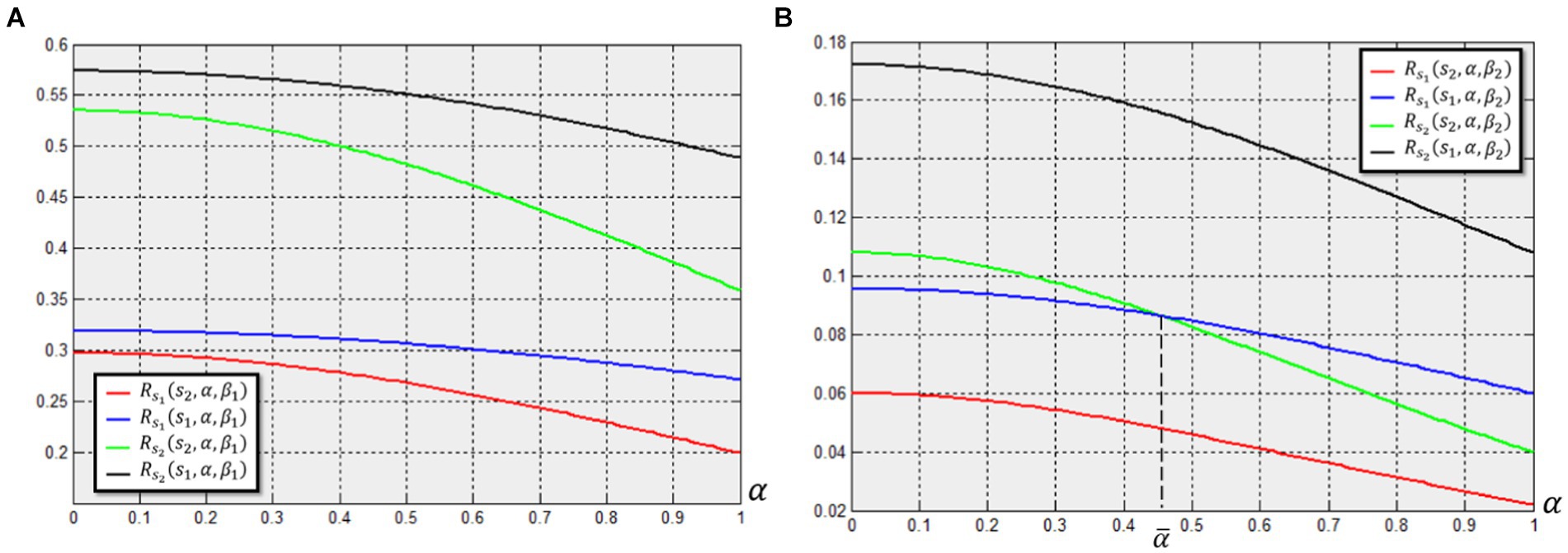
Figure 4. Variation of the health risk according to the MRL level and digital capability of producers with LDC when the effectiveness of control improves from β1 (Curves A) to β2 (Curves B).
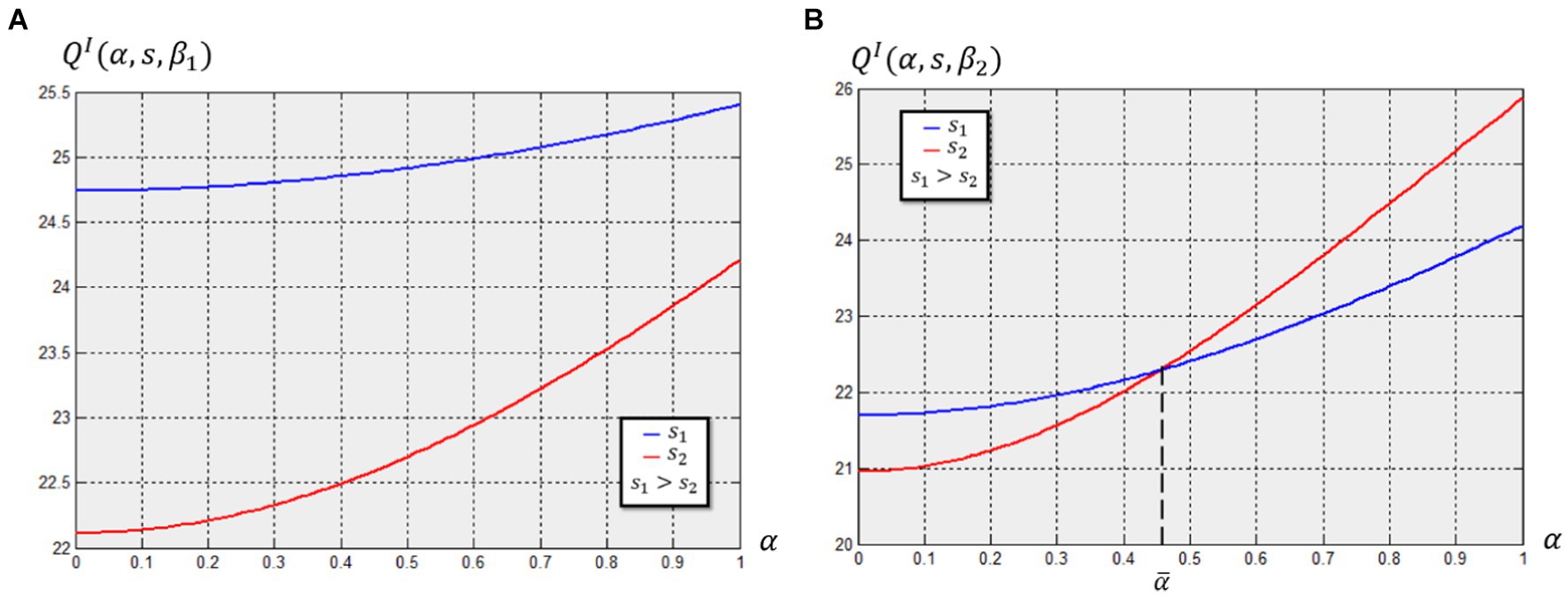
Figure 5. Variation of the available supply according to the MRL level and digital capability of producers with LDC when the effectiveness of control improves from β1 (Curves A) to β2 (Curves B).
It immediately follows from the observation of Figures 4A,B that any digital catch-up does not allow the risk to be kept unchanged by relaxing the constraint on the threshold, i.e., by increasing the MRL threshold.21 In other words, when the digital capability of LDC producers increases, the relaxation of the thresholds cannot be considered unless the health risk increases (Conditions C1, C2 will not be verified).
On the other hand, in order to reduce the risk in the context of a positive development of digital capabilities, it is necessary to lower the current MRL threshold. The observation of the risk (Figure 4A) and supply curves available on the market (Figure 5A) under the assumption of relatively weak control (unchanged with the evolution of digital capabilities), then allows us to state the following proposition.
Proposition 4: In order to reduce the health risk in a context of a positive evolution of digital capabilities, it is necessary to lower the MRL threshold. When control is relatively weak, it is necessary to accommodate a decreasing available offer as digital capabilities increase. When the control is relatively reliable, the available supply only increases with the strengthening of the MRL if the digital progress is sufficiently significant.
At this stage, we can notice, by observing the variations in risks on the curve (Figure 4A), that . In other words, the quantities contaminated with respect to when is officially in force (and strengthened because it is more demanding than ) are greater than the quantities contaminated with respect to when is in force (initial situation before the MRL was strengthened). This result is therefore not sufficient to conclude that it is not advisable to strengthen the MRLs. Only criteria Conditions C1, C2 can be considered for such a recommendation.22
Figures 6A,B respectively represent the profit of producers with LDC curve for relatively low control and for relatively reliable control. Figures 6C,D represent the curves of the profit of producers with HDC for relatively low control, and for relatively reliable control.
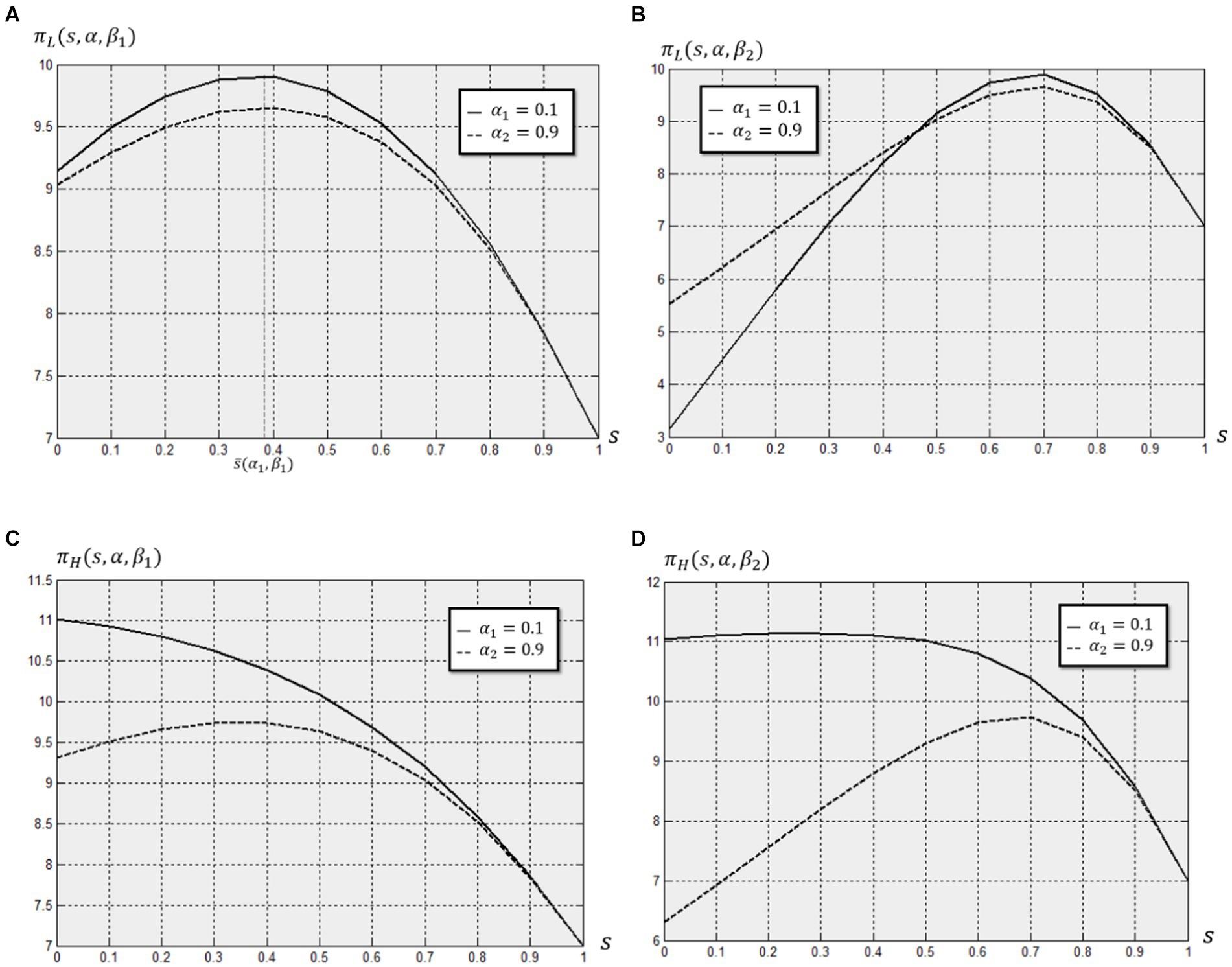
Figure 6. Variation of profit of producers with LDC (respectively producers with HDC) according to the MRL and the level of digital capability of producers with LDC when the effectiveness of control improves from β1 (Curves A) (respectively Curves C) to β2 (Curves B) (respectively Curves D).
The profit graphs of producers in Figure 6 illustrate that, for a given level of digital capability of producers with LDC and control reliability, there exists an MRL threshold ( ), that the importing country authority could consider imposing, thus maximizing producers’ profits. This threshold increases as the level of digital capabilities improves and/or the control efficiency increases. Indeed, a relaxation of the MRL threshold below ( ) leads to a reasonable increase in supply, which will contribute to improving producers’ profits. However, if the MRL threshold is relaxed above ( ), it will lead to larger quantities passing through border controls, thereby reducing market prices. As a result, these producers will see a decrease in their profits.
Figures 6A,B show that the improvement of digital capabilities of producers with LDC is beneficial to them in terms of profit only if the level of Maximum Residue Limits (MRL) is sufficiently strengthened accompanied by reliable control.
6 Conclusion
Few empirical or theoretical economic studies have genuinely examined the effects of smart agriculture on the evolution of international competition and the role it can play in achieving food security (in the quantitative sense) and food safety objectives. Yet, digitization in agriculture can change the structure of trade and international competition through two levers: i) a greater capacity of certain nations to produce more quantities due to a significant reduction in costs and better management of farms, and ii) a greater capacity to produce higher quality goods thanks to precision agriculture, which allows continuous monitoring of the production process and provides plants with solutions to problems that hinder their qualitative development. Cameras and drones are technologies that, for example, can monitor fields and agricultural facilities and detect pest infestations or plant diseases before they spread. By enabling quick and targeted intervention, they reduce the use of pesticides and improve food safety. Smart irrigation systems, which are soil moisture sensors that automatically trigger an irrigation system adjusting the water supply according to the actual needs of the plants, help avoid over-watering and under-watering, which can affect plant health and food safety.
The emergence of so-called smart agriculture can, therefore, be considered a valuable ally for both producers and public authorities concerned with reducing foodborne health risks. However, these optimistic predictions could be thwarted by the existence of a digital divide between different nations participating in international trade. Such a divide can exacerbate or even create a competitive imbalance and inequality in access to international markets between developed countries (the North) and developing countries (the South). Moreover, these disparities between operators and nations, particularly in terms of capacity to meet international requirements, raise questions about equity and competitiveness in the global agri-food sector. Thus, it is crucial to assess the impacts of these inequalities and design appropriate regulations to ensure fair competition and maintain international food safety.
The reduction of the international digital divide represents a true challenge at the international, regional, and national levels. This requires the implementation of ambitious policies, such as the establishment of specific strategies to aid the digital development of the least developed countries. At the local level, policies must be tailored to the specificities of these countries with concrete measures aimed at promoting the adoption of digital technologies and initiating a catch-up dynamic for these countries/regions.23
However, digital catch-up is not a sufficient condition to achieve a satisfactory level of food security (available supply) or a satisfactory level of food safety for consumers. It is necessary to consider a “competition” effect that our model clearly highlights. If the health benefits for consumers in developed importing countries indeed improve thanks to the technological advances of the less advanced exporting countries and their better control of health risks, it can improve even further when the food controls of the developed importing countries are strengthened and made more reliable (Proposition 2, Figures 2, 3). In other words, even if developed countries, in the name of the health of their own consumers, agreed to contribute to the digital upgrading of Southern countries, such a contribution would be suboptimal if it is not accompanied by an improvement in the food control systems. When Southern countries improve their digital capacities, lax control in the North leads to a relative under-investment in “physical” means by Northern producers who are otherwise well-equipped with digital resources. Thus, these lessons suggest that Northern countries must, in the name of their consumers’ health but also in the interest of fair North–South relations (see Proposition 3), couple any potential policy of aid for digital development in favor of Southern countries with a policy of strengthening official controls in the North.
Furthermore, it should be noted that at the European level, strengthened Maximum Residue Limits (MRLs) are regularly implemented for certain substances deemed hazardous to consumer health.24 Our model suggests that within the framework of decreasing MRL thresholds, health risks are further decreased if the digital divide is reduced (Proposition 4). However, the supply is less significant with unreliable control. In other words, to reconcile the quantitative objective (growth of available supply) and its quality (risk reduction) within the framework of a simultaneous reduction trajectory of MRLs and the digital divide, authorities must accompany this trajectory with a dynamic of strengthening controls.
These results lead to some preliminary reflections on a scheme currently under much discussion in French and European forums: the EcoPhyto scheme implemented in France in 2008. The EcoPhyto program primarily aims to reduce the use of phytosanitary products (reduce pesticide use by 50% by 2025) and to decrease their negative impacts on the environment and health. The government is particularly focusing on the gradual substitution by producers of chemical substances used in pest control with more natural products that are respectful of consumer health and the environment.25
The reduction of Maximum Residue Limits (MRLs) is not directly part of the objectives of this program. MRLs are set by European regulations, and their revision is based on scientific assessments and decisions at the European level. By imposing strict standards on pesticide residues in foodstuffs, the MRL legislation, governed by Regulation (EC) No 396/2005, complements to some extent the objectives of Écophyto by ensuring, in the end, that reductions in pesticide use result in an effective decrease in residues in food. Thus, the Écophyto program does not specifically aim to reduce MRLs, but its goals of reducing pesticide use and promoting sustainable agricultural practices can contribute to a decrease in pesticide residues in agricultural products. Theoretically, the quantitative objectives of the Eco-phyto plan and the constraints it imposes on production practices can be interpreted as an imposition of both results and means. Such a normative interpretation suggests that public authorities, through this plan, aim to accelerate the reduction of pesticides, ultimately requiring producers to invest even more resources in their production sites compared to what they invested in response to the official European MRLs.26
By increasing the producers’ ability to better manage risk, particularly through better anticipation of pest-related diseases and more targeted, timely, and precise treatment of infestations, AI could likely help producers better withstand the additional constraints and quantitative objectives set by the Eco Phyto plan. However, the question of the contribution of smart agriculture to the success and acceptability, particularly by operators, of the EcoPhyto mechanisms is yet an open research question that deserves to be explored: what could be the contribution of smart agriculture to the EcoPhyto plan? Could the development of digital agriculture help reduce the costs borne by producers and decrease the state’s support budget? Does the unequal access of producers to digital tools hinder the success of the plan, and is digital catch-up a guarantee of success? Should investments and state support for the plan include the digital transition of producers? Our model is not directly applicable to answering these questions but can, with some necessary adaptations, theoretically contribute to this type of inquiry.
Finally, one of the limitations of the model is that, in its current version, it cannot explain the strategic mechanism leading to the digital divide and its extent, which are assumed to be exogenous: the number of producers with low and high digital capacities and the extent of the divide are pre-determined. Endogenizing producers’ investment decisions in digital capacity would be an interesting extension, which would likely require the extension of the homogeneity hypothesis of producers to a heterogeneous context (producers differing in terms of financing capacity and/or farm size, for example). Such an extension would also allow for the integration of public authorities as actors who can act upstream to limit the digital divide by relying on policies that the model could theoretically test.
Data availability statement
The original contributions presented in the study are included in the article/Supplementary material, further inquiries can be directed to the corresponding author.
Author contributions
LM: Conceptualization, Investigation, Methodology, Writing – original draft. MR: Methodology, Supervision, Writing – review & editing. MP: Investigation, Writing – review & editing. AH: Conceptualization, Funding acquisition, Methodology, Supervision, Writing – review & editing.
Funding
The author(s) declare that financial support was received for the research, authorship, and/or publication of this article. This study has been conducted in the framework of 1) « VALUETEAM project ». funded through ARIMNet2 (2017). ARIMNet2 (ERA-NET) has received funding from the European Union’s Seventh Framework Programme for research, technological development, and demonstration under grant agreement No. 219262, and 2) ValueDate project (2018) funded by CREAD, Algeria.
Conflict of interest
The authors declare that the research was conducted in the absence of any commercial or financial relationships that could be construed as a potential conflict of interest.
Publisher’s note
All claims expressed in this article are solely those of the authors and do not necessarily represent those of their affiliated organizations, or those of the publisher, the editors and the reviewers. Any product that may be evaluated in this article, or claim that may be made by its manufacturer, is not guaranteed or endorsed by the publisher.
Footnotes
1. ^By smart agriculture or precision agriculture or digital agriculture, we refer to the modern type of agricultural management that relies on the use of sensors, geographic information systems (GIS), drones, agricultural robots, big data, artificial intelligence (AI), and the Internet of Things (IoT) to contribute to the production process or to collect, analyze, and interpret agronomic data in real time.
2. ^The Regulation (EC) No 1881/2006 (Council Regulation No 1881/2006) establishes Maximum Residue Levels (MRLs) for mycotoxins in foodstuffs. In addition to the regulatory aspect, programs defining official controls are designed to verify compliance with legislation on animal feed and foodstuffs (Regulation (EC) No 882/2004).
3. ^MRLs are based on toxicological assessments and exposure studies to protect consumers from the harmful effects of pesticide residues and other chemical substances. To comply with MRLs, farmers must follow Good Agricultural Practices (GAP) and use pesticides and other chemicals responsibly and in accordance with regulatory guidelines. This includes adhering to recommended doses, pre-harvest intervals, and appropriate application methods. MRLs help improve consumer confidence in agricultural products and meet the growing demand for healthy and high-quality food. Finally, harmonized MRLs between countries facilitate international trade in agricultural products by ensuring that the products meet the safety standards of different markets. This helps reduce trade barriers and promote economic exchanges.
4. ^Alongside public regulations enforced by authorities, voluntary private standards exist which impose on-site production measures through certifications (for private standards, see Giraud-Héraud et al., 2012).
5. ^Environmental sensors are devices that measure variables such as temperature, humidity, air quality, and soil composition. These data enable farmers to maintain optimal conditions for crop growth and animal health, thereby reducing the risk of diseases and contamination.
6. ^Portable analyzers are connected devices that can analyze the chemical composition of soils or agricultural products on-site at production facilities. They ensure that products, before being marketed, are free from contaminants and comply with food safety standards.
7. ^Many studies have focused on the role of smart agriculture in enhancing resilience to climate impacts through improved productivity and sustainability of agricultural practices – (see, for example, Ahmed et al. 2024).
8. ^This literature aims to demonstrate the effectiveness of these tools, particularly in reducing the use of pesticides and fertilizers, optimizing water usage, optimizing agricultural production processes, improving food security, enhancing the competitiveness of agricultural enterprises, and ensuring the sustainability of production practices. For a systematic review of this branch of literature, (see Rejeb et al. 2022).
9. ^The existing research has primarily focused on the impact of new control and traceability tools made available to authorities to facilitate transactions and reduce international risk (Razak et al., 2023).
10. ^The authors propose a theoretical industrial economic model that identifies the causal link that may exist between public food safety regulations, the expected price in domestic markets, and the rate of exclusion of local producers.
11. ^Disinvestment in good practices and infrastructure, for example, involves disproportionately focusing on the precision and automation of risk control operations while underinvesting in human resources, basic infrastructure, pesticide quality, etc.
12. ^These two categories vary in terms of their level of digital resource endowment, which enables them to fully leverage digital technologies in their agricultural practices. This includes, for example, endowments in farms with digital management tools and the level of training of agricultural workers in these tools, as well as the use of what is known as smart farming practices (see examples in introduction).
13. ^The imperfection of control is modeled in this way in the work of Starbird (2005) and Grazia et al. (2012). As underlined in these two works, the diagnostic errors are due to errors of sensitivity of specificity of the tests. In our work, we only consider the errors associated with the sensitivity of the tests to give a product to contaminate as uncontaminated (and not the other way around).
14. ^Information and Communication Technologies (ICTs) can interact effectively with more traditional and “physical” means deployed on-site to improve product quality, control risk at a lower cost. For example, information management systems that facilitate product traceability, stock management, and hygiene standards monitoring throughout the production chain can increase producers’ ability to comply with hygiene constraints set by regulations within the Hygiene Package. Automated cleaning and disinfection systems operate by ensuring automatic cleaning and disinfection processes, thereby reducing the risk of human contamination during production. Similarly, IoT sensors can monitor real-time temperature, humidity, and the presence of contaminants throughout the production process. The information provided to farm managers triggers preventive alerts when deemed necessary by the digital tool, prompting corrective actions to be taken. This ensures production adheres to hygiene standards and sanitary and phytosanitary constraints.
15. ^In the absence of investment effort the probability that a unit of good produced is in accordance with depends only on the level of the norm ( ). At a given level , if the norm is as demanding as possible ( ), the probability of complying with , depends only on the level of investment and the level of training ( ). The compliant proportion reaches its maximum level weighted by the logistic level and reaches 1 when the drilling of the producers is perfect ( ). When no norm exists, ( ), the probability naturally reaches its maximum ( ).
16. ^We cannot consider the classic criterion of social welfare as a decision criterion for the supranational authority for reasons of realism given the context of multilateral exchanges: the authority cannot consider the surplus consumers from exporting third countries. The criticisms generally made of decisions taken at the regional or multilateral level generally relate to their possible obstacles to trade and lack of international equity (unequal access to international markets, disproportionate reduction in the income of exporting trading partners). It is this idea that we take up by considering only the collateral effects of a reduction in health risk on the profits and surplus of consumers in the region (i.e., the available supply and the price).
17. ^In the rest of the paper, we will assume that
18. ^Due to the complexity of the supply formula and health risk at the equilibrium, numerical analyses allow to illustrate the consequences of the results set out in the proposal on both consumer surplus and health risk. Figures 2, 3 have been plotted for the following parameter values: and .
19. ^Our approach is consistent with the question posed in the empirical work of Otsuki et al. (2001) that assess the risks of chronic diseases at European level due to the presence of Aflatoxin in foods imported from Africa. Otsuki et al. (2001) show that the strict levels applied therein would not result in a significant reduction in health risk to consumers, yet would impose serious costs and/or technical difficulties on the suppliers that must achieve compliance with the regulation (Otsuki et al., 2001).
20. ^We plot the different curves of the Figure 4 by taking the following values of the parameters: , , , , and .
21. ^As the curves (Figures 4A,B) are plotted under the assumption > , It is sufficient to consider that the evolution of the threshold thresholds is from to to deduce that the properties Condition C1 et Condition C2 are not verified.
22. ^The first intuition, therefore, is to rely solely on the criterion of reducing the quantities contaminated in the second situation compared to the first without specifying in more detail the relationships to the different thresholds as done through properties (Conditions C1, C2), is incorrect.
23. ^For example, this involves investments in network infrastructure to provide reliable and affordable Internet access in rural areas, establishing digital technology training programs for farmers, providing grants or low-interest loans to help farmers acquire digital tools, thereby encouraging the development and dissemination of affordable agricultural technologies suited to local conditions, and implementing tax incentives and regulations to facilitate access to information.
24. ^For example, the MRL for glyphosate was reduced from 100 to 20 mg/kg in 2021. The MRL for chlorpyrifos, a neurotoxic insecticide, was lowered from 0.1 to 0.05 mg/kg in 2022, while in 2023, the MRL for the insecticide lindane was reduced from 0.02 mg/kg to 0 mg/kg, confirming a ban since 2004.
25. ^The plan aims to reduce the use of pesticides while maintaining a high level of agricultural production. Support measures are thus defined to, among other things, train and raise awareness among farmers about good practices, and to encourage research and innovation to develop more sustainable and less pesticide-dependent agricultural production methods.
26. ^In interpreting this requirement from the perspective of the model we propose, the Eco Phyto plan in a way requires producers to deviate from their optimal response (in terms of resources) to the thresholds set by the European Union.”
References
Acılar, A. (2011). Exploring the aspects of digital divide in a developing country. Iss. Infor. Sci. Informat. Technol. 8, 231–244. doi: 10.28945/1415
Ahmed, M. M., Sharif, U., Raza, A., Safdar, M., Ali, W., Asim, M., et al. (2024). “Climate-smart agriculture for resilience and profitability” in Sustainable practices for agriculture and marketing convergence (Hershey, Pennsylvania, USA: IGI Global), 27–50. doi: 10.4018/979-8-3693-2011-2.ch002
Bullock, D. S., Mieno, T., and Hwang, J. (2020). The value of conducting on-farm field trials using precision agriculture technology: a theory and simulations. Precis. Agric. 21, 1027–1044. doi: 10.1007/s11119-019-09706-1
Burton, R. J., and Riley, M. (2018). Traditional ecological knowledge from the internet? The case of hay meadows in Europe. Land Use Policy 70, 334–346. doi: 10.1016/j.landusepol.2017.10.014
Dabbene, F., Gay, P., and Tortia, C. (2014). Traceability issues in food supply chain management: a review. Biosyst. Eng. 120, 65–80. doi: 10.1016/j.biosystemseng.2013.09.006
De Gennaro, B. C., and Forleo, M. B. (2019). Sustainability perspectives in agricultural economics research and policy agenda. Agric. Food Econ. 7, 1–5. doi: 10.1186/s40100-019-0134-8
Dragomir, D., Dragomir, M., Acs, D., and Popescu, S. (2019). International cooperation for smart and sustainable agriculture. Sustainability assessment at the 21st century, Rijeka - Croatia: IntechOpen. 79.
El Bilali, H., and Allahyari, M. S. (2018). Transition towards sustainability in agriculture and food systems: role of information and communication technologies. Inf. Process. Agric. 5, 456–464. doi: 10.1016/j.inpa.2018.06.006
Fabregas, R., Kremer, M., and Schilbach, F. (2019). Realizing the potential of digital development: the case of agricultural advice. Science 366:eaay3038. doi: 10.1126/science.aay3038
Farooq, M. S., Riaz, S., Abid, A., Umer, T., and Zikria, Y. B. (2020). Role of IoT Technology in Agriculture: a systematic literature review. Electronics 9:319. doi: 10.3390/electronics9020319
Gebbers, R., and Adamchuk, V. I. (2010). Precision agriculture and food security. Science 327, 828–831. doi: 10.1126/science.1183899
Giraud-Héraud, E., Hammoudi, A., Hoffmann, R., and Soler, L. G. (2012). Joint private safety standards and vertical relationships in food retailing. J. Econ. Manag. Strat. 21, 179–212. doi: 10.1111/j.1530-9134.2011.00320.x
Grazia, C., Hammoudi, A., and Hamza, O. (2012). Sanitary and phytosanitary standards: does consumers’ health protection justify developing countries’ producers’ exclusion? Rev. Agric. Environ. Stud. 119, 1687–1704. doi: 10.4074/S1966960712002020
Griffin, T., Lambert, D., and Lowenberg-DeBoer, J. (2005). “Economics of lightbar and auto-guidance GPS navigation technologies” in Precision agriculture 05 (Wageningen, The Netherlands: Wageningen Academic), 581–587.
Hammoudi, A., Grazia, C., Surry, Y., and Traversac, J. B. (2015). Food safety, market organization, trade and development. Cham: Springer International Publishing, 254.
Hammoudi, A., Hoffmann, R., and Surry, Y. (2009). Food safety standards and Agri-food supply chains: an introductory overview. Eur. Rev. Agric. Econ. 36, 469–478. doi: 10.1093/erae/jbp044
Hennessy, T., Läpple, D., and Moran, B. (2016). The digital divide in farming: a problem of access or engagement? Appl. Econ. Perspect. Policy 38, 474–491. doi: 10.1093/aepp/ppw015
Huo, D., Malik, A. W., Ravana, S. D., Rahman, A. U., and Ahmedy, I. (2024). Mapping smart farming: addressing agricultural challenges in data-driven era. Renew. Sust. Energ. Rev. 189:113858. doi: 10.1016/j.rser.2023.113858
Jararweh, Y., Fatima, S., Jarrah, M., and AlZubi, S. (2023). Smart and sustainable agriculture: fundamentals, enabling technologies, and future directions. Comput. Electr. Eng. 110:108799. doi: 10.1016/j.compeleceng.2023.108799
Melo, O., Engler, A., Nahuehual, L., Cofre, G., and Barrena, J. (2014). Do sanitary, phytosanitary, and quality-related standards affect international trade? Evidence from Chilean fruit exports. World Dev. 54, 350–359. doi: 10.1016/j.worlddev.2013.10.005
Miles, A., Charron-Chénier, R., and Schleifer, C. (2019). Measuring automatic cognition: advancing dual-process research in sociology. Am. Sociol. Rev. 84, 308–333. doi: 10.1177/0003122419832497
Morchid, A., Alblushi, I. G. M., Khalid, H. M., El Alami, R., Sitaramanan, S. R., and Muyeen, S. M. (2024). High-technology agriculture system to enhance food security: a concept of smart irrigation system using internet of things and cloud computing. J. Saudi Soc. Agric. Sci. doi: 10.1016/j.jssas.2024.02.001
Nait Mohand, N., Hammoudi, A., Radjef, M. S., Hamza, O., and Perito, M. A. (2017). How do food safety regulations influence market price? A theoretical analysis. Br. Food J. 119, 1687–1704. doi: 10.1108/BFJ-12-2016-0594
Otsuki, T., Wilson, J. S., and Sewadeh, M. (2001). Saving two in a billion: quantifying the trade effect of European food safety standards on African exports. Food Policy 26, 495–514. doi: 10.1016/S0306-9192(01)00018-5
Paraforos, D. S., Ali, I., Wenzler, I., Griepentrog, H. W., Vassiliadis, V., and Berruto, R. (2016). A farm management information system using future internet technologies. IFAC Papers 49, 324–329. doi: 10.1016/j.ifacol.2016.10.060
Razak, G. M., Hendry, L. C., and Stevenson, M. (2023). Supply chain traceability: a review of the benefits and its relationship with supply chain resilience. Prod. Plan. Control 34, 1114–1134. doi: 10.1080/09537287.2021.1983661
Rejeb, A., Rejeb, K., Abdollahi, A., Al-Turjman, F., and Treiblmaier, H. (2022). The interplay between the internet of things and agriculture: a bibliometric analysis and research agenda. Internet Things 19:100580. doi: 10.1016/j.iot.2022.100580
Rotz, S., Gravely, E., Mosby, I., Duncan, E., Finnis, E., Horgan, M., et al. (2019). Automated pastures and the digital divide: how agricultural technologies are shaping labour and rural communities. J. Rural. Stud. 68, 112–122. doi: 10.1016/j.jrurstud.2019.01.023
Schimmelpfennig, D. (2016). Farm profits and adoption of precision agriculture. USDA 217, 1–46. doi: 10.22004/ag.econ.249773
Schimmelpfennig, D., and Ebel, R. (2016). Sequential adoption and cost savings from precision agriculture. J. Agric. Resour. Econ. 41, 97–115.
Senyolo, M. P., Long, T. B., Blok, V., and Omta, O. (2018). How the characteristics of innovations impact their adoption: an exploration of climate-smart agricultural innovations in South Africa. J. Clean. Prod. 172, 3825–3840. doi: 10.1016/j.jclepro.2017.06.019
Starbird, S. A. (2005). Moral hazard, inspection policy, and food safety. Am. J. Agric. Econ. 87, 15–27. doi: 10.1111/j.0002-9092.2005.00698.x
Swinton, S. M., and Lowenberg-DeBoer, J. (1998). Evaluating the profitability of site-specific farming. J. Prod. Agric. 11, 439–446. doi: 10.2134/jpa1998.0439
Vishnoi, S., and Goel, R. K. (2024). Climate smart agriculture for sustainable productivity and healthy landscapes. Environ. Sci. Pol. 151:103600. doi: 10.1016/j.envsci.2023.103600
Zhang, C., and Kovacs, J. M. (2012). The application of small unmanned aerial systems for precision agriculture: a review. Precis. Agric. 13, 693–712. doi: 10.1007/s11119-012-9274-5
Appendix
Annex 1
Annex 2
Annex 3
We have if and only if and if and only if , with:
,
Where:
Keywords: food safety, producers’ digital capabilities, public policy, health control system, industrial organization
Citation: Meziani L, Radjef MS, Perito MA and Hammoudi A (2024) Will the development of smart agriculture improve food safety in international markets? A theoretical approach. Front. Sustain. Food Syst. 8:1440006. doi: 10.3389/fsufs.2024.1440006
Edited by:
Isabelle Piot-Lepetit, INRAE Occitanie Montpellier, FranceReviewed by:
Helen Onyeaka, University of Birmingham, United KingdomPremanandh Jagadeesan, Abu Dhabi Quality and Conformity Council, United Arab Emirates
Marcello De Rosa, University of Cassino, Italy
Copyright © 2024 Meziani, Radjef, Perito and Hammoudi. This is an open-access article distributed under the terms of the Creative Commons Attribution License (CC BY). The use, distribution or reproduction in other forums is permitted, provided the original author(s) and the copyright owner(s) are credited and that the original publication in this journal is cited, in accordance with accepted academic practice. No use, distribution or reproduction is permitted which does not comply with these terms.
*Correspondence: Abdelhakim Hammoudi, YWJkZWxoYWtpbS5oYW1tb3VkaUBpbnJhZS5mcg==