- 1Department of Environmental and Life Sciences, Trent University, Peterborough, ON, Canada
- 2Graduate Department of Forestry, University of Toronto, Toronto, ON, Canada
- 3Ontario Ministry of Natural Resources and Forestry, Peterborough, ON, Canada
Coyotes (Canis latrans) have established populations in most major urban centers across North America. While the risk of attacks on humans or their pets is low, the presence of carnivores in areas with high human use has resulted in increased public concern. Having a clearer understanding of which animals are more likely to interact with humans and when interactions are more likely to occur will help mitigate human-carnivore conflicts. Despite clear broad-scale patterns of human avoidance, human-coyote interactions occur most frequently in residential areas. Our purpose was to determine if use of residential areas varied consistently across individuals or time. We used locations from GPS collars deployed on 14 coyotes in the Greater Toronto Area, Ontario, Canada from 2012 to 2017 to fit a step selection function. Average (±SE) home range size estimates were 17.3 ± 4.6 km2 for resident coyotes and 102.8 ± 32.9 km2 for non-residents. We found that coyotes used natural areas more (β = 0.07, SE = 0.02, p < 0.0001), and roads (β = −0.50, SE = 0.13, p < 0.0001) and residential areas (β = −0.79, SE = 0.21, p = 0.0001) less during the day than at night. We also found that coyotes were more likely to use residential areas in the breeding season from January to April (β = 0.69, SE = 0.20, p = 0.0007) and the pup rearing season from May to August (β = 0.54, SE = 0.13, p < 0.0001) than in the dispersal season from September to December. Lastly, we found that resident coyotes were less likely to use residential areas than non-resident coyotes (β = −1.13, SE = 0.26, p < 0.0001). As far as we are aware, our study is the first to identify the seasons when coyotes are more likely to use residential areas. The seasonal patterns in habitat use that we observed reflect patterns that have been previously reported for human-coyote conflicts. Our results demonstrate that reducing the availability of anthropogenic food sources in residential areas, particularly in the winter and spring, should be a priority for managers aiming to reduce human-coyote conflict in urban areas.
Introduction
Human-wildlife conflict is often defined as wildlife induced damage to person or property (Dickman, 2010). The protein-rich diet of mammalian carnivores can put them in direct competition for resources with humans, resulting in relatively higher rates of conflict with humans than observed for other mammal species (Treves and Karanth, 2003). Extensive conversion of forests to agricultural land and urban development following European settlement of North America resulted in range contractions for many mammalian carnivores (Woodroffe, 2000; Laliberte and Ripple, 2004). Yet some species have succeeded at adapting to, and even thriving in, human modified landscapes. Habituation to humans, particularly through intentional or unintentional feeding, is widely considered to be one of the main driving forces behind human-carnivore conflict (Albert and Bowyer, 1991; Newsome et al., 2015b). Further, human-wildlife conflicts are more likely to occur when carnivores are in poor health (Towns et al., 2009; Murray et al., 2015a), relying on more anthropogenic food sources (White and Gehrt, 2009; Lukasik and Alexander, 2011; Murray et al., 2015b), or within areas with poor habitat quality (Magle et al., 2014; Poessel et al., 2017a).
Coyotes (Canis latrans) have proven to be particularly successful at taking advantage of anthropogenic changes to landcover, with a documented range expansion of 40% following European settlement (Laliberte and Ripple, 2004) and established populations in most major urban centers in North America (Bateman and Fleming, 2012; Poessel et al., 2017a). The presence of coyotes in cities provides many ecological benefits for the urban ecosystem; including increased bird diversity through mesopredator release (Crooks and Soule, 1999) and controlling populations of rodents and other pest species (Henke and Bryant, 1999; Jones et al., 2016). However, the presence of carnivores in areas of high human density can also lead to human-wildlife conflict. The number of newspaper articles reporting coyote attacks on humans or their pets has increased dramatically in recent decades, resulting in increased public concern over the presence of coyotes in areas with high human use (White and Gehrt, 2009). Human-coyote conflicts in urban areas are most likely to occur in the late winter and early spring, which coincide with the time of year when coyotes are breeding and raising pups (Lukasik and Alexander, 2011; Poessel et al., 2013). Although the actual risk of coyote attacks on humans or their pets is very low (Lukasik and Alexander, 2011; Poessel et al., 2013), when attacks do occur it can be a traumatizing event for those involved (Baker and Timm, 1998; White and Gehrt, 2009). Management strategies that attempt to reduce coyote populations through harvest are ineffective due to high reproductive rates and immigration, so coyote populations in urban areas are presumably here to stay (Knowlton et al., 1999; Conner and Morris, 2015).
Within the urban ecosystem, coyotes predominately reside in areas with greater amounts of natural cover and avoid more developed areas that are associated with increased human use (Riley et al., 2003; Atwood et al., 2004; Gehrt et al., 2009). In more densely populated urban cores coyote home ranges typically include a greater proportion of developed areas (Riley et al., 2003; Gese et al., 2012). However, coyotes that have a greater proportion of developed areas within their home range tend to have larger home ranges (Gehrt et al., 2009). Larger home ranges are an indication of lower habitat quality, because the individual needs more area to meet its daily requirements (MacDonald, 1983). High use of linear features (Grinder and Krausman, 2001; Poessel et al., 2016) and repeat use of specific corridor features (Way and Eatough, 2006) suggests that developed areas are predominately used to travel between natural cover in the urban matrix. When coyotes do go into areas associated with high human use, they tend to do so at night when they are less likely to interact with humans (Gese et al., 2012). Individual-level patterns in habitat selection have been documented within urban coyote populations. Dispersing coyotes, who tend to be subadults searching for a mate, are often relegated to suboptimal habitats (Mitchell et al., 2015). Furthermore, a few studies have found that female coyotes in urban ecosystems have stronger habitat preferences than males (Grinder and Krausman, 2001; Poessel et al., 2016). Despite these strong population level patterns of avoidance, human-coyote interactions in urban centers occur most frequently in residential areas and very rarely in natural habitat fragments (Krester et al., 2008; White and Gehrt, 2009; Lukasik and Alexander, 2011; Poessel et al., 2013).
We had three objectives for this study. Our first objective was to assess whether the coyotes in our study area had patterns of habitat selection indicative of human avoidance, as has been documented in other urban coyote populations. Our second objective was to describe home range size of coyotes in our study area for comparison with other studies of urban space use. Our third objective was to determine if individual and temporal variation in habitat selection by urban coyotes reflects previously reported patterns in human-coyote conflicts. Our focus was on investigating differences in use of residential areas, which are zoning areas that predominantly consist of housing, because this is the land use type where humans and coyotes are most likely to interact and where most conflicts occur (White and Gehrt, 2009). We predicted that (1) coyotes would use natural areas more during the day and developed areas more at night, (2) coyotes would use residential areas more frequently during breeding and pup rearing seasons, (3) resident coyotes would use residential areas less than non-residents, (4) sub-adult coyotes would use residential areas more than adult coyotes, and (5) female coyotes would use residential areas less than male coyotes.
Materials and Methods
Study Site
We conducted our study in the Greater Toronto Area (GTA), Ontario, Canada. Although the GTA consists of a range of landscapes from urban to rural, we focused our study primarily in the 10 municipalities that make up the urban and suburban core (Figure 1). The urban and suburban core of the GTA had a population greater than 5 million people with a population density of ca. 2,169 people per km2 (Statistics Canada, 2011). The study area was located in the Lake Erie Lowland ecoregion, with mean summer temperatures of 18°C, mean winter temperatures of −2.5°C, and annual precipitation ranging from 750 to 900 mm (Ecological Stratification Working Group, 1995). The study site was dominated by developed land covers but scattered throughout were natural and non-natural green spaces. Natural green spaces were predominantly found within a system of ravines that were protected from development across the GTA to mitigate flood damage. Vegetation cover included deciduous and mixed-wood stands; with sugar maple (Acer sacharum), oak (Quercus rubra and Quercus alba), and green ash (Fraxinus pennsylvanica) being some of the common native tree species (Ecological Stratification Working Group, 1995).
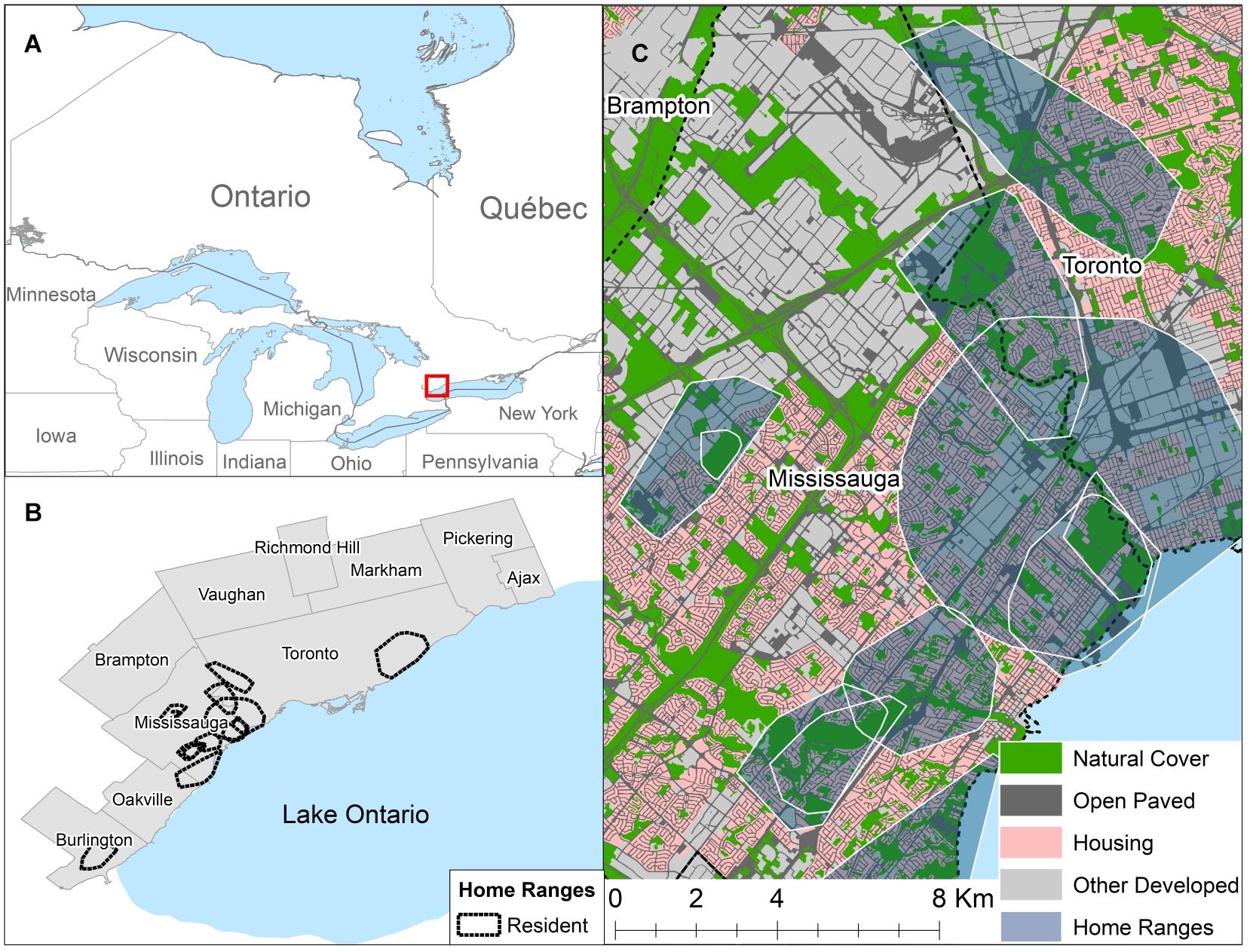
Figure 1. (A) Map of Ontario with a red square showing the location of the study area. (B) Showing the boundaries of 10 municipalities that make up the contiguous urban and suburban core within the Greater Toronto Area, Canada and the location of resident coyote home ranges calculated using 95% minimum convex polygons. (C) A representative example of the distribution of different habitat classes within resident coyote home ranges in the City of Mississauga. Maps were created using ArcGIS 10.3.1. Contains information licensed under the Open Government Licence — Canada.
Habitat Classification
We classified the study area into four habitat types: Natural Cover, Open Paved, Housing, and Other Developed based on information in the Southern Ontario Land and Resource Inventory v. 1.2 (Southern Ontario Land Resource Information System (SOLRIS), 2008) and land use categories from municipal zoning by-law and official plan maps (Peel Region, 2003; Toronto, 2013; Burlington, 2014; Mississauga, 2014; Oakville, 2014). We used ArcGIS 10.3.1 for the creation of habitat maps (ESRI, 2011). We amalgamated municipal zones into either Housing (residential zones) or Other Developed (including commercial, office space, industrial, institutional, and mixed use zones). We extracted areas from the SOLRIS “Built Up Impervious” Class that overlayed Housing and Other Developed zones using the “Clip” function. We combined any remaining area from the “Built Up Impervious class” that did not have a zoning designation with the “Transportation” class to create the Open Paved habitat, which resulted in a land cover type that consists of roads, railways, abandoned lots, and parking lots. For the purpose of this study, we defined natural land use as areas not dominated by buildings, pavement, or other anthropogenic impervious surfaces and therefore include non-natural features (e.g., agriculture, cemeteries, golf courses, etc.). The Natural Cover habitat class was based solely on the SOLRIS classification, and included all wetlands, forests, fields, and urban parks (Figure 1).
Trapping and Radiocollaring
We conducted trapping opportunistically in natural areas using padded footholds (Victor no. 3 Softcatch) from 2012 to 2014. We continuously monitored traps on public lands overnight and covered them with wood boards during the day to avoid incidental interactions with the general public. We anesthetized captured animals using an intramuscular injection of xylazine hydrochloride (2 mg/kg) and Telazol (4 m mg/kg), and we reversed animals after capture using atipamezole (0.2 mg/kg). We fitted captured animals with GPS (Lotek Wildcell SG, Newmarket, Ontario) collars, determined their sex, took morphometric body measurements, and collected hair, whisker, and blood samples. From 2016 to 2017 we also deployed collars on coyotes that were treated for mange and subsequently released by the Toronto Wildlife Centre. We focused our fieldwork in Mississauga, with a few captures in Toronto (Figure 1). Collars were programed to record a location fix either every 2.5 or 3 h. More frequent fixes were used with the collar schedules for some animals but they are not included in this study. We aged coyotes based on tooth wear patterns and classified them as either sub-adult (0–2 years old) or adult (>2 years old) (Gipson et al., 2000). All capture and handling methods were approved by the Ontario Ministry of Natural Resources Animal Care Committee (Protocol nos. 75-12, 75-13, 75-14).
Home Range Analysis
To facilitate comparison with previous urban coyote research, we calculated home range size estimates for both residents and non-residents using 95% minimum convex polygons in the “adehabitatHR” package for R 3.2.4 (Calenge, 2006). Like previous work on urban coyotes, we found that kernel density estimates provided home ranges that were highly fragmented and did not accurately represent coyote territories (Gehrt et al., 2009; Mitchell et al., 2015; Poessel et al., 2016). We classified individuals as either residents or non-residents. We identified residents based on continued use of the same areas for ≥3 months, with home ranges either contained within a single habitat fragment or showing return visits to multiple habitat fragments. Resident coyotes are typically a breeding pair and associated pack members that defend a territory from neighboring residents (Gese et al., 1989). Non-resident coyotes are generally not associated with a pack or territory and roam the landscape widely (Gese et al., 1989). For resident coyotes we also calculated separate home ranges using diurnal and nocturnal locations, and for each biological season. We used Mann-Whitney U-tests to test for differences between home range sizes of resident males vs. females and adults vs. sub-adults. We used a Wilcoxon-signed rank test to test for differences between diurnal and nocturnal home ranges for resident coyotes. Lastly, we used an unbalanced repeated measures ANOVA with individual ID as a random block variable in the R package “car” (Fox and Weisberg, 2019) to determine if there were differences between biological seasons for residents. Non-residents were excluded from these statistical tests because they do not use a well-defined area and home range size estimates are predominantly influenced by duration of collar deployment (Wheeldon, 2020).
Step Selection Functions
Step selection functions are a type of resource selection function that compare habitats “used” by animals to the habitats that were “available” to them. Unlike other resource selection functions that compare observed locations (used) to locations randomly sampled across the home range or wider landscape (available), step selection functions create a paired case-control design by defining available habitat for each used location based on observed movement patterns (Fortin et al., 2005). To create a paired case-control design, step selection functions use “steps” as the base sampling unit. A step is the straight-line between two consecutive locations in a set of animal locations sampled at regular time intervals. Therefore, paired used and available steps compare where an animal at a given location went after a fixed time interval to where it could have gone based on observed step lengths and turning angles (Fortin et al., 2005).
We prepared GPS locations obtained from radiocollars for analysis in a step selection function using the R package “amt” following the recommended workflow outlined by Signer et al. (2019). We resampled locations to either a 2.5 or 3 h fix interval depending on the collar schedule. We classified locations as diurnal or nocturnal based on sunset and sunrise times for Toronto at the corresponding time of year. We also classified locations according to biological season as defined by Gehrt et al. (2009): breeding (January 1–April 30), pup rearing (May 1–August 31), and dispersal (September 1–December 31). We separated locations into “bursts,” which consisted of series of consecutive locations uninterrupted by missed fixes. We removed bursts with fewer than 3 consecutive locations, which is the minimum number required to calculate a turn angle. We converted bursts into a series of steps by creating a straight line between consecutive locations, calculating distance along the line, and the angle of the change in direction from the previous heading. For each individual, we fit observed step lengths to a gamma distribution and observed turn angles were fit to a Von Mises distribution (Forester et al., 2009). For each used step, we drew 25 available steps by randomly selecting step length and turn angle from these distributions. We recorded the habitat at the end of all used and available steps. We created dummy variables for Natural Cover, Open Paved, and Housing habitats, which left Other Developed as the reference habitat. We included the used step in the sample of available steps to ensure that the selected habitat was always present in the pool of available steps (Avgar et al., 2016). We removed available steps that ended in Lake Ontario from the analysis.
Because Step Selection Functions using categorical habitat classifications only provide inferences on habitat selection relative to the reference habitat, we calculated summary statistics of absolute differences between habitat use and availability to provide additional context. We calculated the proportion of used steps that ended in each habitat class for each animal. We also determined the proportion of available steps that ended in each habitat class for each observed step, and then calculated the mean availability across all observed steps for each animal.
Analysis of step selection functions followed the approach outlined in Muff et al. (2020). We used the R package “glmmTMB” (Brooks et al., 2017) to run a Poisson generalized linear mixed model with step ID as random intercept, which is a likelihood equivalent of a conditional logistic regression (Muff et al., 2020). We fixed the variance for the step ID random intercept at 1,000 to allow the estimates of this parameter to vary without shrinkage toward the mean (Muff et al., 2020). All models included a random intercept term for animal ID and a random slope term that varied according to animal ID for every habitat variable and interaction term included in the model.
We conducted model selection using a combination of Akaike’s Information Criteria (AIC) and null hypotheses testing, similar to the approach described in Wood and Augustin (2002). If adding a variable decreased the model AIC by ≥ 2 and the added fixed effect coefficient was significantly different from zero, with a p ≤ 0.05, then there was support for the more complicated model. We used a combined approach because both methods are imperfect. The issues with relying solely on p-values for hypothesis testing are well documented, and the consensus among statisticians is that they need to be considered in conjunction with other statistical values (Wasserstein and Lazar, 2016). Whereas AIC is generally the preferred approach for model selection in ecology, it can overfit models by including uninformative parameters, which is a particularly common issue in studies with large numbers of data points (Arnold, 2010). We fit a habitat-only step selection function that served as the base habitat model in hypothesis testing, where probability of choosing the observed step was a function the habitat type at the destination. To ensure that the base habitat model was an improvement over a null model we compared it to a model that only included random intercepts for step ID and animal ID. We added interaction terms to the base habitat model to test hypotheses about individual-level and temporal variation in habitat use. We created candidate models that included 2-way interaction terms representing hypotheses on the effect of social status (resident vs. non-resident), sex (male vs. female), age (adult vs. subadult), time of day (day vs. night), and biological season (breeding vs. pup rearing vs. dispersal) on the use of different habitats (Natural Cover, Open Paved, and Housing). Lastly, we fit a full model with the base habitat model and all interaction terms that were supported during model selection. All data processing and analyses was done in R 4.0.2 (R Core Team, 2020).
Results
Trapping and Radiocollaring
In total, 18 coyotes were captured and fitted with GPS collars. Locations from collared individuals were within five municipalities: Toronto, Mississauga, Brampton, Oakville and Burlington. We truncated the data from two coyotes when they left the study area and removed four individuals from analysis because their collars were active for <30 days. Four animals were released by the Toronto Wildlife Centre between 2016 and 2017, although only one of these individuals met the criteria to be included in this study. Collars remained on the coyotes for 6 months to a year (mean: 278.4 days ± 82.2 SD) depending on mortality and battery life. The average number of used steps per individual was 1678 ± 555 SD (range: 904–2,517). The individuals used in this study consisted of seven males and seven females with ten adults and four subadults. Several coyotes changed their social status (resident vs. non-resident) during the study. One animal shifted their home range twice while collared. Because each home range was occupied for >3 months and each displayed continued repeat use of a few natural fragments, we treated them as three separate resident states. The number of unique animal-social states consisted of eleven residents and six non-residents. Five of the collared coyotes died during the study, four from vehicle collisions and one from natural causes (with visible signs of mange). One coyote’s fate was unknown (the collar was found, but not the individual).
Home Range Analysis
Average (± SE) 95% minimum convex polygon home range size estimates for residents was 17.3 ± 4.6 km2 (range: 0.9–56.9 km2) and 102.8 ± 32.9 km2 (range: 12.2–198.0 km2) for non-residents. Home range sizes of resident males (15.0 ± 10.4 km2) were not significantly different from home range sizes of resident females (18.3 ± 5.2 km2; w = 11, p = 0.330). Home range sizes of resident adults (20.6 ± 7.1 km2) were not significantly different from home range sizes of resident subadults (12.0 ± 3.1 km2; w = 23, p = 0.724). Resident coyotes used a significantly smaller portion of their home range during the day (13.8 ± 4.0 km2) than at night (16.9 ± 4.6 km2; v = 7, p = 0.005). There was no significant difference between home range sizes of resident coyotes between seasons (χ2 = 0.79, p = 0.673): average breeding, pup rearing, and dispersal home range sizes were 13.4 ± 4.4, 18.5 ± 7.2, and 13.6 ± 4.5 km2, respectively.
Step Selection Functions
On average (±SE), individual coyotes selected Natural Cover 74.1 ± 3.8% of the time, which is more than it occurred in available steps (50.0 ± 2.9%). They selected Open Paved (15.7 ± 3.3%) less than it was found in available steps (20.8 ± 2.0%) and Housing (5.5 ± 1.1%) less than in available steps (19.6 ± 1.5%). Lastly, coyotes selected Other Developed (4.7 ± 1.5%) less than in available steps (19.6 ± 1.5%; Figure 2).
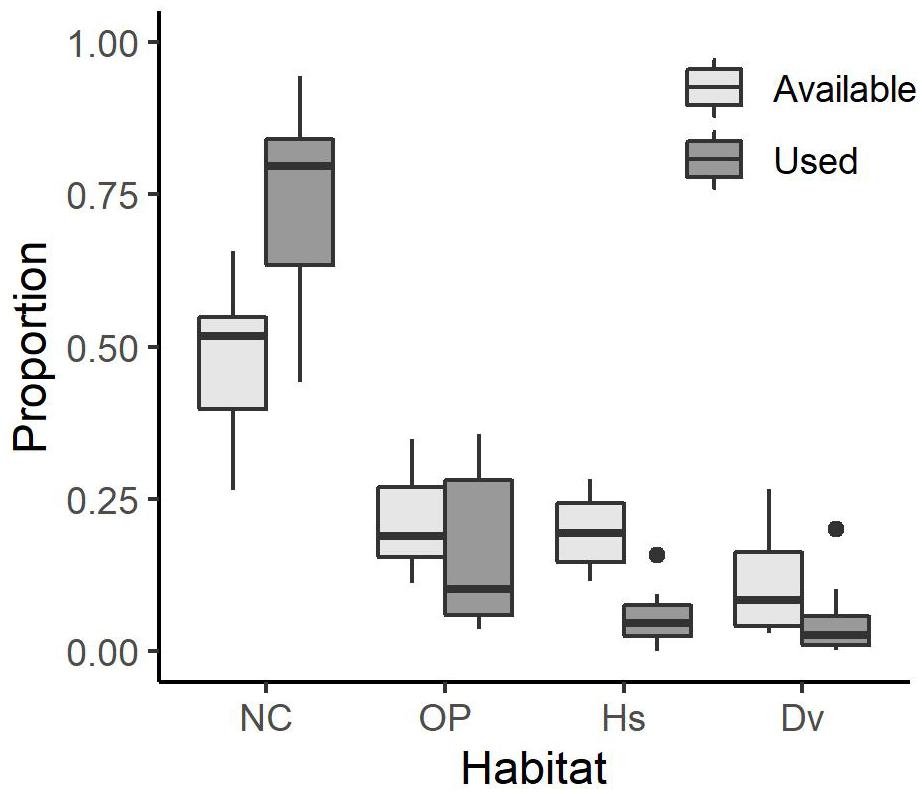
Figure 2. Boxplot showing the proportion of used habitat and mean proportion of available habitat for 14 coyotes with GPS collars in the Greater Toronto Area from 2012 to 2017. The center line represents the median. The limits of the box represent the interquartile range (IQR) and the whiskers represent 1.5 × IQR. Solid circles denote outliers. Habitat types are Natural Cover (NC), Open Paved (OP), Housing (Hs), and Other Developed (Dv).
Model selection (Supplementary Appendix 1) analyzing interactions between individual characteristics or temporal patterns and habitat selection revealed broad selection for Natural Cover (β = 1.50, SE = 0.14, p < 0.001), and Open Paved (β = 0.82, SE = 0.15, p < 0.001) habitats relative to Other Developed habitat types (Table 1). Natural Cover was selected more during the day (β = 0.07, SE = 0.02, p < 0.001) than at night, whereas Open Paved (β = −0.49, SE = 0.13, p < 0.001) and Housing (β = −0.79, SE = 0.21, p = 0.001) habitats were more likely to be selected at night than during the day. There was no statistical support for differences in habitat selection due to age or sex (p < 0.05). There was support for increased use of Housing areas during breeding (β = 0.64, SE = 0.20, p = 0.002) and pup rearing (β = 0.47, SE = 0.13, p < 0.001) seasons relative to the dispersal season. Resident coyotes were less likely to use Housing areas (β = −1.21, SE = 0.28, p < 0.001) than non-resident coyotes (Figure 3).

Table 1. Model output for a step selection function based on 14 coyotes with GPS collars in the Greater Toronto Area from 2012 to 2017.
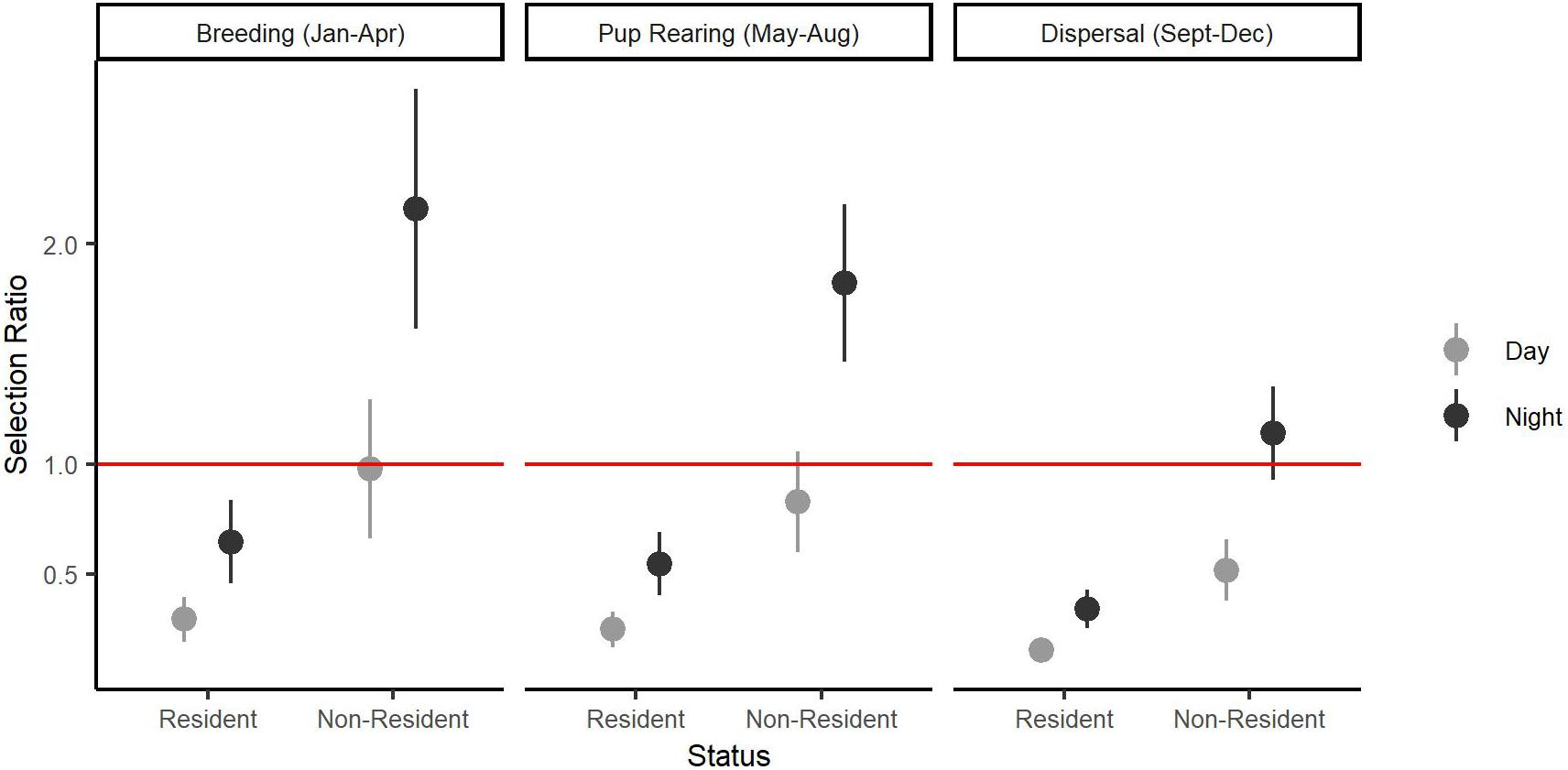
Figure 3. Individual and temporal variation in selection for Housing based on a Step Selection Function conducted on 14 coyotes with GPS collars in the Greater Toronto Area, Canada from 2012 to 2017. Selection Ratio on the y-axis shows how likely an animal will select Housing relative to Other Developed land use classes. Error bars represent standard error around the estimate of the fixed effects. The red line indicates when Housing areas are equally as likely to be selected as Other Developed areas.
Discussion
Overall, habitat selection and home range size showed strong evidence of human avoidance by urban coyotes in the Greater Toronto Area (GTA). Similar to other studies of urban coyote space use, coyotes strongly selected for natural land cover and avoided developed land cover (Riley et al., 2003; Atwood et al., 2004; Gehrt et al., 2009; Gese et al., 2012; Mitchell et al., 2015). When coyotes leave natural areas, there appears to be stronger use of Open Paved habitats over developed land cover types. Roads, railways, abandoned lots, and other open paved areas play an integral role in coyote movements through developed areas. Linear features in the urban landscapes are likely used as travel corridors through areas with higher risks of interactions with humans (Grinder and Krausman, 2001; Atwood et al., 2004). That we observed resident coyotes having smaller home ranges during the day than at night suggests that coyotes in our study area hide in core areas when humans are most active and only use certain portions of their territory when the risk of interaction is lower. This temporal pattern of human avoidance is supported by the observed decrease in use of natural areas and increase in use of developed areas at night. Diurnal shifts in activity and habitat selection by coyotes as a mechanism for avoiding interactions with humans are well documented across North America (Riley et al., 2003; Atwood et al., 2004; Gese et al., 2012; Mitchell et al., 2015; Poessel et al., 2016; Murray and St Clair, 2017).
Average home range size for resident coyotes in our study (17.3 ± 4.6 km2) was larger than reported in previous studies of urban coyotes: Tuscon, AZ (12.6 ± 3.5 km2; Grinder and Krausman, 2001), Chicago, IL (5.0 ± 0.3 km2; Gehrt et al., 2009), Cuyahoga Valley, OH (6.2 ± 2.2 km2; Franckowick et al., 2019), and Denver, CO (11.6 ± 11 km2; Poessel et al., 2016). This is likely because our study was focused entirely within the urban and suburban core of the GTA and did not include surrounding exurban or rural areas. Coyotes within the urban core tend to occupy home ranges with more developed area and therefore have larger home ranges (Riley et al., 2003; Gehrt et al., 2009). Other factors that could contribute to the larger home range sizes observed in our study are latitude (coyote home range sizes generally increase with latitude) and hybridization with wolves/domestic dogs (Ellington and Murray, 2015). As coyotes expanded into eastern North America they hybridized with wolves (Kays et al., 2010) and domestic dogs (Wheeldon et al., 2013). These hybridization events resulted in populations of coyotes that were larger than their western counterparts (Kays et al., 2010). It has been hypothesized that hybridization has also resulted in functional changes to coyote populations and potentially improving the ability of eastern coyotes to adapt to human modified landscapes (Monzõn et al., 2014). However, most research on urban coyotes has been conducted on western coyotes, so it is not yet clear whether hybridization is influencing spatial ecology of urban coyotes. Our study supports previous research demonstrating that eastern coyotes have similar patterns of human-avoidance to western coyotes (Way, 2011; Mitchell et al., 2015; Franckowick et al., 2019).
We did not find support for sex-based differences in home range sizes or habitat selection in our study system. Some other studies of urban coyotes have also found no sex-based differences in habitat selection (Gehrt et al., 2009; Murray and St Clair, 2017; Franckowick et al., 2019). However, a few studies found that female coyotes in urban settings can show stronger avoidance of developed habitats (Grinder and Krausman, 2001; Riley et al., 2003; Poessel et al., 2016). Coyotes are highly adaptable and show a wide range of responses to different anthropogenic and natural conditions. Having a better understanding of the features of urban areas that show differential use by the sexes could improve our understanding of how coyotes are able to adapt to such a wide range of conditions. We also did not find support for age-based differences in home range size or habitat selection, which is a consistent finding across the literature. Young canids tend to stay near their parents and therefore have similar home ranges and habitat selection prior to dispersal (Riley et al., 2003; Gehrt et al., 2009; Murray and St Clair, 2017).
Non-resident coyotes are predominantly young individuals who have dispersed from their natal territory (Gese et al., 1989), although older coyotes can get displaced from their role in a pack and become non-residents as well (Gese et al., 1988). It is a high-risk time in a coyote’s life cycle that individuals need to survive to reach breeding status. Mortality rates are higher for non-residents than resident coyotes (Gese et al., 1989; Harrison et al., 1991), and non-resident coyotes tend to be relegated to sub-optimal habitat (Kamler and Gipson, 2000; Mitchell et al., 2015). Non-residents coyotes in urban environments also include a larger proportion of anthropogenic food in their diets (Newsome et al., 2015a). Experimental studies have shown that food-stressed organisms are more likely to use higher risk habitats to forage (Pettersson and Bronmark, 1993). Increased use of Housing by non-resident coyotes presumably reflects a combination of avoiding areas of high use by territorial residents and increased willingness to look for food sources in areas with a higher risk of interactions with humans.
As far as we are aware, our study is the first to document seasonal changes in use of residential areas by urban coyotes. The seasonal patterns of increased use of residential areas that we documented match increases in the number of human-coyote conflicts that have been reported in the breeding season in Denver, CO (Poessel et al., 2013) and in the pup-rearing season in Calgary, AB (Lukasik and Alexander, 2011). Given the ability of individual coyotes to adapt to a wide range of situations, it is likely that the main drivers of human-coyote conflicts vary from city to city and are dependent on some combination of environmental characteristics and human actions. It has been hypothesized that two of the main drivers of seasonal changes in human-coyote conflicts are temporal patterns in aggression toward competitors and food availability (Lukasik and Alexander, 2011; Poessel et al., 2013).
The most common form of human-coyote conflict in urban areas is attacks on pets. Lukasik and Alexander (2011) found that coyote attacks on pets were more frequent in neighborhoods where coyotes had a greater proportion of anthropogenic food in their diets. However, Poessel et al. (2017b) found that seasonal increases in the frequency of coyote attacks on pets did not result in a seasonal increase in the presence of pets in a coyote’s diet. This suggests that coyotes attack pets because they are either perceived as competitors for food resources or as a threat to their young, not because the pets themselves are perceived as a prey item. Competition within coyote packs is greatest during the breeding season (Gese et al., 1996). This intrapack competition could push subordinate individuals into riskier habitats, such as residential areas, in search of food and therefore make them more likely to interact with pets. On the other hand, coyote aggression against intruding animals as a form of territorial defense is greatest during the breeding and pup rearing seasons, with peaks in the rate of aggressive encounters in March and June (Gese, 2001).
Alternatively, strong seasonal patterns in food availability can result in seasonal dietary shifts toward more anthropogenic food resources (Morey et al., 2007; Larson et al., 2020). Food availability and dietary diversity are lowest for urban coyotes in the winter (Quinn, 1997; Morey et al., 2007). This could force coyotes to look to residential areas for alternative sources of food when there is not a healthy and diverse available prey population. Human-coyote conflicts are more likely in areas that have fewer available natural sources of food (Magle et al., 2014) and when individual coyotes are subsisting on protein-poor diets (Murray et al., 2015b).
Our study demonstrates that there are individual and temporal patterns in use of residential areas, however, these areas are still strongly avoided by coyotes at the population level. Increased use of residential areas does not necessarily result in increased use of anthropogenic foods. Coyotes use residential areas for reasons other than foraging, such as travel (Way and Eatough, 2006) and rest (Murray and St Clair, 2017). There is also strong evidence for individual specialization in diet within urban coyote populations. Generally, coyotes that have more developed land in their home range tend to eat more anthropogenic food, however, some coyotes have territories consisting predominantly of developed habitats that do not significantly rely on anthropogenic food to meet their dietary requirements (Newsome et al., 2015a). Previous research has shown that human-wildlife conflicts predominantly stem from a small number of individuals that have learned to use anthropogenic food sources instead of natural prey items (Sacks et al., 1999; Clark et al., 2002). We encourage mitigation strategies for human-coyote conflicts that focus on preventative measures that stop specific individuals from becoming conflict prone. Preventative measures, such as minimizing available anthropogenic food sources, are more effective at reducing human-wildlife conflict than reactionary measures, such as relocation (Hopkins et al., 2012). Considering residential areas are predominantly private property, public education campaigns focused on how to limit the availability of food sources in residential yards may be vital for reducing human-coyote conflicts. Relatively high use of residential areas by non-resident coyotes in the winter and spring may represent a critical juncture for the creation of problem animals. The combination of low food resources in winter, with higher aggression from conspecifics during the breeding and pup-rearing seasons could increase the likelihood that dispersing individuals turn to anthropogenic food in residential areas despite increased risk of interactions with humans.
Data Availability Statement
The raw data supporting the conclusions of this article will be made available by the authors, without undue reservation.
Ethics Statement
The animal study was reviewed and approved by the Ontario Ministry of Natural Resources Animal Care Committee.
Author Contributions
CT, BP, and JM contributed to the conception and design of the study. BP organized and conducted data collection. CT and JM contributed to data cleaning and preparation. CT did the statistical analysis and wrote the first draft of the manuscript. All authors contributed to editing of the final manuscript.
Funding
This study was funded by the Wildlife Research and Monitoring Section (WRMS) of the Ontario Ministry of Natural Resources and Forestry (OMNRF).
Conflict of Interest
The authors declare that the research was conducted in the absence of any commercial or financial relationships that could be construed as a potential conflict of interest.
Acknowledgments
We thank B. Hennigar, A. McLaren, J. Pisapio, A. Silver, S. Taylor, J. Rodgers, L. Vander Vennen and all other field staff who conducted the trapping and collaring of coyotes for this study. We also thank the staff from the municipalities of Mississauga (particularly L. Dent) and Toronto who helped facilitate finding areas away from regular human use to trap in, and the staff from the Toronto Wildlife Centre who helped with collar deployment from 2016 to 2017. We also thank J. Northup and J. Benson for guidance on statistical methodologies.
Supplementary Material
The Supplementary Material for this article can be found online at: https://www.frontiersin.org/articles/10.3389/fevo.2021.687504/full#supplementary-material
References
Albert, D. M., and Bowyer, R. T. (1991). Factors relating to grizzly bear-human interactions in Denali National Park. Wildl. Soc. Bull. 19, 339–349.
Arnold, T. W. (2010). Uninformative parameters and model selection using Akaike’s Information Criterion. J. Wildl. Manag. 74, 1175–1178. doi: 10.2193/2009-367
Atwood, T. C., Weeks, H. P., and Gehring, T. M. (2004). Spatial ecology of coyotes along a suburban-to-rural gradient. J. Wildl. Manag. 68, 1000–1009.
Avgar, T., Potts, J. R., Lewis, M. A., and Boyce, M. S. (2016). Integrated step selection analysis: bridging the gap between resource selection and animal movement. Methods Ecol. Evol. 7, 619–630. doi: 10.1111/2041-210X.12528
Baker, R. O., and Timm, R. M. (1998). “Management of conflicts between urban coyotes and humans in Southern California,” in Proceedings of the 18th Vertebrate Pest Conference. Costa Mesa, California, March 2-5, 1998, (Davis, CA: University of California), 299–312.
Bateman, P. W., and Fleming, P. A. (2012). Big city life: carnivores in urban environments. J. Zool. 287, 1–23. doi: 10.1111/j.1469-7998.2011.00887.x
Brooks, M. E., Kristensen, K., van Benthem, K. J., Magnusson, A., Berg, C. W., Nielsen, A., et al. (2017). glmmTMB balances speed and flexibility among packages for zero-inflated generalized linear mixed modeling. R J. 9, 378–400.
Burlington (2014). Zoning by-Law 20-20. Available online at: https://www.burlington.ca/en/services-for-you/resources/Ongoing_Projects/Open_Data/OpenDataBurlingtonTermsOfUseSeptember192011.pdf (accessed October, 2014).
Calenge, C. (2006). The package adehabitat for the R software: tool for the analysis of space and habitat use by animals. Ecol. Modell. 197, 516–519. doi: 10.1016/j.ecolmodel.2006.03.017
Clark, J. E., van Manen, F. T., and Pelton, M. R. (2002). Correlates of success for on-site releases of nuisance black bears in Great Smoky Mountains National Park. Wildl. Soc. Bull. 30, 104–111.
Conner, L. M., and Morris, G. (2015). Impacts of mesopredator control on conservation of mesopredators and their prey. PLoS One 10:e0137169. doi: 10.1371/journal.pone.0137169
R Core Team (2020). R: A Language and Environment for Statistical Computing. Vienna: R Foundation for Statistical Computing.
Crooks, K. R., and Soule, M. E. (1999). Mesopredator release and avifaunal extinctions in a fragmented system. Nature 400, 563–565.
Dickman, A. J. (2010). Complexities of conflict: the importance of considering social factors for effectively resolving human-wildlife conflict. Anim. Conserv. 13, 458–466. doi: 10.1111/j.1469-1795.2010.00368.x
Ecological Stratification Working Group (1995). A National Ecological Framework for Canada. Ottawa: Centre for Land and Biological Resources Research and Environment Canada.
Ellington, E. H., and Murray, D. L. (2015). Influence of hybridization on animal space use: a case study using coyote range expansion. Oikos 124, 535–542. doi: 10.1111/oik.01824
Forester, J., Im, H., and Rathouz, P. (2009). Accounting for animal movement in estimation of resource selection functions: sampling and data analysis. Ecology 90, 3554–3565. doi: 10.1890/08-0874.1
Fortin, D., Beyer, H. L., Boyce, M. S., Smith, D. S., Duchesne, T., and Mao, J. S. (2005). Wolves influence elk movements: behavior shapes a trophic cascade in Yellowstone National Park. Ecology 86, 1320–1330. doi: 10.1890/04-0953
Fox, J., and Weisberg, S. (2019). An R Companion to Applied Regression, Third Edn. Thousand Oaks, CA: Sage.
Franckowick, G. A., Perdicas, M., and Smith, G. A. (2019). Spatial ecology of coyotes in the urbanizing landscape of the Cuyahoga Valley, Ohio. PLoS One 14:e0227028. doi: 10.1371/journal.pone.0227028
Gehrt, S. D., Anchor, C., and White, L. A. (2009). Home range and landscape use of coyotes in a metropolitan landscape: conflict or coexistence? J. Mammol. 90, 1045–1057. doi: 10.1644/08-MAMM-A-277.1
Gese, E. M. (2001). Territorial defense by coyotes (Canis latrans) in Yellowstone National Park, Wyoming: who, how, where, when, and why? Can. J. Zool. 79, 980–987. doi: 10.1139/cjz-79-6-980
Gese, E. M., Morey, P. S., and Gehrt, S. D. (2012). Influence of the urban matrix on space use of coyotes in the Chicago metropolitan area. J. Ethol. 30, 413–425. doi: 10.1007/s10164-012-0339-8
Gese, E. M., Rongstad, O. J., and Mytton, W. R. (1988). Home range and habitat use of coyotes in southeastern Colorado. J. Wildl. Manag. 52, 640–646. doi: 10.2307/3800923
Gese, E. M., Rongstad, O. J., and Mytton, W. R. (1989). Population dynamics of coyotes in southeastern Colorado. J. Wildl. Manag. 53, 174–181. doi: 10.2307/3801326
Gese, E. M., Ruff, R. L., and Crabtree, R. L. (1996). Social and nutritional factors influencing the dispersal of resident coyotes. Anim. Behav. 52, 1025–1043. doi: 10.1006/anbe.1996.0250
Gipson, P. S., Ballard, W. B., Nowak, R. M., and Mech, L. D. (2000). Accuracy and precision of estimating age of gray wolves by tooth wear. J. Wildl. Manag. 64, 752–758. doi: 10.2307/3802745
Grinder, M. I., and Krausman, P. R. (2001). Home range, habitat use, and nocturnal activity of coyotes in an urban environment. J. Wildl. Manag. 65, 887–898. doi: 10.2307/3803038
Harrison, D. J., Harrison, J. A., and O’Donoghue, M. (1991). Predispersal movements of coyote (Canis latrans) pups in eastern Maine. J. Mammal. 72, 756–763. doi: 10.2307/1381839
Henke, S. E., and Bryant, F. C. (1999). Effects of coyote removal on the faunal community in Western Texas. J. Wildl. Manag. 63, 1066–1081. doi: 10.2307/3802826
Hopkins, J. B., Koch, P. L., Schwartz, C. C., Ferguson, J. M., Greenleaf, S. S., and Kalinowski, S. T. (2012). Stable isotopes to detect food-conditioned bears to evaluate human-bear management. J. Wildl. Manag. 76, 703–713. doi: 10.1002/jwmg.318
Jones, B. M., Cove, M. V., Lashley, M. A., and Jackson, V. L. (2016). Do coyotes Canis latrans influence occupancy of prey in suburban forest fragments. Curr. Zool. 62, 1–6. doi: 10.1093/cz/zov004
Kamler, J. F., and Gipson, P. S. (2000). Space and habitat use by resident and transient coyotes. Can. J. Zool. 78, 2106–2111. doi: 10.1139/z00-153
Kays, R., Curtis, A., and Kirchman, J. J. (2010). Rapid adaptive evolution of northeastern coyotes via hybridization with wolves. Bot. Lett. 6, 89–93. doi: 10.1098/rsbl.2009.0575
Knowlton, F. F., Gese, E. M., and Jaeger, M. M. (1999). Coyote depredation control: an interface between biology and management. J. Range Manag. 52, 398–412. doi: 10.2307/4003765
Krester, H. E., Sullivan, P. J., and Knuth, B. A. (2008). Housing density as an indicator of spatial patterns of reported human-wildlife interactions in Northern New York. Landsc. Urban Plan. 84, 282–292. doi: 10.1016/j.landurbplan.2007.08.007
Laliberte, A. S., and Ripple, W. J. (2004). Range contractions of North American carnivores and ungulates. Bioscience 54, 123–138.
Larson, R. N., Brown, J. L., Karels, T., and Riley, S. P. D. (2020). Effects of urbanization of resource use and individual specialization by coyotes (Canis latrans) in southern California. PLoS One 15:e0228881. doi: 10.1371/journal.pone.0228881
Lukasik, V. M., and Alexander, S. M. (2011). Human-coyote interactions in Calgary, Alberta. Hum. Dimens. Wildl. 16, 114–127. doi: 10.1080/10871209.2011.544014
Magle, S. B., Poessel, S. A., Crooks, K. R., and Breck, S. W. (2014). More dogs less bite: the relationship between human-coyote conflict and prairie dog colonies in an urban landscape. Landsc. Urban Plan. 127, 146–153. doi: 10.1016/j.landurbplan.2014.04.013
Mississauga (2014). Existing Land Use. City of Mississauga. Available online at: http://www5.mississauga.ca/research_catalogue/CityofMississauga_TermsofUse.pdf (accessed October, 2014).
Mitchell, N., Strohbach, M. W., Pratt, R., Finn, W. C., and Strauss, E. G. (2015). Space use by resident and transient coyotes in an urban-rural landscape mosaic. Wildl. Res. 42, 461–469. doi: 10.1071/WR15020
Monzõn, J., Kays, R., and Dyhuizen, D. E. (2014). Assessment of coyote-wolf-dog admixture using ancestry informative diagnostic SNPs. Mol. Ecol. 23, 182–197. doi: 10.1111/mec.12570
Morey, P. S., Gese, E. M., and Gehrt, S. (2007). Spatial and temporal variation in the diet of coyotes in the Chicago Metropolitan Area. Am. Midl. Nat. 158, 147–161.
Muff, S., Signer, J., and Fieberg, J. (2020). Accounting for individual-specific variation in habitat-selection studies: efficient estimation of mixed effects models using Bayesian or frequentist computation. J. Anim. Ecol. 89, 90–92.
Murray, M. H., Cembrowski, A., Latham, A. D., Lukasik, V. M., Pruss, S., and St Clair, C. C. (2015a). Greater consumption of protein-poor anthropogenic food by urban relative to rural coyotes increases diet breadth and potential for human-wildlife conflict. Ecography 38, 1235–1242. doi: 10.1111/ecog.01128
Murray, M. H., Edwards, M. A., Abercrombie, B., and St Clair, C. C. (2015b). Poor health is associated with use of anthropogenic resources in an urban carnivore. Proc. R. Soc. B. 282:20150009. doi: 10.1098/rspb.2015.0009
Murray, M. H., and St Clair, C. C. (2017). Predictable features attract urban coyotes to residential yards. J. Wildl. Manag. 81, 593–600. doi: 10.1002/jwmg.21223
Newsome, S. D., Garbe, H. M., Wilson, E. C., and Gehrt, S. D. (2015a). Individual variation in anthropogenic resource use in an urban carnivore. Oecologia 178, 115–128. doi: 10.1007/s00442-014-3205-2
Newsome, T. M., Dellinger, J. A., Pavey, C. R., Ripple, W. J., Shores, C. R., Wirsing, A. J., et al. (2015b). The ecological effects of providing resource subsidies to predators. Glob. Ecol. Biogeogr. 24, 1–11. doi: 10.1111/geb.12236
Oakville (2014). Zoning By-Law 2014-014. Available online at: https://www.oakville.ca/data/open_data_licence.html (accessed October, 2014).
Peel Region (2003). Digitized Brampton’s Zoning Areas from Generalized Land Use 2003. Available online at: http://www.peelregion.ca/planning/maps/glum03.pdf (accessed October, 2014).
Pettersson, L. B., and Bronmark, C. (1993). Trading of safety against food: state-dependent habitat choice and foraging in Crucian Carp. Oecologia 95, 353–357. doi: 10.1007/BF00320988
Poessel, S. A., Breck, S. W., and Gese, E. M. (2016). Spatial ecology of urban coyotes in the Denver metropolitan area: influence of the urban matrix. J. Mammal. 97, 1414–1427. doi: 10.1093/jmammal/gyw090
Poessel, S. A., Breck, S. W., Teel, T. L., Shwiff, S., Crooks, K., and Angeloni, L. (2013). Patterns of human-coyote conflict in the Denver metropolitan area. J. Wildl. Manag. 77, 297–305. doi: 10.1002/jwmg.454
Poessel, S. A., Gese, E. M., and Young, J. K. (2017a). Environmental factors influencing the occurrence of coyotes and conflicts in urban areas. Landsc. Urban Plan. 157, 259–269. doi: 10.1016/j.landurbplan.2016.05.022
Poessel, S. A., Mock, E. C., and Breck, S. W. (2017b). Coyote (Canis latrans) diet in an urban environment: variation relative to pet conflicts, housing density, and season. Can. J. Zool. 95, 287–297.
Quinn, T. (1997). Coyote (Canis latrans) food habits in three urban habitat types of Western Washington. Northwest Sci. 71, 1–5.
Riley, S. P., Sauvajot, R. M., Fuller, T. K., York, E. C., Kamradt, D. A., Bromley, C., et al. (2003). Effects of urbanization and habitat fragmentation on bobcats and coyotes in Southern California. Conserv. Biol. 17, 566–576. doi: 10.1046/j.1523-1739.2003.01458.x
Sacks, B. N., Jaeger, M. M., Neale, J. C. C., and McCullough, D. R. (1999). Territoriality and breeding status of coyotes relative to sheep predation. J. Wildl. Manag. 63, 593–605. doi: 10.2307/3802648
Signer, J., Fieberg, J., and Avgar, T. (2019). Animal movement tools (amt): R package for managing tracking data and conducting habitat selection analyses. Ecol. Evol. 9, 880–890. doi: 10.1002/ece3.4823
Southern Ontario Land Resource Information System (SOLRIS) (2008). Land Use Data version 1.2. Toronto, Ont: The Ontario Ministry of Natural Resources.
Statistics Canada (2011). Focus on Geography Series, 2011 Census. Available online at: http://www12.statcan.gc.ca/census-recensement/2011/as-sa/fogs-spg/index-eng.cfm (accessed October, 2014).
Toronto (2013). Zoning Bylaw 569-2013. Available online at: https://www.toronto.ca/city-government/data-research-maps/open-data/open-data-licence/ (accessed October, 2014).
Towns, L., Derocher, A. E., Stirling, I., Lunn, N. J., and Hedman, D. (2009). Spatial and temporal patterns of problem polar bears in Chruchill, Manitoba. Polar Biol. 32, 1529–1537. doi: 10.1007/s00300-009-0653-y
Treves, A., and Karanth, K. U. (2003). Human-carnivore conflict and perspectives on carnivore management worldwide. Conserv. Biol. 17, 1491–1499.
Wasserstein, R. L., and Lazar, N. A. (2016). The ASA statement on p-Values: context, process, and purpose. Am. Stat. 70, 129–133. doi: 10.1080/00031305.2016.1154108
Way, J. G. (2011). Eastern coyote/coywolf (Canis latrans x lycaon) movement patterns: lessons learned in an urbanized ecosystem. Cities Environ. 4:2. doi: 10.15365/cate.4122011
Way, J. G., and Eatough, D. L. (2006). Use of “micro”-corridors by eastern coyotes, Canis latrans, in a heavily urbanized area: implications for ecosystem management. Can. Field Nat. 102, 474–476. doi: 10.22621/cfn.v120i4.358
Wheeldon, T. J. (2020). Population Dynamics of Eastern Coyotes in Southeastern Ontario. Ph.D. thesis. Peterborough, Ont: Trent University.
Wheeldon, T. J., Rutledge, L. Y., Patterson, B. R., White, B. N., and Wilson, P. J. (2013). Y-chromosome evidence supports asymmetric dog introgression into eastern coyotes. Ecol. Evol. 3, 3005–3020. doi: 10.1002/ece3.693
White, L. A., and Gehrt, S. D. (2009). Coyote attacks on humans in the United States and Canada. Hum. Dimens. Wildl. 14, 419–432. doi: 10.1080/10871200903055326
Wood, S. N., and Augustin, N. H. (2002). GAMs with integrated model selection using penalized regression splines and applications to environmental modelling. Ecol. Modell. 157, 157–177.
Keywords: Canis latrans, conflict, coyote, habitat, step selection function, urban, residential
Citation: Thompson CA, Malcolm JR and Patterson BR (2021) Individual and Temporal Variation in Use of Residential Areas by Urban Coyotes. Front. Ecol. Evol. 9:687504. doi: 10.3389/fevo.2021.687504
Received: 29 March 2021; Accepted: 07 May 2021;
Published: 01 June 2021.
Edited by:
Darryl Jones, Griffith University, AustraliaReviewed by:
Sharon Poessel, United States Geological Survey (USGS), United StatesMaureen Murray, Lincoln Park Zoo, United States
Copyright © 2021 Thompson, Malcolm and Patterson. This is an open-access article distributed under the terms of the Creative Commons Attribution License (CC BY). The use, distribution or reproduction in other forums is permitted, provided the original author(s) and the copyright owner(s) are credited and that the original publication in this journal is cited, in accordance with accepted academic practice. No use, distribution or reproduction is permitted which does not comply with these terms.
*Correspondence: Connor A. Thompson, Y29ubm9ydGhvbXBzb25AdHJlbnR1LmNh