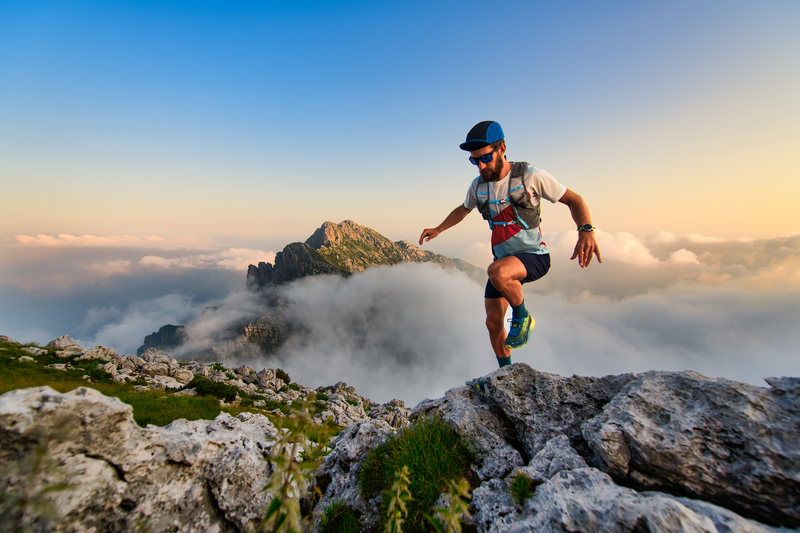
94% of researchers rate our articles as excellent or good
Learn more about the work of our research integrity team to safeguard the quality of each article we publish.
Find out more
ORIGINAL RESEARCH article
Front. Ecol. Evol. , 09 September 2022
Sec. Biogeography and Macroecology
Volume 10 - 2022 | https://doi.org/10.3389/fevo.2022.984842
This article is part of the Research Topic Women in Biogeography and Macroecology 2022 View all 8 articles
Endemic vertebrates are a crucial component of biodiversity, yet face disproportionally high extinction risk as climate and land-use changes drive habitat loss. Large protected areas are therefore deemed necessary to mitigate biodiversity loss. In 2021, China’s Giant Panda National Park (GPNP, 27,134 km2) was established in one of the global endemism hotspots. In this study we ask the question whether this large national park is able to conserve the many threatened endemic vertebrates occurring in the region in the face of climate and land-use changes, in order to assess the long-term effectiveness of the GPNP. We used species distribution modeling techniques to project the distributions of 40 threatened terrestrial (and freshwater) endemic vertebrates under land-use and climate change scenarios SSP2–4.5, SSP3–7.0 and SSP5–8.5 in 2081–2100, and assessed the extent to which their distributions are covered by the GPNP, now and in the future. We found that by 2081–2100, two thirds of the threatened endemic vertebrates are predicted to lose part (15–79%, N = 4) of or (nearly) their entire (80–100% loss, N = 23) range under all three climate and land-use change scenarios. Consequently, fewer species are predicted to occur in the GPNP than at present. Our findings confirm the high vulnerability of threatened endemic species to climate and land-use changes, despite protected areas. Habitat loss due to climate and land-use changes elevate extinction risk of species in endemism hotspots across the globe. Urgent, widespread and intensified mitigation measures and adaptation measures are required at a landscape scale for effective conservation efforts in the future.
Endemism hotpots are valuable in biography as they represent unique aspects of biodiversity (Purvis and Hector, 2000; Isaac et al., 2007; Daru et al., 2020; Murali et al., 2021). They are mostly found on oceanic islands (Kier et al., 2009) and in mountain ranges (Noroozi et al., 2018). Unfortunately, the biophysical features of islands and mountains also make these endemic hotspots highly vulnerable to climate change. Because of spatial isolation and limited surface area of islands and mountain tops, species specialized on these habitat types are constrained in their migration to track their climate envelope, and are confined to smaller and often higher elevation (Dirnböck et al., 2011; Kidane et al., 2019; Veron et al., 2019; Hoffmann et al., 2020). These endemic species therefore face high extinction risks in the face of climate change (Malcolm et al., 2006; Manes et al., 2021). In addition, human activities are projected to substantially alter land cover and pose high extinction risks on endemic species (Kier et al., 2009; Bellard et al., 2014; Jantz et al., 2015; Li et al., 2017; Chaudhary et al., 2018). Endemic species that are already threatened are facing an even higher extinction risk due to expected habitat loss; they are predicted to lose relatively more habitats than non-endemic and non-threatened species (Li et al., 2013). It is therefore important to understand and anticipate the impacts of environmental change on the spatial distributions of threatened endemic species if we want to conserve them and to mitigate biodiversity loss effectively.
Despite the uniqueness of endemic species and the vulnerability of endemic centers to global change, protected areas are not sufficient to conserve the habitats of endemic species (Rodrigues et al., 2004). Not only have endemic species lost major parts of their historical ranges (Brooks et al., 2002), but also remaining endemic centers are in present times largely left unprotected and exposed to high extinction risks (Gonçalves-Souza et al., 2020; Murali et al., 2021). Furthermore, climate change exacerbates the negative impact of habitat destruction and fragmentation on biodiversity, lowering extinction thresholds (Travis, 2003; Mantyka-pringle et al., 2012). Climate change will further reduce the effectiveness of existing protected areas as climatic niches are shifting (Barredoa et al., 2016) and extreme events will be more common. This is especially problematic at high elevations and at locations that provide irreplaceable habitats for threatened species (Hoffmann and Beierkuhnlein, 2020). Protected areas should therefore take the impact of global change into account to effectively conserve biodiversity.
Considerably increasing the acreage of protected areas has been put forward as an integrated solution for climate change mitigation and nature conservation (CBD, 2020; Roberts et al., 2020; Allan et al., 2021). In 2019, China announced a plan to establish ten terrestrial national parks covering a total area of 222,900 km2 (State Forestry and Grassland Administration, 2019). The second largest national park, Giant Panda National Park (GPNP) is officially established in 2021 (Huang et al., 2020; The People’s Government of Sichuan Province, 2021). It covers an area of 27,134 km2, three times the size of Yellow Stone National Park (United States) (Huang et al., 2020). Although the main goal of GPNG is to connect the suitable habitat of the giant panda (Ailuropoda melanoleuca) (Huang et al., 2020), the large surface area of the GPNP harbors at least 3,446 plant and 641 vertebrate species, including large carnivores and many rare and threatened species, and a variety of ecosystems (National Forestry Grassland Administration and National Park Administration, 2019; Tian et al., 2021). Furthermore, the GPNP is located in the northeast of the Hengduan Mountains, one of the global phylogenetic endemism hotspots (Murali et al., 2021). In China, all endemic centers are located in mountainous areas (Tang et al., 2006), where the mammal, bird and seed plant endemism peaks in Hengduan Mountains (Huang et al., 2016; Li and Pimm, 2016; Wu et al., 2017). Based on the International Union for Conservation of Nature’s Red List of Threatened Species (IUCN Red List) (IUCN, 2021b), out of in total 528 endemic terrestrial (and freshwater) vertebrates in China, 183 species (34.7%) are threatened with extinction to a certain extent (i.e., IUCN red list categories critically endangered [CR], endangered [EN], and vulnerable [VU]) (IUCN, 2021a). 40 of these 183 threatened endemic vertebrates (21.9%) live in this area. Thanks to its size, the GPNP might contribute to the conservation of both endemic and endangered species, whose spatial ranges rarely overlap and therefore necessitate large protected areas to protect them (Orme et al., 2005; Tang et al., 2006). In addition, the high topographic complexity in the area of the GPNP might make it a suitable location for biodiversity conservation considering climate change adaptation (Lu et al., 2020).
However, the suitability of the newly established GPNP to conserve threatened endemic species is potentially uncertain in the face of climate change. The focal species of the GPNP, the giant panda, is predicted to face further habitat fragmentation under climate change (Kong et al., 2021), where 26 out of 56 isolated populations would not be protected by the GPNP, and 41 of these 56 populations would face an extinction risk >50% in 100 years (Kong et al., 2021). In the same area, alpine plants are projected to shift upslope and contract their distribution ranges (Ying et al., 2016; He et al., 2019a,b, 2020; Li et al., 2019, but see Liang et al., 2018). For vertebrates, the pattern in distribution range changes is not identical across species (Li et al., 2013; Chen, 2017; Hu et al., 2020; Lu et al., 2020). Several studies assessed the impact of climate change on a number of vertebrates in the region, predicting range loss, range reduction, habitat fragmentation or degradation with a few exceptions of range expansion (Lu et al., 2012; Li et al., 2013, 2021; Lei et al., 2014; Feng et al., 2015; Xu et al., 2020). These studies, however, only considered the possible effect of climate change and not of land-use change. On top of the projected negative impact of climate change on biodiversity, human land-use is expected to further reduce natural vegetation in biodiversity hotspots (Jantz et al., 2015) and compromise the protective effects of protected areas (Montesino Pouzols et al., 2014). Accounting for land use change in predictive studies is therefore essential (Jetz et al., 2007; Tingley et al., 2013). Furthermore, the potential range shifts of many rare vertebrates are understudied, possibly due to data deficiency. The impact of climate and land-use changes on the spatial distributions of threatened endemic vertebrates thus needs to be better understood to assess the conservation effectiveness of protected areas such as the GPNP. This study therefore assesses the impact of climate and land-use changes on the potential spatial distributions of threatened terrestrial (and freshwater) endemic vertebrates in northeast Hengduan Mountains using species distribution modeling techniques, in order to locate the current and future endemism hotspots and to assess the extent to which the GPNP overlaps with (future) hotspots. In doing so, we aim to provide insights in the (future) vulnerability of these species to inform conservation efforts.
The study area spans from 100°50′00″E, 27°00′00″N to 110°00′00″E, 36°00′00″N (Figure 1), in the northeast of Hengduan Mountains, a chain of mountains bordering Qinghai-Tibetan Plateau and Myanmar to its west and Sichuan Basin and Yunnan-Guizhou Plateau to its east. The annual mean temperature ranged from about 5 to 13°C in the start of the 2000s and the annual precipitation ranges from 500 to 1000 mm (Li et al., 2010). More than half of the area is covered by coniferous forests, meadows and steppes, and there are dramatic variations in topography with elevation differences over 6,000 m (Ye et al., 2015). Within the study area, the GPNP is located on the mountain edge northwest to the Sichuan Basin, covering an area of 27,134 km2 (Huang et al., 2020).
Figure 1. Study area and its relative position in China (Hijmans, 2021) (inset). The red contour is the GPNP (Huang et al., 2020).
We selected a set of terrestrial (and freshwater) vertebrates which fulfilled the following criteria: (1) endemic to China; (2) the conservation status was critically endangered (CR), endangered (EN) or vulnerable (VU) according to the IUCN Red List; (3) the study area covered at least 30% of the species’ current range or the species’ current range covered at least 30% of the study area; (4) occurrence data were either available, or could be geographically referenced from published maps/sightings, or the IUCN published range was available. A total of 40 species fulfilled all four criteria according to the IUCN Red List (IUCN, 2021b) and literature review (Figure 2 and Table 1) and were thus included in our study for further assessment.
Figure 2. Selection process and the number of studied species. Selection based on type and conservation status was done using information from the IUCN; overlap with the study area was checked either via online available occurrence data, published maps, or the IUCN published range.
The occurrence data were coordinates either directly obtained or georeferenced from published maps, or sampled (following complete spatial randomness, sampling 1,200 points per species to provide sufficient information about its presence) from published polygons of species ranges (Table 1). The Global Biodiversity Information Facility (GBIF1) provided coordinates for six bird species and five amphibian species, using all available data due to scarcity of records. Coordinates were also found for one bird species from literature (Lu et al., 2012). Georeferenced locations were used for one mammal (Li et al., 2018, 2021). Furthermore, distribution ranges published by the IUCN were downloaded for all 40 species. As a result, there were in total 52 occurrence datasets for 40 species.
The sample size of occurrence data was set to be at least 11, as this was found to be the minimum needed to generate accurate distributions for species of low prevalence (i.e., 5% of raster cells where the species is present) (van Proosdij et al., 2016). The directly obtained and georeferenced coordinates for studied species were all below 5% prevalence in the study area, which was as expected considering they were both endemic and threatened.
Previous studies revealed important environmental variables for 23 of the 40 species and distribution range predictions for 24 species which were, when possible, included in this study (Supplementary Appendix I). For the other species, no earlier study was found. In spite of the fact that for species with a narrow niche, microhabitat conditions could be of vital importance [i.e., water velocity for Scutiger liupanensis (Zuo et al., 2017)], this study focused on generic bioclimatic, land-use, topographic and lithological variables due to constraints on data accessibility. In addition, including microhabitat variables would require a finer scale which was not possibly due to unavailability of fine scale bioclimatic and land-use variables. 19 bioclimatic variables were obtained from WorldClim version 22, 14 land-use variables were obtained from Land-Use Harmonization2 (LUH23), three topographic factors were calculated based on a digital elevation model downloaded from DIVA-GIS4, namely slope, aspect, and normalized topographic position index (Normalized TPI). Normalized topographic index measures the difference between elevation at the central point and the average elevation within a predetermined radius surrounding it (de Reu et al., 2013). Normalized TPI measures the topographic position as a fraction of local relief normalized to the standard deviation of the elevation (de Reu et al., 2013). Another three topographic factors were computed from inland water (rivers and lakes) and road maps obtained from DIVA-GIS and converted to distance maps, and lithology was sourced from literature (Hartmann and Moosdorf, 2012a; Table 2). Lithology describes the geochemical, mineralogical, and physical properties of rocks (Hartmann and Moosdorf, 2012b). It is a key factor in fields like landscape evolution, river chemical composition, matter supply to ecosystems, etc. (Hartmann and Moosdorf, 2012b) and was therefore included as a potential predictor variable in the models. The lithological variable contained 13 rock types in the study area ranked by ascending resistance to weathering and erosion (Dürr et al., 2005). It was used as a continuous variable representing the gradient of rocks’ sensitivity to weathering and erosion.
To identify the current (near historic) distribution ranges, we used bioclimatic and land-use variables from the period of 1970–2000, both available at a resolution of 30 arcseconds (approximately 0.8 km by 0.8 km in the study area). The future period under consideration was 2081–2100 for bioclimatic and land-use predictor variables, at a resolution of 2.5 arcminute (approximately 4 km by 4 km in the study area) and 30 arcseconds respectively. Unfortunately, 30 arcsecond data were not available for future bioclimatic variables. We therefore downscaled the data from 2.5 arcminutes to 30 arcseconds to match the resolution of the other variables. Because the land-use projections were available for each year in each period, we averaged the grid-cell values across years to match the averages as provided for the same periods for the bioclimatic variables. Topographic (available at a resolution of 30 arcseconds) and lithological variables (available at a resolution of 30 arcminutes) were kept constant between current and future periods.
Three scenarios of bioclimatic variables were used from the general circulation model The Beijing Climate Center Climate System Model (BCC-CSM2-MR), since its focus is on East Asia (Wu et al., 2019), namely the Shared Socioeconomic Pathways (SSP) 2–4.5, SSP3–7.0 and SSP5–8.5 (IPCC, 2021). Under SSP2–4.5, the most optimistic scenario in this study, CO2 emission will be around the current level until 2050 after which it declines to net zero by 2100. Compared to 1850–1900, the global annual mean temperature is estimated to be between 2.1 to 3.5°C higher in 2081–2100 (IPCC, 2021). The average annual global land precipitation is projected to increase with 1.5–8% relative to 1995–2014, with stronger seasonality in more areas (IPCC, 2021). An important feature in global land-use change is the initial decrease in forest with about 43 million ha from 2000 to 2050, with a subsequent increase in forest with about 331 million ha from 2050 to 2100, resulting in a positive forest cover gain by 2100 (Hurtt et al., 2020). Under SSP3–7.0, CO2 emission will double by 2100 and global annual mean temperature in the years 2081–2100 is estimated to be about 2.8 to 4.6°C higher compared to the years 1850–1900 (IPCC, 2021). The SSP3–7.0 scenario features the highest rate of net land transition globally, with a strong expansion of crop and pasture land and large-scale deforestation (Hurtt et al., 2020). Under the SSP5–8.5, the worst emission scenario in this study, CO2 emission will triple by 2075 and the global annual mean temperature is estimated to be 3.3 to 5.7°C higher by the end of this century compared to 1850–1900 (IPCC, 2021). Precipitation is projected to increase with 1–13% by 2081–2100 relative to 1995–2014 with stronger seasonality in more areas (IPCC, 2021). This scenario predicts a strong expansion of cropland on the expense of pasture and forest land and large increases in irrigated area (Hurtt et al., 2020).
Data analysis was conducted in three phases (Figure 3). In the pre-processing phase, occurrence data were set to coordinate system (WSG84). For the 12 species for which we were able to obtain GBIF data or (georeferenced) occurrences from literatures, the spatial extents for generating the random pseudo-absences were the intersections of the study area and the concave shapes which enclosed all sample points, calculated in R studio (RStudio Team, 2021) using packages ‘sf’ (Pebesma, 2018) and ‘concaveman’ (Gombin et al., 2020). The spatial extents for pseudo-absence data that were extracted from IUCN ranges for all 40 species were the intersections of the study area and the occurrence ranges plus buffer zones which were calculated in the ‘rgeos’ package (Bivand and Rundel, 2021). The width of the buffer zone equaled the diameter of a circle which had the same surface area as the occurrence range. The sizes of the buffer zones were therefore species-dependent. They were designed to create adequate contrast between presence and pseudo-absence locations, i.e., areas in which species may be present and in which pseudo-absences are meaningful. This allows Maxent to better determine variable importance and relation with species presence, while avoiding AUC inflation (Barve et al., 2011; Barbet-Massin et al., 2012).
After the occurrence and random pseudo-presence data were collected, all coordinates (those obtained from the IUCN ranges as well as those from GBIF data and [georeferenced] occurrences from literatures) were cleaned by removing duplicates and records with little accuracy (i.e., fewer than two decimal places), and thinned to a 30 arcsecond resolution grid. The remaining number of occurrences per species is the sample size. Afterward, the pseudo-absence points were sampled within the above-described spatial extents and in the same sample size as the occurrences for each species.
Environmental variables were first set to the same temporal (Current = 1970–2000, future = 2081–2100) and, species specific spatial extents (see above), and then standardized to zero mean and unit variance. Topographical predictor variables were calculated in R studio (RStudio Team, 2021) using packages ‘raster’ (Hijmans, 2022), ‘spatialEco’ (Evans, 2021), and ‘sf’ (Pebesma, 2018). To shorten computation time, distances to road, river and lake were calculated in 2.5 arcminute resolution, which was aggregated and then rescaled to 30 arcsecond via bilinear interpolation after the calculation. Afterward, to reduce the multicollinearity among predictor variables, a number of environmental variables were selected per species within its spatial extent. Variable selection was based on the variance inflation factor (VIF) they expressed. The predictor variable that had the highest VIF was chosen from a cluster of correlated predictor variables (correlation coefficient > | 0.5|), as variable representing the group of correlated variables. The remaining level of multicollinearity between kept predictors was assess using VIF as calculated with the ‘usdm’ package (Naimi et al., 2014) in R studio (RStudio Team, 2021). The choice of correlation coefficient | 0.5| as threshold for predictor variable clustering was motivated by otherwise serious multicollinearity issues which would be encountered in the processing phase and the resulted indeterministic models.
In the processing phase, the maximum entropy algorithm (Maxent) (Phillips et al., 2006) was fitted to relate species occurrences to a selection of environmental variables, where we used the implementation of the ‘sdm’ package (Naimi and Araújo, 2016) in R studio (RStudio Team, 2021). Compared to other species distribution modeling algorithms, Maxent has shown good performance across sample sizes including small data sets (Wisz et al., 2008). Ten-fold cross-validation was used for occurrence datasets with more than 100 occurrence points (Naimi et al., 2014). For samples that were smaller than 100 points (in practice less than 77 points), leave-one-out cross-validation was used since ten-fold cross validation is less appropriate for datasets with limited occurrences (Pearson et al., 2007). Using leave-one-out cross-validation maximizes the use of limited data for training and equalizes the contribution of each point in testing.
For model fitting we used the ‘sdm’ function from the ‘sdm’ package (Naimi and Araújo, 2016) in R studio (RStudio Team, 2021). The model fitting started with all predictor variables. Then, variable importance and exclusion was evaluated based on Pearson correlation between the fitted values and the predictions when the focal variable was randomly permutated 5 times using the ‘getVarImp’ function from the ‘sdm’ package in R (Thuiller et al., 2009; Naimi and Araújo, 2016). The lower the correlation, and thus the higher “1-correlation,” the more important the variable was to the model (Thuiller et al., 2009; Naimi and Araújo, 2016). Changing the values of an independent variable and assessing the sensitivity of the predictions using a correlation based variable importance metric may highlight more transferable variables, which are important for the purpose of distribution projection under future environmental conditions (Phillips, 2017). Using a large range of variables that may show high collinearity in models requires a priori variable selection (Braunisch et al., 2013; Muscatello et al., 2021). We therefore implemented the model fitting process as an iteration which removed the least relevant variable(s) from the fitted model and then repeated model fitting until only two predictor variables were left in the model following methods proposed by Zeng et al. (2016). This process therefore yielded a series of models with a different number of explanatory variables, of which the first model always included all predictor variables and the last model only included two predictor variables. The models were subsequently assessed in the post-processing phases to determine the best model for each species. The model fitting and modification process was repeated three times with different random realizations to permutate variable importance for each occurrence dataset. We repeated the model fitting and modification process to assess the robustness of the results, assuming robustness if the three series of models that were generated produced similar results. As a result, for each occurrence dataset, the processing phase produced three sets of fitted models.
Model fitting was proceeded by model selection, prediction of current and future species distributions, and computation of the fraction of species ranges that were covered by the GPNP. Model selection was based on two criteria. First, the three fitted models from three model modification runs had to consist of the same explanatory variables. Secondly, the AUC score evaluated on the test datasets and the number of predictors in a model had to fulfill the requirement of model parsimony. In practice, models with AUC value +0.2 per additional predictor were selected, i.e., if, for a particular species, model 1 had 4 predictor variables and an AUC of 0.80 and model 2 had 5 predictor variables and an AUC of 0.82, we would select model 2 to be the better model. If for a species all models had AUC values <0.7, the projections made by this model were considered with low confidence. Because predictor variable elimination in the pre-processing phase was likely to capture the major variances in the environmental conditions but not necessarily the required niche by the species, models for species with small occurrence record datasets (<100) were indeterministic where each model modification process generated models with different predictors. For this reason, model selection was based on the consistency of models besides AUC scores and the number of predictors.
Finally, after the best model was selected for each species (given the above-listed criteria), probability maps of species presence were generated for the present time and for the future under three climate and land-use scenarios. For each species we thus had three probability maps for the present time and nine probability maps for the future (three times three scenarios). These maps were converted to binomial habitat suitability maps with a threshold value specified per model [i.e., Maximized sum of sensitivity and specificity (Nenzén and Araújo, 2011; Liu et al., 2016)]. We therefore generated three presence-absence maps from three simulation runs per species. Then, these three binary maps were overlayed and the intersection became the presence in a combined presence-absence map for each species. The approach we took was that if a grid-cell had a value of 1 in all three probability maps, that particular grid-cell would receive a 1 in the final suitability map. If on the other hand it only had a 1 in two out of three probability maps, it would receive a 0 in the final suitability map. A Pairwise Wilcoxon Rank Sum Test in R studio (RStudio Team, 2021) was used to assess if species range sizes changed significantly. Afterward, 40 binomial maps were summed up to produce a set of congruent suitability maps for the present and under each future scenario, where the value of a grid cell can range between 0 to 40, indicating the number of species which may occur in each cell. The current and future maps were compared to assess species distribution range changes and changes in endemism hotspots. Finally, the congruent suitability maps were overlayed with the GPNP to assess conservation coverage for now and for the future. QGIS (QGIS.org, 2022) and R studio (RStudio Team, 2021) were used for analyses.
The remaining environmental variables kept for prediction all had a VIF < 2, thus the kept predictors had very low levels of multicollinearity. Out of the 40 selected models, 31 models generated species presence-absence maps with a high confidence level (average AUC ≥ 0.7 and comparable projections across three simulation runs). The other 9 models generated species distribution projections with a low confidence level either because of low average AUC scores (<0.7) and/or because of less similar projections from three simulation runs (see Supplementary Appendix II for model per species and model statistics).
A range of variables were important in explaining the distribution ranges of the species assessed and the most important explanatory variable was generally species specific (see Supplementary Appendix II). Regarding all species together, the explanatory variable that most frequently explaining their geographic distribution was a land-use variable: the mean age of secondary land. It was one of the most important explanatory variables for 8 out of 40 species. The mean age of secondary land is predicted to decrease in most areas in and around the Sichuan Basin except for in the north where it is predicted to increase. The precipitation of the driest quarter was one of the most important explanatory variables for 7 out of 40 species. The precipitation of the driest quarter is predicted to increase in the southeast of the study area in future and decrease in the rest of the region. The species distributions mostly responded to both variables unimodally with a species-specific threshold. Furthermore, distance to the nearest lake (negative relationship with area suitability) and lithology also both appeared as in the most important explanatory variables for 6 species.
For most amphibians (6 out of 24), the mean age of secondary land was one of the most important explanatory predictor variables in which the response was usually unimodally and varied per species. For most birds (3 out of 13) the precipitation of the driest quarter was one of the most important predictor variables in which suitability increased with an increasing amount of precipitation during the driest quarter of the year until a species specific optimum after which suitability declined again. Mammals’ distributions were predicted by different environmental variables per species. Lithology was an important predictor for the distribution ranges of both reptiles; the suitability for both species to occur was highest in areas with rock types that were medium resistant to weathering and erosion. Precipitation seasonality was an important predictor variable for the Sichuan rat snake (Euprepiophis perlacea), in which probability of its occurrence first increased with increasing precipitation seasonality until a peak at 0.75 (coefficient of variation) after which it declined. Distance to the nearest lake was important for the Wa Shan Keelback (Hebius metusium) in which the probability of its occurrence declined with an increasing distance to the nearest lake.
At present, 37 out of 40 threatened endemic vertebrates in the region are present in the GPNP. However, climate and land use change is predicted to significantly alter the range size of the species assessed in future (Pairwise Wilcoxon rank sum test p < 0.001 for all scenarios [SSP2–4.5, SSP3–7.0, and SSP5–8.5]). More than half of the evaluated species (23 out of 40 species, 57.5%) is projected to lose between 80 to 100% of their current range under all three future scenarios. 78.0% of evaluated birds, more than half of the evaluated amphibians, and half of the evaluated mammals and reptiles are predicted to lose more than 80% of their suitable ranges (Supplementary Appendix III). Four species (10.0%) are predicted to lose between 15 and 80% of their ranges (Figure 4 and Supplementary Appendix III). Depending on the climate change scenario either only 17 or only 18 of these species can still occur in the GPNP. Three species are expected to maintain their current ranges (7.5%, i.e., range changes between −15 and 15%), and six species (15.0%, all amphibians) are projected to expand their distribution ranges (i.e., range changes >15%).
Figure 4. Percentage change in suitable habitat size per species under (A) SSP2–4.5, (B) SSP3–7.0, and (C) SSP5–8.5. The species are 1, O. multipunctatus; 2, S. liupanensis; 3, O. omeimontis; 4, A. loloensis; 5, S. wanglangensis; 6, S. muliensis; 7, S. chintingensis; 8, S. tuberculatus; 9, H. metusium; 10, G. sukatschewi; 11, T. wenxianensis; 12, T. pseudoverrucosus; 13, P. puxiongensis; 14, P. bedfordi; 15, L. omeiensis; 16, T. taliangensis; 17, R. rex; 18, O. puxiongensis; 19, L. boringii; 20, S. zappeyi; 21, O. chuanbeiensis; 22, A. rufipectus; 23, O. kuangwuensis; 24, P. internigrans; 25, B. londongensis; 26, A. melanoleuca; 27, B. pinchonii; 28, B. tibetanus; 29, E. perlacea; 30, L. lhuysii; 31, O. liangbeiensis; 32, O. nanjiangensis; 33, O. pingii; 34, R. roxellana; 35, S. variegaticeps; 36, S. jiulongensis; 37, S. pingwuensis; 38, S. reevesii; 39, S. przewalskii; 40, M. nankiangensis.
The impact of climate change on the extent of range size change of the species assessed is generally consistent across the three different future scenarios (Pairwise Wilcoxon rank sum test p > 0.950).
Since it is predicted that most species will contract their ranges in future, the hotspots of threatened endemic species in the study area, and in the GPNP, will also contract. Currently, the hotspots are located in the mountain ranges to the northwest and the southwest of the Sichuan basin, where a maximum of 18 evaluated species can co-occur (Figure 5A). By the end of this century, no region in the study area would possess the climatic and land-use conditions to support more than 10 threatened endemic vertebrates to occur simultaneously (Figures 5B–D). Regarding the GPNP, about 70% of the area is currently suitable to simultaneously host five to ten of the species included in our study (Figure 6A). In 2081–2100 this number is predicted to have been reduced to one to three species regardless the future scenario (Figures 6B–D).
Figure 5. Projections of suitable habitats for 40 threatened endemic species (A) at present (i.e., 1970–2000) and in 2081–2100 under climatic and land-use scenario (B) SSP3–4.5, (C) SSP3–7.0, and (D) SSP5–8.5, in overlap with the GPNP. Each map is an overlay of 40 presence-absence maps of 40 species. The color scale indicates the number of species that is expected to (co-)occur in the region. The blue contour indicates the borders of the GPNP.
Figure 6. Density plot of number of co-occurring species in GPNP (A) at present and under scenario (B) SSP2–4.5, (C) SSP3–7.0, and (D) SSP5–8.5 in 2018–2100.
Endemic vertebrates are a crucial component of biodiversity because of their unique and sometimes long evolutional histories (Purvis and Hector, 2000; Isaac et al., 2007; Murali et al., 2021). Unfortunately, endemic vertebrates are facing disproportionally high extinction risks due to climate and land-use change related habitat loss (Kier et al., 2009; Dirnböck et al., 2011; Bellard et al., 2014; Manes et al., 2021). Large protected areas are deemed necessary to halt biodiversity loss (CBD, 2020; Allan et al., 2021), however, their effectiveness in biodiversity conservation is uncertain because of global change (Hoffmann and Beierkuhnlein, 2020) and the subsequent biodiversity redistribution (Pecl et al., 2017). To enhance long-term conservation effectiveness of protected areas it is important to get a better understanding of the impact of future environmental change on the distribution of threatened endemic species. In this paper, we quantified the extent with which a large protected area (Giant Panda National Park, GPNP, part of the Hengduan Mountains, China) in a global endemism hotspot (Murali et al., 2021) will cover (part of) the future distribution ranges of 40 threatened endemic vertebrates. At present, the GPNP covers part of the endemism hotspot located in the northwest of the Sichuan Basin, and covers (part of) the distribution ranges of 37 out of the 40 threatened endemic vertebrates included in our study. This is because the distribution range of the Giant panda overlaps with the distribution ranges of a high portion of endemic vertebrates in China (Li and Pimm, 2016). The conservation of the pandas’ habitat thus provides umbrella protection to the species that co-occur in the area (Li and Pimm, 2016).
We found that by 2081–2100, more than half of the studied species is projected to lose 80 to 100% of their current range due to climate and land use changes. Range loss was generally associated with a combination of a predicted reduction of precipitation in the driest quarter in large parts of the study region and the predicted declining mean age of secondary land in future. Changes in the mean age of secondary land appeared to be the most important driver of range loss for amphibians, closely followed by changes in the precipitation regime during the driest quarter of the year. Amphibians are declining worldwide due to a variety of reasons (Beebee and Griffiths, 2005). Secondary land has more often been found to support high numbers of amphibians (Thompson and Donnelly, 2018); destruction of and changes to secondary land are therefore likely to cause changes in geographic ranges of amphibians. Furthermore, changes in the precipitation regime have also been mentioned as likely having large impacts on amphibians. The Sichuan rat snake was predicted to lose its entire range, likely due to shifts in precipitation seasonality. The Wa Shan Keelback on the other hand was predicted to maintain its entire range.
Also other studies suggest that climate and non-climate threats threaten amphibians and birds in the Hengduan Mountains (Foden et al., 2013), and that habitat alteration is the foremost driver of extinction risk for threatened terrestrial vertebrates (Ducatez and Shine, 2016). We for instance predict that the suitable range from a species like the Kuang-wu Shan frog (Odorrana kuangwuensis) will (nearly) entirely disappear in future. Suitability for the species to occur was predicted by, amongst others a positive relationship with the mean age of secondary land. The species’ habitat, mountain streams and their surroundings, is currently mostly threatened by the removal of large rocks and boulders that are used for construction purposes (Fei et al., 2012). Based on our results, added land use changes will likely form an additional threat to the species persistence. Another species that is likely to lose large parts of its range in future based on our results is the Sichuan partridge (Arborophila rufipectus). Areas it currently inhibits are, amongst others, associated with, for the area, medium to large amounts of precipitation during the driest quarter of the year. Climate change is thus expected to negatively affect the species if it is not able to disperse or adapt [also see Lei et al. (2014)]. The extinction risk for such species is therefore high and our analyses suggests that the current endemic vertebrate hotspot may disappear in 60–80 years’ time. Few species (all amphibians) were expected to increase their range in future. However, other aspects not taken into account may still negatively affect species. For instance, a species like the Lolokou sucker frog (Amolops loloensis), which has shown adaptability to past climatic changes, may be negatively impacted by hydropower development in the region (Gong et al., 2020). Considering the predicted fate of all species assessed together, the conservation effectiveness of the GPNP is expected to decrease over time as the endemism hotspot disappears in future, with only a maximum of 18 assessed species being predicted to still occur in the GPNP by the end of the century. The diminishing conservation effectiveness of the GPNP is not surprising considering the fact that the primary objective of the GPNP is to improve the connectivity of current habitats for the Giant panda (Huang et al., 2020), which may not be aligned with facilitating species conservation in general. The role of the Giant panda as umbrella species and the justification for placing a disproportionally high conservation effort on the species may need re-evaluation in the face of global change.
That the effectiveness of the GPNP, a large protected area for biodiversity conservation, is forecasted to decline is consistent with findings for other protected areas in Europe (Araújo et al., 2011), but in contrast with findings in southern Africa (Thuiller et al., 2006; Hoveka et al., 2022). This may be explained by species’ exposure to hazards (i.e., extrinsic factors such as climate change and human disturbances) rather than by species’ sensitivity and adaptive capacity to hazards (i.e., intrinsic factors of vulnerability) (Foden et al., 2013, 2019). Although the Hengduan Mountains likely served as a refugium during climatic changes in the past and maintained high diversity and endemism, this region is predicted to experience large changes in climatic conditions and land use (Wu et al., 2017; Enquist et al., 2019; Brown et al., 2020). The protected areas in the Hengduan Mountains and in Europe are projected to experience larger climate anomalies compared to protected areas in southern Africa (Hoffmann and Beierkuhnlein, 2020). In addition, both our study area and Europe have a relatively high human footprint index compared to southern Africa (Venter et al., 2016).
Due to the rarity of the species included in this study, one major challenge we encountered was that little data and information were available and accessible regarding these species. Few to no studies could be found for more than one third of the species studied. We therefore deliberately chose to use all available sources for species occurrences (i.e., IUCN, GBIF, georeferencing), and to select the most performant model from models generated from these different datasets. GBIF data were available for only 11 out of 40 species considered, but occurrences were limited (<70). The uncertainty in these datasets was therefore high as occurrence records in GBIF are likely to be spatially biased toward better-surveyed areas which may lead to inaccurate models (Tessarolo et al., 2021). As a result, only for one species the best performing model was based on the GBIF dataset. Similarly, also the dataset constructed from georeferenced coordinates yielded the best performing model for only one species. For all the other species the best performing model was based on occurrences extracted from IUCN ranges. Lack of sufficient trustworthy occurrence data may thus undermine efforts to identify ecologically meaningful variables and predict distribution ranges. However, even though we focused on rare species with only scare data, our models had a high confidence for 31 out of 40 species. Four of the nine species for which we were unable to generate a decent model have small distribution ranges. We were unable to extract a sufficient amount of occurrence records from their ranges and their models thus all suffered from low AUC scores (<0.7). This outcome illustrates that adequate survey data can be crucial for reliably modeling species distributions [but see Pearson et al. (2007)], especially when the study landscape is complex and heterogenous. Alternatively, besides the inadequacy of survey data, this finding may indicate that the processes behind these species’ distributions were not captured faithfully by our models, for example because we did not explicitly account for functional connectivity and thus reachability of habitat (Kool et al., 2012; Villard and Metzger, 2014; Brennan et al., 2022).
Despite the uncertainty in the occurrence data and the scarce species-specific information, the results of our study are consistent with global evaluations (Foden et al., 2013; Manes et al., 2021). Our findings highlight the vulnerability of threatened endemic vertebrates to environmental change in an important biodiversity hotspot, and this hotspot is predicted to disappear in future, despite a large protected area. These results suggest that protected areas alone will not be able to counter-balance human-induced species extinctions (Pimm et al., 2014; Ceballos et al., 2020). Indeed, human pressures have increased inside protected areas worldwide (Geldmann et al., 2019). In that light, our study also illustrates the importance of taking potential land-use changes into account next to climate change when modeling species distributions (Wisz et al., 2008). Land-use change and intensification can aggravate the negative impacts of climate change on biodiversity conservation as human activities compete for suitable habitat, reduce migration connectivity, and intensify the impacts of extreme climate events and disturbance regimes (de Chazal and Rounsevell, 2009; Gimona et al., 2012; Oliver and Morecroft, 2014; Jantz et al., 2015; Tang et al., 2020). While human land-use intensifies, adaptive measures require a landscape approach which engages multiple stakeholders, reconciles competing ecosystem services, and enables participatory and adaptive management to be successful (Sayer et al., 2013). Multiple techniques can assist its spatial design (e.g., Moilanen et al., 2011; Mastrantonis et al., 2022), but more importantly, the paradigm shift from project-oriented to process-oriented, from top-down to bottom-up approaches can only take place if it is supported by the local social and political context (Biesbroek et al., 2010; Voß and Bornemann, 2011; Patrick Bixler et al., 2015). Conservation measures targeted at individual species such as captive breeding and assisted migration, although successful on project level, will not effectively mitigate global biodiversity loss given the rapid deteriorating status of vertebrates (Butchart et al., 2006; Hoffmann et al., 2010; Ceballos et al., 2020). If we want to save at least some of the threatened endemic vertebrates, prompt, widespread and throughout mitigation measures must considerably reduce the exposure of endemism hotspots to both climate change as well as land-use change within this century. Urgent intensification of (inter)national climate change policies are necessary to lessen climate anomalies, and land-use policies on a much larger scale than protected areas are required to curb human disturbances.
The original contributions presented in this study are included in the article/Supplementary material, further inquiries can be directed to the corresponding author/s.
DW, HK, and AH contributed to conception and design of the study. DW organized the database, performed the statistical analysis, and wrote the first draft of the manuscript. All authors contributed to manuscript revision, read, and approved the submitted version.
We would like to thank FV and GC for their time and efforts in providing valuable feedback and in doing so contributing to improving this manuscript.
The authors declare that the research was conducted in the absence of any commercial or financial relationships that could be construed as a potential conflict of interest.
All claims expressed in this article are solely those of the authors and do not necessarily represent those of their affiliated organizations, or those of the publisher, the editors and the reviewers. Any product that may be evaluated in this article, or claim that may be made by its manufacturer, is not guaranteed or endorsed by the publisher.
The Supplementary Material for this article can be found online at: https://www.frontiersin.org/articles/10.3389/fevo.2022.984842/full#supplementary-material
Allan, J. R., Possingham, H. P., Atkinson, S. C., Waldron, A., di Marco, M., Adams, V. M., et al. (2021). The minimum land area requiring conservation attention to safeguard biodiversity. bioRxiv [Preprint]. doi: 10.1101/839977v2
Araújo, M. B., Alagador, D., Cabeza, M., Nogués-Bravo, D., and Thuiller, W. (2011). Climate change threatens European conservation areas. Ecol. Lett. 14, 484–492. doi: 10.1111/j.1461-0248.2011.01610.x
Barbet-Massin, M., Jiguet, F., Albert, C. H., and Thuiller, W. (2012). Selecting pseudo-absences for species distribution models: how, where and how many? Methods Ecol. Evol. 3, 327–338. doi: 10.1111/j.2041-210X.2011.00172.x
Barredoa, J. I., Caudulloa, G., and Dosiob, A. (2016). Mediterranean habitat loss under future climate conditions: assessing impacts on the Natura 2000 protected area network. Appl. Geogr. 75, 83–92.
Barve, N., Barve, V., Jiménez-Valverde, A., Lira-Noriega, A., Maher, S. P., Peterson, A. T., et al. (2011). The crucial role of the accessible area in ecological niche modeling and species distribution modeling. Ecol. Model. 222, 1810–1819.
Beebee, T. J. C., and Griffiths, R. A. (2005). The amphibian decline crisis: a watershed for conservation biology? Biol. Conserv. 125, 271–285.
Bellard, C., Leclerc, C., Leroy, B., Bakkenes, M., Veloz, S., Thuiller, W., et al. (2014). Vulnerability of biodiversity hotspots to global change. Glob. Ecol. Biogeogr. 23, 1376–1386. doi: 10.1111/geb.12228
Biesbroek, G. R., Swart, R. J., Carter, T. R., Cowan, C., Henrichs, T., Mela, H., et al. (2010). Europe adapts to climate change: comparing national adaptation strategies. Glob. Environ. Change 20, 440–450.
Bivand, R., and Rundel, C. (2021). rgeos: Interface to Geometry Engine - Open Source (‘GEOS’). R package version 0.5-8.
Braunisch, V., Coppes, J., Arlettaz, R., Suchant, R., Schmid, H., and Bollmann, K. (2013). Selecting from correlated climate variables: a major source of uncertainty for predicting species distributions under climate change. Ecography 36, 971–983.
Brennan, A., Naidoo, R., Greenstreet, L., Mehrabi, Z., Ramankutty, N., and Kremen, C. (2022). Functional connectivity of the world’s protected areas. Science 376, 1101–1104. doi: 10.1126/science.abl8974
Brooks, T. M., Mittermeier, R. A., Mittermeier, C. G., da Fonseca, G. A. B., Rylands, A. B., Konstant, W. R., et al. (2002). Habitat loss and extinction in the hotspots of biodiversity. Conserv. Biol. 16, 909–923. doi: 10.1046/j.1523-1739.2002.00530.x
Brown, S. C., Wigley, T. M. L., Otto-Bliesner, B. L., Rahbek, C., and Fordham, D. A. (2020). Persistent Quaternary climate refugia are hospices for biodiversity in the Anthropocene. Nat. Clim. Change 10, 244–248.
Butchart, S. H. M., Stattersfield, A. J., and Collar, N. J. (2006). How many bird extinctions have we prevented? Oryx 40, 266–278. doi: 10.1017/S0030605306000950
CBD (2020). Post-2020 Global Biodiversity Framework: Scientific and Technical Information to Support the Review of the Updated Goals and Targets, and Related Indicators and Baselines. Proposed Indicators and Monitoring Approach for the Post-2020 Global Biodiversity Framework. Note by the Executive Secretary. Dubai: CBD.
Ceballos, G., Ehrlich, P. R., and Raven, P. H. (2020). Vertebrates on the brink as indicators of biological annihilation and the sixth mass extinction. Proc. Natl. Acad. Sci. U.S.A. 117, 13596–13602. doi: 10.1073/pnas.1922686117
Chaudhary, A., Pourfaraj, V., and Mooers, A. O. (2018). Projecting global land use-driven evolutionary history loss. Divers. Distrib. 24, 158–167. doi: 10.1111/ddi.12677
Chen, Y. (2017). Amphibian Distributions and Extinction Risk in China Under Climate and Land Use Change. Doctor of Philosophy thesis. Edmonton: University of Alberta.
Daru, B. H., Farooq, H., Antonelli, A., and Faurby, S. (2020). Endemism patterns are scale dependent. Nat. Commun. 11, 1–11.
de Chazal, J., and Rounsevell, M. D. A. (2009). Land-use and climate change within assessments of biodiversity change: a review. Glob. Environ. Change 19, 306–315.
de Reu, J., Bourgeois, J., Bats, M., Zwertvaegher, A., Gelorini, V., de Smedt, P., et al. (2013). Application of the topographic position index to heterogeneous landscapes. Geomorphology 186, 39–49. doi: 10.1016/j.scitotenv.2021.147955
Dirnböck, T., Essl, F., and Rabitsch, W. (2011). Disproportional risk for habitat loss of high-altitude endemic species under climate change. Glob. Change Biol. 17, 990–996. doi: 10.1111/j.1365-2486.2010.02266.x
Ducatez, S., and Shine, R. (2016). Drivers of extinction risk in terrestrial vertebrates. Conserv. Lett. 10, 186–194.
Dürr, H. H., Meybeck, M., and Dürr, S. H. (2005). Lithologic composition of the Earth’s continental surfaces derived from a new digital map emphasizing riverine material transfer. Glob. Biogeochem. Cycles 19:2515.
Enquist, B. J., Feng, X., Boyle, B., Maitner, B., Newman, E. A., Jørgensen, P. M., et al. (2019). The commonness of rarity: global and future distribution of rarity across land plants. Sci. Adv. 5:eaaz0414. doi: 10.1126/sciadv.aaz0414
Fei, L., Ye, C., and Jiang, J. (2012). Colored Atlas of Chinese Amphibians and Their Distributions. Chengdu: Sichuan Publishing House of Science and Technology.
Feng, X., Lin, C., Qiao, H., and Ji, L. (2015). Assessment of climatically suitable area for Syrmaticus reevesii under climate change. Endang. Spec. Res. 28, 19–31.
Fick, S. E., and Hijmans, R. J. (2017). WorldClim 2: new 1km spatial resolution climate surfaces for global land areas. Int. J. Climatol. 37, 4302–4315.
Fleming, M. D., and Hoffer, R. M. (1979). “Machine processing of landsat MSS data and DMA topographic data for forest cover type mapping,” in Proceedings of the LARS Symposium on Machine Processing of Remotely Sensed Data, West Lafayette.
Foden, W. B., Butchart, S. H. M., Stuart, S. N., Vié, J. C., Akçakaya, H. R., Angulo, A., et al. (2013). Identifying the world’s most climate change vulnerable Species: a systematic trait-based assessment of all birds, amphibians and corals. PLoS One 8:e65427. doi: 10.1371/journal.pone.0065427
Foden, W. B., Young, B. E., Akçakaya, H. R., Garcia, R. A., Hoffmann, A. A., Stein, B. A., et al. (2019). Climate change vulnerability assessment of species. Wiley Interdiscip. Rev. Clim. Change 10:e551. doi: 10.1002/wcc.551
GBIF.org (2021d). GBIF Occurrence Download. Available online at: https://doi.org/10.15468/dl.dtptkp (accessed November 2, 2021).
GBIF.org (2021b). GBIF Occurrence Download. Available online at: https://doi.org/10.15468/dl.6k4dhd (accessed November 2, 2021).
GBIF.org (2021e). GBIF Occurrence Download. Available online at: https://doi.org/10.15468/dl.eedz74 (accessed November 2, 2021).
GBIF.org (2021i). GBIF Occurrence Download. Available online at: https://doi.org/10.15468/dl.r8rfy7 (accessed November 2, 2021).
GBIF.org (2021k). GBIF Occurrence Download. Available online at: https://doi.org/10.15468/dl.ztjkx9 (accessed November 2, 2021).
GBIF.org (2021h). GBIF Occurrence Download. Available online at: https://doi.org/10.15468/dl.k5w8ff (accessed November 2, 2021).
GBIF.org (2021j). GBIF Occurrence Download. Available online at: https://doi.org/10.15468/dl.raq7fv (accessed November 2, 2021).
GBIF.org (2021g). GBIF Occurrence Download. Available online at: https://doi.org/10.15468/dl.hcnpk4 (accessed November 2, 2021).
GBIF.org (2021a). GBIF Occurrence Download. Available online at: https://doi.org/10.15468/dl.2drw2m (accessed November 2, 2021).
GBIF.org (2021f). GBIF Occurrence Download. Available online at: https://doi.org/10.15468/dl.gp3rjc (accessed November 2, 2021).
GBIF.org (2021c). GBIF Occurrence Download. Available online at: https://doi.org/10.15468/dl.85rgqq (accessed November 2, 2021).
Geldmann, J., Manica, A., Burgess, N. D., Coad, L., and Balmford, A. (2019). A global-level assessment of the effectiveness of protected areas at resisting anthropogenic pressures. Proc. Natl. Acad. Sci. U.S.A. 116, 23209–23215. doi: 10.5061/dryad.p8cz8w9kf
Gimona, A., Poggio, L., Brown, I., and Castellazzi, M. (2012). Woodland networks in a changing climate: threats from land use change. Biol. Conserv. 149, 93–102.
Gombin, J., Vaidyanathan, R., and Agafonkin, V. (2020). concaveman: A Very Fast 2D Concave Hull Algorithm. R package version 1.1.0.
Gonçalves-Souza, D., Verburg, P. H., and Dobrovolski, R. (2020). Habitat loss, extinction predictability and conservation efforts in the terrestrial ecoregions. Biol. Conserv. 246:108579.
Gong, M., Shafer, A. B. A., Hu, X., Huang, Y., Zhang, L., Li, H., et al. (2020). Population demographic history and adaptability of the vulnerable Lolokou Sucker Frog. Genetica 148, 207–213. doi: 10.1007/s10709-020-00105-3
Hartmann, J., and Moosdorf, N. (2012a). Global Lithological Map Database v1.0 (gridded to 0.5° spatial resolution).
Hartmann, J., and Moosdorf, N. (2012b). The new global lithological map database GLiM: a representation of rock properties at the Earth surface. Geochem. Geophys. Geosyst. 13:12004. doi: 10.1029/2012GC004370
He, X., Burgess, K. S., Gao, L. M., and Li, D. Z. (2019a). Distributional responses to climate change for alpine species of Cyananthus and Primula endemic to the Himalaya-Hengduan Mountains. Plant Divers. 41, 26–32. doi: 10.1016/j.pld.2019.01.004
He, X., Burgess, K. S., Yang, X. F., Ahrends, A., Gao, L. M., and Li, D. Z. (2019b). Upward elevation and northwest range shifts for alpine Meconopsis species in the Himalaya–Hengduan Mountains region. Ecol. Evol. 9, 4055–4064. doi: 10.1002/ece3.5034
He, Y., Xiong, Q., Yu, L., Yan, W., and Qu, X. (2020). Impact of climate change on potential distribution patterns of alpine vegetation in the Hengduan Mountains Region, China. Source Mount. Res. Dev. 40, 48–54.
Hijmans, R. J. (2021). Spatial Data Download | DIVA-GIS. Available online at: http://diva-gis.org/datadown (accessed December 1, 2021).
Hoffmann, D., de Vasconcelos, M. F., and Wilson Fernandes, G. (2020). The fate of endemic birds of eastern Brazilian mountaintops in the face of climate change. Perspect. Ecol. Conserv. 18, 257–266.
Hoffmann, M., Hilton-Taylor, C., Angulo, A., Böhm, M., Brooks, T. M., Butchart, S. H. M., et al. (2010). The impact of conservation on the status of the world’s vertebrates. Science 330, 1503–1509. doi: 10.1126/science.1194442
Hoffmann, S., and Beierkuhnlein, C. (2020). Climate change exposure and vulnerability of the global protected area estate from an international perspective. Divers. Distrib. 26, 1496–1509. doi: 10.1111/ddi.13136
Hoveka, L. N., van der Bank, M., and Davies, T. J. (2022). Winners and losers in a changing climate: how will protected areas conserve red list species under climate change? Divers. Distrib. 28, 782–792. doi: 10.1111/ddi.13488
Hu, R., Gu, Y., Luo, M., Lu, Z., Wei, M., and Zhong, J. (2020). Shifts in bird ranges and conservation priorities in China under climate change. PLoS One 15:e0240225. doi: 10.1371/journal.pone.0240225
Huang, J., Huang, J., Liu, C., Zhang, J., Lu, X., and Ma, K. (2016). Diversity hotspots and conservation gaps for the Chinese endemic seed flora. Biol. Conserv. 198, 104–112.
Huang, Q., Fei, Y., Yang, H., Gu, X., and Songer, M. (2020). Giant Panda National Park, a step towards streamlining protected areas and cohesive conservation management in China. Glob. Ecol. Conserv. 22:e00947.
Hurtt, G. C., Chini, L., Sahajpal, R., Frolking, S., Bodirsky, B. L., Calvin, K., et al. (2020). Harmonization of Global Land-Use Change and Management for the Period 850-2100 (LUH2) for CMIP6. Göttingen: Geoscientifc Model Development.
IPCC (2021). “Summary for policymakers,” in Climate Change 2021: The Physical Science Basis. Contribution of Working Group I to the Sixth Assessment Report of the Intergovernmental Panel on Climate Change, eds V. Masson-Delmotte, P. Zhai, A. Pirani, S. L. Connors, C. Péan, S. Berger, et al. (Geneva: IPCC).
Isaac, N. J. B., Turvey, S. T., Collen, B., Waterman, C., and Baillie, J. E. M. (2007). Mammals on the EDGE: conservation priorities based on threat and phylogeny. PLoS One 2:e296. doi: 10.1371/journal.pone.0000296
IUCN (2021d). The IUCN Red List of Threatened Species. Version 2021-3 [cited 2022 Dec 1]. Gland: IUCN.
IUCN (2021c). The IUCN Red List of Threatened Species. Version 2021-3 [cited 2022 Mar 1]. Gland: IUCN.
Jantz, S. M., Barker, B., Brooks, T. M., Chini, L. P., Huang, Q., Moore, R. M., et al. (2015). Future habitat loss and extinctions driven by land-use change in biodiversity hotspots under four scenarios of climate-change mitigation. Conserv. Biol. 29, 1122–1131. doi: 10.1111/cobi.12549
Jetz, W., Wilcove, D. S., and Dobson, A. P. (2007). Projected impacts of climate and land-use change on the global diversity of birds. PLoS Biol. 5:e157. doi: 10.1371/journal.pbio.0050157
Kidane, Y. O., Steinbauer, M. J., and Beierkuhnlein, C. (2019). Dead end for endemic plant species? A biodiversity hotspot under pressure. Glob. Ecol. Conserv. 19:e00670.
Kier, G., Kreft, H., Lee, T. M., Jetz, W., Ibisch, P. L., Nowicki, C., et al. (2009). A global assessment of endemism and species richness across island and mainland regions. PNAS 106, 9322–9327. doi: 10.1073/pnas.0810306106
Kong, L., Xu, W., Xiao, Y., Pimm, S. L., Shi, H., and Ouyang, Z. (2021). Spatial models of giant pandas under current and future conditions reveal extinction risks. Nat. Ecol. Evol. 5, 1309–1316. doi: 10.1038/s41559-021-01520-1
Kool, J. T., Moilanen, A., and Treml, E. A. (2012). Population connectivity: recent advances and new perspectives. Landsc. Ecol. 28, 165–185. doi: 10.1007/s10980-012-9819-z
Lei, J., Xu, H., Cui, P., Guang, Q., and Ding, H. (2014). The potential effects of climate change on suitable habitat for the Sichuan hill partridge (Arborophila rufipectus, Boulton): based on the maximum entropy modelling. Polish J. Ecol. 62, 771–787. doi: 10.3161/104.062.0419.full
Li, B., Pimm, S. L., Li, S., Zhao, L., and Luo, C. (2017). Free-ranging livestock threaten the long-term survival of giant pandas. Biol. Conserv. 216, 18–25.
Li, B. V., and Pimm, S. L. (2016). China’s endemic vertebrates sheltering under the protective umbrella of the giant panda. Conserv. Biol. 30, 329–339. doi: 10.1111/cobi.12618
Li, J., Li, D., Xue, Y., Wu, B., He, X., and Liu, F. (2018). Identifying potential refugia and corridors under climate change: a case study of endangered Sichuan golden monkey (Rhinopithecus roxellana) in Qinling Mountains, China. Am. J. Primatol. 80:e22929. doi: 10.1002/ajp.22929
Li, P., Zhu, W., Xie, Z., and Qiao, K. (2019). Integration of multiple climate models to predict range shifts and identify management priorities of the endangered Taxus wallichiana in the Himalaya–Hengduan Mountain region. J. For. Res. 31, 2255–2272. doi: 10.1007/s11676-019-01009-5
Li, X., Tian, H., Wang, Y., Li, R., Song, Z., Zhang, F., et al. (2013). Vulnerability of 208 endemic or endangered species in China to the effects of climate change. Region. Environ. Change 13, 843–852.
Li, X., Zhao, X., and Li, M. (2021). Future effects of climate change and human footprint on the geographical distribution of three snub-nosed monkeys in China. Acta Theriol. Sin. 41, 310–321.
Li, Z., He, Y., Xin, H., Wang, C., Jia, W., Zhang, W., et al. (2010). Spatio-temporal variations of temperature and precipitation in Mts. Hengduan Region during 1960-2008. Acta Geogr. Sin. 65, 563–579.
Liang, J. (2019). The Relationship Between the Spatial Pattern of Pterodactyl Species Diversity and Environmental Factors in Guizhou Province. Master degree thesis. Guiyang: Guizhou Normal University.
Liang, Q., Xu, X., Mao, K., Wang, M., Wang, K., Xi, Z., et al. (2018). Shifts in plant distributions in response to climate warming in a biodiversity hotspot, the Hengduan Mountains. J. Biogeogr. 45, 1334–1344. doi: 10.1111/jbi.13229
Liu, C., Newell, G., and White, M. (2016). On the selection of thresholds for predicting species occurrence with presence-only data. Ecol. Evol. 6, 337–348. doi: 10.1002/ece3.1878
Lu, N., Jing, Y., Lloyd, H., and Sun, Y. H. (2012). Assessing the distributions and potential risks from climate change for the Sichuan jay (Perisoreus internigrans). Condor 114, 365–376.
Lu, Y., Xu, P., Li, Q., Wang, Y., and Wu, C. (2020). Planning priority conservation areas for biodiversity under climate change in topographically complex areas: a case study in Sichuan province, China. PLoS One 15:e0243425. doi: 10.1371/journal.pone.0243425
Malcolm, J. R., Liu, C., Neilson, R. P., Hansen, L., and Hannah, L. (2006). Global warming and extinctions of endemic species from biodiversity hotspots. Conserv. Biol. 20, 538–548. doi: 10.1111/j.1523-1739.2006.00364.x
Manes, S., Costello, M. J., Beckett, H., Debnath, A., Devenish-Nelson, E., Grey, K. A., et al. (2021). Endemism increases species’ climate change risk in areas of global biodiversity importance. Biol. Conserv. 257:109070.
Mantyka-pringle, C. S., Martin, T. G., and Rhodes, J. R. (2012). Interactions between climate and habitat loss effects on biodiversity: a systematic review and meta-analysis. Glob. Change Biol. 18, 1239–1252. doi: 10.1111/j.1365-2486.2011.02593.x
Mastrantonis, S., Craig, M. D., Hobbs, R. J., Grigg, A. H., and Renton, M. (2022). Identifying optimal solutions between competing economic and conservation land use objectives for species that require widely distributed resources. Environ. Model. Softw. 148:105292.
Moilanen, A., Anderson, B. J., Eigenbrod, F., Heinemeyer, A., Roy, D. B., Gillings, S., et al. (2011). Balancing alternative land uses in conservation prioritization. Ecol. Appl. 21, 1419–1426. doi: 10.1890/10-1865.1
Montesino Pouzols, F., Toivonen, T., di Minin, E., Kukkala, A. S., Kullberg, P., Kuusterä, J., et al. (2014). Global protected area expansion is compromised by projected land-use and parochialism. Nature 516, 383–386. doi: 10.1038/nature14032
Murali, G., Gumbs, R., Meiri, S., and Roll, U. (2021). Global determinants and conservation of evolutionary and geographic rarity in land vertebrates. Sci. Adv. 7:eabe5582. doi: 10.1126/sciadv.abe5582
Muscatello, A., Elith, J., and Kujala, H. (2021). How decisions about fitting species distribution models affect conservation outcomes. Conserv. Biol. 35, 1309–1320. doi: 10.1111/cobi.13669
Naimi, B., and Araújo, M. B. (2016). sdm: a reproducible and extensible R platform for species distribution modelling. Ecography 39, 368–375.
Naimi, B., Hamm, N. A. S., Groen, T. A., Skidmore, A. K., and Toxopeus, A. G. (2014). Where is positional uncertainty a problem for species distribution modelling? Ecography 37, 191–203.
National Forestry Grassland Administration and National Park Administration (2019). The Overall Development Plan of Giant Panda National Park.
Nenzén, H. K., and Araújo, M. B. (2011). Choice of threshold alters projections of species range shifts under climate change. Ecol. Model. 222, 3346–3354. doi: 10.1016/j.ecolmodel.2011.07.011
Noroozi, J., Talebi, A., Doostmohammadi, M., Rumpf, S. B., Linder, H. P., and Schneeweiss, G. M. (2018). Hotspots within a global biodiversity hotspot - areas of endemism are associated with high mountain ranges. Sci. Rep. 8, 1–10.
Oliver, T. H., and Morecroft, M. D. (2014). Interactions between climate change and land use change on biodiversity: attribution problems, risks, and opportunities. Wiley Interdiscip. Rev. Clim. Change 5, 317–335.
Orme, C. D. L., Davies, R. G., Burgess, M., Eigenbrod, F., Pickup, N., Olson, V. A., et al. (2005). Global hotspots of species richness are not congruent with endemism or threat. Nature 436, 1016–1019.
Patrick Bixler, R., Dell’Angelo, J., Mfune, O., and Roba, H. (2015). The political ecology of participatory conservation: institutions and discourse. J. Polit. Ecol. 22, 164–182.
Pearson, R. G., Raxworthy, C. J., Nakamura, M., and Townsend Peterson, A. (2007). Predicting species distributions from small numbers of occurrence records: a test case using cryptic geckos in Madagascar. J. Biogeogr. 34, 102–117. doi: 10.1111/j.1365-2699.2006.01594.x
Pebesma, E. (2018). Simple features for R: standardized support for spatial vector data. R J. 10, 439–446.
Pecl, G. T., Araújo, M. B., Bell, J. D., Blanchard, J., Bonebrake, T. C., Chen, I. C., et al. (2017). Biodiversity redistribution under climate change: impacts on ecosystems and human well-being. Science 355:eaai9214. doi: 10.1126/science.aai9214
Phillips, S. B., Aneja, V. P., Kang, D., and Arya, S. P. (2006). “Modelling and analysis of the atmospheric nitrogen deposition in North Carolina,” in International Journal of Global Environmental Issues, ed. M. A. Dorgham (Geneva: Inderscience Publishers), 231–252.
Phillips, S. J. (2017). A Brief Tutorial on Maxent. Available online at: http://biodiversityinformatics.amnh.org/open_source/maxent/ (accessed November 2, 2021).
Pimm, S. L., Jenkins, C. N., Abell, R., Brooks, T. M., Gittleman, J. L., Joppa, L. N., et al. (2014). The biodiversity of species and their rates of extinction, distribution, and protection. Science 344:1246752. doi: 10.1126/science.1246752
Ritter, P. A. (1987). Vector-based slope and aspect generation algorithm. Photogrammetr. Eng. Remote Sens. 53, 1109–1111.
Roberts, C. M., O’Leary, B. C., and Hawkins, J. P. (2020). Climate change mitigation and nature conservation both require higher protected area targets. Philos. Trans. R. Soc. B 375:20190121. doi: 10.1098/rstb.2019.0121
Rodrigues, A. S. L., Andelman, S. J., Bakarr, M. I., Boitani, L., Brooks, T. M., Cowling, R. M., et al. (2004). Effectiveness of the global protected area network in representing species diversity. Nature 428, 640–643.
Sayer, J., Sunderland, T., Ghazoul, J., Pfund, J. L., Sheil, D., Meijaard, E., et al. (2013). Ten principles for a landscape approach to reconciling agriculture, conservation, and other competing land uses. Proc. Natl. Acad. Sci. U.S.A. 110, 8349–8356. doi: 10.1073/pnas.1210595110
State Forestry and Grassland Administration (2019). China Will Establish National Parks in 2020. Available online at: http://www.gov.cn/xinwen/2019-10/18/content_5441647.htm (accessed August 30, 2021).
Tang, F., Fu, M., Wang, L., and Zhang, P. (2020). Land-use change in Changli County, China: predicting its spatio-temporal evolution in habitat quality. Ecol. Indic. 117:106719.
Tang, Z., Wang, Z., Zheng, C., and Fang, J. (2006). Biodiversity in China’s mountains. Front. Ecol. Environ. 4, 347–352.
Tessarolo, G., Ladle, R. J., Lobo, J. M., Rangel, T. F., and Hortal, J. (2021). Using maps of biogeographical ignorance to reveal the uncertainty in distributional data hidden in species distribution models. Ecography 44, 1743–1755.
The People’s Government of Sichuan Province (2021). Giant Panda National Park is Officially Established!. Available online at: https://www.sc.gov.cn/10462/12771/2021/10/12/fb884dd92def4831bf9369adb542f1a6.shtml (accessed March 31, 2022).
Thompson, M. E., and Donnelly, M. A. (2018). Effects of secondary forest succession on amphibians and reptiles: a review and meta-analysis. Copeia 106, 10–19.
Thuiller, W., Lafourcade, B., Engler, R., and Araújo, M. B. (2009). BIOMOD - A platform for ensemble forecasting of species distributions. Ecography 32, 369–373.
Thuiller, W., Midgley, G. F., Hughes, G. O., Bomhard, B., Drew, G., Rutherford, M. C., et al. (2006). Endemic species and ecosystem sensitivity to climate change in Namibia. Glob. Change Biol. 12, 759–776. doi: 10.1111/j.1365-2486.2006.01140.x
Tian, J., Zhu, S., Zhang, X., He, L., Gu, X., Guan, T., et al. (2021). The diversity of large- and medium-sized terrestrial mammals and birds in the Giant Panda National Park: a review based on camera-trapping data. Biodivers. Sci. 29, 1490–1504. doi: 10.17520/biods.2021165
Tingley, M. W., Estes, L. D., and Wilcove, D. S. (2013). Climate change must not blow conservation off course. Nature 500, 271–272.
Travis, J. M. J. (2003). Climate change and habitat destruction: a deadly anthropogenic cocktail. Proc. R. Soc. Lond. B Biol. Sci. 270, 467–473. doi: 10.1098/rspb.2002.2246
van Proosdij, A. S. J., Sosef, M. S. M., Wieringa, J. J., and Raes, N. (2016). Minimum required number of specimen records to develop accurate species distribution models. Ecography 39, 542–552.
Venter, O., Sanderson, E. W., Magrach, A., Allan, J. R., Beher, J., Jones, K. R., et al. (2016). Global terrestrial Human Footprint maps for 1993 and 2009. Sci. Data 3, 1–10. doi: 10.1038/sdata.2016.67
Veron, S., Mouchet, M., Govaerts, R., Haevermans, T., and Pellens, R. (2019). Vulnerability to climate change of islands worldwide and its impact on the tree of life. Sci. Rep. 9, 1–14.
Villard, M. A., and Metzger, J. P. (2014). REVIEW: beyond the fragmentation debate: a conceptual model to predict when habitat configuration really matters. J. Appl. Ecol. 51, 309–318. doi: 10.1111/1365-2664.12190
Voß, J. P., and Bornemann, B. (2011). The politics of reflexive governance: challenges for designing adaptive management and transition management. Ecol. Soc. 16:9.
Wang, B., Xu, Y., and Ran, J. (2017). Predicting suitable habitat of the Chinese monal (Lophophorus lhuysii) using ecological niche modeling in the Qionglai Mountains, China. PeerJ 5:e3477. doi: 10.7717/peerj.3477
Wisz, M. S., Hijmans, R. J., Li, J., Peterson, A. T., Graham, C. H., Guisan, A., et al. (2008). Effects of sample size on the performance of species distribution models. Divers. Distrib. 14, 763–773.
Wu, T., Lu, Y., Fang, Y., Xin, X., Li, L., Li, W., et al. (2019). The Beijing climate center climate system model (BCC-CSM): the main progress from CMIP5 to CMIP6. Geosci. Model Dev. 12, 1573–1600.
Wu, Y., DuBay, S. G., Colwell, R. K., Ran, J., and Lei, F. (2017). Mobile hotspots and refugia of avian diversity in the mountains of south-west China under past and contemporary global climate change. J. Biogeogr. 44, 615–626. doi: 10.1111/jbi.12862
Xu, Y., Wang, B., Zhong, X., Yang, B., Zhang, J., Zhao, C., et al. (2020). Predicting range shifts of the Chinese monal (Lophophorus lhuysii) under climate change: implications for long-term conservation. Glob. Ecol. Conserv. 22:e01018.
Ye, X., Liu, G., Li, Z., Wang, H., and Zeng, Y. (2015). Assessing local and surrounding threats to the protected area network in a biodiversity hotspot: the hengduan mountains of Southwest China. PLoS One 10:e0138533. doi: 10.1371/journal.pone.0138533
Ying, L., Liu, Y., Chen, S., and Shen, Z. (2016). Simulation of the potential range of Pistacia weinmannifolia in Southwest China with climate change based on the maximum-entropy (Maxent) model. Biodivers. Sci. 24:453. doi: 10.17520/biods.2015246
Zeng, Y., Low, B. W., and Yeo, D. C. J. (2016). Novel methods to select environmental variables in MaxEnt: a case study using invasive crayfish. Ecol. Model. 341, 5–13.
Zuo, J. (2018). The Population Ecology and Species Conservation of Scutiger Liupanensis. Lanzhou: Northwest Normal University.
Zuo, J., Pang, D., Li, L., and Huang, Q. (2017). “Spring habitat characteristics of Scutiger liupanensis in Liupanshan National Forest Park, Ningxia,” in Proceedings of the 13th National Wildlife and Resource Conservation Academic Seminar and the 6th Zoology of Western China Academic Seminar, Geneva.
Keywords: climate change, Giant Panda National Park, habitat loss, Hengduan mountains, endemism hotspot, land-use, Maxent, threatened endemic vertebrates
Citation: Wang D, de Knegt HJ and Hof AR (2022) The effectiveness of a large protected area to conserve a global endemism hotspot may vanish in the face of climate and land-use changes. Front. Ecol. Evol. 10:984842. doi: 10.3389/fevo.2022.984842
Received: 02 July 2022; Accepted: 23 August 2022;
Published: 09 September 2022.
Edited by:
Mirko Di Febbraro, University of Molise, ItalyReviewed by:
Gianpasquale Chiatante, University of Pavia, ItalyCopyright © 2022 Wang, de Knegt and Hof. This is an open-access article distributed under the terms of the Creative Commons Attribution License (CC BY). The use, distribution or reproduction in other forums is permitted, provided the original author(s) and the copyright owner(s) are credited and that the original publication in this journal is cited, in accordance with accepted academic practice. No use, distribution or reproduction is permitted which does not comply with these terms.
*Correspondence: Danyang Wang, RGFueWFuZy53YW5nLjIwMTFAZ21haWwuY29t
Disclaimer: All claims expressed in this article are solely those of the authors and do not necessarily represent those of their affiliated organizations, or those of the publisher, the editors and the reviewers. Any product that may be evaluated in this article or claim that may be made by its manufacturer is not guaranteed or endorsed by the publisher.
Research integrity at Frontiers
Learn more about the work of our research integrity team to safeguard the quality of each article we publish.