- 1Department of Biology, Fordham University, Bronx, NY, United States
- 2Department of Biology, University of Maryland, College Park, College Park, MD, United States
- 3Migratory Bird Center, Smithsonian Conservation Biology Institute, Smithsonian Institution, Washington, DC, United States
- 4Calder Biological Field Station, Fordham University, Armonk, NY, United States
- 5Department of Biology and McCourt School of Public Policy, Georgetown University, Washington, DC, United States
Free-ranging domestic cats are a detriment to wildlife and humans by preying on native species and transmitting disease. As a result, removing free-ranging cats from the landscape has become a conservation and public health priority. Estimating cat population size with an unbiased sampling design, however, especially in human-dominated areas, is logistically challenging and rarely done. The lack of robust cat population sampling limits our understanding of where cats pose risks, which is important for evaluating management strategies, such as trap-remove or trap-neuter-return. We hypothesized that cat abundance and activity both depend on human land use and demographics. Using a network of sites participating in a community science program, we conducted transect and camera trap surveys to test predictions of cat population abundance and activity across a gradient of residential land use intensity. Both sampling methods determined that cat abundance was greatest in areas with intermediate human population density and lower educational attainment. Transect data also provided evidence that cat abundance was greatest at intermediate levels of impervious surface cover (e.g., road and buildings), while data from camera traps also showed that cat abundance was positively associated with household income. Using counts of cats observed on cameras, we found that the timing of cat activity varied depending on the degree of urban intensity. Cats were more strictly nocturnal in medium and high intensity residential land-use areas, possibly because a greater proportion of these cats are unowned or because they avoid human activity. These results suggest that transect surveys conducted during the day may undercount cats in urban environments where unowned free-ranging cats predominate. Taken together, our results highlight the importance of incorporating human demographics, land use patterns, and urban context in estimating the abundance of free-ranging cats to better inform management decisions and improve conservation outcomes.
Introduction
Free-ranging domestic cats cause serious ecological damage and can be hazardous to human health. Cats have caused the extinction and extirpation of native wildlife (Medina et al., 2011; Doherty et al., 2016; Greenwell et al., 2019) and represent a major source of mortality for wildlife populations (Loss et al., 2013; Loss and Marra, 2017). Cats are also the definitive host for the protozoan parasite Toxoplasma gondii (Frenkel et al., 1970), which causes serious human health problems, including miscarriages, schizophrenia, and increased risk of suicide (Sahwi et al., 1995; Brown et al., 2005; Ling et al., 2011). As the negative effects of cats are better understood, there has been an increased call to remove free-ranging cats from landscapes (Winter, 2004; Nogales et al., 2013; Doherty et al., 2016; Crawford et al., 2019). Accurate data on cat abundance, including how cats are distributed across heterogeneous human-dominated landscapes, are necessary for developing effective management strategies.
As owned and unowned populations often occupy the same landscape, enumerating free-ranging cats presents a distinct challenge. The majority of survey efforts, particularly those conducted using social surveys, have largely focused on the owned population (Lepczyk et al., 2004; Baker et al., 2008; Sims et al., 2008; Murray et al., 2010; Thomas et al., 2012) and often yield conflicting results. For example, Murray et al. (2010) surveyed over 3,000 households in the United Kingdom and reported higher cat abundance in rural and semi-urban areas than in urban areas whereas Sims et al. (2008) and Thomas et al. (2012) surveyed over 1,500 and 2,000 United Kingdom households, respectively, and reported an increase in owned cat density with increased housing density. Such disparities could reflect differences amongst cat owners in the propensity to give cats outdoor access or have them sterilized (e.g., Childs, 1990; Murray et al., 2015). Moreover, because both owned and unowned cats negatively impact ecosystems (Blancher, 2013; Loss et al., 2013), further work is needed to understand abundance patterns across ownership classes and geographic contexts.
Recognizing the limitation of social surveys for estimating free-ranging cat abundance, researchers have implemented other approaches. For example, Schmidt et al. (2007b) used mark-recapture and distance sampling to estimate the abundance of free-ranging cats in an urban landscape in Caldwell, TX, United States, and demonstrated that distance sampling provides adequate estimates of free-ranging cat abundance in urban areas. Because their study did not include land use covariates, however, their estimates are not directly applicable across heterogeneous urban landscapes. Davis and Lepczyk (2010) used distance sampling transects at known cat aggregations to estimate cat abundance in a population characterized by clusters of individuals at feeding locations. Elizondo and Loss (2016) evaluated cat abundance across a gradient of local urban land use intensity using camera traps at 15 sites in Stillwater, OK, United States, but did not observe variation in abundance across the gradient. The authors recommended surveying cats across more sites and a wider range of the urban land use gradient. Cove et al. (2018) used an extensive camera network at over 100 sites to survey cat populations on public lands in the Florida Keys and were able to generate robust population density, activity, and movement estimates. However, cats also frequently use privately owned residential, commercial, and industrial areas. Flockhart et al. (2016), conducted 145 repeated distance sampling transect surveys across various land-use types in Guelph, ON, Canada and reported that cat density was highest in residential areas and increased with increased building density and distance from wooded areas. They argued these relationships are most likely a consequence of cat ownership and the dependence of urban cat populations on anthropogenic resources (Tennent and Downs, 2008; Normand et al., 2019). The same study also reported greater cat abundance in areas with lower household income, consistent with past research showing that cat abundance is greater in areas of lower socioeconomic status (Calhoon and Haspel, 1989; Finkler et al., 2011).
Behavioral patterns of cats and their owners may also affect our ability to detect cats on surveys. Cats vary their activity levels depending on weather (Churcher and Lawton, 1987; Haspel and Calhoon, 1993) and time of day (Konecny, 1987; Haspel and Calhoon, 1993; Alterio and Moller, 1997; Horn et al., 2011; Kays et al., 2015; Cove et al., 2018). No information exists, however, on variation in the timing of cat activity across an urban gradient. This is potentially a major gap in knowledge because surveys conducted at the same times assume similar activity rates across sites (see Flockhart et al., 2016), which may not be a safe assumption. For example, Kays et al. (2015) reported that cats in eastern United States urban yards tended to be more diurnal than those in forested protected areas. Horn et al. (2011) reported that unowned cats in Urbana-Champaign, IL, United States, were more strictly nocturnal than owned cats. Similarly, Cove et al. (2018) identified two distinct activity regimes among cat populations in the Florida Keys, United States, with owned cats most active at dawn and dusk and unowned cats predominantly nocturnal. These behavioral differences may temporally bias cat survey efforts if owned and unowned cats are unevenly distributed across the urban gradient.
Our objective was to characterize the abundance and activity patterns of domestic cats across a gradient of residential land use intensity (hereafter, referred to as urban intensity and the urban gradient). We hypothesized that cat abundance and activity depend on human land-use and demographics. Considering the results of Flockhart et al. (2016), we predicted that cat abundance would increase with impervious surface coverage [e.g., roads, buildings, and parking lots (Yang et al., 2003)] and human population density, two indicators of urban intensity, and decrease with median income and mean education level, two indicators of socioeconomic status (Calhoon and Haspel, 1989; Finkler et al., 2011; Flockhart et al., 2016). Given previous findings that owned cats are more likely to be observed during the day (Haspel and Calhoon, 1993; Cove et al., 2018) and the propensity to allow owned cats outdoors varies with urban context (Clancy et al., 2003; Lepczyk et al., 2004), we predicted that patterns of daily cat activity varies across the urban gradient, specifically that cats would be more active at night in high urban intensity areas relative to low and medium urban intensity environments.
Materials and Methods
Site Selection
This study was conducted between June and August of 2016 in the greater Washington, DC, United States, metropolitan area (Supplementary Figure 1). Study sites were considered to comprise a 100-m radius circle geographically centered at private homes (n = 48) or apartment complexes (n = 5) whose owner, tenant, or manager participated in the Neighborhood Nestwatch program (hereafter, NN), a community science program with 280 participants in the region (Evans et al., 2005). The study site area of 3.14 ha is consistent with estimates of cat home range size in residential areas (Haspel and Calhoon, 1989; Barratt, 1997; Schmidt et al., 2007a; Kays et al., 2019).
We obtained land cover data from the Multi-Resolution Land Cover Characteristics Consortium (Yang et al., 2018) and human demographic data from the United States Census Bureau’s 2014 American Community Survey (Walker, 2016; Glenn, 2019). We used the raster package (Hijmans, 2020) in R (R Core Team, 2019) to determine the mean percentage of impervious surface cover within a 100-m radius of the participants’ homes. Impervious surface correlates strongly with urban land cover (Evans et al., 2015, appendix A) and is associated with a number of environmental and ecological indicators (Schiff and Benoit, 2007; Evans et al., 2015). From the Census data, we extracted the median household income, percent of population to obtain at least an associate degree, and population density at the level of a census tract (see Flockhart et al., 2016).
We selected a subset of NN sites that maximized the evenness of the impervious surface distribution and filtered sites such than none of the 100-m radius circles were overlapping. We evaluated whether our sites were representative of the urban gradient in our study region using a two-tailed Kolmogorov–Smirnov test. We compared the proportion of impervious surface cover at our sites with a random sample of 1,000 locations within our study extent. Impervious surface at our sites was not significantly different from the region at large (P = 0.12).
Data Collection
We sampled cats using distance sampling transects and motion-sensing camera traps. The cat population in our study area was assumed to be demographically closed. This assumption is supported by previous research on the survival of free-ranging cats. For example, Schmidt et al. (2007a) reported survival among feral, semi-feral, and owned free-ranging cats to be 56, 90, and 100%, respectively, over a 14-month period – this corresponds to estimated survival rates of 87, 97, and 100% over our 3-month study period. We could not account for the possibility of a cat leaving or arriving in the vicinity of a study site over the course of the study (see Kilgour et al., 2017); however, because cats frequently to maintain an established home range (e.g., Haspel and Calhoon, 1989; Barratt, 1997; Schmidt et al., 2007a; Kays et al., 2019), we judged this unlikely to meaningfully affect our final results. To avoid over-counting cats, individuals that could not be separated based on markings or physical characteristics were considered to be the same cat, even in ambiguous cases. Because the ability to detect cats may differ seasonally, our sampling schedule was designed to avoid temporal bias. Each camera was deployed for a total of 3 weeks at each site, divided into three 1-week deployments that were spread over the 3 months of the study. The order of site visits was randomized within months. To avoid clustering visits temporally, we required at least a 1-week gap between sampling events.
During distance sampling counts, an observer walked 200 m of road along a straight-line transect with its center in front of an NN house or apartment. The boundaries of the transect were determined using digital orthophotos. The observer counted any visible cats and estimated their perpendicular distance from the transect line (see Buckland et al., 2001). Each transect was sampled six times, and the same observer conducted every count. Distance sampling assumes transects are placed randomly with respect to the distribution of the sampled individuals, though this assumption is violated due to the necessity of sampling along roads. Though other similar studies have faced this issue (Schmidt et al., 2007b; Flockhart et al., 2016), the consequences of the violation of this assumption on abundance estimates are not clear. We used a second detection method, motion-sensor camera traps, in part to understand possible biases in transect sampling of human-dominated environments.
We deployed one Reconyx PC800 Hyperfire (Holmen, WI, United States) motion-sensor camera per site at all but five sites (n = 48) to survey cats. Sites without cameras were homeowners that declined to participate in this aspect of the program. We set cameras approximately 0.5 m above the ground and programmed them to take five photographs when triggered, with no delay period between triggers. We did not change the position of cameras between deployments, with one exception due to a vehicle obstructing the camera area. Camera trapping assumes that cats are distributed randomly with respect to the camera. Cameras were generally deployed to capture an open yard area, so detection was not influenced by known cat aggregations.
Statistical Analysis of Abundance
We estimated free-ranging cat abundance for transect and camera data using the R package unmarked (Fiske and Chandler, 2011). Following Chandler et al. (2011), abundance in the transect area was modeled as the mean of a negative binomial random variable, λ. An individual may not have been available for detection at a given sampling occasion at some probability, ϕ. Because owned cats may have been kept inside the home during the survey, time of day was included as a covariate of ϕ. An individual available during a survey was detected at probability p, calculated by integrating over a hazard rate detection function that decreases with distance from the observer. Due to potential temporal and weather-related differences in cat activity, survey date, time, temperature, and dew point were included as covariates of p (Supplementary Table 1). We obtained temperature and dew point using the data reported on the Weather Underground Weather History and Data Archive (Weather Underground, 2016) from the closest available weather station at the nearest hour to the time of the survey.
We used N-mixture models to estimate abundance from camera data (Royle, 2004). N-mixture models produce more reliable estimates of relative abundance than absolute abundance (Barker et al., 2018) but have been found to perform equivalently to other common approaches (Ficetola et al., 2018). Abundance was modeled as the number of trials of a binomial probability distribution, λ, given the probability of detection, p. Each day of a camera’s deployment was considered a sampling instance. This approach allowed us to examine detection covariates at a finer scale than a full week. We considered date, daily high temperature, low temperature, high dew point, and low dew point as covariates for p (Supplementary Table 1). Abundance and detection variables were scaled to allow model β estimates to be compared to one another (see van den Berg et al., 2006).
For both transect and camera analyses, we first compared models including detection and availability covariates while keeping abundance constant. The detection and availability covariates of the best-supported null abundance models were included in the final model set comparing covariates for abundance.
To test our prediction that cat abundance increases with increasing urban intensity, we compared models with linear and quadratic expressions for impervious surface within a 100-m radius against a model of uniform abundance (Supplementary Table 1). The quadratic term was included to examine whether cat abundance peaks in suburban areas. To examine socioeconomic factors affecting cat abundance, we compared an additional set of models with human demographic variables against a model of uniform abundance. Parameters included human population density, median income, and proportion of residents with at least an associate degree. Income and education were correlated (r = 0.50, P < 0.001), but there was substantial variation in income at high levels of educational attainment (Supplementary Figure 2). When building model sets, we first determined whether linear or quadratic expressions for population density were better supported by comparing cumulative evidence ratios for all models that included either form of the variable. For both camera and transect model sets, a quadratic expression for human population density was best supported. The final model sets were symmetrical and comprised of eight models (Supplementary Table 1).
We used Akaike’s Information Criterion (AICc), corrected for small sample size (Akaike, 1973; Hurvich and Tsai, 1989), to select the best-supported model or models from the candidate sets. Models with a ΔAICc value <2 were considered to be substantially better supported than other models (Burnham and Anderson, 2002). AICc weights were used to evaluate the relative weight of each model’s support in the set. To determine model support for a given covariate, we calculated evidence ratios by dividing the cumulative AICc weight for models with the covariate by the cumulative weight for models that excluded the covariate. Because multiple models may provide useful information about responses to predictor variables (Burnham and Anderson, 2002), we averaged β coefficients, abundance estimates and standard errors by their AICc weights using the R package AICcmodavg (Mazerolle and Mazerolle, 2017). Because the sample area is not defined for N-mixture models, we did not directly compare abundance estimates from each sampling method.
Statistical Analysis of Daily Activity
Cats are generally more active at night, but their degree of nocturnality can vary (Konecny, 1987; Haspel and Calhoon, 1993; Alterio and Moller, 1997; Horn et al., 2011; Kays et al., 2015; Cove et al., 2018). To evaluate the timing of cat activity in association with urban intensity, we examined the time of day in which cats were detected on cameras. We first categorized sites as low (<10% impervious surface), medium (10–40% impervious surface), and high intensity (>40% impervious surface) residential land use classes, based roughly on classifications of Lu and Weng (2006). Detections were then assigned to the three urban intensity classes based on the location of the camera trap. Detections that were less than 30 min from the previous detection of the same cat were considered non-independent and were excluded from the analysis (Ridout and Linkie, 2009). For each detection, we then calculated the time of civil sunrise and sunset on the day of detection using the R package suncalc (Thieurmel and Elmarhraoui, 2019).
To test our prediction that cats would exhibit different activity patterns across the urban gradient, we compared the density distributions of daily activity in low, medium, and high urban intensity sites. Given that day length varied over the 3-month period of this study, and this could bias our results (Nouvellet et al., 2012), we first transformed the times of detection to solar times, which are defined here as radian time values relative to civil sunrise times for a given date and location (Nouvellet et al., 2012; Vazquez et al., 2019). We then fit Von Mises circular kernel density distributions to detections within each urban intensity class using the R package activity (Rowcliffe, 2019). Following Ridout and Linkie (2009), we calculated the degree of overlap () between fitted distributions and used randomization (with replacement; n = 10,000 iterations) to test the probability that detections come from the same distribution (α = 0.05).
To test our prediction that cats in urban environments would be more active at night, we used randomization with replacement (10,000 iterations) to assess the proportion of nighttime (civil sunset to sunrise) and daytime (civil sunrise to sunset) observations in each land use class. We compared the observed difference, Δ, in the proportion of observations between classes with a null distribution of differences between classes. Null distributions were generated by randomizing land use class labels while keeping the number of cats per land use class constant. Significance was determined by calculating the proportion of iterations in which Δ values for the resampled observations were greater than or equal to the observed value of Δ (α = 0.05).
Results
Our transect effort encompassed 318 sampling events across 53 sites, and our camera trapping effort encompassed 1,008 trap nights across 48 sites. In total, we recorded 335 detections of 43 individual cats during transect counts and 188 detections of 45 individuals with camera traps. Cats were detected at 24 of 53 sites by transect surveys and at 25 of 48 sites by cameras. Constant detection was best supported by the distance sampling data (Supplementary Table 2), so our ability to detect cats on transects was not dependent on dew point, temperature, or day of the year. Models that included a negative relationship between time of day and availability for detection were best supported by transect data (Supplementary Table 2), providing evidence that cats were less available for detection on transects as the day progressed. Among camera-based models, the best supported models indicated a lower probability of detecting cats at high dew points (Supplementary Table 2).
Abundance
Across distance sampling transects, the abundance of outdoor cats was predicted to be 5.9 [95% CI (2.6, 13.2)] with a predicted density of 2.9 cats/ha [95% CI (1.3, 6.6)]. The abundance of cats across the impervious surface gradient (Table 1 and Figure 1) was uniform for camera data whereas distance sampling data suggested that cat abundance was highest at intermediate levels of impervious surface, with a peak density of 6.7 cats/ha [95% CI (2.7, 16.5)] at 34.6% impervious surface. The model-averaged β coefficient of impervious surface and impervious surface squared, was 1.11 ± 0.39 (SE) and −1.06 ± 0.35, respectively (Figure 2). The evidence ratio of transect-based models that contained the quadratic term for impervious surface to the models without it was 138.73. The camera trap data did not provide supportive evidence for an association between cat abundance and impervious surface cover.
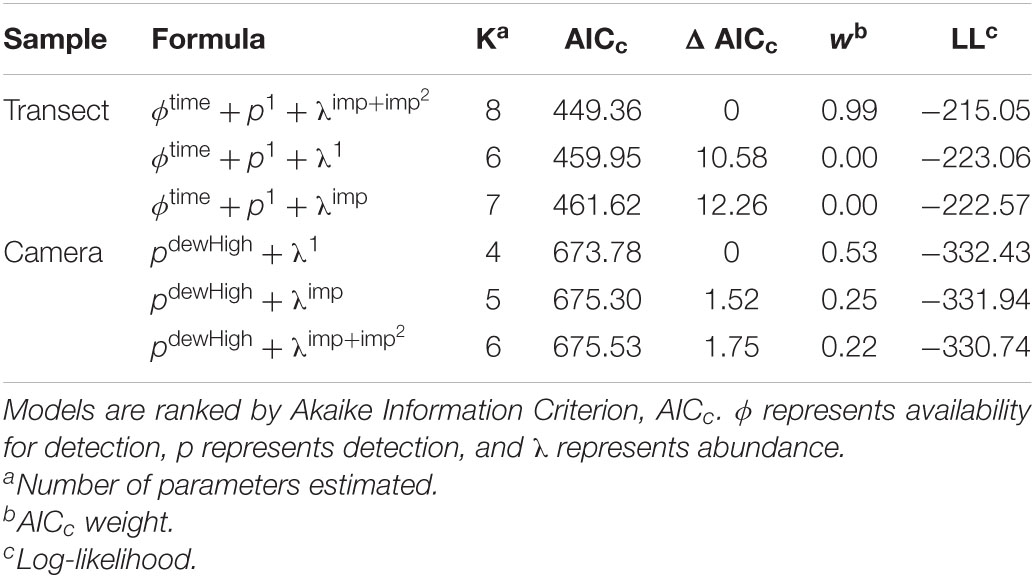
Table 1. Summary statistics of the candidate models examining the relationship between impervious surface cover and cat abundance in the Washington, DC region, summer 2016.
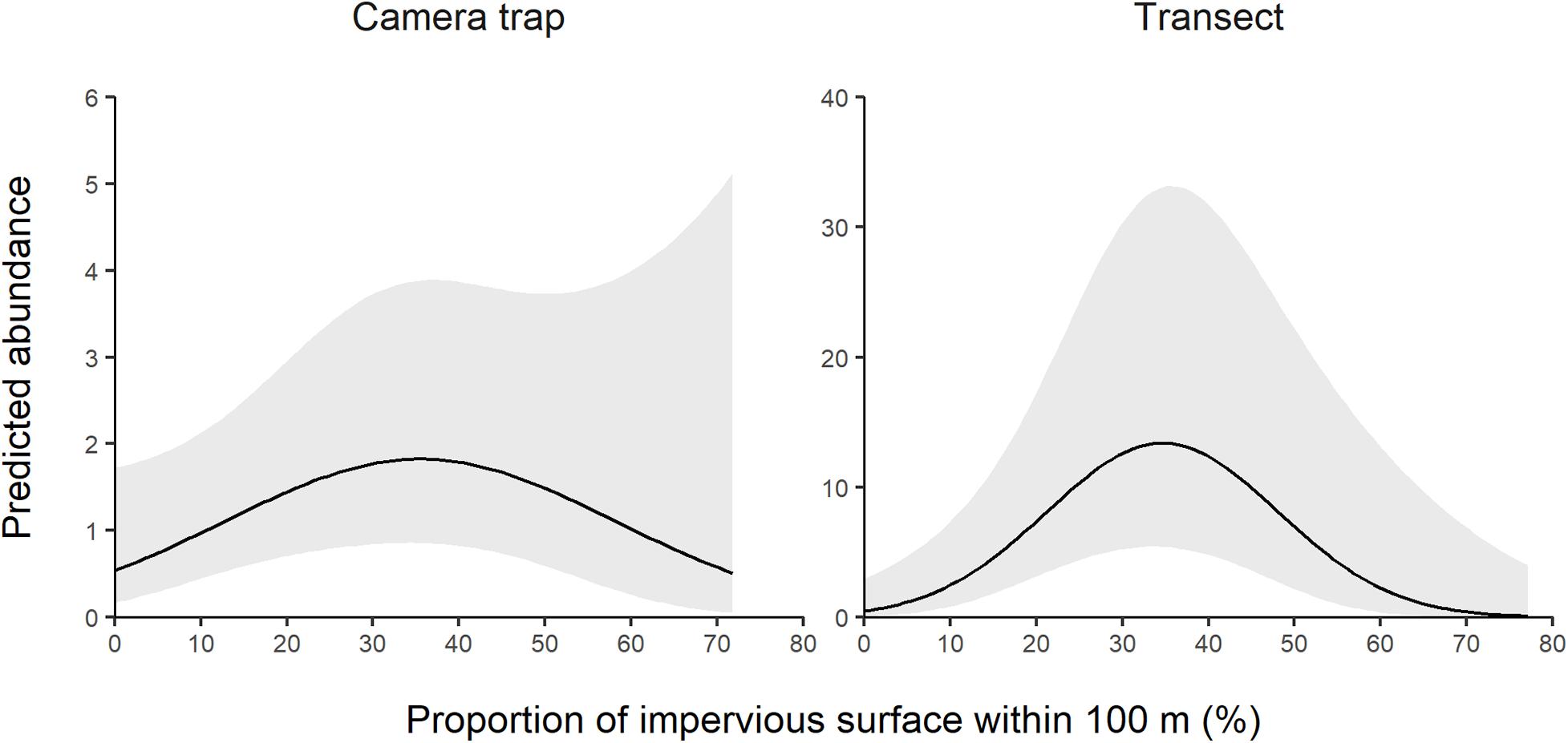
Figure 1. Estimates of cat abundance across an urban gradient in the Washington, DC region in summer 2016. Shaded regions represent the 95% confidence intervals in cat abundance estimates at a given proportion of impervious surface.
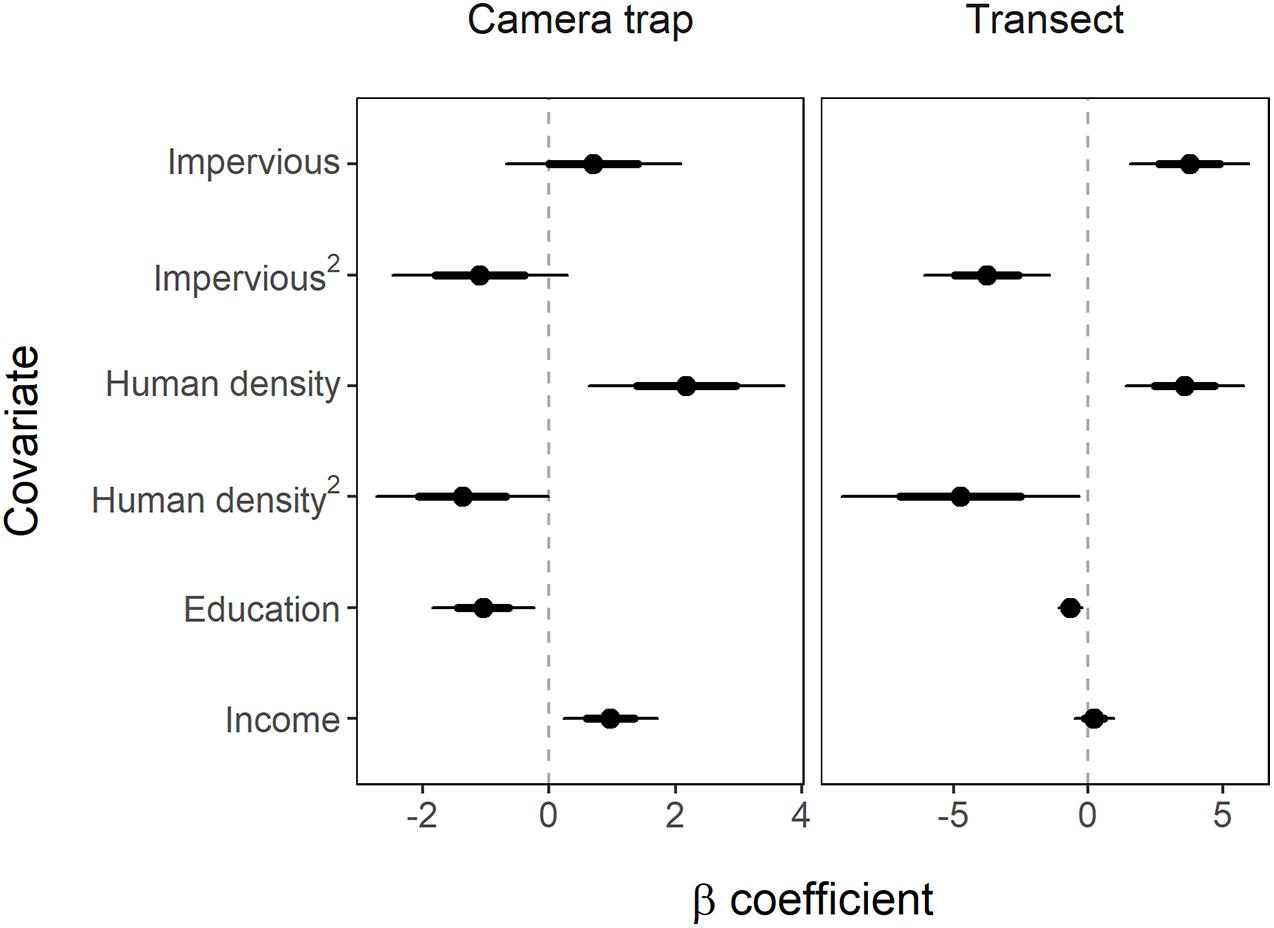
Figure 2. Model-averaged estimates of β coefficient (effect sizes) of predictor variables on cat abundance in the Washington, DC region in summer 2016, estimated using data from transect and camera trap surveys. Thick lines represent the model-averaged standard errors relative to the β prediction. Thin lines represent the 95% confidence intervals. All variables were converted to a common scale prior to model fitting, so β values represent the slope associated with scaled variables. An explanation of each variable can be found in Supplementary Table 1.
The patterns of association between human demographic variables and cat abundance were similar for transect and camera trap data (Figure 2). For the transect data, the best-supported models showed peak cat abundance at intermediate levels of human population density (βavg = 1.63 ± 0.51, β2avg = −1.25 ± 0.59) and declining cat abundance as education levels increased (βavg = −0.64 ± 0.25; Table 2 and Figure 3). The evidence ratio of transect-based models that contained the quadratic population density term to those without it was 427.55. Among camera-based models (Figure 4), the model best supported by the data showed peak cat abundance at intermediate levels of human population density (βavg = 1.27 ± 0.87, β2avg = −0.26 ± 0.21), declining cat abundance with increasing levels of education (βavg = −0.94 ± 0.50), and a slight increase in cat abundance as income increased (βavg = 0.79 ± 0.51; Table 2). The evidence ratio of camera-based models that contained the quadratic population density covariate (four of eight models) to those without it was 3.13.
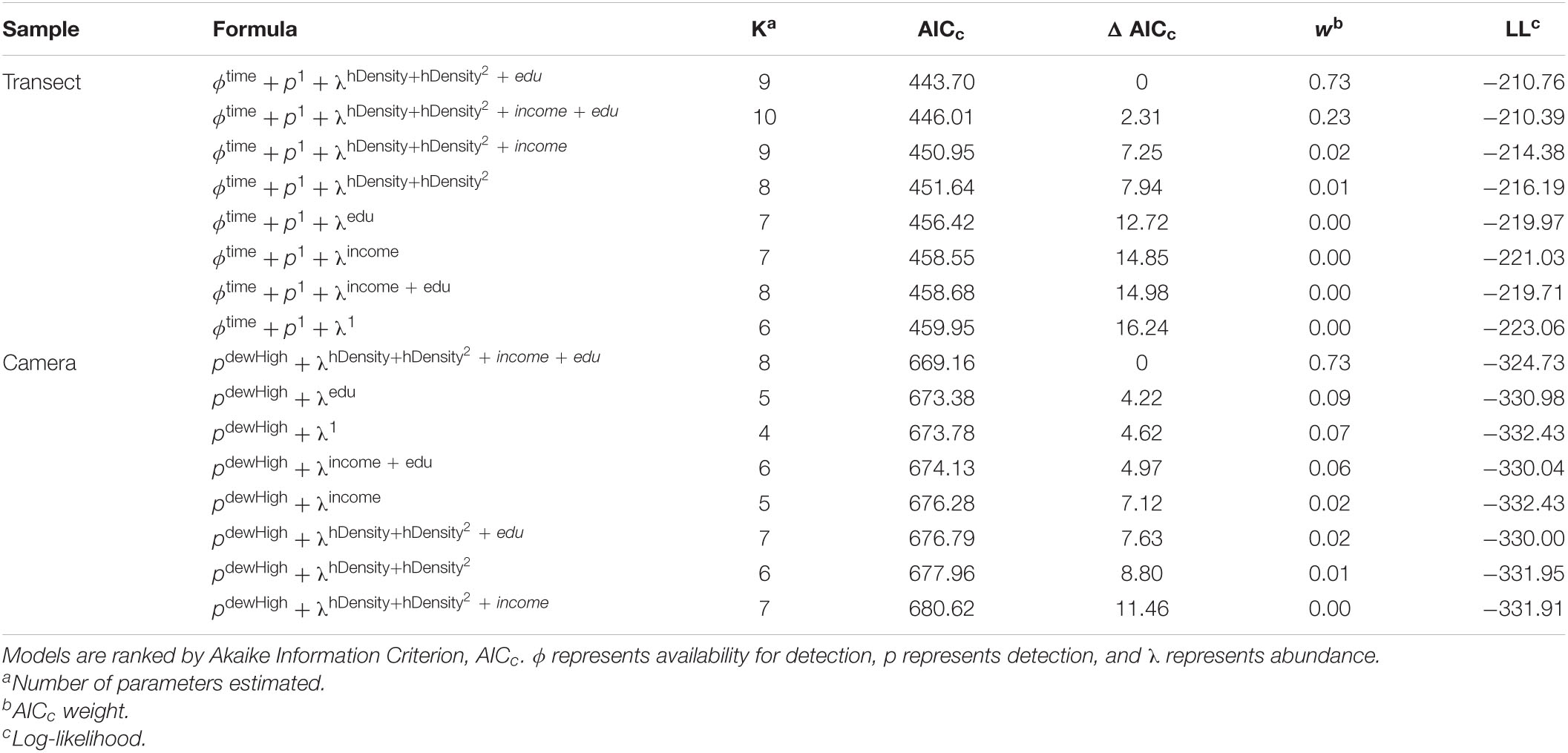
Table 2. Summary statistics of the candidate models examining the relationship between human demographics and cat abundance in the Washington, DC region, summer 2016.
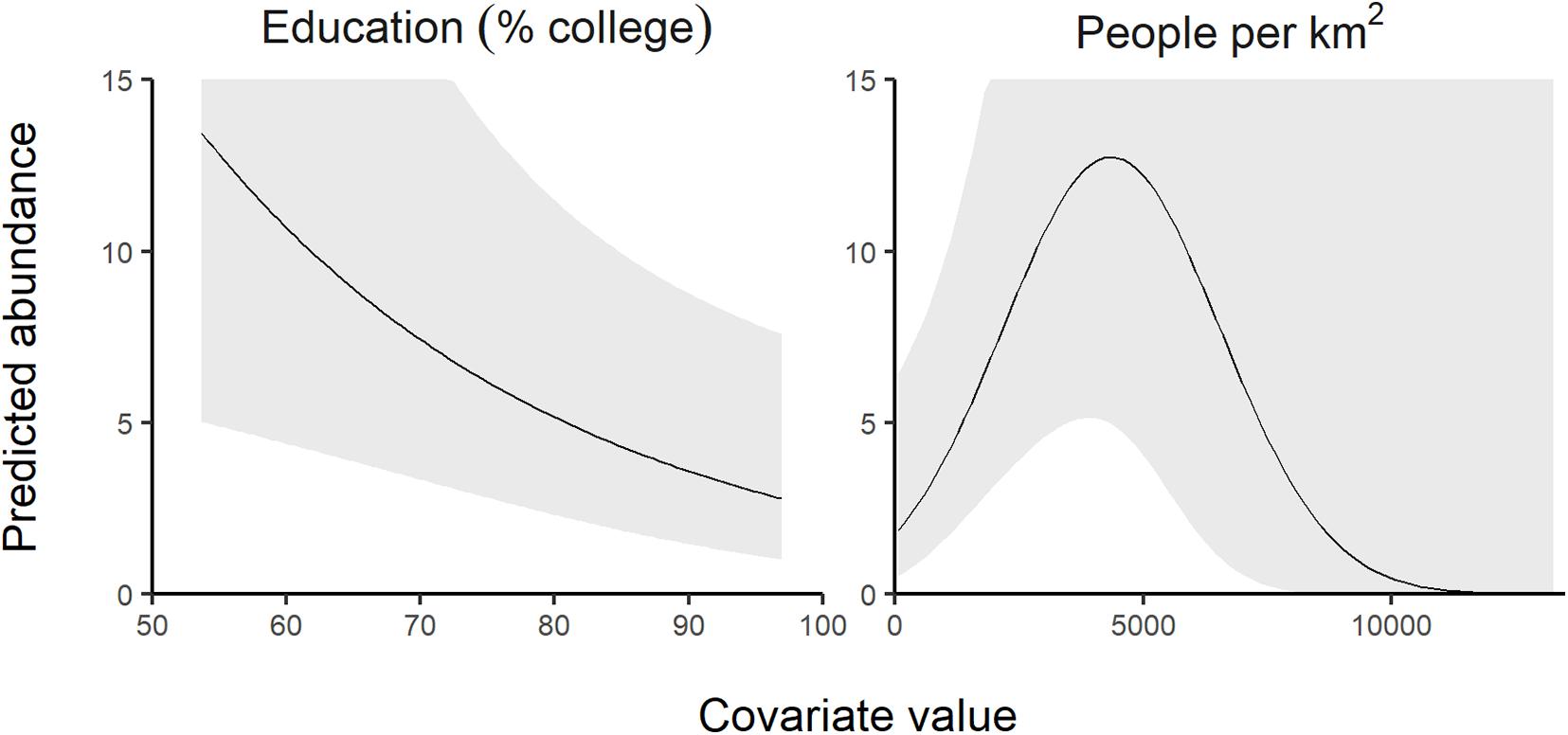
Figure 3. Estimates of cat abundance from models using transect data across gradients of population density and the proportion of the population with a college education within a census tract. Shaded regions represent the 95% confidence intervals in cat abundance estimates at a given level of each human demographic variable.
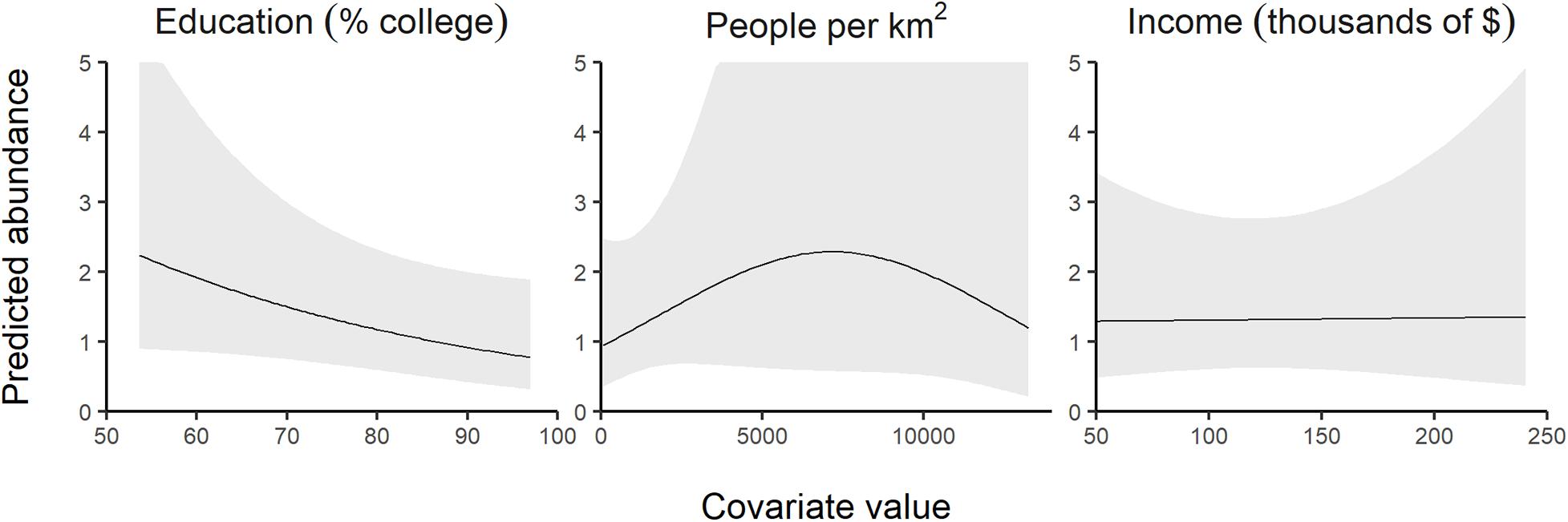
Figure 4. Estimates of cat abundance from models using camera trap data across gradients of population density and the proportion of the population with a college education within a census tract. Shaded regions represent the 95% confidence intervals in cat abundance estimates at a given level of each human demographic variable.
Daily Activity
In low, medium, and high intensity urban environments, camera traps captured 34, 115, and 39 independent detections of cats, respectively. Overall, cats were most active in the early morning and late at night, although this pattern was found to be dependent on urban land use intensity (Figure 5). The distribution of daily activity differed among urban intensity classes, with low intensity cats most active in the day, medium intensity cats most active after midnight, and high intensity cats mostly active in the evening before midnight. For each paired urban intensity class, we rejected the null hypothesis that the observations were drawn from the same distribution (Table 3).
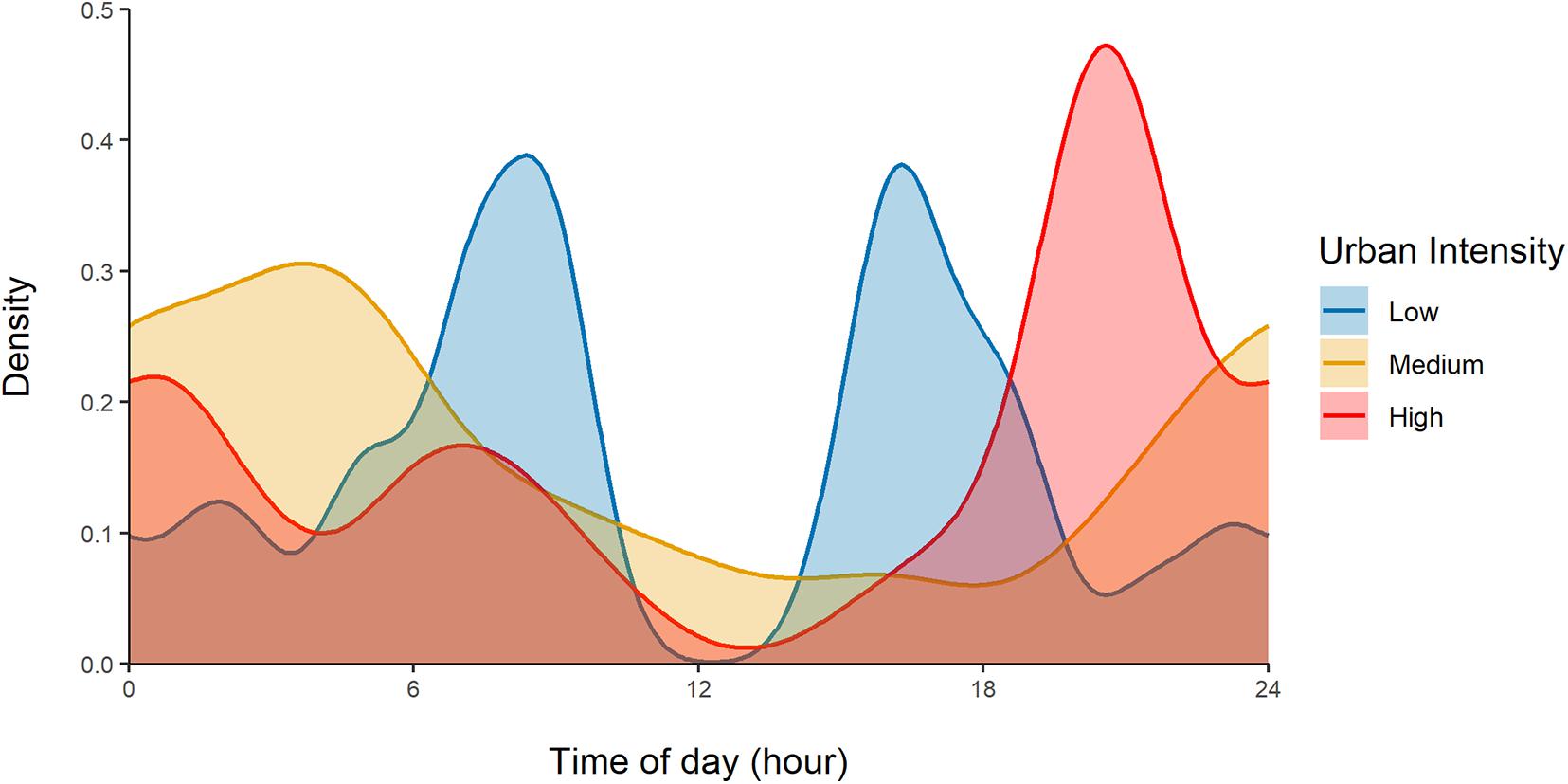
Figure 5. Estimated daily timing of cat activity in the Washington, DC region in summer 2016 based on detections by camera traps. Land-use classes of sites were defined as follows: 0–10% impervious surface within a 100 m radius, “low intensity” urban; 10–40% impervious surface, “medium intensity”; greater than 40% impervious surface, “high intensity.”
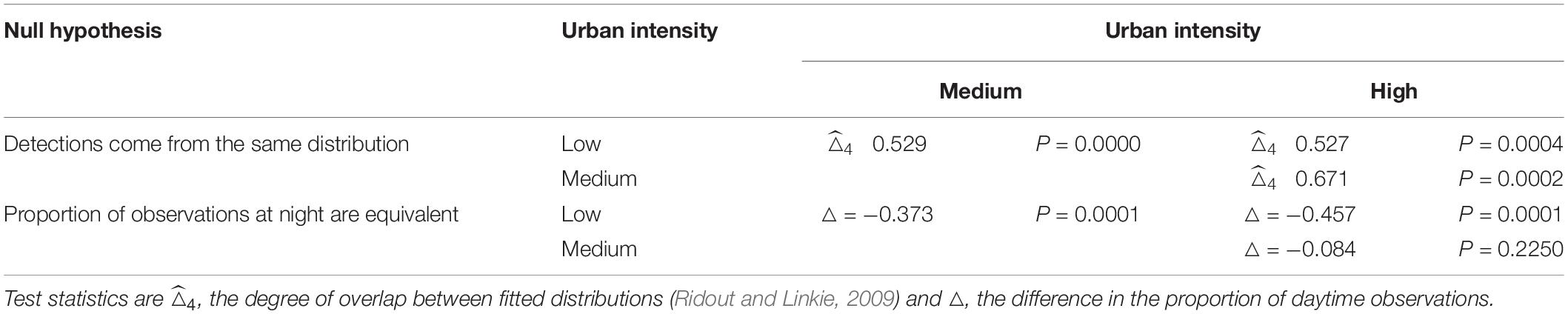
Table 3. Summary statistics describing differences in the daily activity of cats in low, medium, and highurban intensity sites under the null hypotheses that: (1) the circular kernel density distributions are equivalent across land use classes; and (2) the proportion of observations at night are equivalent across land use classes.
Cats in medium and high intensity urban environments were more likely to be observed at night than those in low intensity environments. Nighttime detections represented only 23.5% of observations in low intensity urban environments but made up 60.9 and 69.2% of detections in medium and high intensity urban environments, respectively. We rejected the null hypothesis that the proportion of cats observed at night at low intensity sites was equivalent to that of medium or high intensity sites (Table 3). There was no evidence that the proportion of nighttime observations varied between medium and high intensity sites.
The degree of overlap between the timing of transect surveys and cat activity differed with respect to land-use class. Though 95% of transect surveys were conducted between 05:56 and 13:24 only 23.4% of camera detections occurred during this time window. Among low, medium, and high intensity land uses classes, 35.6, 19.2, and 20.5% of detections occurring during this time window, respectively. Thus, transect surveys were more likely to be conducted at times when cats were active in low intensity areas than medium or high intensity areas (Figure 4).
Discussion
The results from our surveys supported our hypothesis that cat abundance and activity patterns are associated with land-use and human demographics. Transect results identified impervious surface, human population density, and level of education as important predictors of cat abundance. Camera data supported associations between abundance and level of education, income, and population density. Both methods yielded similar patterns of abundance in response to covariates, though the degree of influence and variance in the β estimates differed between methods (Figure 2). Our findings that the timing of cat activity varies with urban land use intensity provides insight into the complexity of enumerating free-ranging cats, given that the populations are often comprised of both owned and unowned individuals.
Results from both sampling methods suggest that cat abundance peaks at intermediate levels of human populations density, and transect analyses showed highest abundances at intermediate levels of impervious surface. This may represent a balance where provisioning of cats in human-populated areas (Tennent and Downs, 2008) is offset by high mortality in urban centers, e.g., from automobiles (Childs and Ross, 1986) or disease (Natoli et al., 2005), or less outdoor access for owned urban cats (Lepczyk et al., 2004). Previous studies found that cat abundance increased linearly with human population density or urban intensity (Lepczyk et al., 2004; Sims et al., 2008; Murray et al., 2010; Thomas et al., 2012; Flockhart et al., 2016). The discrepancy between our results and those of social surveys may be due to differential patterns of abundance for owned and unowned cat populations across the urban gradient. Horn et al. (2011) showed that owned and unowned cats occupied different home range sizes, used different habitat types, and had different temporal activity patterns. Social surveys primarily focused on owned cats may therefore miss part of the picture that is available to transect or camera sampling efforts, for example, unowned cats in mid-intensity urban areas. Additionally, human pet-owning behavior varies by region. For instance, urban cat owners in the United Kingdom, where several previous studies were conducted, may be more likely to allow owned cats outside than owners in the United States (Hall et al., 2016; Crowley et al., 2019). Urban context may be another determinant of observed abundance patterns across the urban gradient. Previous research has found a linear positive relationship between cat abundance and building density in Guelph, ON, Canada (Flockhart et al., 2016), a linear negative relationship between cat abundance and the distance from human dwellings in Big Pine Key, FL, United States (Cove et al., 2018), and no relationship between cat abundance and urban density in Stillwater, OK, United States (Elizondo and Loss, 2016). The urban gradients in these communities represent only a portion of the gradient across the greater Washington, DC metropolitan region. Moreover, other location-specific factors may also influence cat abundance. For example, cats may avoid areas frequented by predators such as coyotes (Crooks and Soulé, 1999; Kays et al., 2015). The extent to which predators use different habitats may vary geographically (Slate, 1985; Prange et al., 2003) or over time (Hody and Kays, 2018). Likewise, latitude may influence abundance through altering carrying capacity and limiting annual fecundity (see Flockhart and Coe, 2018). The discrepancy in results between our study and previous efforts may therefore suggest that cat abundance is dependent on more complex factors than any single measure of urban intensity and not directly comparable across regions or between different urban contexts.
Both transects and camera traps suggested an association between socioeconomic status and cat abundance. Among transect-based models, college education was an important covariate, and among camera-based models, college education and income were important covariates. In both analyses, as level of education increased, cat abundance decreased. If education is viewed as a component of socioeconomic status, our results support previous findings that areas of lower socioeconomic status have larger free-ranging cat populations (Finkler et al., 2011), possibly owing to lower rates of sterilization (Chu et al., 2009; Finkler et al., 2011) and more available food resources (Calhoon and Haspel, 1989). Surprisingly, in the camera analysis, cat abundance was also found to be positively associated with income, though this had a limited effect on abundance (Figure 4). One possible explanation for a lack of a negative association between income and cat abundance in our study is the range of incomes sampled—the lowest median income in a census block for our study was roughly $50,000/year, which is close to the highest median income in the study by Flockhart et al. (2016). Education may also play a role beyond being an indicator of socioeconomic status. Survey respondents with a college degree from across the United States were less likely than those without a degree to hold the view that cats fill a natural role in the ecosystem (Peterson et al., 2012). Relatedly, Loyd and Miller (2010) reported that as education increased, so did the preference for euthanasia-based cat management among Illinois survey respondents. Our study points to the importance of considering social factors in management of cat populations.
By examining when cameras detected cats, we characterized how cat activity differs over the course of the day. Overall, cats were most active late at night and in the early morning. This finding agrees with the results of our transect analysis, which showed that cats’ availability for detection declined between early morning and mid-afternoon. This result is also consistent with previous findings that cats were most active at dawn and dusk and least active around midday in the Galápagos Islands (Konecny, 1987) and Florida Keys (Cove et al., 2018) and that cats were four times as active between 22:00 and 07:00 as during the rest of the day in Brooklyn, NY, United States (Haspel and Calhoon, 1993).
Timing of cat activity also varied among land-use classes. Cats at low intensity residential sites were active in the mid-morning and early evening whereas cats at medium and high intensity sites were most active at night. These differences may reflect behavior of cat owners and differences among owned and unowned cats. Cat owners’ propensity to let their cats outside and when they do so may depend on the characteristics of their local neighborhood. In southern Michigan, for example, urban residents were less likely than rural or suburban residents to have partially or fully outdoor cats (Lepczyk et al., 2004). A survey of cat owners from the eastern United States reported that rural residents allowed their cats more outdoor access compared to urban or suburban residents, though the difference was not statistically significant (Clancy et al., 2003). Differences in cat owner behavior across the urban gradient may result in a greater proportion of cats in Washington, DC being unowned in high and medium intensity residential areas than low intensity residential areas. Evidence from previous studies suggests that unowned and owned cats exhibit distinct activity patterns. Of the respondents to the survey of Clancy et al. (2003), 97% of indoor-outdoor cat owners reported keeping their cats inside overnight. Unowned cats in central Illinois were more definitively nocturnal than owned cats (Horn et al., 2011). Owned cat activity varied less over the course of the day with peaks around dawn and dusk (Horn et al., 2011), a pattern similar to the one observed among cats at our low intensity residential sites. This pattern would also explain the results of Kays et al. (2015), who reported that cats captured on camera traps in forested protected areas exhibited more nocturnal activity patterns than cats in residential yards. Similarly, Cove et al. (2018) reported that in the Florida Keys, a population of largely owned cats was most active at dawn and dusk, while a population of largely unowned cats was mostly active late at night. These results suggest that low intensity residential areas in Washington, DC may contain more owned cats, while medium and high intensity residential areas may contain more unowned cats, resulting in more nocturnal activity. An alternative possibility is that activity patterns of humans vary across the urban gradient, and cats vary their activity accordingly. For example, in areas of high and medium urban intensity, cats may avoid car and foot traffic by limiting their active hours to nighttime.
The observed differences in cat activity over the land-use gradient in this study has important implications for sampling cat populations. Transect surveys are a common method for sampling animal populations (Marshall et al., 2008) but are often conducted at a particular time each day. Our transect surveys were conducted only during the day, so low intensity residential land-use cats may have been overrepresented relative to medium and high intensity land-use cats. However, because our transect and camera results were largely in agreement, and the timing of activity was similar at medium and high intensity sites, it is unlikely that differences in activity patterns introduced undue bias into our transect results. Nevertheless, researchers should not assume that daytime transects will provide an unbiased sample of cat abundance across an urban gradient. Cat activity patterns may differ across the gradient and between owned and unowned cats. Ecological and cultural differences among cities, in addition to affecting cat abundance, may also affect their behavior. We recommend that, when feasible, exploratory camera trap surveys should be used to identify optimal times to conduct transect surveys across different study areas.
Several limitations of this study should be noted. First, because transect and camera surveys sampled the landscape at different spatial scales, we were unable to directly compare abundance estimates between sampling approaches. Future studies can address this limitation by deploying multiple cameras per site (see Rowcliffe et al., 2008). Second, like other similar studies (Schmidt et al., 2007b; Flockhart et al., 2016), we conducted distance sampling along roads, which violates the assumption that sampled animals are randomly distributed with respect to the transect. Though this may bias abundance estimates, especially in high intensity land use areas with many human structures along the road, similar abundance patterns between methods suggest that this did not meaningfully affect our results. Third, we sampled cats at a relatively small scale centered around a single home or apartment, but human demographic data was measured at the census tract scale. A future remedy would be to conduct transects throughout the census tract to thoroughly sample for cats at the same scale as predictor variables for human demographics. Finally, although NN sites presented a unique opportunity for this work, our study sites were almost exclusively in neighborhoods comprised of single-family homes. This led to low confidence in our estimates within the rural and urban portions of the urban gradient. Regional assessment of cat abundance in urban areas requires sampling across multiunit dwellings, undeveloped land, commercial, and industrial areas, as each likely exhibits unique patterns of cat abundance and behavior (Schmidt et al., 2007a; Flockhart et al., 2016). Additionally, areas of high urban intensity or containing mostly clusters of cats in colonies may require their own survey methods (see Davis and Lepczyk, 2010).
Estimates of domestic cat abundance and understanding the predictors of abundance are critical for wildlife conservation. Deterministic models, some of which are currently being implemented to explore management alternatives like trap-remove and trap-neuter-return, often rely on accurate abundance estimates (Andersen et al., 2004; Flockhart and Coe, 2018). Because vital rates in these models may be constrained by estimated carrying capacities, inaccurate abundance estimates can yield unrealistic outcomes. Moreover, accurate count data are necessary for monitoring the efficacy of management programs following implementation (Gunther et al., 2020). Our results suggest that future efforts should account for predictors of cat abundance, such as human population density and socioeconomics, to estimate model parameters. Differences between our results and those of other studies, especially those based on social surveys, highlight the need to take geographic and cultural context into account when estimating cat abundance – predictors of cat abundance for one region may not be directly applicable to others.
Data Availability Statement
The datasets presented in this study can be found in online repositories. The names of the repository/repositories and accession number(s) can be found below: https://github.com/SMBC-NZP/dc_cats.
Ethics Statement
Ethical review and approval was not required for the animal study because data were collected passively (i.e., in situ counts of organisms). Written informed consent was obtained from the owners for the participation of their animals in this study.
Author Contributions
KB conducted all field work. KB, BE, and PM conceived of the study and developed the sampling design. KB and BE analyzed the data and wrote the manuscript. PM and JC supervised the writing process. All authors contributed to the article and approved the submitted version.
Conflict of Interest
The authors declare that the research was conducted in the absence of any commercial or financial relationships that could be construed as a potential conflict of interest.
Acknowledgments
We thank R. Reitsma and D. L. Narango for helping to coordinate between this study and the larger NN project and NN participants who permitted us access to their property. We also thank to M. V. Cove, who provided helpful comments that improved the manuscript. W. J. McShea, R. W. Kays, and the eMammal project generously lent us motion-sensor cameras.
Supplementary Material
The Supplementary Material for this article can be found online at: https://www.frontiersin.org/articles/10.3389/fevo.2021.643845/full#supplementary-material
References
Akaike, H. (1973). “Information theory and an extension of the maximum likelihood principle,” in Second International Symposium on Information Theory, eds F. Csaki and B. Petrov (Budapest: Akamemiai Kiado), 267–281.
Alterio, N., and Moller, H. (1997). Daily activity of stoats (Mustela erminea), feral ferrets (Mustela furo) and feral house cats (Felis catus) in coastal grassland, Otago Peninsula, New Zealand. N. Z. J. Ecol. 21, 89–95.
Andersen, M. C., Martin, B. J., and Roemer, G. W. (2004). Use of matrix population models to estimate the efficacy of euthanasia versus trap-neuter-return for management of free-roaming cats. J. Am. Vet. Med. Assoc. 225, 1871–1876. doi: 10.2460/javma.2004.225.1871
Baker, P. J., Molony, S. E., Stone, E., Cuthill, I. C., and Harris, S. (2008). Cats about town: is predation by free−ranging pet cats Felis catus likely to affect urban bird populations? IBIS 150, 86–99. doi: 10.1111/j.1474-919x.2008.00836.x
Barker, R. J., Schofield, M. R., Link, W. A., and Sauer, J. R. (2018). On the reliability of n−mixture models for count data. Biometrics 74, 369–377. doi: 10.1111/biom.12734
Barratt, D. G. (1997). Home range size, habitat utilisation and movement patterns of suburban and farm cats Felis catus. Ecography 20, 271–280. doi: 10.1111/j.1600-0587.1997.tb00371.x
Blancher, P. (2013). Estimated number of birds killed by house cats (Felis catus) in Canada. Avian Conserv. Ecol. 8:3. doi: 10.5751/ACE-00557-080203
Brown, A. S., Schaefer, C. A., Quesenberry, C. P. Jr., Liu, L., Babulas, V. P., and Susser, E. S. (2005). Maternal exposure to toxoplasmosis and risk of schizophrenia in adult offspring. Am. J. Psychiatry 162, 767–773. doi: 10.1176/appi.ajp.162.4.767
Buckland, S. T., Anderson, D. R., Burnham, K. P., Laake, J. L., Borchers, D. L., and Thomas, L. (2001). Introduction to Distance Sampling: Estimating Abundance of Biological Populations. Oxford: Oxford University Press.
Burnham, K. P., and Anderson, D. R. (2002). Model Selection and Multimodel Inference: A Practical Information-Theoretic Approach, 2nd Edn. New York, NY: Springer-Verlag.
Calhoon, R. E., and Haspel, C. (1989). Urban cat populations compared by season, subhabitat and supplemental feeding. J. Anim. Ecol. 58, 321–328. doi: 10.2307/5003
Chandler, R. B., Royle, J. A., and King, D. I. (2011). Inference about density and temporary emigration in unmarked populations. Ecology 92, 1429–1435. doi: 10.1890/10-2433.1
Childs, J., and Ross, L. (1986). Urban cats: characteristics and estimation of mortality due to motor vehicles. Am. J. Vet. Res. 47, 1643–1648.
Childs, J. E. (1990). Urban cats: their demography, population density, and owner characteristics in Baltimore, Maryland. Anthrozoös 3, 234–244. doi: 10.2752/089279390787057432
Chu, K., Anderson, W. M., and Rieser, M. Y. (2009). Population characteristics and neuter status of cats living in households in the united states. J. Am. Vet. Med. Assoc. 234, 1023–1030. doi: 10.2460/javma.234.8.1023
Churcher, P., and Lawton, J. (1987). Predation by domestic cats in an English village. J. Zool. 212, 439–455. doi: 10.1111/j.1469-7998.1987.tb02915.x
Clancy, E. A., Moore, A. S., and Bertone, E. R. (2003). Evaluation of cat and owner characteristics and their relationships to outdoor access of owned cats. J. Am. Vet. Med. Assoc. 222, 1541–1545. doi: 10.2460/javma.2003.222.1541
Cove, M. V., Gardner, B., Simons, T. R., Kays, R., and O’Connell, A. F. (2018). Free-ranging domestic cats (Felis catus) on public lands: estimating density, activity, and diet in the Florida Keys. Biol. Invasions 20, 333–344. doi: 10.1007/s10530-017-1534-x
Crawford, H. M., Calver, M. C., and Fleming, P. A. (2019). A case of letting the cat out of the bag—why trap-neuter-return is not an ethical solution for stray cat (Felis catus) management. Animals 9:171. doi: 10.3390/ani9040171
Crooks, K. R., and Soulé, M. E. (1999). Mesopredator release and avifaunal extinctions in a fragmented system. Nature 400, 563–566. doi: 10.1038/23028
Crowley, S. L., Cecchetti, M., and McDonald, R. A. (2019). Hunting behaviour in domestic cats: an exploratory study of risk and responsibility among cat owners. People Nat. 1, 18–30. doi: 10.1002/pan3.6
Davis, A., and Lepczyk, C. A. (2010). “Feral cat population surveys and management options for the University of Hawai‘i at Mânoa,” in Proceedings of the 24th Vertebrate Pest Conference, eds R. M. Timm and K. A. Fagerstone (Davis: University of California), 68–71.
Doherty, T. S., Glen, A. S., Nimmo, D. G., Ritchie, E. G., and Dickman, C. R. (2016). Invasive predators and global biodiversity loss. Proc. Natl. Acad. Sci. U. S. A. 113, 11261–11265. doi: 10.1073/pnas.1602480113
Elizondo, E. C., and Loss, S. R. (2016). Using trail cameras to estimate free-ranging domestic cat abundance in urban areas. Wildl. Biol. 22, 246–252. doi: 10.2981/wlb.00237
Evans, B. S., Ryder, T. B., Reitsma, R., Hurlbert, A. H., and Marra, P. P. (2015). Characterizing avian survival along a rural-to-urban land use gradient. Ecology 96, 1631–1640. doi: 10.1890/14-0171.1
Evans, C., Abrams, E., Reitsma, R., Roux, K., Salmonsen, L., and Marra, P. P. (2005). The neighborhood nestwatch program: participant outcomes of a citizen−science ecological research project. Conserv. Biol. 19, 589–594. doi: 10.1111/j.1523-1739.2005.00s01.x
Ficetola, G. F., Barzaghi, B., Melotto, A., Muraro, M., Lunghi, E., Canedoli, C., et al. (2018). N-mixture models reliably estimate the abundance of small vertebrates. Sci. Rep. 8, 1–8.
Finkler, H., Hatna, E., and Terkel, J. (2011). The influence of neighbourhood socio-demographic factors on densities of free-roaming cat populations in an urban ecosystem in Israel. Wildl. Res. 38, 235–243. doi: 10.1071/wr10215
Fiske, I., and Chandler, R. (2011). Unmarked: an R package for fitting hierarchical models of wildlife occurrence and abundance. J. Stat. Softw. 43, 1–23.
Flockhart, D. T., and Coe, J. B. (2018). Multistate matrix population model to assess the contributions and impacts on population abundance of domestic cats in urban areas including owned cats, unowned cats, and cats in shelters. PloS One 13:e0192139. doi: 10.1371/journal.pone.0192139
Flockhart, D. T., Norris, D. R., and Coe, J. B. (2016). Predicting free−roaming cat population densities in urban areas. Anim. Conserv. 5, 472–483. doi: 10.1111/acv.12264
Frenkel, J. K., Dubey, J. P., and Miller, N. L. (1970). Toxoplasma gondii in cats: fecal stages identified as coccidian oocysts. Science 167, 893–896. doi: 10.1126/science.167.3919.893
Glenn, E. H. (2019). Acs: Download, Manipulate, and Present American Community Survey and Decennial Data from the US Census. R package version 2.1.4. Available online at: https://CRAN.R-project.org/package=acs (accessed February 19).
Greenwell, C. N., Calver, M. C., and Loneragan, N. R. (2019). Cat gets its tern: a case study of predation on a threatened coastal seabird. Animals 9:445. doi: 10.3390/ani9070445
Gunther, I., Azriel, L., Wolf, H., Raz, T., and Klement, E. (2020). An accessible scheme for monitoring free-roaming cat population trends. Ecol. Evol. 10, 1288–1298. doi: 10.1002/ece3.5982
Hall, C. M., Adams, N. A., Bradley, J. S., Bryant, K. A., Davis, A. A., Dickman, C. R., et al. (2016). Community attitudes and practices of urban residents regarding predation by pet cats on wildlife: an international comparison. PloS One 11:e0151962. doi: 10.1371/journal.pone.0151962
Haspel, C., and Calhoon, R. E. (1989). Home ranges of free-ranging cats (Felis catus) in Brooklyn, New York. Can. J. Zool. 67, 178–181. doi: 10.1139/z89-023
Haspel, C., and Calhoon, R. E. (1993). Activity patterns of free-ranging cats in Brooklyn, New York. J. Mammal. 74, 1–8. doi: 10.2307/1381900
Hijmans, R. J. (2020). Raster: Geographic Data Analysis and Modeling. R Package Version 2.5-8. Available online at: https://CRAN.R-project.org/package=raster (accessed June 18, 2021).
Hody, J. W., and Kays, R. (2018). Mapping the expansion of coyotes (Canis latrans) across North and Central America. Zookeys 759, 81–97. doi: 10.3897/zookeys.759.15149
Horn, J. A., Mateus-Pinilla, N., Warner, R. E., and Heske, E. J. (2011). Home range, habitat use, and activity patterns of free-roaming domestic cats. J. Wildl. Manag. 75, 1177–1185. doi: 10.1002/jwmg.145
Hurvich, C. M., and Tsai, C.-L. (1989). Regression and time series model selection in small samples. Biometrika 76, 297–307. doi: 10.1093/biomet/76.2.297
Kays, R., Costello, R., Forrester, T., Baker, M. C., Parsons, A. W., Kalies, E. L., et al. (2015). Cats are rare where coyotes roam. J. Mammal. 96, 981–987. doi: 10.1093/jmammal/gyv100
Kays, R., Dunn, R., Parsons, A., Mcdonald, B., Perkins, T., Powers, S., et al. (2019). The small home ranges and large local ecological impacts of pet cats. Anim. Conserv. 23, 516–523. doi: 10.1111/acv.12563
Kilgour, R. J., Magle, S. B., Slater, M., Christian, A., Weiss, E., and DiTullio, M. (2017). Estimating free-roaming cat populations and the effects of one year trap-neuter-return management effort in a highly urban area. Urban Ecosyst. 20, 207–216. doi: 10.1007/s11252-016-0583-8
Konecny, M. J. (1987). Home range and activity patterns of feral house cats in the Gálapagos Islands. Oikos 50, 17–23. doi: 10.2307/3565397
Lepczyk, C. A., Mertig, A. G., and Liu, J. (2004). Landowners and cat predation across rural-to-urban landscapes. Biol. Conserv. 115, 191–201. doi: 10.1016/s0006-3207(03)00107-1
Ling, V. J., Lester, D., Mortensen, P. B., Langenberg, P. W., and Postolache, T. T. (2011). Toxoplasma gondii seropositivity and suicide rates in women. J. Nerv. Ment. Dis. 199, 440–444. doi: 10.1097/nmd.0b013e318221416e
Loss, S. R., and Marra, P. P. (2017). Population impacts of free−ranging domestic cats on mainland vertebrates. Front. Ecol. Environ. 15, 502–509. doi: 10.1002/fee.1633
Loss, S. R., Will, T., and Marra, P. P. (2013). The impact of free-ranging domestic cats on wildlife of the united states. Nat. Commun. 4:1396. doi: 10.1038/ncomms2380
Loyd, K. A. T., and Miller, C. A. (2010). Influence of demographics, experience and value orientations on preferences for lethal management of feral cats. Hum. Dimen. Wildl. 15, 262–273. doi: 10.1080/10871209.2010.491846
Lu, D., and Weng, Q. (2006). Use of impervious surface in urban land-use classification. Remote Sens. Environ. 102, 146–160. doi: 10.1016/j.rse.2006.02.010
Marshall, A. R., Lovett, J. C., and White, P. C. (2008). Selection of line−transect methods for estimating the density of group−living animals: lessons from the primates. Am. J. Primatol. 70, 452–462. doi: 10.1002/ajp.20516
Mazerolle, M. J., and Mazerolle, M. M. J. (2017). Package ‘Aiccmodavg’. R Package Version 2.3-1. Available online at: https://cran.r-project.org/package=AICcmodavg (accessed June 28, 2020).
Medina, F. M., Bonnaud, E., Vidal, E., Tershy, B. R., Zavaleta, E. S., Josh Donlan, C., et al. (2011). A global review of the impacts of invasive cats on island endangered vertebrates. Glob. Change Biol. 17, 3503–3510. doi: 10.1111/j.1365-2486.2011.02464.x
Murray, J., Browne, W., Roberts, M., Whitmarsh, A., and Gruffydd-Jones, T. (2010). Number and ownership profiles of cats and dogs in the UK. Vet. Rec. 166, 163–168. doi: 10.1136/vr.b4712
Murray, J., Mosteller, J. R., Loberg, J. M., Anderson, M., and Benka, V. A. (2015). Methods of fertility control in cats: owner, breeder and veterinarian behavior and attitudes. J. Feline Med. Surg. 17, 790–799. doi: 10.1177/1098612x15594994
Natoli, E., Say, L., Cafazzo, S., Bonanni, R., Schmid, M., and Pontier, D. (2005). Bold attitude makes male urban feral domestic cats more vulnerable to feline immunodeficiency virus. Neurosci. Biobehav. Rev. 29, 151–157. doi: 10.1016/j.neubiorev.2004.06.011
Nogales, M., Vidal, E., Medina, F. M., Bonnaud, E., Tershy, B. R., Campbell, K. J., et al. (2013). Feral cats and biodiversity conservation: the urgent prioritization of island management. Bioscience 63, 804–810. doi: 10.1525/bio.2013.63.10.7
Normand, C., Urbanek, R. E., and Gillikin, M. N. (2019). Population density and annual and seasonal space use by feral cats in an exurban area. Urban Ecosyst. 22, 303–313. doi: 10.1007/s11252-018-0812-4
Nouvellet, P., Rasmussen, G. S. A., Macdonald, D. W., and Courchamp, F. (2012). Noisy clocks and silent sunrises: measurement methods of daily activity pattern. J. Zool. 286, 179–184. doi: 10.1111/j.1469-7998.2011.00864.x
Peterson, M. N., Hartis, B., Rodriguez, S., Green, M., and Lepczyk, C. A. (2012). Opinions from the front lines of cat colony management conflict. PLoS One 7:e44616. doi: 10.1371/journal.pone.0044616
Prange, S., Gehrt, S. D., and Wiggers, E. P. (2003). Demographic factors contributing to high raccoon densities in urban landscapes. J. Wildl. Manag. 67, 324–333. doi: 10.2307/3802774
R Core Team. (2019). R: A Language and Environment for Statistical Computing. Vienna: R foundation for statistical computing.
Ridout, M. S., and Linkie, M. (2009). Estimating overlap of daily activity patterns from camera trap data. J. Agric. Biol. Environ. Stat. 14, 322–337. doi: 10.1198/jabes.2009.08038
Rowcliffe, J. M. (2019). activity: Animal Activity Statistics. R package version 1.3. Available online at: https://CRAN.R-project.org/package=activity (accessed February 24, 2021).
Rowcliffe, J. M., Field, J., Turvey, S. T., and Carbone, C. (2008). Estimating animal density using camera traps without the need for individual recognition. J. Appl. Ecol. 45, 1228–1236. doi: 10.1111/j.1365-2664.2008.01473.x
Royle, J. A. (2004). N−mixture models for estimating population size from spatially replicated counts. Biometrics 60, 108–115. doi: 10.1111/j.0006-341x.2004.00142.x
Sahwi, S. Y., Zaki, M. S., Haiba, N. Y., Elsaid, O. K., Anwar, M. Y., and AbdRabbo, S. A. (1995). Toxoplasmosis as a cause of repeated abortion. J. Obstet. Gynaecol. 21, 145–148. doi: 10.1111/j.1447-0756.1995.tb01087.x
Schiff, R., and Benoit, G. (2007). Effects of impervious cover at multiple spatial scales on coastal watershed streams. J. Am. Water Resour. Assoc. 43, 712–730. doi: 10.1111/j.1752-1688.2007.00057.x
Schmidt, P. M., Lopez, R. R., and Collier, B. A. (2007a). Survival, fecundity, and movements of free−roaming cats. J. Wildl. Manag. 71, 915–919. doi: 10.2193/2006-066
Schmidt, P. M., Lopez, R. R., and Pierce, B. L. (2007b). Estimating free-roaming cat densities in urban areas: comparison of mark-resight and distance sampling. Wildl. Biol. Pract. 3, 18–27.
Sims, V., Evans, K. L., Newson, S. E., Tratalos, J. A., and Gaston, K. J. (2008). Avian assemblage structure and domestic cat densities in urban environments. Divers. Distrib. 14, 387–399. doi: 10.1111/j.1472-4642.2007.00444.x
Slate, D. (1985). Movement, Activity and Home Range Patterns Among Members of a High Density Suburban Raccoon Population. Ph. D. dissertation. New Brunswick: Rutgers University.
Tennent, J., and Downs, C. T. (2008). Abundance and home ranges of feral cats in an urban conservancy where there is supplemental feeding: a case study from South Africa. Afr. Zool. 43, 218–229. doi: 10.3377/1562-7020-43.2.218
Thieurmel, B., and Elmarhraoui, A. (2019). suncalc: Compute Sun Position, Sunlight Phases, Moon Position and Lunar Phase. R package version 0.5.0. Available online at: https://CRAN.R-project.org/package=suncalc (accessed April 3, 2019).
Thomas, R. L., Fellowes, M. D., and Baker, P. J. (2012). Spatio-temporal variation in predation by urban domestic cats (Felis catus) and the acceptability of possible management actions in the UK. PLoS One 7:e49369. doi: 10.1371/journal.pone.0049369
van den Berg, R. A., Hoefsloot, H. C., Westerhuis, J. A., Smilde, A. K., and van der Werf, M. J. (2006). Centering, scaling, and transformations: improving the biological information content of metabolomics data. BMC Genomics 7:142. doi: 10.1186/1471-2164-7-142
Vazquez, C., Rowcliffe, J. M., Spoelstra, K., and Jansen, P. A. (2019). Comparing diel activity patterns of wildlife across latitudes and seasons: time transformations using day length. Methods Ecol. Evol. 10, 2057–2066. doi: 10.1111/2041-210x.13290
Walker, K. (2016). Tigris: an R package to access and work with geographic data from the US census bureau. R J. 8, 231–242. doi: 10.32614/rj-2016-043
Weather Underground. (2016). Historical Weather. Available online at: https://www.wunderground.com/history (accessed May 28, 2018).
Winter, L. (2004). Trap-neuter-release programs: the reality and the impacts. J. Am. Vet. Med. Assoc. 225, 1369–1376. doi: 10.2460/javma.2004.225.1369
Yang, L., Huang, C., Homer, C. G., Wyloe, B. K., and Coan, M. J. (2003). An approach for mapping large-area impervious surfaces: synergistic use of Landsat-7 ETM+ and high spatial resolution imagery. Can. J. Remote Sens. 29, 230–240. doi: 10.5589/m02-098
Yang, L., Jin, S., Danielson, P., Homer, C., Gass, L., Bender, S. M., et al. (2018). A new generation of the united states national land cover database: requirements, research priorities, design, and implementation strategies. ISPRS J. Photogramm. Remote Sens. 146, 108–123. doi: 10.1016/j.isprsjprs.2018.09.006
Keywords: abundance, domestic cat, distance sampling, camera trap, residential, urban ecology
Citation: Bennett KFP, Evans BS, Clark JA and Marra PP (2021) Domestic Cat Abundance and Activity Across a Residential Land Use Gradient. Front. Ecol. Evol. 9:643845. doi: 10.3389/fevo.2021.643845
Received: 18 December 2020; Accepted: 23 June 2021;
Published: 14 July 2021.
Edited by:
Christopher Lepczyk, Auburn University, United StatesReviewed by:
Christopher Richard Dickman, The University of Sydney, AustraliaSeth Magle, Lincoln Park Zoo, United States
Copyright © 2021 Bennett, Evans, Clark and Marra. This is an open-access article distributed under the terms of the Creative Commons Attribution License (CC BY). The use, distribution or reproduction in other forums is permitted, provided the original author(s) and the copyright owner(s) are credited and that the original publication in this journal is cited, in accordance with accepted academic practice. No use, distribution or reproduction is permitted which does not comply with these terms.
*Correspondence: Brian S. Evans, ZXZhbnNickBzaS5lZHU=
†These authors have contributed equally to this work and share first authorship