- 1Migratory Bird Center, Smithsonian Conservation Biology Institute, Washington, DC, United States
- 2University of California, Santa Cruz, Santa Cruz, CA, United States
- 3University of North Carolina at Chapel Hill, Chapel Hill, NC, United States
The ability of organisms to disperse across urban landscapes is theorized to be constrained by habitat fragmentation. While previous research has shown the distribution of forest patches is a determinant of dispersal patterns among forest-obligate bird species, the impacts of habitat distribution on the dispersal of “urban-adapted” species, has yet to be examined. Here, we use capture-reencounter data of birds banded over a 9-year period at six banding stations in greater Washington, DC to assess dispersal in four species of songbirds and a translocation experiment to examine the influence of land cover on movement. Point count and land cover data were used to construct habitat suitability and landscape permeability surfaces, with the latter representing potential travels costs from the capture location to the surrounding landscape. To assess how dispersal processes are affected by urban land cover, we searched for previously banded birds at sampling locations within 1.5 km of each banding station and compared the distribution of sampling locations with and without observations of previously-banded birds. We found evidence that settlement of two of four focal species, the Northern Cardinal (Cardinalis cardinalis) and Gray Catbird (Dumetella carolinensis), was more likely in sites with high relative permeability. To experimentally explore the consequences of the urban matrix habitat on movement, we attached radio transmitters to male Cardinals, translocated individuals 1.5 km across high-intensity urban, suburban, and forested landscapes, and recorded the time to return to their territory. Return time was dependent on land cover with Cardinals translocated across suburban habitats returning significantly faster than those moved across the other two land use classes. Combined, our findings suggest that, even among some “urban-adapted” species, dispersal within urban environments may be influenced by landscape structure and composition.
Introduction
Habitat fragmentation is hypothesized to be one of the primary mechanisms through which the expansion of urban environments has led to a global decline in biodiversity (McKinney, 2002). As environments are modified by urbanization, the area associated with available high quality habitat is often reduced for many species (i.e., habitat loss). While often treated synonymously in the literature, habitat loss describes the conversion of habitat by anthropogenic land use practices while habitat fragmentation is the physical breaking apart of once contiguous habitats (Wilcove et al., 1986). Fragmentation may physically isolate patches of habitat, which decreases the structural connectivity of a landscape and may impede the movement and dispersal of individuals (Fischer and Lindenmayer, 2007). Habitat modification due to urbanization therefore reduces habitat quality for many species and functionally isolates individuals and populations from portions of the landscape (Andren and Delin, 1994; Moilanen and Nieminen, 2002). The movement of individuals between birthplace and first breeding location (natal dispersal) or between successive breeding locations (breeding dispersal), is critical for metapopulation persistence through linking local populations and permitting gene flow across a landscape (e.g., Levins, 1969; Hanski, 1999; Clobert, 2001). As human-built habitats likely affect patterns of dispersal in many urban regions, determining how organisms disperse within such environments provides an important tool for understanding the impact of urbanization on wildlife populations (Crooks and Sanjayan, 2006).
Dispersal is described as a three-step process involving emigration from breeding or natal sites, movement between sites, and settlement to new breeding locations in a subsequent year (Weisser, 2001; Bowler and Benton, 2005). This process is more complex for migratory species, as conditions on wintering grounds have been found to determine the condition of individuals and the degree of natal dispersal in subsequent years (i.e., carry-over effects, e.g., Studds et al., 2008). Even among resident species, the costs associated with one stage of dispersal affect subsequent stages because the condition prior to emigration, the movement trajectory to new breeding locations, and the success of an individual following settlement are interdependent (Stamps et al., 2005; Clobert et al., 2009). For dispersal to be advantageous, the benefits of establishing new territories must outweigh the costs associated with increased predation risk, stress, and energy depletion for dispersing individuals (Greenwood and Harvey, 1982). Although both emigration and settlement are thought to be a function of multiple factors (e.g., conspecific density and patch size), movement is primarily dependent on an organism's perception of the landscape and the ability of an individual to travel through the inter-patch matrix (Baguette and Van Dyck, 2007). As such, the physical arrangement of habitat patches, the life history traits of the associated species, and the species-specific quality of the inter-patch matrix are expected to be determinants of whether individuals can successfully disperse between patches (Opdam et al., 1985; Urban and Keitt, 2001; Bélisle, 2005).
While the influence of habitat modification on the movement of organisms has often been considered largely a function of the physical distance between resource patches and the arrangement of those patches within a given landscape, the habitat encountered during transit is likely critical for dispersing birds (Haila, 2002; Ewers and Didham, 2006). The extent to which the movement of individuals may be facilitated or impeded by the resources and conditions encountered is described as landscape permeability, which is a continuous metric that is a determinant of the structural and functional connectivity of landscapes (e.g., Taylor et al., 1993; Kupfer et al., 2006; Lindenmayer and Fischer, 2006). The ability of organisms to move across landscapes fragmented by urbanization, and thus access patches of resources, is contingent both on the quality of the landscape matrix and species-specific response to landscape features (Wiens, 1976; Kotliar and Wiens, 1990; Pearson et al., 1996). For example, in the study of a single landscape, Bunn et al. (2000) found that their study landscape had high permeability for the American Mink (Mustela vison) and low permeability for the Prothonotary Warbler (Protonotaria citrea) due to their different dispersal abilities.
An organism's response to the landscape during dispersal is expected to be dependent on its life history traits as well as the landscape-specific costs and benefits associated with movement and settlement decisions (Stamps et al., 2005; Bonte et al., 2012; Burgess et al., 2012). Dispersal events that incorporate exploratory behaviors (e.g., as a consequence of foraging activities) are expected to minimize dispersal costs as exploration increases the efficiency by which individuals settle in suitable habitat, thus reducing the risk of unsuccessful dispersal (Baguette et al., 2013). For dispersal events that include exploratory behaviors, it is expected that the path taken between previous natal or breeding sites and settlement locations will be strongly affected by the distribution and quality (i.e., permeability) of habitat encountered during dispersal (Van Dyck and Baguette, 2005). As a result, patches of suitable habitat that are located within a matrix of low habitat quality are expected to have a lower probability of settlement. If the distance between patches exceeds the perceptual range of a species or patches are embedded within a low quality matrix, directed dispersal events (i.e., those that involve long distance straight-line movements) are expected to predominate and intervening land cover will have little effect on the probability that a location will be settled (Wolff et al., 1997; Mennechez et al., 2003). Directed dispersal events may be less energetically costly for dispersing individuals but may increase the risk of unsuccessful dispersal (Zollner and Lima, 1999; Bonte et al., 2012).
Direct studies of animal movement within fragmented landscapes demonstrate that land cover features are critical to constraining or facilitating travel. For example, previous research using simulated territorial intrusion (Awade and Metzger, 2008), simulated predation threat (Bélisle and Desrochers, 2002), and radio-telemetry (Bayne and Hobson, 2001) have shown that forest bird species exhibit behavioral avoidance when crossing open habitats (i.e., gap-crossing decisions). At larger spatial scales, patterns of seed dispersal and translocation experiments have provided proximate evidence that birds utilize corridors of habitat when moving across fragmented landscapes (e.g., Haddad et al., 2003; Carlo et al., 2013) and that avian movement is impacted by the degree of human land use intensity (e.g., Kennedy et al., 2010). At still larger scales, graph models, which are network models in which landscapes are delineated into interconnected habitat patches, have provided a link between bird dispersal behavior and the structure and composition of landscapes (Bunn et al., 2000; Urban and Keitt, 2001; Minor and Urban, 2008). The applicability of graph models, however, is dependent on the patchiness of the landscape and may be difficult to apply in regions with large contiguous habitat patches or for species that utilize the inter-patch matrix (Minor E.S., personal communication). Despite evidence that urban land cover mediates movement, there remain comparatively few studies that empirically link movement and land cover to dispersal events in urban environments (but see Delgado et al., 2010) and none to our knowledge that have assessed the impacts of land cover on the dispersal of species common to urban and suburban habitats.
Here, we consider the breeding dispersal syndromes of four songbird species within urban landscapes of varying intensity in metropolitan Washington, DC, USA. Our study species, which include the American Robin (Turdus migratorius), Gray Catbird (Dumetella carolinensis), House Sparrow (Passer domesticus), and Northern Cardinal (Cardinalis cardinalis), co-occur across the rural-to-urban gradient but represent distinctly different life history strategies (e.g., resident species and short-distance and Neotropical migrants) and are thus expected to have a differential response to the composition and structure of landscapes. We address the breeding dispersal of these species using two levels of inference—a mark-recapture study to assess the influence of landscape permeability on settlement patterns and a translocation experiment to evaluate the effect of rural, suburban, and high-intensity urban land cover on movement. We test the hypothesis that landscape permeability affects settlement patterns by assessing the spatial distribution of previously marked individuals at sampling locations within a 1.5 km radius of six banding stations. We predicted that the likelihood of settlement of a sampling location is positively correlated with landscape permeability due to exploratory movements during the dispersal process. Because events outside of the breeding landscape have been found to strongly affect the settlement patterns of migrant species (e.g., Studds et al., 2008), we expected that permeability will be a greater determinant of settlement patterns for resident (Northern Cardinal and House Sparrow) than migrant birds (American Robin and Gray Catbird) in urban environments. We further examined the impact of land cover on the movement phase of dispersal by conducting a translocation experiment across landscapes of differing urban intensity with one of our focal species, the Northern Cardinal.
Materials and Methods
To assess patterns of breeding dispersal, birds color-banded at six banding stations in metropolitan Washington DC between the years of 2003 and 2011 were resighted during the breeding seasons of 2011 and 2012. Banded birds were aged by plumage and skull ossification (see Pyle, 1997)—because hatch year birds are expected to have dispersal kernel extents that exceed the spatial extent of our sampling, only adult birds banded in previous years were maintained for this analysis. Banding stations were representative of rural (Rock Creek Park, Maryland), suburban (Spring and Opal Daniels Parks Takoma Park, Maryland, Bethesda Maryland), and high-intensity urban (Foggy Bottom and the National Mall in Washington, DC) landscapes. For each banding station, the surrounding area, hereafter referred to as the study landscape, was binned into four distance classes (the banding location, 150–500 m, 500–1,000 m, and <1,000–1,500 m) and four directional classes, representing each cardinal direction. Sampling locations were selected at random from each directional and distance bin with the number of samples per bin stratified by distance such that an equal proportional area was sampled within each bin. The sampling extents for Spring and Opal Daniels Parks were partially overlapping—because color-banded birds that were banded at either station can be resighted, these landscapes contain more sampling locations within the area of overlap than do the other study landscapes. As our study region is predominantly composed of privately-owned land, sampling locations were adjusted when possible to the nearest accessible area and a minimum distance of 100 m was maintained between samples. For each sampling location, a minimum of 20 min, when possible, was spent searching for color-banded birds within an area of 50 m from the center of the sample using playback of conspecific song and mobbing calls. Time spent resighting averaged 36 min per sampling location and ranged from a minimum of 13 min to a maximum of 70 min. Resighted birds were identified by color bands and their location was recorded using a handheld GPS.
To evaluate how landscape permeability affects settlement patterns, we estimated habitat suitability using fixed radius (50 m) point count data and land cover metrics expected to be representative of habitat preference. Point counts were conducted during the breeding season between 2009 and 2012 by technicians as a part of the Neighborhood Nestwatch program at 102 sites across the rural-to-urban gradient within the Washington DC metropolitan area (for full description of point count methodology, see Evans, 2015). Proportional canopy cover and impervious surface (30 m resolution, Xian et al., 2011) were used as proxy variables in constructing habitat suitability models. While we acknowledge that these variables do not represent the totality of habitat variation along the rural-to-urban gradient, these land cover metrics have been shown to be predictive of avian nest success (canopy cover and impervious surface, Ryder et al., 2010), adult survival (impervious surface, Evans et al., 2015), and community composition (impervious surface, Evans, 2015). All spatial analyses were carried out in R using packages raster (Hijmans, 2015) and sp (Pebesma and Bivand, 2005). We calculated the proportional cover of impervious surface and canopy within a 3 × 3 cell neighborhood moving window (for R code used throughout this manuscript, see Supplementary Material). Because several of our species are considered to be edge specialists, we also calculated the standard deviation of canopy cover values for each 3 × 3 cell neighborhood (root mean squared, RMS). This metric is considered a continuous analog of categorical habitat heterogeneity as high standard deviation in canopy cover values relates to habitats associated with forest edges (see McGarigal et al., 2009).
We used the N-mixture models of Royle et al. (2004) in the R package unmarked (function pcount; Fiske and Chandler, 2011) to model the habitat suitability for each species as a function of land cover metrics. Point count data were subset such that only sites with a minimum of three counts were evaluated. Because detection likely varies by observer, we included observer within the detection parameter of abundance models. As species may show a differential response to canopy cover at different levels of development (impervious surface) and degrees of heterogeneity (standard deviation of canopy cover), interaction terms were included between these variables and proportional canopy cover as well as an interaction term between impervious surface and heterogeneity. Akaike's Information Criteria, adjusted for small sample size (AICc), were used to assess models (Burnham and Anderson, 2002). To evaluate the explanatory power of models, we used Nagelkerke's (2004) R-squared index. To avoid overemphasizing potentially spurious relationships between explanatory variables and avian abundance, and because the purpose of constructing these models was to develop habitat suitability surfaces rather than determining which explanatory variables best explain avian distributions, we used model averaging of our symmetrical model set to predict habitat suitability across each sample landscape (Figure 1; see Burnham and Anderson, 2002; Lukacs et al., 2010; Mazerolle, 2015). Habitat suitability values were estimated for each focal species and scaled from zero to one for each landscape surrounding banding stations, with zero representing unsuitable habitat, and one for suitable habitat.
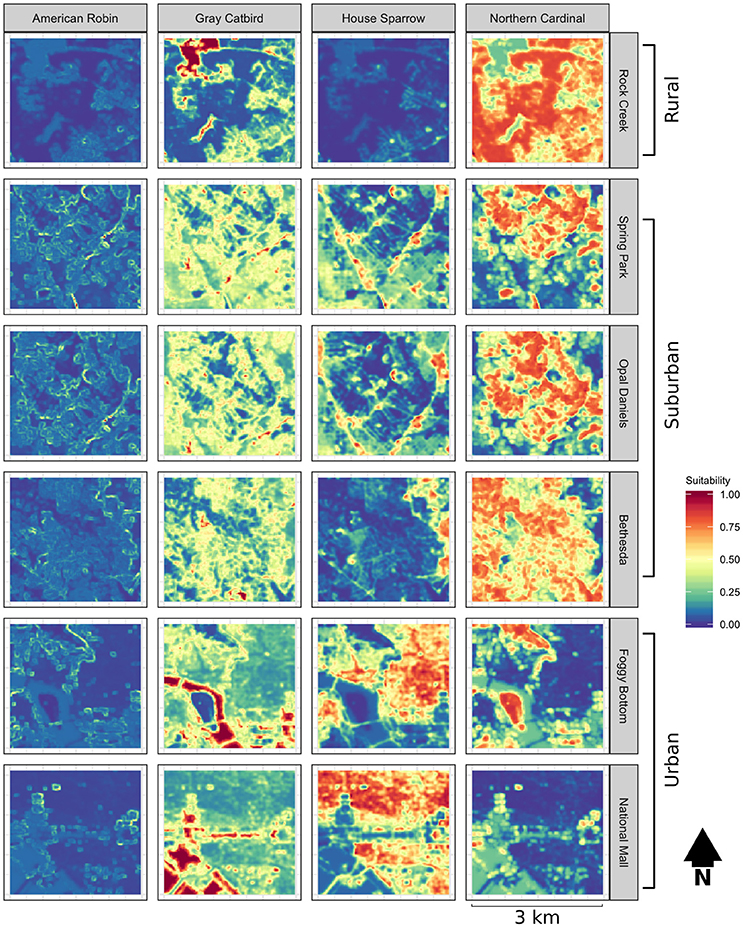
Figure 1. Habitat suitability estimates obtained from point count data, scaled from 0 to 1, for sample landscapes encompassing rural, suburban, and urban habitat types.
Habitat suitability models were used to estimate the permeability from the banding station to surrounding raster cells for each study landscape using the package gDistance (functions transition, geoCorrection, and accCost; Van Etten, 2015). We calculated a transition matrix between adjacent raster cells (queen's case) weighted by the inverse of the habitat suitability rasters—thus suitability provides a measure of conductance between cells (see McRae et al., 2008). Transition matrices were used to develop a cost distance raster representing the walk distance, in meters, between the banding station and all surrounding cells with the probability of an individual moving between cells weighted by habitat suitability. Because cost distance increased and the prevalence of resighted birds decreased as a function of the Euclidean distance from the banding station, we defined the permeability for cells within study landscapes as the ratio of the cost distance and random walk distance between the banding station and each raster cell. Thus, permeability rasters represent the functional distance between capture and all cells within a given study landscape after removing the effect of Euclidean distance. Because there was considerable variation among study landscapes in suitability and permeability, rasters were scaled (z-scores) such that cell values are provided relative to all other values within a given study landscape.
To test our prediction that habitat permeability impacts settlement patterns, we compared models of dispersal in which sampling locations were occupied by banded birds as a function of the habitat suitability of the settlement location and the permeability between the banding station and sampling location for each study landscape. Crucially, the level of observation for this study is the sampling locations rather than resighted birds. Therefore, while a single sampling location may include several resighted individuals, each location is only representative of one positive sample per species resighted. Sampling locations within each landscape were subset such that samples within 150 m of the center of the banding station were not considered to have dispersed. This distance, while conservative, may be representative of within-territory movement of birds maintaining the same territories in subsequent years—thus patterns of observations of previously banded birds in this distance range likely result from biological processes other than dispersal. Additionally, not all species were resighted across all study landscapes—only sampling locations within a landscape with a resight for a given species were included in our analysis. Permeability and habitat suitability raster values were extracted to each sampling location and the influence of these variables were assessed in a mixed effect logistic regression framework in the R package lme4 (Bates et al., 2015). We developed a candidate set of models that included study landscape as a random effect and the fixed effects of habitat suitability, permeability, and the additive and multiplicative effects of these predictors. We evaluated support for the effect of permeability on settlement locations by comparing ΔAICc scores and evidence ratios of models with and without this variable (see Arnold, 2010; Burnham et al., 2011).
To further assess dispersal across matrices of varying urban intensity, we explored the influence of land cover on movement by conducting a translocation experiment across landscapes representing rural, suburban, and high-intensity urban land use classes. This experiment provides us with proximate evidence of how dispersal patterns in human-dominated landscapes might be shaped by variation in the movement of birds in response to human land use practices. We conducted the experiment on eight male Northern Cardinals captured at the banding stations during the breeding seasons of 2011 and 2012. Northern Cardinals were chosen because they were the most frequently re-encountered birds among our focal species and exhibit the highest degree of territoriality. On each bird, we attached a 0.39 g VHF radio transmitter (Advanced Telemetry Systems, Isanti, MN) using a backpack harness of elastic thread (Rappole and Tipton, 1991). Birds were translocated 1.5 km from the capture location across one of eight pre-determined landscapes, and tracked for a period of no less than 0.5 h twice per day following release. The location of the bird was determined using triangulation and, when possible, visible identification of the individual. To assess the effect of intervening land cover on movement, we then calculated the latency (days-to-return) for each individual. Behavioral latency was evaluated as a function of the three land use classes within a generalized linear model framework (Poisson distribution) and assessed using AICc as above. We were unable to observe a return for two of the eight individuals, one translocated across a forested habitat matrix and the other across a suburban matrix. Only three of the eight individuals were able to be tracked while moving to their capture location.
Results
Habitat suitability models developed from point count data show species-specific differences in suitability across study landscapes. The fit of abundance models was highest for the House Sparrow and Northern Cardinal (pseudo-R2 = 0.97 and 0.72, respectively) and lowest for the American Robin (0.50) and Gray Catbird (0.65, see Table 1 for abundance model selection). Raster predictions of American Robin habitat suitability exhibited considerably lower variability than that of the remaining species (Figure 1). These results suggest that, given the environmental variables used to develop these models, the Northern Cardinal and House Sparrow are found in a narrower portion of environmental niche space within our study landscapes than are the Gray Catbird and American Robin.
Among 2,706 identifiable adult birds that were color-banded at the six banding stations, a total of 127 birds were re-encountered in this study, with 70 individuals observed at a distance of greater than 150 m from the banding station (Table 2). This yielded a total of 53 sampling locations greater than 150 m from the initial capture location with resighted color-banded birds. Observed settlement patterns highlight species-specific differences in how landscape permeability and habitat suitability affect dispersal, with supportive evidence for our prediction that patterns of settlement would reflect the permeability between capture and resight locations for two of our four focal species (Table 3, Figures 2, 3). There was model support that the probability of settlement was higher in sites with high landscape permeability for the Northern Cardinal and Gray Catbird, with evidence ratios of 17.0 and 17.5, respectively, relative to the best performing model that excluded this variable. These results support the hypothesis of dispersal as a consequence of exploratory movements. There was no evidence that American Robin and House Sparrow settlement patterns were impacted by permeability, as the null and permeability models received equivalent support for these species. As such, there was no support for our hypothesis that intervening land cover is a determinant of American Robin and House Sparrow dispersal behavior. Additionally, as Northern Cardinal and House Sparrow are resident species, and Gray Catbird and American Robin are migrants, our results did not fit our expectation that residents would be more likely to be influenced by landscape permeability.
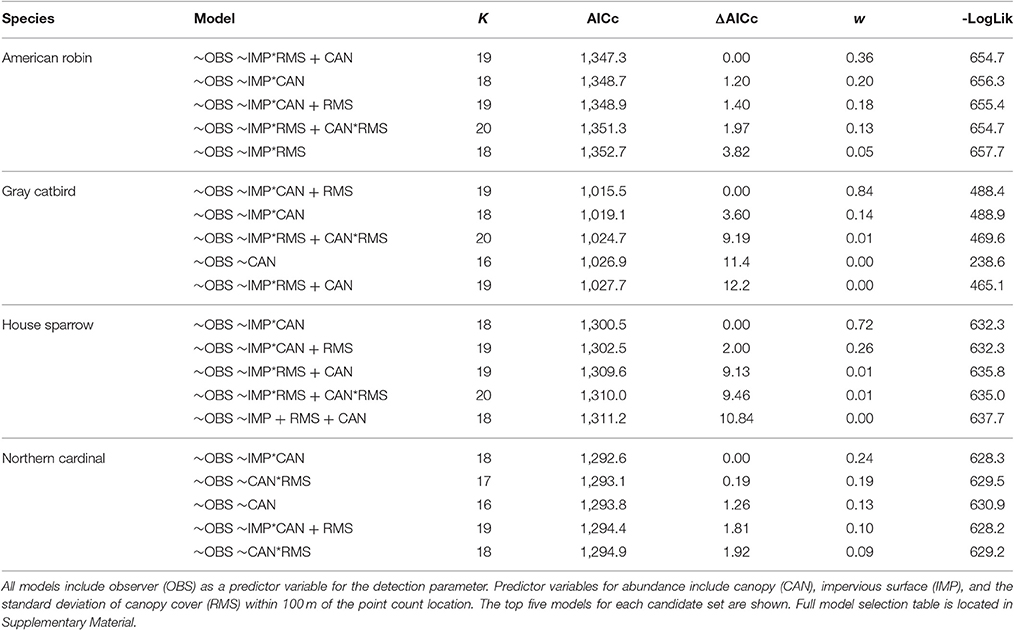
Table 2. Model selection table for distance models used to estimate the abundance of four focal species at sites throughout the Washington D.C. metropolitan region.
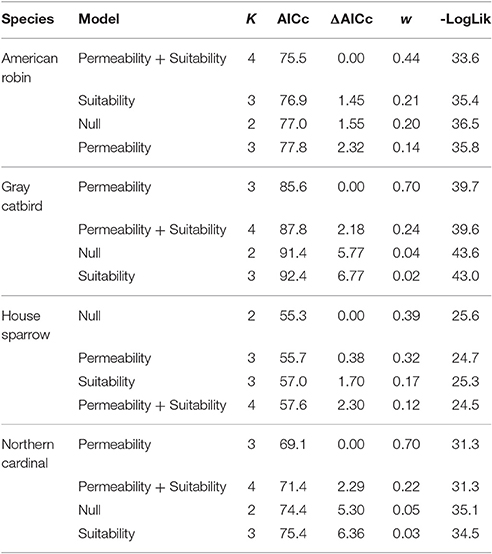
Table 3. Model selection table for logistic mixed effects models describing the probability that settlement will occur between the banding station and a sampling location as a function of habitat suitability and permeability between the two points.
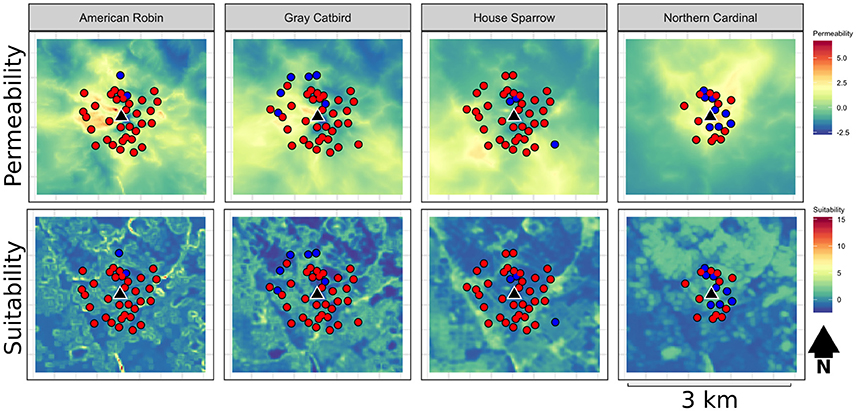
Figure 2. Example habitat suitability and permeability raster maps, in addition to sampling locations with (blue) and without (red) resighted individuals for each of our four focal species surround the suburban banding station located at Spring Park in Takoma Park, Maryland. See Supplementary Figure 1 for suitability and permeability maps across banding stations.
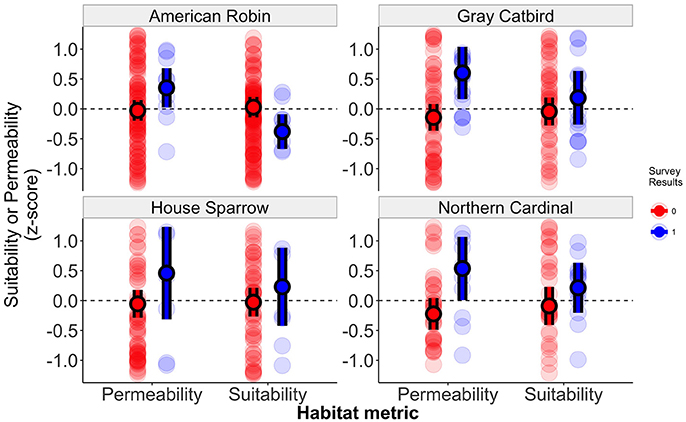
Figure 3. Habitat connectivity and suitability of sites in which birds were resighted or in which no banded birds were observed. Standardized effect sizes represent habitat connectivity and suitability values for each banding station, with values above zero representing samples with high suitability or connectivity. Solid points represent mean values for each category with error bars representing 95% confidence intervals about the mean. Semi-transparent points represent the observed data.
Our translocation experiment supported the prediction that movement patterns of the Northern Cardinal are influenced by the degree of urban intensity between capture and release locations. Cardinals translocated 1.5 km over suburban landscapes returned in 1 and 3 days, whereas those that were moved over forested landscapes returned in 5 and 7 days and those moved over high-intensity urban landscapes returned in 9 and 14 days. Despite sample size limitations, the model that included land cover variables as a predictor of days-to-return received considerably more support than the null model (ΔAICc = 7.24). We tracked movements over a portion of the route for both of the suburban Cardinals and one of the urban Cardinals, while the remaining individuals were not able to be observed until they returned to their respective capture locations. Movements of suburban Cardinals predominantly followed forest edges, when available, as they returned to the capture location. The high-intensity urban Cardinal moved a distance of roughly 0.5 km on the day following release and remained at that site for 8 days until traveling back to the capture location in a single flight.
Discussion
This study addresses the impact of urbanization on the dispersal syndromes of birds through environments of varying urban intensity—we assess patterns of settlement of migrant and resident birds one or more years after banding to evaluate dispersal, and the return time of Northern Cardinals translocated across rural, suburban, and high-intensity urban environments to explore the influence of urban land cover on movement. The settlement patterns of banded Gray Catbird and Northern Cardinal in relation to the habitat suitability and permeability of the landscape provide support for our prediction that landscape permeability impacts the dispersal of individuals within urbanized environments. In conjunction with the results of our translocation experiment, these findings provide evidence for a link between the structural and functional connectivity of urban landscapes (Tischendorf and Fahrig, 2000; Schooley and Wiens, 2003; Kindlmann and Burel, 2008).
Our observation that the behavioral latency of translocated Northern Cardinal is affected by urban intensity is comparable to those of previous translocation experiments. For example, Kennedy et al. (2010) found the return time and success of translocated American Redstart (Setophaga ruticilla) and Jamaican Todies (Todus todus) was dependent on human land use practices, with the days-to-return for individuals increasing with the intensity of anthropogenic habitat modification. In our study, Northern Cardinals were observed to have the shortest return time when moved across suburban habitats, habitats which likely represent high suitability for this species (see Evans, 2015). Cardinals exhibited intermediate return times across forested habitats and the longest return time across the most heavily urban landscapes, which reflect moderate and low estimated habitat suitability, respectively. The influence of forested habitat on Cardinal return time especially underscores the species-specific nature of landscape permeability as Northern Cardinal tend to occupy forest edge and open or shrub-dominated at comparatively high densities relative to intact forest (see Leston and Rodewald, 2006). It would therefore not be expected that a forested landscape would be highly connected for this species (see Wiens, 1989; Pearson et al., 1996). Indeed, for the Northern Cardinal in our study region habitat “patches” largely represent suburban environments fragmented by forests. An important caveat to our results, however, is that the movement patterns observed in a translocation experiment may not be representative of movement during breeding dispersal events, as the motivation and behavior during transit may differ considerably between dispersing and translocated individuals (see Betts et al., 2015). Despite this limitation, translocations provide proxy information regarding how animals move through urban landscapes—used in conjunction with patterns of settlement, the results of our translocation experiment support our hypothesis that urban land cover shapes Northern Cardinal dispersal patterns. Inference from our translocation analysis, however, is constrained considerably by low sample size and our inability to track birds throughout their return to their breeding territories (see Zeller et al., 2012).
The permeability of urban landscapes may be a determinant of dispersal patterns if individuals exhibit behavioral avoidance of some habitat features (i.e., matrix resistance and boundary effects, Haddad, 1999; Ricketts, 2001) or if the travel phase of dispersal occurs as a consequence of routine behavior, such as during foraging activities (Baguette and Van Dyck, 2007). Observations of gap-crossing behavior suggest that Northern Cardinal do not avoid gaps and thus are not expected to exhibit behavioral avoidance of the urban matrix (Grubb and Doherty, 1999). Likewise, translocated Northern Cardinals within the current study were observed to travel greater distances when intervening land cover was of low suitability, even when locations were available to minimize the flight distances (e.g., shrub or tree perches). This may suggest that, while landscape conductance and resistance are mathematically reciprocal, and thus either would yield equivalent settlement patterns, behavioral motivation during transit is more likely representative of exploratory behavior rather than avoidance. The influence of landscape permeability on the settlement patterns of the migratory Gray Catbird may also be a consequence exploratory movement. Because territories are established soon after arrival to the breeding grounds, and the post-breeding period has been found to involve considerable landscape exploration during foraging activities (e.g., Rappole and Ballard, 1987; Heise and Moore, 2003), it is likely that the influence of intervening land cover on dispersal reflects exploratory movement rather than behavioral avoidance of matrix habitat.
While our data support the hypothesis that dispersal is influenced by habitat between banding and settlement locations for two of our study species, behavioral motivation (e.g., exploratory movements) for habitat selection during the movement phase of dispersal events could not be directly assessed. Motivation for landscape resistance or conductance is dependent on the behavioral state of dispersing individuals. For example, in their study of the movement of pumas in southern California, Zeller et al. (2014) found that pumas exhibited strong behavioral avoidance of agricultural and urban areas during resource use (e.g., foraging), as evaluated by the path tortuosity of radio-tagged animals. Settlement locations provide insufficient evidence for behavioral motivation and, because we were largely unable to follow translocated Cardinals throughout their return to their respective banding locations, we were unable to assess the behavioral state of radio-tagged individuals. Moreover, we acknowledge that behaviors, such as conspecific attraction, impact movement and settlement decisions and these behaviors are not observable with settlement data alone. It therefore remains uncertain which mechanisms best explain the observed settlement patterns, especially as the path taken between breeding patches cannot be known within this context. Likewise, low reencounter rates may suggest that our study was necessarily biased toward short-distance dispersers, which are expected to be more responsive to landscape pattern (Van Dyck and Baguette, 2005). Thus there may be multiple behavioral strategies driving movement within our sampled population. These caveats highlight a need to track individuals during the movement phase of dispersal, assess dispersal events across the annual cycle of birds, and expand the spatial extent of sampling in order to develop a better understanding of the dispersal syndromes of our study species.
Though we failed to observe a direct effect of habitat suitability on settlement patterns, the low explanatory power of suitability within our dispersal models is representative only of resighted birds, not of the occupancy distribution as a whole. Indeed, while sample locations spanned a range of suitability values, samples with and without resighted birds were often occupied by unbanded individuals of each species. As dispersing birds typically encounter other territory-holders when attempting to establish a new breeding territory, it is therefore unsurprising that we observed no influence of suitability on patterns of settlement. Alternatively, lack of observed influence of habitat suitability may also be representative of limitations in the use of point count data to estimate suitability within our study region. Because point counts, especially those conducted in residential areas where private lands predominate (see Evans, 2015), are able to sample only a subset of a given landscape, the accuracy of estimates may be limited in un-sampled and under-sampled portions of the evaluated environmental gradients. This limitation is exemplified by suitability values for the Gray Catbird in the Foggy Bottom study landscape, in which open water (the Potomac River) was estimated to have much higher suitability than the surrounding region (see Figure 2).
Dispersal syndromes for the House Sparrow and American Robin were not shown to be influenced by habitat permeability. Indeed, we found no evidence that American Robin settlement patterns were affected by either landscape permeability or habitat suitability. The low explanatory power of American Robin dispersal models may have been driven in part by low variation in predicted habitat suitability of our study landscapes (Figure 1). For House Sparrow, however, the observed landscapes had low suitability overall, and this species was only observed at sampling locations with considerable urban development. It is expected the overall degree of suitability of a landscape is a determinant of whether differences in permeability may impact dispersal. Conversely, the lack of an observed effect of landscape permeability for these species may also be a consequence of a differential behavioral response to the urban environment, such as low predator avoidance behavior (observed in House Sparrow, Tsurim et al., 2008). Our results may also represent a response to the landscape that occurs at a larger spatial grain and extent than accounted for in this study. For example, the breeding home range size of the resident House Sparrow is estimated to encompass several kilometers (Bennett, 1990) and previous findings have shown low rates of dispersal for this species (Altwegg et al., 2000; Pärn et al., 2009). Therefore, the distribution of resighted House Sparrows may represent observations within individuals' home ranges rather than patterns of dispersal. Likewise, settlement patterns observed during the breeding season reflect components of dispersal (i.e., emigration, movement, and settlement) that may occur at varying spatial scales across birds' annual cycles. For example, although the American Robin typically holds territory sizes roughly equivalent to the Northern Cardinal and Gray Catbird during the breeding season, subsequent to breeding this species often forms wide-ranging foraging flocks (Janousek et al., 2014; Vanderhoff et al., 2014; Rodewald, 2015). Therefore, movement outside of the breeding period may increase an individual's perceptual range of its environment. Finally, the sample size for these species was limited relative to that of the Gray Catbird and Northern Cardinal—this may suggest a dispersal kernel that is considerably larger than the 1.5 km radius sampled in this study. Cumulatively, the results for American Robin and House Sparrow emphasize that determining the influence of habitat composition and structure on dispersal necessitates investigating the scale by which species respond to their environment (Wiens, 1989).
Conclusion
With the spread of urbanization and associated fragmentation of urban landscapes, the maintenance of functional connectivity is increasingly recognized as critical for supporting biodiversity (Wiens, 1997). To date, few have addressed patterns of dispersal across urban environments (see LaPoint et al., 2015) and research on how habitat fragmentation influences bird dispersal has reflected a strong bias toward forested environments and forest specialist species (Bayard and Elphick, 2010). As ecological patterns in forested habitats may not be applicable across habitat types (see Rudnicky and Hunter, 1993), the current study provides an important step toward understanding the impact of humans on ecological processes that are shaping bird populations and communities with the expansion of urban landscapes. Overall, for two of our four focal species, our results support the hypothesis that the permeability of landscapes is a determinant of dispersal in urban environments and thus structural components of urban habitats likely govern the functional connectivity of these landscapes. While we acknowledge that patterns of settlement are not directly representative of the path taken between capture and settlement location and, likewise, translocation experiments may not adequately reflect the behavior of organisms during dispersal, our results offer key evidence that land cover associated with urban environments affect avian dispersal syndromes. Despite the unprecedented spatial distribution of resighting effort in this study, we were constrained by the number of banded birds across species, we were able to re-encounter—this suggests limited applicability of using resighting data to identify short-distance dispersal events. With recent technological advancements in the study of birds, it is now becoming feasible to track individuals throughout a bird's annual cycle—including the processes of emigration, movement, and settlement that comprise dispersal events (see Bridge et al., 2011; Hallworth and Marra, 2015). Such advances will greatly increase our understanding of the influence of landscape structure and composition on dispersal across scales.
Ethics Statement
This research project was approved by the Smithsonian Conservation Biology Institute's Institutional Animal Care and Use Committee. All precautions were taken to minimize any potential stress during handling, including checking nets at a minimum frequency of once per 15 min, handling birds for as short a time as possible, and banding only under optimal weather conditions (e.g., temperatures of less than 32°C). We observed no mortality while conducting this study.
Author Contributions
BE: Primary author, conducted field work (banding and resight), sampling design for resight activities, and statistical analysis. AK: Second author, provided major edits to manuscript and its proposal, developed sampling design for banding activities, conducted field work (banding). AH: Third author, provided major edits to each draft of the manuscript and its proposal, including additional text, statistical advising, and sample design advising. PM: Fourth author, provided major edits to each draft of the manuscript and its proposal, including additional text, and sample design advising.
Funding
BSE was awarded a fellowship from the Smithsonian Fellowship Program for resighting activities and statistical analyses. Banding data was funded by National Institute of Allergy and Infectious Disease contract NO1-AI-25490, grant 2003–0209–000 from the National Fish and Wildlife Foundation, and by core funding to the Consortium for Conservation Medicine at Wildlife Trust from the V. Kann Rasmussen Foundation.
Conflict of Interest Statement
The authors declare that the research was conducted in the absence of any commercial or financial relationships that could be construed as a potential conflict of interest.
Acknowledgments
We thank the many field researchers who contributed to this project. We also thank Thomas B. Ryder and manuscript reviewers whose helpful comments on earlier versions of this work greatly improved our manuscript.
Supplementary Material
The Supplementary Material for this article can be found online at: http://journal.frontiersin.org/article/10.3389/fevo.2017.00063/full#supplementary-material
Supplementary Figure 1. Boxplot displaying the distance between the initial banding location and the locations of resighted birds for each of our four target species.
References
Altwegg, R., Ringsby, T. H., and SÆther, B. E. (2000). Phenotypic correlates and consequences of dispersal in a metapopulation of house sparrows Passer domesticus. J. Anim. Ecol. 69, 762–770. doi: 10.1046/j.1365-2656.2000.00431.x
Andren, H., and Delin, A. (1994). Habitat selection in the eurasian red squirrel, Sciurus vulgaris, in relation to forest fragmentation. Oikos 70, 43–48. doi: 10.2307/3545697
Arnold, T. W. (2010). Uninformative parameters and model selection using Akaike's Information Criterion. J. Wildlife Manage. 74, 1175–1178. doi: 10.1111/j.1937-2817.2010.tb01236.x
Awade, M., and Metzger, J. P. (2008). Using gap-crossing capacity to evaluate functional connectivity of two Atlantic rainforest birds and their response to fragmentation. Aust. Ecol. 33, 863–871. doi: 10.1111/j.1442-9993.2008.01857.x
Baguette, M., Blanchet, S., Legrand, D., Stevens, V. M., and Turlure, C. (2013). Individual dispersal, landscape connectivity and ecological networks. Biol. Rev. 88, 310–326. doi: 10.1111/brv.12000
Baguette, M., and Van Dyck, H. (2007). Landscape connectivity and animal behavior: functional grain as a key determinant for dispersal. Landsc. Ecol. 22, 1117–1129. doi: 10.1007/s10980-007-9108-4
Bates, D., Maechler, M., Bolker, B., and Walker, S. (2015). Fitting linear mixed-effects models using lme4. J. Stat. Softw. 67, 1–48. doi: 10.18637/jss.v067.i01
Bayard, T. S., and Elphick, C. S. (2010). How area sensitivity in birds is studied. Conserv. Biol. 24, 938–947. doi: 10.1111/j.1523-1739.2010.01480.x
Bayne, E. M., and Hobson, K. A. (2001). Movement patterns of adult male ovenbirds during the post-fledging period in fragmented and forested boreal landscapes. Condor 103, 343–351. doi: 10.1650/0010-5422(2001)103[0343:MPOAMO]2.0.CO;2
Bélisle, M. (2005). Measuring landscape connectivity: the challenge of behavioral landscape ecology. Ecology 86, 1988–1995. doi: 10.1890/04-0923
Bélisle, M., and Desrochers, A. (2002). Gap-crossing decisions by forest birds: an empirical basis for parameterizing spatially-explicit, individual-based models. Landsc. Ecol. 17, 219–231. doi: 10.1023/A:1020260326889
Bennett, W. A. (1990). Scale of investigation and the detection of competition: an example from the house sparrow and house finch introductions in North American. Am. Nat. 135, 725–747. doi: 10.1086/285071
Betts, M. G., Gutzwiller, K. J., Smith, M. J., Robinson, W. D., and Hadley, A. S. (2015). Improving inferences about functional connectivity from animal translocation experiments. Landsc. Ecol. 30, 585–593. doi: 10.1007/s10980-015-0156-x
Bonte, D., Van Dyck, H., Bullock, J. M., Coulon, A., Delgado, M., Gibbs, M., et al. (2012). Costs of dispersal. Biol. Rev. 87, 290–312. doi: 10.1111/j.1469-185X.2011.00201.x
Bowler, D. E., and Benton, T. G. (2005). Causes and consequences of animal dispersal strategies: relating individual behaviour to spatial dynamics. Biol. Rev. 80, 202–225. doi: 10.1017/S1464793104006645
Bridge, E. S., Thorup, K., Bowlin, M. S., Chilson, P. B., Diehl, R. H., Fleron, R. W., et al. (2011). Technology on the move: recent and forthcoming innovations for tracking migratory birds. Bioscience 61, 689–698. doi: 10.1525/bio.2011.61.9.7
Bunn, A. G., Urban, D. L., and Keitt, T. H. (2000). Landscape connectivity: a conservation application of graph theory. J. Environ. Manage. 59, 265–278. doi: 10.1006/jema.2000.0373
Burgess, S. C., Tremel, E. A., and Marshal, D. J. (2012). How do dispersal costs and habitat selection influence realized population connectivity? Ecology 93, 1378–1387. doi: 10.1890/11-1656.1
Burnham, K. P., and Anderson, D. R. (2002). Model Selection and Inference. A Practical Information-Theoretic Approach. New York, NY: Springer.
Burnham, K. P., Anderson, D. R., and Huyvaert, K. P. (2011). AIC model selection and multimodel inference in behavioral ecology: some background, observations, and comparisons. Behav. Ecol. Sociobiol. 65, 23–35. doi: 10.1007/s00265-010-1029-6
Carlo, T. A., García, D., Martínez, D., Gleditsch, J. M., and Morales, J. M. (2013). Where do seeds go when they go far? Distance and directionality of avian seed dispersal in heterogeneous landscapes. Ecology 94, 301–307. doi: 10.1890/12-0913.1
Clobert, J., Le Galliard, J. F., Cote, J., Meylan, S., and Massot, M. (2009). Informed dispersal, heterogeneity in animal dispersal syndromes and the dynamics of spatially structured populations. Ecol. Lett. 12, 197–209. doi: 10.1111/j.1461-0248.2008.01267.x
Crooks, K. R., and Sanjayan, M. (eds.) (2006). “Connectivity conservation: maintaining connections for nature,” in Connectivity Conservation (New York, NY: Cambridge University Press), 1–10. doi: 10.1017/CBO9780511754821.001
Delgado, M. D. M., Penteriani, V., Revilla, E., and Nams, V. O. (2010). The effect of phenotypic traits and external cues on natal dispersal movements. J. Anim. Ecol. 79, 620–632. doi: 10.1111/j.1365-2656.2009.01655.x
Evans, B. S. (2015). The Ecology of Birds in the Urban Landscape: Avian Community Composition, Dispersal, and Survival Across the Rural-to-Urban Gradient in Washington DC [dissertation]. Chapel Hill, NC, University of North Carolina at Chapel Hill.
Evans, B. S., Ryder, T. B., Reitsma, R., Hurlbert, A. H., and Marra, P. P. (2015). Characterizing avian survival along a rural-to-urban land use gradient. Ecology 96, 1631–1640. doi: 10.1890/14-0171.1
Ewers, R., and Didham, R. (2006). Confounding factors in the detection of species responses to habitat fragmentation. Biol. Rev. 81, 117–142. doi: 10.1017/S1464793105006949
Fischer, J., and Lindenmayer, D. B. (2007). Landscape modification and habitat fragmentation: a synthesis. Glob. Ecol. Biogeogr. 16, 265–280. doi: 10.1111/j.1466-8238.2007.00287.x
Fiske, I., and Chandler, R. (2011). unmarked: An R Package for Fitting Hierarchical Models of Wildlife Occurrence and Abundance. J. Stat. Softw. 43, 1–23. doi: 10.18637/jss.v043.i10
Greenwood, P. J., and Harvey, P. H. (1982). The natal and breeding dispersal of birds. Annu. Rev. Ecol. Syst. 13, 1–21. doi: 10.1146/annurev.es.13.110182.000245
Grubb, T. C., and Doherty, P. F. Jr. (1999). On home-range gap-crossing. Auk 116, 618–628. doi: 10.2307/4089323
Haddad, N. M. (1999). Corridor use predicted from behaviors at habitat boundaries. Amer. Nat. 153, 215–2277. doi: 10.1086/303163
Haddad, N. M., Bowne, D. R., Cunningham, A., Danielson, B. J., Levey, D. J., Sargent, S., et al. (2003). Corridor use by diverse taxa. Ecology 84, 609–615. doi: 10.1890/0012-9658(2003)084[0609:CUBDT]2.0.CO;2
Haila, Y. (2002). A conceptual genealogy of fragmentation research: from island biogeography to landscape ecology. Ecol. Appl. 12, 321–334. doi: 10.2307/3060944
Hallworth, M. T., and Marra, P. P. (2015). Miniaturized GPS tags identify non-breeding territories of a small breeding migratory songbird. Sci. Rep. 5:11069. doi: 10.1038/srep11069
Heise, C. D., and Moore, F. R. (2003). Age-related differences in foraging efficiency, molt, and fat deposition of Gray Catbirds prior to autumn migration. Condor 3, 496–504. doi: 10.1650/7183
Hijmans, R. J (2015). Raster: Geographic Data Analysis and Modeling. R package version 2.4-20. Available online at: http://CRAN.R-project.org/package=raster
Janousek, W. M., Marra, P. P., and Kilpatrick, A. M. (2014). Avian roosting behavior influences vector-host contact for West Nile virus hosts. Parasites Vectors 7:399. doi: 10.1186/1756-3305-7-399
Kennedy, C., Marra, P. P., Fagan, W., and Neel, M. (2010). Matrix mediates avian movements in tropical forested landscapes: inference from experimental translocations. Biol. Conserv. 143, 2136–2145. doi: 10.1016/j.biocon.2010.05.025
Kindlmann, P., and Burel, F. (2008). Connectivity measures: a review. Landsc. Ecol. 23, 879–890. doi: 10.1007/s10980-008-9245-4
Kotliar, N. B., and Wiens, J. A. (1990). Multiple scales of patchiness and patch structure: a hierarchical framework for the study of heterogeneity. Oikos 59, 253–260. doi: 10.2307/3545542
Kupfer, J. A., Malanson, G. P., and Franklin, S. B. (2006). Not seeing the ocean for the islands: the mediating influence of matrix-based processes on forest fragmentation effects. Glob. Ecol. Biogeogr. 15, 8–20. doi: 10.1111/j.1466-822X.2006.00204.x
LaPoint, S., Balkenhol, N., Hale, J., Sadler, J., and van der Ree, R. (2015). Ecological connectivity research in urban areas. Funct. Ecol. 29, 868–878. doi: 10.1111/1365-2435.12489
Leston, L. F., and Rodewald, A. D. (2006). Are urban forests ecological traps for understory birds? An examination using Northern cardinals. Biol. Conserv. 131, 566–574. doi: 10.1016/j.biocon.2006.03.003
Levins, R. (1969). Some demographic and genetic consequences of environmental heterogeneity for biological control. Bull. ESA 15, 237–240. doi: 10.1093/besa/15.3.237
Lindenmayer, D., and Fischer, J. (2006). Habitat Fragmentation and Landscape Change: An Ecological and Conservation Synthesis. Washington, DC: Island Press.
Lukacs, P. M., Burnham, K. P., and Anderson, D. R. (2010). Model selection bias and Freedman's paradox. Ann. Inst. Stat. Math. 62, 117–125. doi: 10.1007/s10463-009-0234-4
Mazerolle, M. J (2015). AICcmodavg: Model Selection and Multimodel Inference based on (Q)AIC(c). R Package Version 2.0-3. Available online at: http://CRAN.R-project.org/package=AICcmodavg
McGarigal, K., Tagil, S., and Cushman, S. A. (2009). Surface metrics: an alternative to patch metrics for the quantification of landscape structure. Landsc. Ecol. 24, 433–450. doi: 10.1007/s10980-009-9327-y
McKinney, M. L. (2002). Urbanization, biodiversity, and conservation. BioScience 52, 883–890. doi: 10.1641/0006-3568(2002)052[0883:UBAC]2.0.CO;2
McRae, B. H., Dickson, B. G., Keitt, T. H., and Shah, V. B. (2008). Using circuit theory to model connectivity in ecology, evolution, and conservation. Conserv. Ecol. 89, 2712–2724. doi: 10.1890/07-1861.1
Mennechez, G., Schtickzelle, N., and Baguette, M. (2003). Metapopulation dynamics of the bog fritillary butterfly: comparison of demographic parameters and dispersal between a continuous and a highly fragmented landscape. Landsc. Ecol. 18, 279–291. doi: 10.1023/A:1024448829417
Minor, E. S., and Urban, D. L. (2008). A graph-theory framework for evaluating landscape connectivity and conservation planning. Conserv. Biol. 22, 297–307. doi: 10.1111/j.1523-1739.2007.00871.x
Moilanen, A., and Nieminen, M. (2002). Simple connectivity measures in spatial ecology. Ecology 83, 1131–1145. doi: 10.1890/0012-9658(2002)083[1131:SCMISE]2.0.CO;2
Nagelkerke, N. J. D. (2004). A note on a general definition of the coefficient of determination. Biometrika 78, 691–692. doi: 10.1093/biomet/78.3.691
Opdam, P., Rijsdijk, G., and Hustings, F. (1985). Bird communities in small woods in an agricultural landscape: effects of area and isolation. Biol. Conserv. 34, 333–352. doi: 10.1016/0006-3207(85)90039-4
Pärn, H., Jensen, H., Ringsby, T. H., and SÆther, B. E. (2009). Sex-specific fitness correlates of dispersal in a house sparrow metapopulation. J. Anim. Ecol. 78, 1216–1225. doi: 10.1111/j.1365-2656.2009.01597.x
Pearson, S. M., Turner, M. G., Gardner, R. H., and O'Neill, R. V. (1996). “An organism based perspective of habitat fragmentation,” in Biodiversity in Managed Landscapes: Theory and Practice, ed R. C. Szaro (Oxford: Oxford University Press), 77–95.
Pebesma, E. J., and Bivand, R. S (2005). Classes and Methods for Spatial Data in R. R News 5. Available online at: http://cran.r-project.org/doc/Rnews/
Pyle, P. (1997). Identification Guide to North American Birds: Columbidae to Ploceidae. Bolinas, CA: Slate Creek Press.
Rappole, J. H., and Ballard, K. (1987). Postbreeding movements of selected species of birds in Athens, Georgia. Wilson Bull. 99, 475–480.
Rappole, J. H., and Tipton, A. R. (1991). New harness design for attachment of radio transmitters to small passerines. J. Field Ornithol. 62, 335–337.
Ricketts, T. H. (2001). The matrix matters: effective isolation in fragmented landscapes. Am. Nat. 158, 87–99. doi: 10.1086/320863
Rodewald, P. (ed.). (2015). The Birds of North America. Ithaca, NY: Cornell Laboratory of Ornithology. Available online at: http://bna.birds.cornell.edu.libproxy.lib.unc.edu/BNA/
Royle, J. A., Dawson, D. K., and Bates, S. (2004). Modeling abundance effects in distance sampling. Ecology 85, 1591–1597. doi: 10.1890/03-3127
Rudnicky, T. C., and Hunter, M. L. Jr. (1993). Avian nest predation in clearcuts, forests, and edges in a forest-dominated landscape. J. Wildl. Manage. 57, 358–364. doi: 10.2307/3809434
Ryder, T. B., Reitsma, R., Evans, B. S., and Marra, P. P. (2010). Quantifying avian nest survival along an urbanization gradient using citizen-and scientist-generated data. Ecol. Appl. 20, 419–426. doi: 10.1890/09-0040.1
Schooley, R. L., and Wiens, J. A. (2003). Finding habitat patches and directional connectivity. Oikos 102, 559–570. doi: 10.1034/j.1600-0706.2003.12490.x
Stamps, J. A., Krishnan, V. V., and Reid, M. L. (2005). Search costs and habitat selection by dispersers Ecology 86, 510–518. doi: 10.1890/04-0516
Studds, C. E., Kyser, T. K., and Marra, P. P. (2008). Natal dispersal driven by environmental conditions interacting across the annual cycle of a migratory songbird. Proc. Natl. Acad. Sci. U.S.A. 105, 2929–2933. doi: 10.1073/pnas.0710732105
Taylor, P. D., Fahrig, L., Henein, K., and Merriam, G. (1993). Connectivity is a vital element of landscape structure. Oikos 68, 571–573. doi: 10.2307/3544927
Tischendorf, L., and Fahrig, L. (2000). On the usage and measurement of landscape connectivity. Oikos 90, 7–19. doi: 10.1034/j.1600-0706.2000.900102.x
Tsurim, I., Abramsky, Z., and Kotler, B. P. (2008). Foraging behavior of urban birds: Are human commensals less sensitive to predation risk than their nonurban counterparts. Condor 110, 772–776. doi: 10.1525/cond.2008.8572
Urban, D., and Keitt, T. (2001). Landscape connectivity: a graph-theoretic perspective. Ecology 82, 1205–1218. doi: 10.1890/0012-9658(2001)082[1205:LCAGTP]2.0.CO;2
Vanderhoff, N., Sallabanks, R., and James, F. C. (2014). American Robin (Turdus migratorius). The birds of North America 462.
Van Dyck, H., and Baguette, M. (2005). Dispersal behaviour in fragmented landscapes: routine or special movements? Basic Appl. Ecol. 6, 535–545. doi: 10.1016/j.baae.2005.03.005
Van Etten, J. (2015). gdistance: Distances and Routes on Geographical Grids. R package version1.1-7. Available online at: http://CRAN.R-project.org/package=gdistance
Wiens, J. A. (1976). Population responses to patchy environments. Annu. Rev. Ecol. Syst. 7, 81–120. doi: 10.1146/annurev.es.07.110176.000501
Wiens, J. A. (1997). “The emerging role of patchiness in conservation biology,” in The Ecological Basis of Conservation, eds S. T. A. Pickett, R. S. Ostfeld, M. Shachak, and G. E. Likens (New York, NY: Springer), 93–107. doi: 10.1007/978-1-4615-6003-6_10
Wilcove, D. S., McLellan, C. H., and Dobson, A. P. (1986). Habitat fragmentation in the temperate zone. Conserv. Biol. 6, 237–256.
Wolff, J. O., Schauber, E. M., and Edge, W. D. (1997). Effects of habitat loss and fragmentation on the behavior and demography of gray-tailed voles. Conserv. Biol. 11, 945–956. doi: 10.1046/j.1523-1739.1997.96136.x
Xian, G., Homer, C., Dewitz, J., Fry, J., Hossain, N., and Wickham, J. (2011). Change of impervious surface area between 2001 and 2006 in the conterminous United States. Photogramm. Eng. Remote Sens. 77, 758–762.
Zeller, K. A., McGarigal, K., Beier, P., Cushman, S. A., Vickers, T. W., and Boyce, T. M. (2014). Sensitivity of landscape resistance estimates based on point selection functions to scale and behavioral state: pumas as a case study. Landsc. Ecol. 29, 541–557. doi: 10.1007/s10980-014-9991-4
Zeller, K. A., McGarigal, K., and Whiteley, A. R. (2012). Estimating landscape resistance to movement: a review. Landsc. Ecol. 27, 777–797. doi: 10.1007/s10980-012-9737-0
Keywords: landscape ecology, urban ecology, avian dispersal, movement ecology, avian ecology
Citation: Evans BS, Kilpatrick AM, Hurlbert AH and Marra PP (2017) Dispersal in the Urban Matrix: Assessing the Influence of Landscape Permeability on the Settlement Patterns of Breeding Songbirds. Front. Ecol. Evol. 5:63. doi: 10.3389/fevo.2017.00063
Received: 20 February 2017; Accepted: 31 May 2017;
Published: 15 June 2017.
Edited by:
Amanda D. Rodewald, Cornell University, United StatesReviewed by:
Stephen N. Matthews, The Ohio State University Columbus, United StatesIan Ausprey, University of Florida, United States
Copyright © 2017 Evans, Kilpatrick, Hurlbert and Marra. This is an open-access article distributed under the terms of the Creative Commons Attribution License (CC BY). The use, distribution or reproduction in other forums is permitted, provided the original author(s) or licensor are credited and that the original publication in this journal is cited, in accordance with accepted academic practice. No use, distribution or reproduction is permitted which does not comply with these terms.
*Correspondence: Brian S. Evans, evansbr@si.edu