- 1Key Laboratory of Sugarcane Biotechnology and Genetic Improvement (Guangxi), Ministry of Agriculture and Rural Area, Sugarcane Research Center, Chinese Academy of Agricultural Sciences, Guangxi Key Laboratory of Sugarcane Genetic Improvement, Sugarcane Research Institute, Guangxi Academy of Agricultural Sciences, Nanning, China
- 2Guangxi Key Laboratory of Crop Genetic Improvement and Biotechnology, Nanning, China
- 3Plant Cytogenetics and Molecular Biology Group, Institute of Biology, Biotechnology and Environmental Protection, Faculty of Natural Sciences, University of Silesia in Katowice, Katowice, Poland
- 4International Co-Operation Division, Guangxi Academy of Agricultural Sciences, Nanning, China
- 5College of Agriculture, Guangxi University, Nanning, China
Soil microbes have a direct impact on plant metabolism and health. The current study investigates the comparative rhizobiome between sugarcane monoculture and sugarcane–soybean intercropping. A greenhouse experiment was performed with two treatments: (1) sugarcane monoculture and (2) sugarcane–soybean intercropped. We used a high-throughput sequencing (HTS) platform to analyze the microbial community. We used the 16S rRNA gene and internal transcribed spacer region primers to identify the microbial diversity. HTS results revealed that a total of 2,979 and 124 bacterial and fungal operational taxonomic units (OTUs) were observed, respectively. Microbial diversity results concluded that the intercropping system has a beneficial impact on soil microbes. The highest numbers of bacterial and fungal OTUs were found in the intercropping system, and these results also collaborated with quantitative PCR results. Additionally, intercropped sugarcane plants showed a higher weight of above- and below-ground parts than the monoculture. Soil chemical analysis results also complemented that the intercropping system nourished organic carbon, total nitrogen, and soil enzyme activities. Correlation analysis of the diversity index and abundance concluded that soil nutrient content positively influenced the microbial abundance that improves plant growth. The present study frames out the profound insights of microbial community interaction under the sugarcane–soybean intercropping system. This information could help improve or increase the sugarcane crop production without causing any negative impact on sugarcane plant growth and development.
Introduction
Soil bacteria and fungi play a significant part in plant growth promotion (PGP) via various direct and indirect mechanisms (Glick, 1995; Olanrewaju et al., 2017). Soil nutrient mobilization is also one of the PGP traits (Meliani et al., 2012). The soil microbial community's interventions in all biological activities are well-known. However, a small amount of microbial structure and function has been documented, and still several microbial niches of structure and function are unknown (Torsvik et al., 2002; Gams, 2007; Buee et al., 2009; Berendsen et al., 2012). The rhizosphere is the soil region influenced by the plant root zone, containing up to 1011 microbial cells per gram and more than 30,000 prokaryotic species. The pooled genome of the microbial community is presented in large amounts in a plant's soil, and it is also called the plant's second genome (Berendsen et al., 2012). Rhizosphere soil is a sophisticated and active element of the plant ecosystem. The rhizospheric microbiome mainly plays a significant role in organic matter recycling and mineral immobilization for plants (Avis et al., 2008; Pii et al., 2015). A variety of rhizosphere microorganisms are involved in the different kinds of mechanisms, such as nitrogen fixation, iron chelation, mineral solubilization, pathogen suppression, and stimulation of plant defense against biotic and abiotic stresses (Patten and Glick, 1996; Andrews and Harris, 2000; Yang et al., 2016). Several studies indicate that biotic and abiotic factors influence microbial diversity, community composition, and their deviations, but there is limited literature on the involvement of these factors in soil microbial evaluation (Deng et al., 2012; Li and Wu, 2018). The high-throughput sequencing (HTS) approach helps identify and characterize the unculturable microbial gene pools from different biological systems. Universal gene-based microbial diversity analysis is one of the best methods to determine microbial abundance and structure (Bhat, 2013). HTS technology facilitates the diversity and structural analysis of rhizosphere bacterial and fungal communities in several plant-microbiome studies (Berlanas et al., 2019; Zhang et al., 2019).
Intercropping is now an attractive and common practice in the Americas, Asia, Africa, and Europe. It plays an essential role in maintaining biodiversity and high yields in agro-ecosystems (Li et al., 2018). Monoculture is generally followed in sugarcane production globally. Still, it also harms the soil nutrients, reduces the yield in ratoons, enhances fertilizer inputs, and stimulates several biotic and abiotic factors (Shoko et al., 2007). Intercropping systems change the bacterial diversity of soils and decrease the disease rates of the crops. It can efficiently utilize water and land resources and increase the yield and economic benefits of farmers (Tang et al., 2021a).
Sugarcane–legume intercropping offers a unique perspective that breaks the monoculture cycles in several plants. Sugarcane–legume intercropping is essential for various benefits, including cost-effective utilization of available land, water, light, and other natural resources. It plays a crucial role in developing and commercializing the sugarcane crop in China (Lu et al., 2011; Teshome et al., 2015). For sugarcane crop production, a high amount of nitrogen-containing fertilizers are required (Yang et al., 2013). Utilizing a high amount of nitrogen fertilizers, sugarcane crops face several problems, such as increased production costs, soil infertility, and environmental pollution (Li and Yang, 2015). Intercropping has the potential to reduce worldwide requirements of synthetic N fertilizer, and therefore, it can support the development of more sustainable cropping systems (Jensen et al., 2020).
Soybean is one of the short-duration crops, and it is appropriate for sugarcane intercropping because it can adjust in harsh conditions and fix atmospheric nitrogen in the plants (Lu et al., 2011). Sugarcane–legume intercrops properly utilize soil nutrients and atmospheric nitrogen to accomplish plant growth and minimize the quantity of fertilizer that protects the environment. Soybean intercropping reduces nitrogen input and could increase crop productivity and reduce the carbon footprint of sugarcane fields in China (Wang et al., 2020). Several studies determine that intercrops can enhance soil fertility and the microbial community (Wang et al., 2014; Solanki et al., 2018). The comparative analysis of intercropping systems is studied in maize–peanut (Li et al., 2018), mulberry–soybean (Li et al., 2016), different legume and grass species (Zhou et al., 2017), and sugarcane–soybean (Lian et al., 2019; Solanki et al., 2019). However, more studies need to understand the role of bacterial and fungal communities in the plant rhizobiome that can stimulate soil and plant health. Therefore, the present study examines the relationship between soil properties and plant growth traits with the microbial communities (bacteria and fungi) in the sugarcane–legume rhizosphere. The following objectives were tested: (1) microbial shift in sugarcane monoculture and sugarcane–soybean intercropping by the 16S rRNA gene and ITS region sequencing, (2) role of soil parameters in the microbial structure and diversity, (3) relationship of microbial communities (bacteria and fungi) and plant growth parameters. We hypothesized that the sugarcane–soybean intercropping would have significant impact on the soil properties that influence the microbial community and plant growth rate.
Materials and Methods
Plants, Experimental Design, and Sampling
Sugarcane seedlings (varGXB9) and soybean (varGC5) were collected from the breeding unit of the Sugarcane Research Institute and Cash Crop Research Institute, Guangxi Academy of Agricultural Sciences (GXAAS), Nanning, Guangxi, China. A greenhouse experiment was conducted with two treatments: sugarcane monoculture (C) and sugarcane–soybean intercropping (B) (Figure 1). In brief, all plants within a pot (diameter 30 cm; height 35 cm) were filled with 20 kg of sieved soil (<2 mm) and considered as one replicate. Two sugarcane seedlings were planted in a pot under the monoculture system, and two sugarcane seedlings with four soybean seeds were planted in the intercrop system. The greenhouse is maintained with natural night (10 h) and temperature (22°C−35°C). Pots contained soil with the following properties: pH 6.10, organic matter (OM) 11.2 g kg−1, total N 0.64 g kg−1, total P 0.52 g kg−1, total K 8.05 g kg−1, -N 3.68 mg kg−1, -N 8.09 mg kg−1, available P 26.8 mg kg−1, and available K 44.8 mg kg−1. Pots were watered every 3 days. Rhizosphere soils of intercrop and monoculture were sampled at 60 days after sowing (Figure 1).
Rhizosphere soil was recovered separately by shaking roots for 5 min into a bag and mixing thoroughly. Contact between samples was avoided. Approximately 5 g of soil from each treatment was collected. Soil samples were passed through a 2-mm sieve and stored in an ultralow temperature refrigerator at −80°C for analysis.
DNA Extraction, Sequencing PCR
The GnS-GII protocol was used for the extraction of genomic DNA from rhizosphere soil samples (Plassart et al., 2012), DNA purification was processed by the Ezup Column Soil DNA Purification Kit (Sangon Biotech, Shanghai, China), and DNA concentration was measured by NanoDrop ND-2000 UV-Vis Spectrophotometer (Thermo Scientific, Wilmington, DE, USA). 515F/806R (Peiffer et al., 2013) and ITS1F/ITS2 (Mueller et al., 2014) primer sets were used for the 16S rRNA and ITS genes, respectively. Thermal cycling consisted of initial denaturation at 98°C for 1 min, followed by 30 cycles of denaturation at 98°C for 10 s, annealing at 50°C for 30 s, and elongation at 72°C for 60 s, finally, 72°C for 5 min, following the protocols reported previously by Zhou et al. (2017). PCR products were mixed in equal density ratios. Then, the mixture of PCR products was purified with the GeneJET Gel Extraction Kit (Thermo Scientific). The library was constructed by using the TruSeq® DNA. By the use of Qubit and Q-PCR, the created library was quantified. After qualification, the library was sequenced using HiSeq2500PE250.
Bioinformatics
Raw reads were filtered by FLASH (Magoč and Salzberg, 2011), and high-quality tags data were obtained according to Bokulich et al. (2013). According to Caporaso et al. (2010), the quality check was done, and chimera sequences were filtered by UCHIME (Edgar et al., 2011; Haas et al., 2011). GRAPhlAn analysis was done by the method of Edgar et al. (2011). Operational taxonomic unit (OTU) clustering was created via UPARSE software with 97% identity (Edgar, 2013). The Mothur method and SILVA database were used for OTU annotation from phyla to species (Wang et al., 2007). QIIME software (Version 1.7.0) was used to calculate the Alpha diversity indices (Observed-species, Chao1, Shannon, and PD_whole_tree index) and Beta diversity measures. The rarefied OTU table and the phylogenetic tree were used as inputs for the subsequent Alpha and Beta diversity analyses. The principal coordinate analysis (PCoA) was used to visualize the Bray–Curtis dissimilarity matrices based on the 97% OTU level across treatments (Caporaso et al., 2010). A tree was constructed from a gap-filtered alignment using FastTree (Price et al., 2009), and the network was plotted by using Cytoscape software. The raw data of 16S rRNA and ITS were deposited in the NCBI, SRA database with accession nos. PRJNA599269 and PRJNA600092, respectively.
Real-Time PCR
The bacterial and fungal gene copy numbers were quantified using real-time PCR to estimate the abundance of bacteria and fungi. Primer 341F/518R (Moore et al., 2011) was used for bacteria and 5.8S/ITS1F (Fierer et al., 2005) for fungi. The real-time PCR assays were conducted on a real-time PCR system (Analytik Jena AG, Jena, Germany). The PCR reaction mixer contained 10 μl (2×) PCR buffer (iQTMSYBR Green Supermix, Bio-Rad), 2.5 μl of each primer (2 μmol l−1), 1 μl of template DNA, and 20 μl of sterile deionized water was added. Conditions of the qPCR were initial denaturation at 95°C for 5 min; 40 cycles of denaturation at 95°C for 15 s, annealing at 56°C (bacteria) and 55°C (fungi) for 30 s. The standard curve of DNA and copy number was constructed by the standard formula: y = −3.406 × 37.05 [y: Ct value × LOG10 (copy number)].
Soil Parameters
Soil chemical analysis and enzyme activities were carried out as described by Solanki et al. (2019). Soil pH (soil:water = 1:1) was analyzed by a pH meter, and soil organic carbon was measured by dichromate oxidation (Walkley and Black, 1934). Total N was estimated through the semi-micro-Kjeldahl method (Bremmer and Mulvaney, 1982). The FeSO4/Zn reduction method was used for the estimation of nitrate-nitrogen (NO3 -N) and ammonium nitrogen (NH4 -N) (Carter, 1993). Total phosphorus (P) was measured via the sodium carbonate fusion method (Carter, 1993). Available P was estimated by the sample extraction method (Bao, 2002). Total K estimation was done by the photometry method (Bao, 2002). The ammonium acetate extraction-flame photometry method was applied to detect the available K in the soil (Bao, 2002). Urease enzyme was determined by using phenol-sodium hypochlorite calorimetry (Guan et al., 1986). The Gerry reagent method was used to assess the nitrate reductase enzyme (Li et al., 2008). Soil enzyme dehydrogenase (DHA) was assessed by the procedure of Singh and Singh (2005), and nitrogenase was determined by measuring the acetylene reduction assay (ARA) (Hardy et al., 1968). All analysis was performed in three replicates.
Plant Parameters
Sugarcane plant growth parameters, such as fresh weight, dry weight, and plant height, were evaluated 60 days after showing by randomly selected sugarcane plants from each treatment. In addition, chlorophyll content was measured by chlorophyll meter (SPAD-502 plus Konica Minolta).
Statistical Analysis
Analysis of variance (ANOVA) following Duncan's multiple range test (DMRT) was used to analyze all experimental data. Standard errors were calculated for all mean values. Differences were considered significant at the p < 0.05 level. All experiments were performed in three replicates, and the results were expressed as mean values. Correlation analysis was performed by PAST3 software.
Results
Sequencing and Microbial Diversity
The statistical results of the sequence obtained by each step in the data processing are shown in Supplementary Table S1. Total reads of bacterial 16S rRNA were 227,090, and total reads of fungal ITS were 243,921. The average bacterial 16S rRNA total tag was 31,723, and the average OTUs were 1,691. For fungal ITS, the total tag was 40,146, and the average OTUs were 52 (Supplementary Figures S1, S2). All sequence tags were assigned to 2,979 bacterial and 124 fungal OTUs. Good's coverage values of observed species were accounted for bacterial (1533.17 ± 76.44) and fungi (47.00 ± 6.33), respectively. The alpha diversity index, such as observed specie, Chao, Shannon, A.C.E., and PD_whole_tree were found significantly higher in the bacterial soil samples of the intercropping treatment (Figure 2; Supplementary Table S2). However, the fungal alpha diversity index was not significantly higher than in the monoculture (Supplementary Table S2). Shannon diversity estimates ranged from 8.33 to 9.39 in the 16S rRNA and from 0.08 to 0.46 in the ITS samples. The Chao1 diversity estimator ranged from 1442.70 to 1924.05 in the 16S rRNA and 36.50 to 71.50 in the ITS samples. The phylogenetic distance of the whole tree estimator ranged from 123.37 to 158.81 in the 16S rRNA and from 9.39 to 17.94 in the ITS samples (Figure 2). A PCoA further demonstrated that the variation in the total data set could be attributed to monoculture and intercropping. A PCoA identified three principal component factors concerning the bacterial and fungal abundance of soil samples, explaining 55.93% and 24.31% and 50.19% and 35.06% of the total variation in monoculture and intercropping samples, respectively (Supplementary Figure S3). The PCoA plot indicates that the soil microbiota of both treatments was separated. In the case of the bacterial community, there was a separation between both treatments. Grouped intercropping samples and scattered monoculture samples show the different microbial activities. However, the fungal community-based PCoA plot diverged. The Venn diagrams show shared and unique OTUs in between both treatments. In detail, both treatments classified a total of 2,979 bacterial OTUs. Among these OTUs, 53% OTUs were common in monoculture and intercropping, and 27% unique bacterial OTUs were associated with intercropping. Besides this, in the monoculture treatment, 20% of unique bacterial OTUs were found. Moreover, a total of 124 fungal OTUs were identified, 35% of OTUs were shared between monoculture and intercropping, a higher percentage of unique fungal OTUs (40%) were found in intercropping, and the monoculture treatment had only 20% unique fungal OTUs found (Supplementary Figure S4). To quantify bacterial and fungal microbial abundance in both treatments, we used q-PCR by using the 16S rRNA-V4 gene and ITS gene primers. The results describe that intercropping samples have more gene copy numbers than the monoculture (Supplementary Figure S5).
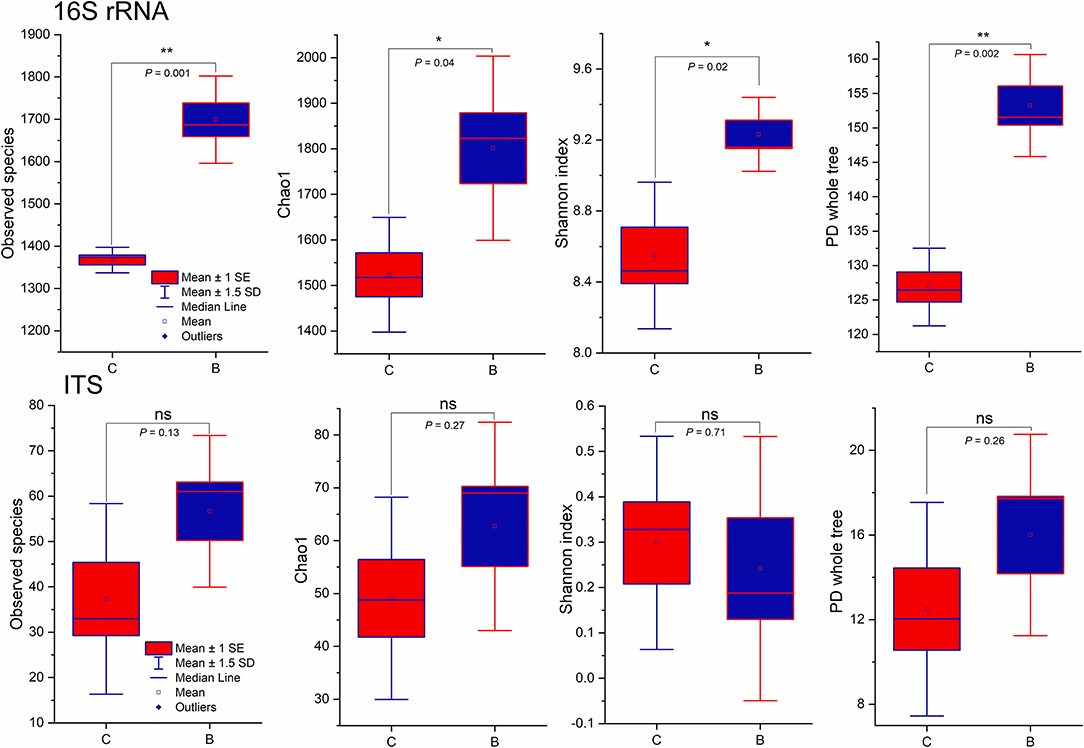
Figure 2. Alpha diversity of the microbial community associated with rhizospheric soil. Column pairs marked with an asterisk indicate significant differences between the means according to the DMRT test (p < 0.05*, P < 0.01**) B: Intercrop, C: Monoculture.
Composition and Dynamics of Bacterial and Fungal Communities
The relative abundance of each phylum was highly diverse across the monoculture (C) and intercrop (B) samples (Figure 3). In the monoculture samples, we observed a predominance of Proteobacteria (32.42% vs. 27.31% in intercrop samples), Actinobacteria (14.32% vs. 9.11% in intercrop samples), Bacteroidetes (8.14% vs. 4.78% in intercrop samples), and Firmicutes (1.45% vs. 0.61% in intercrop samples). In the intercropping samples, we observed a predominance of Acidobacteria (14.88% vs. 13.96% in monoculture samples), Chloroflexi (13.47% vs. 11.76% in monoculture samples), Cyanobacteria (10.80% vs. 4.21% in monoculture samples), Verrucomicrobia (1.82% vs. 1.26% in monoculture samples), Armatimonadetes (1.02% vs. 0.53% in monoculture samples), and Nitrospirae (0.93% vs. 0.60% in monoculture samples). The dominant fungal phyla present in monoculture were Zygomycota (97.59%), Ascomycota (2.26%), Basidiomycota (0.05%), Chytridiomycota (0%), Glomoromycota (0.004%), and others (0.08%) and in intercrop Zygomycota (97.83%), Ascomycota (0.83%), Basidiomycota (0.88%), Chytridiomycota (0.11%), Glomoromycota (0.014%), and others (0.31%) (Figure 3). The OTU species annotation results for a group of all samples were presented in conjunction with the GRAPhlAn. An OTU tree of 16S rRNA and ITS monoculture and intercrop are shown in Figures 4, 5, respectively. The circles in the figure represent different classification levels from the inside out. The size of the ring and the abundance of the species are proportional. Different colors represent diverse phylum, and solid circles represent the top 40 species in abundance. According to the species annotation and abundance information, the top 35 genera were selected according to the abundance information of each sample, and clustering was performed (Figure 6). In the case of 16S rRNA Genera, Nitrispira and Chlorobia were significantly higher compared with monoculture. In the case of ITS, Tricoderma, Curvoliria, and Cryptococcous were found to be higher as compared with the monoculture. Moreover, 15 bacterial genera were significantly higher in intercropping than monoculture (Figure 7). Except for Rhodococcus, about 15 genera, such as Haliangium, Bryobacter, Sorangium, Parafilimonas, Geobacter, Aquicella, Bdellovibria, Azoarcus, Physelicystis, Rhodoplanes, Ideonella, Polycyclovorans, Roseomonas, Desulfovirgia, and Pseudogulbenkiania, were significantly higher as compared with the monoculture.
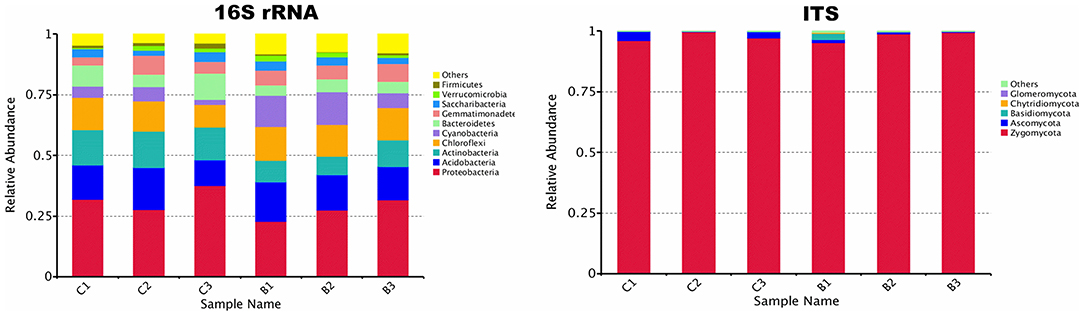
Figure 3. Relative abundance of bacterial and fungal phylum; B: Intercrop, C: Monoculture (C1, C2, C3 and B1, B2, B3 are the replicates of monoculture and intercrop treatments).
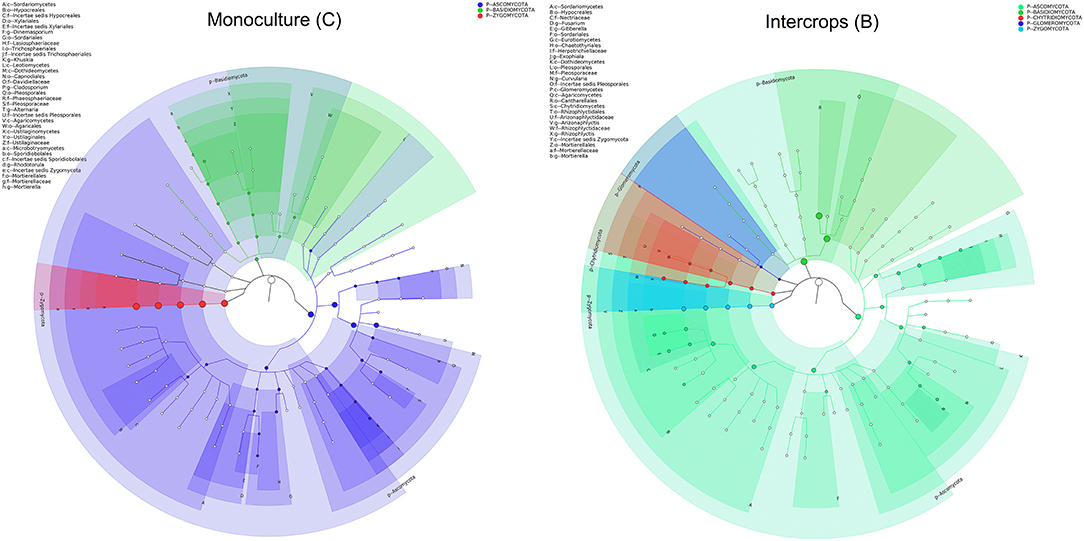
Figure 4. Species annotation of 16S rRNA groups presented in conjunction with the GRAPhlAn. The circles in the graph represent different classification levels from the inside out, the different colors represent different phylum, and the top 40 species in abundance are represented by solid circles. B: Intercrop, C: Monoculture.
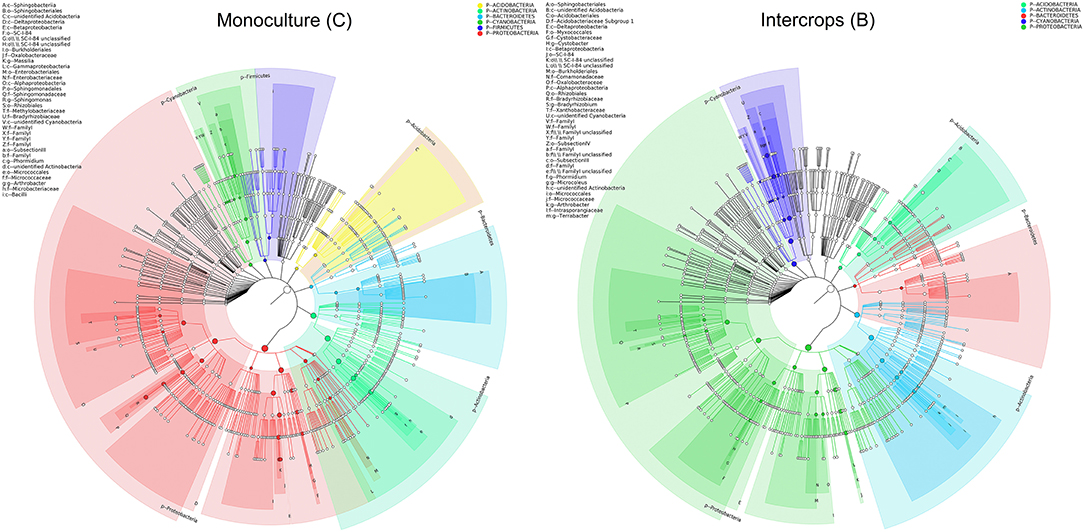
Figure 5. Species annotation of ITS groups presented in conjunction with the GRAPhlAn. The circles in the figure represent different classification levels from the inside out, the different colors represent different phylum, and the top 40 species in abundance are represented by solid circles. B: Intercrop, C: Monoculture.
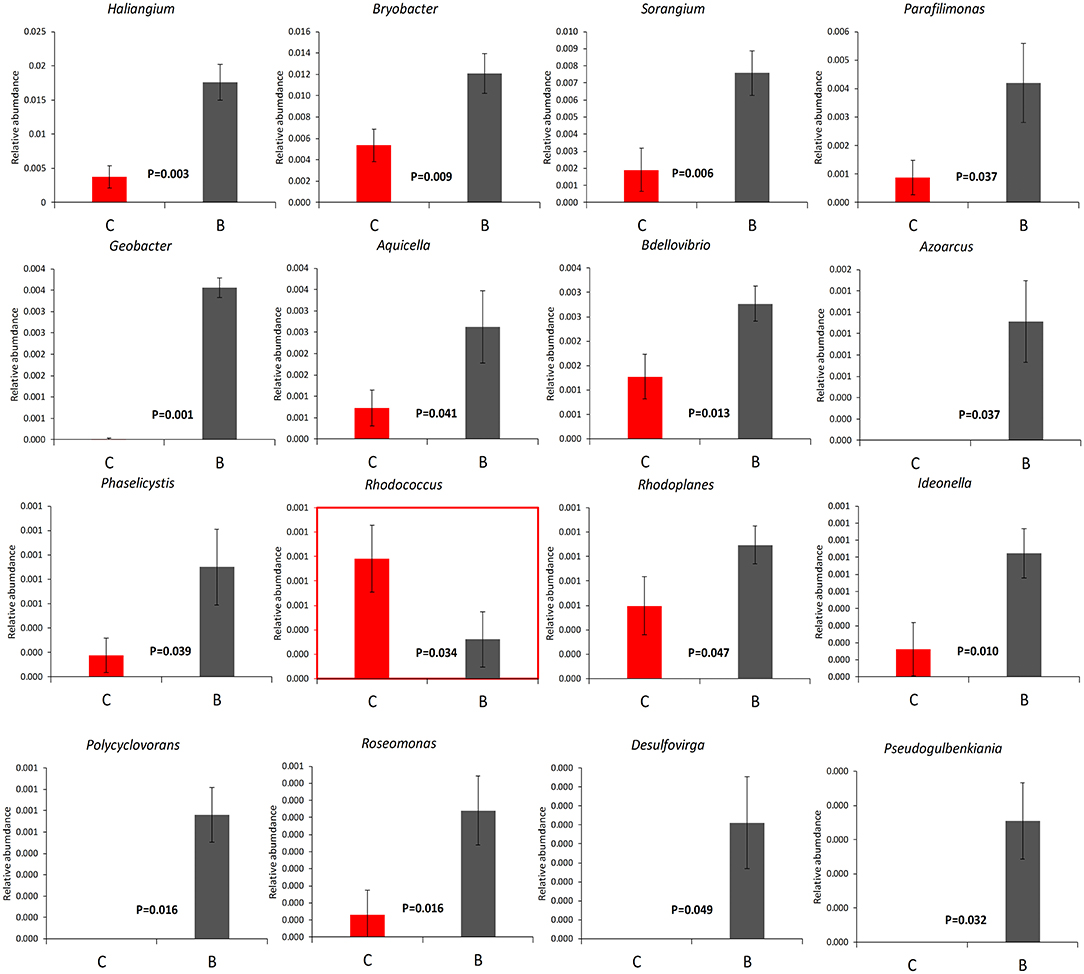
Figure 7. The relative abundance of 16 significant bacterial genera. B: Intercrop, C: Monoculture. P-values were calculated through the DMRT test and are mentioned in between both columns, mean ± standard error.
In the Beta diversity study, the weighted and unweighted unifrac distance were used to measure the dissimilarity coefficient between the two samples. The similarity between different samples was used for cluster analysis, and a sample cluster tree was constructed. Unweighted pair-group method with arithmetic mean cluster analysis was performed using the weighted unifrac distance matrix. The clustering results were incorporated with the species relative abundance column chart at the phyla taxon level (Supplementary Figure S6) for each sample of the bacterial and fungal communities.
Microbial Interactions in Samples
In the present study, relationships between genera in the samples were calculated using the correlation coefficient and visualized as a network. The absolute value of the correlation coefficient is filtered with cutoffs at 0.7, the map is taken in conjunction with the abundance of the species, and the network diagram is illustrated in Figure 8. A total of 17 bacterial phyla were found to be hub genera in the network, such as Nitrospirae, Saccharibacteria, Verrucomicrobia, Acidobacteria, Chloroflexi, Bacteroidetes, Thermomicrobia, Actinobacteria, Fibrobacteres, Armatimonadetes, Proteobacteria, Planctomycetes, Gemmatimonadetes, Cyanobacteria, Deinococcus-Thermus, Chlamydiae, and Firmicutes. Simultaneously, five dominated fungal phyla were found to be a hub in the network, such as Ascomycota, Zygomycota, Basidiomycota, Chytridiomycota, and Glomoromycota.
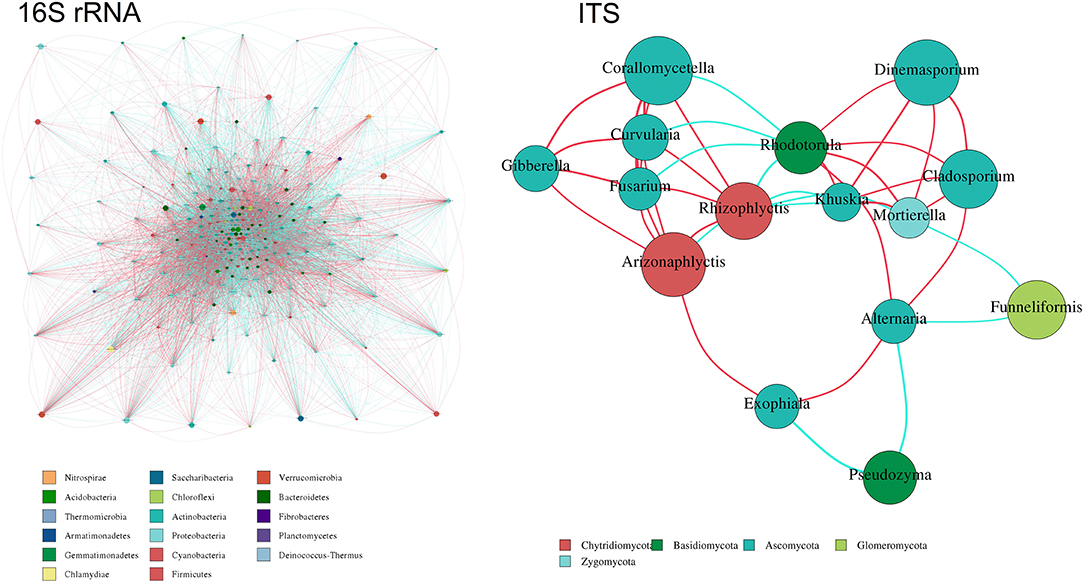
Figure 8. Co-occurrence network analysis of 16S rRNA and ITS. Different nodes represent different genus, node size represents the average relative abundance of the genus, the same branch of the node color is the same (as shown in the example), and the thickness of the connection between the nodes and the species of the correlation coefficient absolute value is positively correlated.
Soil Chemical Properties and Enzyme Activity
In the case of soil pH, compared with monoculture, intercropping decreased soil pH from 6.04 to 5.8. Organic carbon, total nitrogen, and -N were significantly increased compared with the monoculture (p < 0.05) (Table 1) although no significant effect was observed in TK, AP, AK, and . Moreover, the activity of the urease and nitrogenase was recorded significantly higher as compared with monoculture, and the intercropping system was less influenced by the nitrate reductase and dehydrogenase activities of the soil (Supplementary Figure S7).
Effect of Intercropping on Sugarcane Growth
Intercropping significantly increased the sugarcane growth and chlorophyll content over monoculture (Figure 9). The intercropping system greatly influenced the sugarcane root fresh weight and aerial part up to 14.7% and 19.2%, respectively, compared with the monoculture, and the dry weight of the root and aerial part was also increased up to 26% and 19.7%, respectively, over monoculture. Additionally, chlorophyll content was also found to be higher as compared with monoculture.
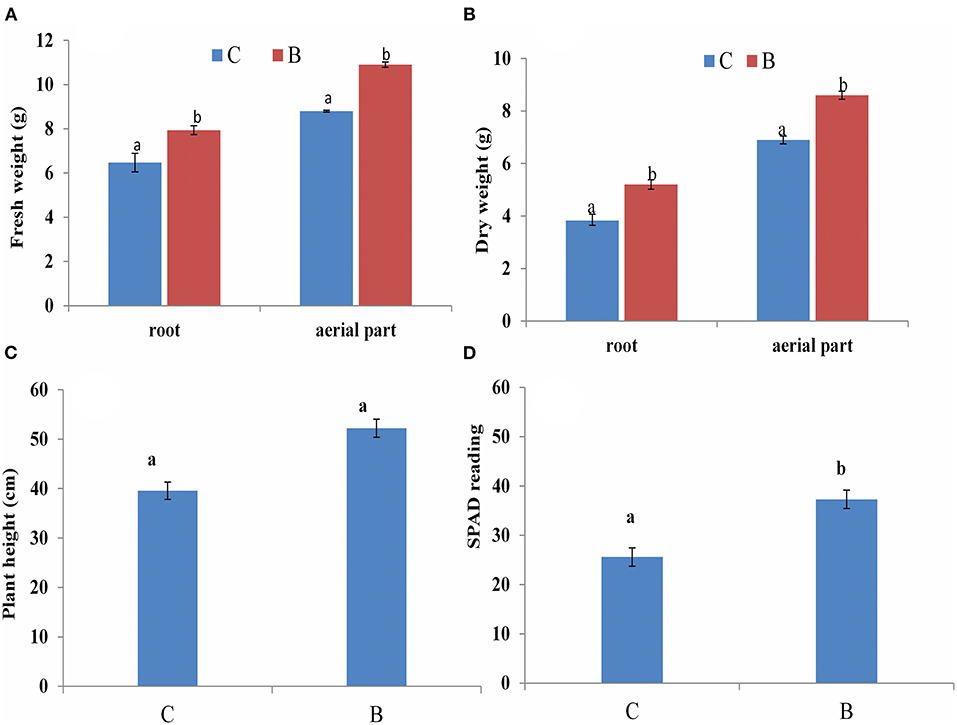
Figure 9. Sugarcane plant growth at 60 days (A) Fresh weight (B) Dry weight (C) plant height, (D) Chlorophyll content (SPAD reading). C: monoculture; B: intercrop; Columns marked with different letters indicate significant differences between the means according to DMRT test, (Mean ± SD, n = 3).
Spearman's Rank Correlation Between Soil Attributes and Relative Abundance of Soil Bacterial and Fungal Phylum
Spearman's rank correlation analysis was calculated among all the chemical properties and taxon abundance levels of bacteria and fungi, and values were illuminated in heat maps (Figures 10, 11). Correlations were observed between various soil properties, enzyme activities, and plant growth parameters with microbial community taxa. The correlation was observed between chemical properties, i.e., pH, OM, N, P, K, available P and K, NO3, and NH4. Soil enzymes included nitrogenase, urease, nitrate reductase, and dehydrogenase. Plant growth traits included root fresh weight (RFW), aerial part fresh weight (AFW), root dry weight (RDW), aerial part dry weight (ADW), plant height (PH), and chlorophyll (Chl). Spearman's rank correlation coefficients were calculated to assess the association between soil physiochemical properties and dominant bacterial and fungal taxa (genera). The relationships were estimated between these taxa and physiochemical properties to understand role of the microbial community shift. Related heat maps show that soil physiochemical factors significantly affected the relative abundance of bacterial taxa (phyla and genera) in intercropping. Among all bacteria, Sorangium, Pseudomonas, and Flavisolibacter were correlated (p < 0.05) positively with a higher number of soil and plant parameters. Soil pH had a maximum positive correlation (p < 0.05) with Sphingomonas, Massilia, Bryobacter, Curtobacterium, Pseudomonas, Parafilimonas, and Nitrospiraceae. Organic matter had a positive correlation (p < 0.05) with Sphingomonas, Flavisolibacter, Candidatus_Solibacter, Sorangium, Rhizomicrobium, Candidatus_Koribacter, Pseudomonas, Bdellovibrio, and Gemmatimonadetes. Total nitrogen and N-NO3 correlated significantly (p < 0.05) with Flavisolibacter. Phosphorus and potassium had a maximum positive correlation with Haliangium, Streptomyces, Massilia, and Bryobacter. N-NH4 showed the highest positive correlation with Sphingomonas. Enzymes nitrogenase and dehydrogenase had a maximum correlation with Sorangium and Pseudomonas. In comparison, urease showed a significant correlation with Arthrobacter, Rhizobium, Curtobacterium, and Parafilimonas. Although nitrate reductase negatively correlated with all bacterial taxa. Sorangium is significantly associated with RFW, AFW, ADW, and Chl for plant parameters.
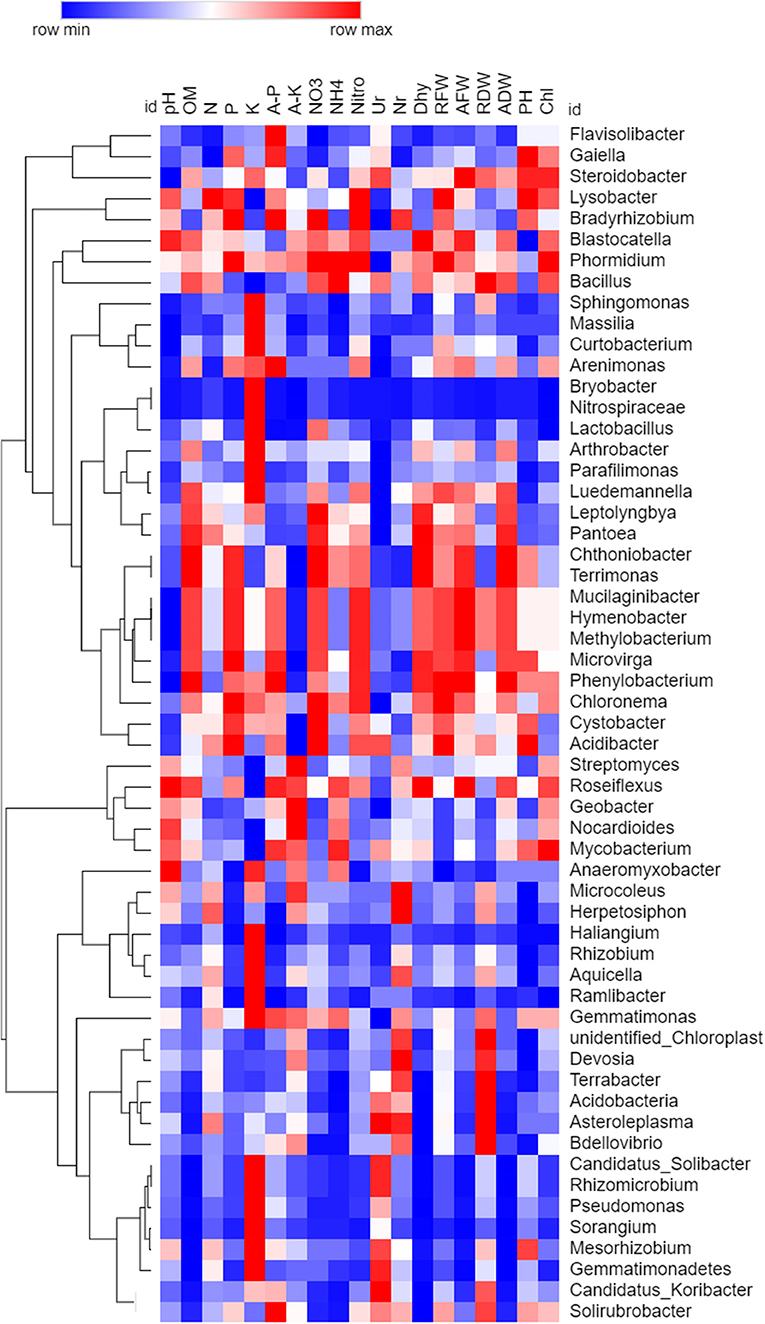
Figure 10. Spearman's rank correlation analysis between soil chemical properties, soil enzymes, and plant growth parameters with bacterial communities. The heat map is drawn based on P-value (Higher to minimum p-value indicated through red to blue color, respectively).
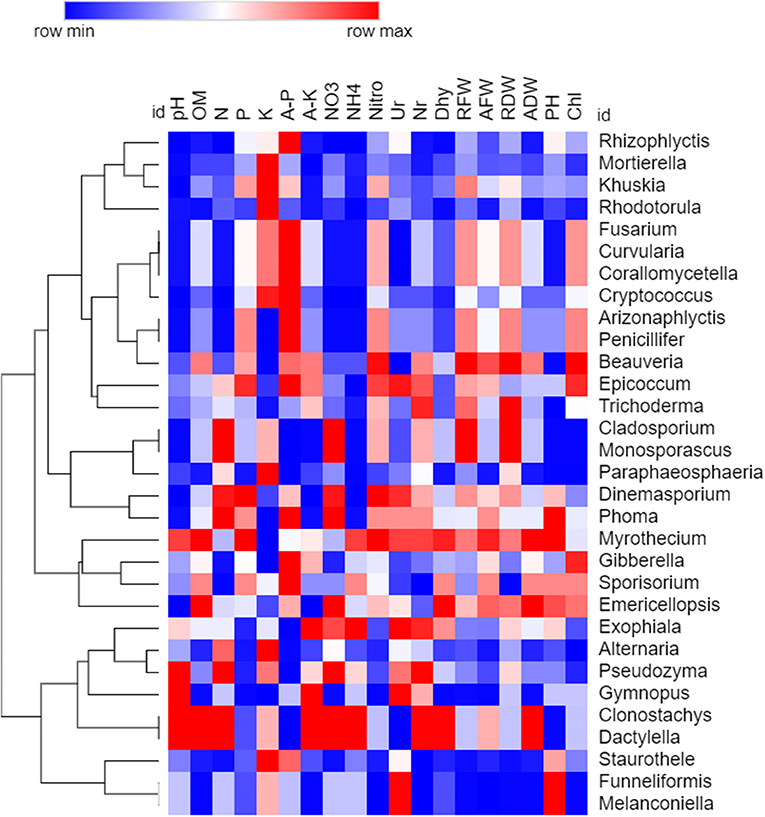
Figure 11. Spearman's rank correlation analysis between soil chemical properties, soil enzymes, and plant growth parameters with fungal communities. The heat map is drawn based on P-value (Higher to minimum p-value indicated through red to blue color, respectively).
For fungi, genus Staurothele exhibited a higher positive correlation with different soil (organic matter, total N, N-NO3, nitrogenase, nitrate reductase, and dehydrogenase) and plant parameters (RFW, RDW, AFW, and ADW). Genus Fusarium, Curvularia, and Corallomycetella were positively correlated with soil pH, total N, N-NO3, N-NH4, enzyme urease, and plant height, N, and nitrate. Similarly, the Cryptococcus genus positively correlated with all these soil parameters and dehydrogenase except urease and plant height. Genus Rhodotorula was positively correlated with soil pH, organic matter, available K, N-NH4, enzyme dehydrogenase, and AFW.
Discussion
The intercropping system is essential in modern agricultural techniques. It can improve crop yield and soil quality, and therefore, changes in soil properties manipulate the activities and community structure of the soil microorganisms (Zhang et al., 2018; Tang et al., 2020). Intercropping plays an essential role in greenhouse production and affects soil physiochemical properties and soil microbial communities' structure (Li and Wu, 2018). It has been broadly used to control plant diseases and improve the growth of crops (Li et al., 2018). In addition, it can enhance the microbial activity of the soil. Without causing any negative impact on soil parameters, soil fertility enhancement could be a new approach to increase crop production (Solanki et al., 2020).
The present study demonstrates that intercropping enriches the soil chemical property and the microbial community structure. We found that the microbial community structure associated with intercropping was more significant than that associated with monoculture. The present study results are consistent with recent reports in which sugarcane intercropped with peanut and mustard or potato showed that intercropping increased soil microbial diversity and improved soil quality and crop productivity compared with the monoculture (Singh et al., 2021; Tang et al., 2021b).
Moreover, we observed that intercropping increased the soil enzyme activity and the growth of sugarcane plants. Previously, intercropping promoted the rhizosphere microbial population and increased enzyme activity and soil nutrition compared with monoculture (Yang et al., 2011). First, in the present study, monoculture and intercropping decreased soil pH, but intercropping decreased more than monoculture. Previous reports indicate that lower soil pH might be related to higher nutrient availability and consequent uptake by roots directly (Eskelinen et al., 2009; Ghani et al., 2019). These results are consistent with previous intercropping studies in cassava–soybean (Makinde et al., 2006) and pepper–garlic (Ahmad et al., 2013), which report that the intercropping system decreased the soil pH. Some other reports indicate that intercropping changed the soil properties, positively altering the microbial community structure (Li et al., 2016; Lian et al., 2019). Total nitrogen (TN) was significantly increased in this study. These were consistent with various intercropping studies, for instance, sugarcane–soybean (Lian et al., 2019) and cassava–peanut intercropping (Tang et al., 2020). It is accepted that TN was increased because of the biological nitrogen fixation associated with a legume (Wu et al., 2017). In addition, intercropping of soybean with sugarcane improved soil TN and SOC due to the organic matter form of litterfall (Lian et al., 2019). The present study results of soil enzymes indicate that intercropping significantly increased urease and nitrogenase activities compared with monoculture. Our results are consistent with Li et al. (2012), who report that intercropping sugarcane and soybean promoted nitrogenase and urease activity. These results are also compatible with several studies that indicate the intercropping system improved the soil enzyme activities and soil nutrient content compared with monoculture (Zhang and Li, 2003; Wang et al., 2014; Solanki et al., 2018).
The present study indicates that the bacterial community associated with intercropping was significantly higher than that with monoculture. However, the diversity of the fungal communities associated with intercropping was not significantly increased but was more elevated than monoculture (Supplementary Table S2; Figure 3). Intercropping of sugarcane with soybean enhanced the bacterial and fungal abundances, consistent with several previous studies (Li et al., 2016; Li and Wu, 2018; Lian et al., 2019). Recently, Liu et al. (2021) reported that sugarcane varieties intercropped with soybean significantly increased bacterial diversity and played a significant role in shifting the root environment to help the healthy bacterial community enhance plant growth.
Elevated bacterial and fungal abundances can reveal that the intercropping system stimulates the roots to release high levels of nutrients (Song et al., 2007). Environmental factors, such as pH and soluble organic carbon (SOC), often play essential roles in influencing microbial community composition and diversity (Ma et al., 2018; Tripathi et al., 2018). In this study, we observed the dominant taxonomic groups for microbial analysis of rhizospheric soil, including Proteobacteria, Acidobacteria, Actinobacteria, and Bacteroidetes. However, monoculture soils contained more Proteobacteria than the corresponding intercropped soil. Although intercropping carried more Chloroflexi than monoculture, this result is inconsistent with the previous study (Li et al., 2016). In the present study, phyla Acidobacter, Chloroflexi, Cynobacteria, Verrucomircobia, and Nitrospora were higher than monoculture. Acidobacteria and Chloroflexi play a vital role in litter decomposition (Eichorst et al., 2011; Purahong et al., 2016). In addition, we observed that the percentage of Nitrospirae and Gemmatimonadetes is higher in intercropping systems than in monoculture. This result is consistent with a recent study on peanut–cassava intercropping, which reported that the percentage of Nitrospirae, Verrucomicrobia, and Gemmatimonadetes in the rhizospheric soils of intercropping systems were higher than in monoculture (Tang et al., 2020). Moreover, in the present study, Protebacteria and acidobacteria were significantly higher in intercropping compared with monoculture. Proteobacteria are significantly associated with the plant rhizosphere, and several nonsymbiotic proteobacteria have been recognized as free-living diazotrophs, such as Azospirillum, Azospira, Azotobacter, Burkholderia, Herbaspirillum, Pelomonas, Pseudacidovorax, and Sphingomonas (Pankievicz et al., 2015; Roley et al., 2019; Solanki et al., 2020).
In comparison, fungal communities showed differences with intercropping and monoculture. Zygomycota, Basidiomycota, Chytridiomycota, and other groups were found to be higher in intercropping than monoculture, which allies with previous studies (Lian et al., 2018, 2019). Members of Zygomycota and Chytridiomycota are known to utilize dead plant material, which serves as a significant nutrient source (Misra et al., 2019). The beta diversity analysis results showed a substantial proportion of microbial communities and composition variations across the rhizosphere soil samples. Proteobacteria, Acidobacteria, and Actinobacteria comprised the predominant bacterial content of the microbiomes. This result is consistent primarily with previous articles investigating the bacterial community in sugarcane monoculture (Gao et al., 2019) and sugarcane intercrop with soybean (Lian et al., 2019). Correlation analysis results show that, among all bacteria, Sorangium, Pseudomonas, and Flavisolibacter correlate (p < 0.05) positively with a higher number of soil and plant parameters. Sorangium and its subgroup have the potential for secondary metabolite production (Lee et al., 2013), Psedomonas are well-known for plant growth and plant disease suppression activity (Tao et al., 2020), and Flavisolibacter also play a role in PGP (Lin et al., 2021).
The present study shows that intercropping has a significant impact on sugarcane biomass as compared with monoculture. These results are consistent with a previous study in which the stalk diameter, cane yield, and sugar production were significantly affected by sugarcane–soybean intercropping compared with monoculture (Yang et al., 2013). Besides this, Khippal et al. (2016) also observed that other crops, such as chickpea and lentils could be effectively intercropped with sugarcane to improve the cane quality and soil physical condition for sustainable agricultural crop production. Recent reports also indicate that maize intercropping improves nutrition (Ridaura et al., 2021), reduces disease (Chang et al., 2020), and enhances the yield of the crops (Crusciol et al., 2020).
Conclusions
The cropping system plays a vital role in soil chemical and natural properties. In the present study, soil organic matter and microbial biomass were higher in the intercropping system: sugarcane–soybean intercropping increased microbial diversity and soil physiochemical properties, and furthermore, intercropping enhanced soil enzyme activities and sugarcane plant growth. Moreover, the bacterial and fungal communities are influenced by the intercropping system. Some of the bacterial genera were significantly increased due to intercropping. It is further needed to work out the isolation of potential bacteria and their role in plant growth. Our overall perception of these diverse sets of data indicate that sugarcane and soybean intercropping could improve the soil chemical property and increase the microbial diversity and growth of sugarcane. Although the present study results are held in the greenhouse to be transferred to field conditions, it can serve as background for further field studies.
Data Availability Statement
The datasets presented in this study can be found in online repositories. The names of the repository/repositories and accession number(s) can be found in the article/Supplementary Material.
Author Contributions
MM, Y-RL, and L-TY: conceived and designed the experiments. MM and C-NL: performed the experiments. MM, MS, C-NL, and ZW: analyzed the data. MM, YZ, RS, KV, PS, H-RH, and X-PS: contributed reagents/materials/analysis tools. MM, MS, and Y-RL: wrote the paper. All authors have read and agreed to the published version of the manuscript.
Funding
This work was supported by grants from the National Natural Science Foundation of China (Grant Numbers 31471449 to Y-RL), Natural Science Foundation of Guangxi Province (Grant Numbers 2016GXNSFAA380126 and 2019GXNSFDA185004 to C-NL), Guangxi Innovation Term of Modern Agriculture Technology (Grant Numbers gjnytxgxcxtd-03-01 to Y-RL), Guangxi R&D Program (Grant Numbers GKG1598006-1-1 and GuiKeAD17195100 to Y-RL).
Conflict of Interest
The authors declare that the research was conducted in the absence of any commercial or financial relationships that could be construed as a potential conflict of interest.
Publisher's Note
All claims expressed in this article are solely those of the authors and do not necessarily represent those of their affiliated organizations, or those of the publisher, the editors and the reviewers. Any product that may be evaluated in this article, or claim that may be made by its manufacturer, is not guaranteed or endorsed by the publisher.
Acknowledgments
The authors would like to thank National Natural Science Foundation of China, China Postdoctoral Foundation, Guangxi Innovation Term of Modern Agriculture Technology, and Guangxi Academy of Agricultural Sciences Nanning, Guangxi, China, for providing the necessary facilities for this study.
Supplementary Material
The Supplementary Material for this article can be found online at: https://www.frontiersin.org/articles/10.3389/fsufs.2021.606595/full#supplementary-material
References
Ahmad, I., Cheng, Z., Meng, H., Liu, T., Wang, M., Ejaz, M., et al. (2013). Effect of pepper-garlic intercropping system on soil microbial and bio-chemical properties. Pak. J. Bot. 45, 695–702.
Andrews, J. H., and Harris, R. F. (2000). The ecology and biogeography of microorganisms on plant surfaces. Ann. Rev. Phytopathol. 38, 145–180. doi: 10.1146/annurev.phyto.38.1.145
Avis, T. J., Gravel, V., Antoun, H., and Tweddell, R. J. (2008). Multifaceted beneficial effects of rhizosphere microorganisms on plant health and productivity. Soil. Biol. Biochem. 40, 733–1740. doi: 10.1016/j.soilbio.2008.02.013
Berendsen, R. L., Pieterse, C. M. J., and Bakker, P. A. H. M. (2012). The rhizospheremicrobiome and plant health. Trends Plant Sci. 17:8. doi: 10.1016/j.tplants.2012.04.001
Berlanas, C., Berbegal, M., Elena, G., Laidani, M., Cibriain, J. F., Sagües, A., et al. (2019). The fungal and bacterial rhizosphere microbiome associated with grapevine rootstock genotypes in mature and young vineyards. Front. Microbiol. 10:1142. doi: 10.3389/fmicb.2019.01142
Bhat, A. K. (2013). Preserving microbial diversity of Soil ecosystem: a key to sustainable productivity. Int. J. Curr. Microbiol. Appl. Sci. 2, 85–101.
Bokulich, N. A., Subramanian, S., Faith, J. J., Gevers, D., Gordon, J. I., Knight, R., et al. (2013). Quality-filtering vastly improves diversity estimates from Illuminaamplicon sequencing. Nat. Methods 10, 57–59. doi: 10.1038/nmeth.2276
Bremmer, J. M., and Mulvaney, C. S. (1982). “Total nitrogen,” in Methods of Soil Analysis—Part 2. In Chemical and Microbiological Properties, ed C. A. Bluck (Madison: American Society of Agronomy), 595–624.
Buee, M., Reich, M., Murat, C., Morin, E., Nilsson, R. H., Uroz, S., et al. (2009). 454 Pyrosequencing analyses of forest soils reveal an unexpectedly high fungal diversity. New Phytol. 184, 449–456. doi: 10.1111/j.1469-8137.2009.03003.x
Caporaso, J. G., Kuczynski, J., Stombaugh, J., Bittinger, K., Bushman, F. D., Costello, E. K., et al. (2010). QIIME allows analysis of high-throughput community sequencing data. Nat. Methods 7, 335–336. doi: 10.1038/nmeth.f.303
Chang, X., Yan, L., Naeem, M., Khaskheli, M. I., Zhang, H., Gong, G., et al. (2020). Maize/Soybean relay strip intercropping reduces the occurrence of fusarium root rot and changes the diversity of the pathogenic Fusarium Species. Pathogens 9:211; doi: 10.3390/pathogens9030211
Crusciol, C. A. C., Mateus, G. P., Momesso, M. L., Pariz, C. M., Castilhos, A. M., Calonego, J. C., et al. (2020). Nitrogen-fertilized systems of maize intercropped with tropical grasses for enhanced yields and estimated land use and meat production. Front. Sustain. Food Syst. 4:544853. doi: 10.3389/fsufs.2020.544853
Deng, Y., Jiang, Y. H., Yang, Y., He, Z., Luo, F., and Zhou, J. (2012). Molecular ecological network analyses. BMC. Bioinform. 13:113. doi: 10.1186/1471-2105-13-113
Edgar, R. C. (2013). UPARSE: highly accurate OTU sequences from microbial amplicon reads. Nat. Methods. 10, 996–998. doi: 10.1038/nmeth.2604
Edgar, R. C., Haas, B. J., Clemente, J. C., Quince, C., and Knight, R. (2011). UCHIME improves sensitivity and speed of chimera detection. Bioinformatics 27, 2194–2200. doi: 10.1093/bioinformatics/btr381
Eichorst, S. A., Kuske, C. R., and Schmidt, T. M. (2011). Influence of plant polymers on the distribution and cultivation of bacteria in the phylum Acidobacteria. Appl. Environ. Microbiol. 77, 586–596. doi: 10.1128/AEM.01080-10
Eskelinen, A., Stark, S., and Männistö, M. (2009). Links between plant community composition, soil organic matter quality, and microbial communities in contrasting tundra habitats. Oecologia 161, 113–123. doi: 10.1007/s00442-009-1362-5
Fierer, N., Jackson, J. A., Vilgalys, R., and Jackson, R. B. (2005). Assessment of soil microbial community structure by use of taxon-specific quantitative PCR assays. Appl. Environ. Microbiol. 71, 4117–4120. doi: 10.1128/AEM.71.7.4117-4120.2005
Gams, W. (2007). Biodiversity of soil-inhabiting fungi. Biodivers. Conserv. 16, 69–72. doi: 10.1007/s10531-006-9121-y
Gao, X., Wu, Z., Liu, R., Wu, J., Zeng, Q., and Qi, Y. (2019). Rhizosphere bacterial community characteristics over different years of sugarcane ratooning in consecutive monoculture. BioMed Res. Int. 2019:4943150. doi: 10.1155/2019/4943150
Ghani, M. I., Ali, A., Atif, M. J., Ali, M., Amin, B., Anees, M., et al. (2019). Changes in the soil microbiome in eggplant monoculture revealed by high-throughput illumine miseq sequencing as influenced by raw garlic stalk amendment. Int. J. Mol. Sci. 20:2125. doi: 10.3390/ijms20092125
Glick, B. R. (1995). The enhancement of plant growth by free-living bacteria. Can. J. Microbiol. 41, 109–117.
Guan, S. Y., Zhang, D., and Zhang, Z. (1986). Soil Enzyme and Its Research Methods. Beijing: Agricultural.
Haas, B. J., Gevers, D., Earl, A. M., Feldgarden, M., Ward, D. V., Giannoukos, G., et al. (2011). Chimeric 16SrRNA sequence formation and detection in Sanger and 454-pyrosequencedPCR amplicons. Genome Res. 21, 494–504. doi: 10.1101/gr.112730.110
Hardy, R. W., Holsten, R. D., Jackson, E. K., and Burns, R. C. (1968). The acetyleneethylene assay for n(2) fixation: laboratory and field evaluation. Plant Physiol. 43, 1185–1207. doi: 10.1104/pp.43.8.1185
Jensen, E. S., Carlsson, G., and Nielsen, H. H. (2020). Intercropping of grain legumes and cereals improves the use of soil N resources and reduces the requirement for synthetic fertilizer N: a global-scale analysis. Agron. Sustain. Dev. 40:5. doi: 10.1007/s13593-020-0607-x
Khippal, A., Singh, S., Meharchand, S, heokand, R., Singh, J., Verma, R., et al. (2016). Mechanized and profitable intercropping of legumes in autumn planted sugarcane. Legume Res. 39, 411–418. doi: 10.18805/lr.v0iOF.10283
Lee, C., An, D., Lee, H., and Cho, K. (2013). Correlation between Sorangium cellulosum subgroups and their potential for secondary metabolite production. J. Microbiol. Biotechnol. 23, 297–303. doi: 10.4014/jmb.1210.10054
Li, Q., Chen, J., Wu, L., Luo, X., Li, N., Arafat, Y., et al. (2018). Belowground interactions impact the soil bacterial community, soil fertility, and crop yield in maize/peanut intercropping systems. Int. J. Mol. Sci. 19:622. doi: 10.3390/ijms19020622
Li, S., and Wu, F. (2018). Diversity and co-occurrence patterns of soil bacterial and fungal communities in seven intercropping systems. Front. Microbiol. 9:1521. doi: 10.3389/fmicb.2018.01521
Li, X., Penttinen, P., Gu, Y., and Zhang, X. (2012). Diversity of nifH gene in rhizosphere and non-rhizosphere soil of tobacco in Panzhihua, China. Ann. Microbiol. 62, 995–1001. doi: 10.1007/s13213-011-0339-x
Li, X., Sun, M., Zhang, H., Xu, N., and Sun, G. (2016). Use of mulberry–soybean intercropping in salt–alkali soil impacts the diversity of the soil bacterial community. Microb. Biotechnol. 9, 293–304. doi: 10.1111/1751-7915.12342
Li, Y. R., and Yang, L.T. (2015). Sugarcane agriculture and sugar industry in China. Sugar Tech. 17, 1–8. doi: 10.1007/s12355-014-0342-1
Li, Z. G., Luo, Y. M., and Teng, Y. (2008). Research Methods for Soil and Environmental Microbiology. Beijing: Science Press.
Lian, T., Mu, Y., Jin, J., Ma, Q., Cheng, Y., Cai, Z., et al. (2019). Impact of intercropping on the coupling between soil microbial community structure, activity, and nutrient-use efficiencies. Peer J. 7:e6412. doi: 10.7717/peerj.6412
Lian, T., Mu, Y., Ma, Q., Cheng, Y., Gao, R., Cai, Z., et al. (2018). Use of sugarcane–soybean intercropping in acid soil impacts the structure of the soil fungal community. Sci. Rep. 8:14488. doi: 10.1038/s41598-018-32920-2
Lin, H., Liu, C., Li, B., and Dong, Y. (2021). Trifolium repens L. regulated phytoremediation of heavy metal contaminated soil by promoting soil enzyme activities and beneficial rhizosphere associated microorganisms. J. Hazard. Mater. 402:123829. doi: 10.1016/j.jhazmat.2020.123829
Liu, Y., Yang, H., Liu, Q., Zhao, X., Xie, S., Wang, Z., et al. (2021). Effect of two different sugarcane cultivars on rhizosphere bacterial communities of sugarcane and soybean upon intercropping. Front. Microbiol. 11:596472. doi: 10.3389/fmicb.2020.596472
Lu, C. J., Ming, W. J., and Zhong, S. H. (2011). A review on the researches on sugarcane-soybean intercropping system. J. Southern Agric. 42, 898–900.
Ma, B., Lv, X., Cai, Y., Chang, S. X., and Dyck, M. F. (2018). Liming does not counteract the influence of long-term fertilization on soil bacterial community structure and its co-occurrence pattern. Soil Biol. Biochem. 123, 45–53. doi: 10.1016/j.soilbio.2018.05.003
Magoč, T., and Salzberg, S. L. (2011). FLASH: fast length adjustment of short reads to improve genome assemblies. Bioinformatics 27, 2957–2963. doi: 10.1093/bioinformatics/btr507
Makinde, E., Oluwatoyinbo, F. I., and Ayoola, O. (2006). Intercropping and crop residue incorporation: effects on soil nutrient status. J. Plant Nutr. 29, 235–244. doi: 10.1080/01904160500474082
Meliani, A., Bensoltane, A., and Mederbel, K. (2012). Microbial diversity and abundance in soil: related to plant and soil type. Am. J. Plant Nutr. Fertilization Technol. 2, 10–18. doi: 10.3923/ajpnft.2012.10.18
Misra, P., Maji, D., Awasthi, A., Pandey, S. S., Yadav, A., Pandey, A., et al. (2019). Vulnerability of soil microbiome to monocropping of medicinal and aromatic plants and its restoration through intercropping and organic amendments. Front. Microbiol. 10:2604. doi: 10.3389/fmicb.2019.02604
Moore, T. A., Xing, Y., Lazenby, B., Lynch, M. D. J., Schiff, S., Robertson, W. D., et al. (2011). Prevalence of anaerobic ammonium-oxidizing bacteria in contaminated groundwater. Environ. Sci. Technol. 45, 7217–7225. doi: 10.1021/es201243t
Mueller, R. C., Paula, F. S., Mirza, B. S., Rodrigues, J. L. M., Nüsslein, K., and Bohannan, B. J. M. (2014). Links between plant and fungal communities across a deforestation chronosequence in the Amazon rainforest. ISME J. 8, 1548–1550. doi: 10.1038/ismej.2013.253
Olanrewaju, O. S., Glick, B. R., and Babalola, O. O. (2017). Mechanisms of action of plant growth promoting bacteria. World J. Microbiol. Biotechnol. 33:197. doi: 10.1007/s11274-017-2364-9
Pankievicz, V. C. S., do Amaral, F. P., Santos, K. F. D. N., Agtuca, B., Xu, Y., Schueller, M. J., et al. (2015). Robust biological nitrogen fixation in a model grass-bacterial association. Plant J. 81, 907–919. doi: 10.1111/tpj.12777
Patten, C. L., and Glick, B. R. (1996). Bacterial biosynthesis of indole-3-acetic acid. Can. J. Microbiol. 42, 207–220. doi: 10.1139/m96-032
Peiffer, J. A., Spor, A., Koren, O., Jin, Z., Tringe, S. G., Dangl, J. L., et al. (2013). Diversity and heritability of the maize rhizospheremicrobiome under field conditions. Proc. Natl. Acad. Sci. U.S.A. 110, 6548–6553. doi: 10.1073/pnas.1302837110
Pii, Y., Mimmo, T., Tomasi, N., Terzano, R., Cesco, S., and Crecchio, C. (2015). Microbial interactions in the rhizosphere: beneficial influences of plant growth-promoting rhizobacteria on nutrient acquisition process: a review. Biol. Fertil. Soils. 51, 403–415. doi: 10.1007/s00374-015-0996-1
Plassart, P., Terrat, S., Thomson, B., Griffiths, R., Dequiedt, S., Lelievre, M., et al. (2012). Evaluation of the ISO Standard 11063 DNA Extraction Procedure for Assessing Soil Microbial Abundance and Community Structure. PLoS ONE 7:e44279. doi: 10.1371/journal.pone.0044279
Price, M. N., Dehal, P. S., and Arkin, A. P. (2009). FastTree: computing large minimum evolution trees with profiles instead of a distance matrix. Mol. Biol. Evol. 26, 1641–1650. doi: 10.1093/molbev/msp077
Purahong, W., Wubet, T., Lentendu, G., Schloter, M., Pecyna, M. J., Kapturska, D., et al. (2016). Life in leaf litter: novel insights into community dynamics of bacteria and fungi during litter decomposition. Mol. Ecol. 25, 4059–4074. doi: 10.1111/mec.13739
Ridaura, S. L., Escoto, L. B., Ramirez, C. A. R., Sum, C., Rojas, N. P., and Gerard, B. (2021). Maize intercropping in the milpa system. Diversity, extent and importance for nutritional security in the Western Highlands of Guatemala. Sci. Rep. 11:3696. doi: 10.1038/s41598-021-82784-2
Roley, S. S., Xue, C., Hamilton, S. K., Tiedje, J. M., and Robertson, G. P. (2019). Isotopic evidence for episodic nitrogen fixation in switchgrass (Panicum virgatum L.). Soil Biol. Biochem. 129, 90–98. doi: 10.1016/j.soilbio.2018.11.006
Shoko, M. D., Tagwira, F., and Zhou, M. (2007). The potential of reducing nitrogen fertilizers in a soybean-sugarcane production system in Zimbabwe. Afr. J. Agric. Res. 2, 475–480.
Singh, J., and Singh, D. K. (2005). Dehydrogenase and phosphomonoesterase activities in groundnut (Arachishypogaea L.) field after diazinon, imidacloprid and lindane treatments. Chemosphere 60, 32–42. doi: 10.1016/j.chemosphere.2004.11.096
Singh, S. R., Yadav, P., Singh, D., Shukla, S. K., Tripathi, M. K., Lal, B., et al. (2021). Intercropping in sugarcane improves functional diversity, soil quality, and crop productivity. Sugar Tech. 23, 794–810. doi: 10.1007/s12355-021-00955-x
Solanki, M. K., Wang, F. Y., Li, C. N., Wang, Z., Lan, T. J., Singh, R. K., et al. (2019). Impact of sugarcane–legume intercropping on diazotrophic microbiome. Sugar Tech. 22, 52–64. doi: 10.1007/s12355-019-00755-4
Solanki, M. K., Wang, F. Y., Wang, Z., Li, C. N., Lan, T. J., Singh, R. K., et al. (2018). Rhizospheric and endospheric diazotrophs mediated soil fertility intensification in sugarcane-legume intercropping systems. J. Soil Sediments 19, 1911–1927. doi: 10.1007/s11368-018-2156-3
Solanki, M. K., Wang, Z., Wang, F. Y., Li, C. N., Lal, C. G., Singh, R. K., et al. (2020). Assessment of diazotrophic proteobacteria in sugarcane rhizosphere when intercropped with legumes (Peanut and Soybean) in the field. Front. Microbiol. 11:1814. doi: 10.3389/fmicb.2020.01814
Song, Y. N., Marschner, P., Li, L., Bao, X. G., Sun, J. H., and Zhang, F. S. (2007). Community composition of ammonia-oxidizing bacteria in the rhizosphere of intercropped wheat (Triticumaestivum L.), maize (Zea mays L.), and faba bean (Viciafaba L.). Bio. Fert. Soils. 44, 307–314. doi: 10.1007/s00374-007-0205-y
Tang, X., Jiang, J., Huang, Z., Wu, H., Wang, J., He, L., et al. (2021a). Sugarcane/peanut intercropping system improves the soil quality and increases the abundance of beneficial microbes. J. Basic Microbiol. 61, 165–176. doi: 10.1002/jobm.202000750
Tang, X., Zhang, Y., Jiang, J., Meng, X., Huang, Z., Wu, H., et al. (2021b). Sugarcane/peanut intercropping system improves physicochemical properties by changing N and P cycling and organic matter turnover in root zone soil. Peer J. 9:e10880. doi: 10.7717/peerj.10880
Tang, X., Zhong, R., Jiang, J., He, L., Huang, Z., Shi, G., et al. (2020). Cassava/peanut intercropping improves soil quality via rhizospheric microbes increased available nitrogen contents Tang et al. BMC Biotechnol. 20:13. doi: 10.1186/s12896-020-00606-1
Tao, C., Li, R., Xiong, W., Shen, Z., Liu, S., Wang, B., et al. (2020). Bio-organic fertilizers stimulate indigenous soil Pseudomonas populations to enhance plant disease suppression. Microbiome 8, 1–14. doi: 10.1186/s40168-020-00892-z
Teshome, Z., Fantaye, A., and Hagos, H. (2015). Effect of nitrogen and phosphorus on yield components, yield, and sugarcane juice quality parameters of soybean-sugarcane intercropping at tendaho sugar factory. Biochem. Physiol. 4:1. doi: 10.4172/2168-9652.1000151
Torsvik, V., Øvreås, L., and Thingstad, T.F. (2002). Prokaryotic diversity–magnitude, dynamics, and controlling factors. Science 296, 1064–1066. doi: 10.1126/science.1071698
Tripathi, B. M., Stegen, J. C., Kim, M., Dong, K., Adams, J. M., and Lee, Y. K. (2018). Soil pH mediates the balance between stochastic and deterministic assembly of bacteria. ISME J. 12, 1072–1083. doi: 10.1038/s41396-018-0082-4
Walkley, A., and Black, I. A. (1934). An examination of the Degtjareff method for determining soil organic matter, and a proposed modification of the chromic acid titration method. Soil Sci. 37, 29–38. doi: 10.1097/00010694-193401000-00003
Wang, Q., Garrity, G. M., Tiedje, J. M., and Cole, J. R. (2007). Naive Bayesian classifier for rapid assignment of rRNA sequences into the new bacterial taxonomy. Appl. Environ. Microbiol.73, 5261–5267. doi: 10.1128/AEM.00062-07
Wang, X., Feng, Y., Yu, L., Shu, Y., Tan, F., Gou, Y., et al. (2020). Sugarcane/soybean intercropping with reduced nitrogen input improves crop productivity and reduces carbon footprint in China. Sci. Total Environ. 719:137517. doi: 10.1016/j.scitotenv.2020.137517
Wang, Z. G., Jin, X., Bao, X. G., Li, X. F., Zhao, J. H., Sun, J. H., et al. (2014). Intercropping enhances productivity and maintains the most soil fertility properties relative to sole cropping. PLoS ONE 9:e113984. doi: 10.1371/journal.pone.0113984
Wu, G., Liu, Y., Tian, F., and Shi, Z. (2017). Legumes functional group promotes soil organic carbon and nitrogen storage by increasing plant diversity. Land Degrad. Dev. 28, 1336–1344. doi: 10.1002/ldr.2570
Yang, W., Li, Z., Wang, J., Wu, P., and Zhang, Y. (2013). Crop yield, nitrogen acquisition and sugarcane quality as affected by interspecific competition and nitrogen application. Field Crop Res. 146, 44–50. doi: 10.1016/j.fcr.2013.03.008
Yang, Z., Yang, W., Li, S., Hao, J., Su, Z., Sun, M., et al. (2016). Variation of bacterial community diversity in rhizosphere soil of sole-cropped versus intercropped wheat field after harvest. PLoS ONE 11:e0150618. doi: 10.1371/journal.pone.0150618
Yang, Z. P., Hao, J. M., and Miao, G. Y. (2011). Utilization of mixture cropping to improve immature loess soil (in chinese). Chin J. Appl. Environ. Biol. 17, 388–392. doi: 10.3724/SP.J.1145.2011.00388
Zhang, F., and Li, L. (2003). Using competitive and facilitative interactions in intercropping systems enhances crop productivity and nutrient-use efficiency. Plant Soil 248, 305–312. doi: 10.1023/A:1022352229863
Zhang, M. M., Wang, N., Hu, Y. B., and Sun, G. Y. (2018). Changes in soil physicochemical properties and soil bacterialcommunity in mulberry (Morus alba L.)/alfalfa (MedicagosativaL.) intercropping system. Microbiol. Open 7:e555. doi: 10.1002/mbo3.555
Zhang, T., Wang, Z., Lv, X., Li, Y., and Zhuang, L.i (2019). High-throughput sequencing reveals the diversity and community structure of rhizosphere fungi of Ferula Sinkiangensis at different soil depths. Sci. Rep. 9:6558. doi: 10.1038/s41598-019-43110-z
Keywords: microbial diversity, sugarcane rhizobiome, soil properties, plant growth, high-throughput sequencing
Citation: Malviya MK, Solanki MK, Li C-N, Wang Z, Zeng Y, Verma KK, Singh RK, Singh P, Huang H-R, Yang L-T, Song X-P and Li Y-R (2021) Sugarcane-Legume Intercropping Can Enrich the Soil Microbiome and Plant Growth. Front. Sustain. Food Syst. 5:606595. doi: 10.3389/fsufs.2021.606595
Received: 15 September 2020; Accepted: 19 July 2021;
Published: 21 September 2021.
Edited by:
Jitendra Paul Khurana, University of Delhi, IndiaReviewed by:
Nikolaos Vasileios Paranychianakis, Technical University of Crete, GreeceWenxiong Lin, Fujian Agriculture and Forestry University, China
Copyright © 2021 Malviya, Solanki, Li, Wang, Zeng, Verma, Singh, Singh, Huang, Yang, Song and Li. This is an open-access article distributed under the terms of the Creative Commons Attribution License (CC BY). The use, distribution or reproduction in other forums is permitted, provided the original author(s) and the copyright owner(s) are credited and that the original publication in this journal is cited, in accordance with accepted academic practice. No use, distribution or reproduction is permitted which does not comply with these terms.
*Correspondence: Xiu-Peng Song, xiupengsong@gxaas.net; Yang-Rui Li, liyr@gxaas.net; orcid.org/0000-0002-7559-9244
†These authors have contributed equally to this work