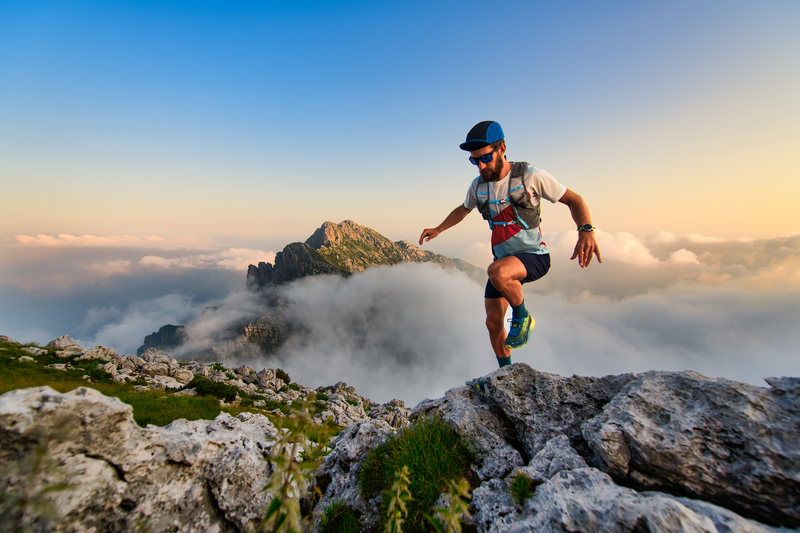
94% of researchers rate our articles as excellent or good
Learn more about the work of our research integrity team to safeguard the quality of each article we publish.
Find out more
ORIGINAL RESEARCH article
Front. Educ. , 13 February 2025
Sec. Assessment, Testing and Applied Measurement
Volume 10 - 2025 | https://doi.org/10.3389/feduc.2025.1483092
The current investigation aims to adapt and validate the Teacher Education and Development Study-Instruct observation instrument for assessing teaching quality in new contexts: Norwegian Grade 6 mathematics and science lessons. More specifically, the article examines content validity and reliability in the new contexts using a multi-methods approach, involving the Delphi technique and generalizability theory. Findings suggest that while the core components of the instrument are relevant in the new contexts, specific adaptations are necessary to capture teaching quality in a more nuanced and meaningful way. Based on the findings, specific adaptions are made to the instrument. Finally, recommendations for developing and using the instrument in the new contexts are provided. The current investigation underscores the importance of contextual sensitivity in the assessment of teaching quality.
Standardized observation instruments are widely recognized and used as effective tools for assessing teaching quality (Bell et al., 2019; Janik et al., 2009; Praetorius and Charalambous, 2018). Developing these instruments is a time-intensive and complex process (Praetorius and Charalambous, 2018). Consequently, it is common practice to “export” observation instruments from one context — such as national, subject, or grade-level context — to another. For example, the Classroom Assessment Scoring System (CLASS), originally developed at the University of Virginia in the US (Pianta et al., 2008), has been widely used in countries across the globe as diverse as Australia (Thorpe et al., 2023), Chile (Leyva et al., 2015), China (Hu et al., 2016), Ecuador (Araujo et al., 2016), Finland (Virtanen et al., 2018), Germany (Bihler et al., 2018), the Netherlands (Slot et al., 2017), Norway (Westergård et al., 2019), Portugal (Cadima et al., 2010), and Singapore (Ng et al., 2021). Similarly, the Protocol for Language Arts Teaching Observation (PLATO) was developed at the University of Pennsylvania to assess English language arts instruction (Grossman et al., 2013) and later adopted by the Linking Instruction and Student Achievement (LISA) study to assess mathematics teaching in Nordic classrooms (Klette et al., 2017).
However, observation instruments generally conceptualize and operationalize a community’s view of quality teaching and learning (Bell et al., 2019). Given that ‘quality’ is an inherently vague and ambiguous concept that requires value judgments (Wittek and Kvernbekk, 2011; Berliner, 2005), it is only natural that most observation instruments are context-specific and context-sensitive. As a result, applying observation instruments in contexts vastly different from those in which they were originally developed can be highly problematic (Liu et al., 2019; Muijs et al., 2018). For example, although transferring instruments across related subjects is often more feasible (Cohen et al., 2018; Praetorius et al., 2016), exporting them across national border can be problematic since educational systems might define key-components of high-quality teaching differently (Berliner, 2005; Luoto et al., 2022; Muijs et al., 2018)1. This issue is exacerbated when researchers use existing evidence of an instruments’ reliability and validity from one context to assert its applicability in another, neglecting that such evidence is not inherent to a specific instrument but rather to specific empirical studies using the instrument in their own unique context (AERA, APA, NCME, 2014; Liu et al., 2019; Praetorius and Charalambous, 2018). Therefore, it is essential to reassess whether an observation instrument can be used in a meaningful and relevant way when applied in new contexts (Liu et al., 2019; Luoto et al., 2022).
The current study addresses this issue by examining the extent to which the Teacher Education and Development Study–Instruct (TEDS-Instruct) standardized observation instrument — developed in Germany (Schlesinger and Jentsch, 2016; Schlesinger et al., 2018; Kaiser et al., 2017) — can be used to assess teaching quality in new contexts: Norwegian Grade 6 mathematics and science lessons. To this end, the present investigation contains two separate but complementary empirical studies. The first study aims to obtain validity evidence based on the content of the instrument, which is at the core of validation and the beginning of any validation process (AERA, APA, NCME, 2014; Liu et al., 2019; Praetorius and Charalambous, 2018). Taking the stance that teaching quality is context-specific and context-sensitive, we employ the Delphi technique to elicit the opinions of Norwegian mathematics and science education experts regarding the relevance and representativeness of TEDS-Instruct for its new contexts.
The second study aims to obtain evidence on the reliability of TEDS-Instruct within its new contexts by employing generalizability theory (GT) using a sample of Norwegian mathematics and science lessons rated by four trained raters using TEDS-Instruct. GT is a powerful way to examine reliability as it enables researchers to systematically distinguish between multiple sources of error (Brennan, 1992; Praetorius and Charalambous, 2018). This can be useful for understanding various sources of error and designing more efficient measurement procedures (Brennan, 1992). The results from both studies will be used (1) make necessary adaptations to the instrument, (2) provide recommendations for its further development, and (3) provide recommendations for its use in the new contexts.
In this study, we define teaching quality as those classroom interactions, both among students and between students and teachers, that provide (sustained) learning opportunities and align with contemporary educational norms and standards (Berliner, 1987; Charalambous et al., 2021; Fenstermacher and Richardson, 2005; Praetorius and Charalambous, 2023). Decades of research have established teaching quality as one of the most significant malleable factors in schools that influence student learning outcomes (Muijs et al., 2014; Scheerens et al., 2007; Seidel and Shavelson, 2007). A wide range of theoretical frameworks, models, and (observation) instruments have been developed to understand and assess teaching quality (e.g., Creemers and Kyriakides, 2008; Ferguson and Danielson, 2015; Klieme et al., 2009), each with distinct theoretical underpinning and development processes (Praetorius and Charalambous, 2018). Consequently, they reflect a community’s view on teaching and learning, resulting in variations in their coverage, structure, and terminology (Bell et al., 2019; Blikstad-Balas et al., 2021; Senden et al., 2022).
In addition, there has been an ongoing debate about the extent to which the measurement of teaching quality should attend to subject-specific teaching practices, which are informed by the demands of teaching in a specific discipline, or to generic teaching practices that adhere to the demands of teaching across disciplines (Charalambous and Kyriakides, 2017; Cohen et al., 2018). Scholars have argued that including both sets of practices can provide a more complete picture of what happens in the classroom (Blazar et al., 2017; Charalambous and Praetorius, 2018). This might especially be important since subject-specific teaching practices have been shown to explain a substantial amount of variance in student learning outcomes (Baumert et al., 2010; Charalambous and Kyriakides, 2017; Seidel and Shavelson, 2007). Including both set of practices can be done by simultaneously employing subject-specific and generic observation instruments, as done in the Measures of Effective Teaching (MET) project (Kane and Cantrell, 2010). Another option is to employ observation instruments which include both sets of practices, so called hybrid observation instruments (Charalambous and Praetorius, 2018; Senden et al., 2022).The present study draws on such a hybrid instrument to conceptualize and operationalize teaching quality: the TEDS-Instruct observation instrument (Schlesinger et al., 2018).
The observation instrument was developed as part of the German TEDS-Instruct study to examine student learning of mathematics in Grades 7–10, independent of the topic discussed in class (Kaiser et al., 2017). In response to repeated calls for bringing together generic and subject-specific teaching practices (Blazar et al., 2017; Charalambous and Praetorius, 2018), the developers of the TEDS-Instruct observation instrument extended the well-established generic framework of the Three Basic Dimensions of teaching quality (Klieme et al., 2001; Klieme et al., 2009; Praetorius et al., 2018; Praetorius et al., 2020) with mathematics-specific teaching practices (Schlesinger and Jentsch, 2016; Schlesinger et al., 2018). To achieve this, a mathematic-specific description of teaching quality was developed based, among others, on a systematic literature review of existing classroom observation instruments (Schlesinger and Jentsch, 2016; Schlesinger et al., 2018). The observation instrument was initially piloted in the TEDS-Instruct study, and later employed in the TEDS-Validate study (Kaiser et al., 2017; Kaiser and König, 2020; Schlesinger et al., 2018). The obtained data was additionally used to further develop the instrument, which led to the use of a refined four-dimensional conceptualization, including the Three Basic Dimensions — classroom management, personal learning support, and cognitive activation — and a fourth dimension specific to mathematics education: educational structuring (Jentsch et al., 2020; Jentsch et al., 2021b).
1. Classroom management refers to the strategies and techniques teachers use to organize and manage a complex classroom environment (Doyle, 1985; Emmer and Stough, 2001). In a well-managed classroom, undesirable behaviors are prevented from happening and desirable behaviors are identified and encouraged, thereby fostering a positive and respectful atmosphere while providing opportunities for instruction and learning (Doyle, 1985; Emmer and Stough, 2001). Effectively managed classrooms are characterized by the teacher setting clear and consistent rules and expectations for student behavior, providing stable routines and well-structured and planned lessons, and monitoring and redirecting student behavior (Klieme et al., 2009; Kounin, 1970). Effective classroom management can support students’ social–emotional and academic learning, increase student and teacher retention, prevent burnout and stress symptoms of teachers, and avert serious aggression or behavioral problems among students (Brouwers and Tomic, 2000; Oliver et al., 2011; Sabornie and Espelage, 2022; Seidel and Shavelson, 2007).
2. Personal learning support focuses on the teacher’s capacity to deal with heterogeneity in students’ abilities and respond to comprehension difficulties of the individual student, and to the collective student body (Kunter and Voss, 2013). As such, it includes aspects of instruction related to collaborative learning, inclusion, and differentiation. Personal learning support is assumed to increase the active participation of students, which can lead to more successful learning processes (Turner et al., 1998)
3. Cognitive activation refers to instructional strategies that facilitate opportunities for students to engage in higher-level cognitive thinking that promotes conceptual understanding (Klieme et al., 2009; Lipowsky et al., 2009). More specifically, it pertains to instructional situations in which learning is challenging, interactive, and co-constructive, and in which the teacher provides support for students’ individual construction of knowledge (Baumert et al., 2010; Lipowsky et al., 2009). Cognitive activation is assumed to increase students’ knowledge and understanding (Klieme et al., 2009).
4. Educational structuring includes subject-specific aspects of instruction that originally pertain to the demands of teaching mathematics. Educational structuring addresses the degree to which teachers adapt to students’ individual cognitive abilities and provide instructional support if needed (so-called scaffolding, van de Pol et al., 2010). This adds to the previous quality dimensions by taking further aspects of teaching into account (e.g., structural clarity, explanations, consolidation, see also Drollinger-Vetter, 2011). Kleickmann et al. (2010) report positive associations between educational structuring (termed cognitive support in their study) and student achievement in science classrooms.
To accurately capture the four dimensions, each dimension is represented by several high-inferences sub-dimensions. These sub-dimensions are further broken down into specific indicators, which reflect typical observable behaviors associated with each item (see Appendix Table 1). It is important to note, that while observers use these indicators to guide their assessment, they actually rate the high-inference sub-dimensions themselves, not the indicators. Ratings are assigned using a four-point scale. Additionally, the instrument is accompanied by a comprehensive rating manual that provides further guidelines for scoring, as well as a detailed description of the high-inference sub-dimensions.
The current study aims to obtain validity evidence based on the content of TEDS-Instruct by eliciting the opinions of subject-matter experts using the Delphi technique. The study is guided by the following research questions (RQs):
RQ1: To what extent do subject-matter experts agree that TEDS-Instruct can be used for a relevant assessment of teaching quality in Norwegian Grade 6 mathematics and science lessons?
RQ2: To what extent do subject-matter experts agree that the sub-dimensions assessed through TEDS-Instruct are representative of the overarching dimensions of teaching quality in Norwegian Grade 6 mathematics and science lessons?
The preliminary phase of this study involved the first four authors forming a focus group to assess the relevance of TEDS-Instruct for the new contexts: Norwegian Grade 6 mathematics and science lessons. The focus group met on five occasions, each lasting between 1.5–2 h. Initially, the group focused on identifying sub-dimensions and indicators requiring adjustment. It was concluded that the sub-dimensions and indicators of the three basic dimensions — classroom management, personal learning support, and cognitive activation — required minimal adaptions to be applicable for Grade 6 mathematics and science lessons in Norway. However, the fourth dimension, educational structuring, which was tailored specifically to mathematics, was identified as needing several adaptations to be applicable to science lessons.
Subsequent adaptations to the instrument were based on dialogue and discussion, supplemented by examples from other observation instruments. Adaptations were kept to a minimum and only when there was sufficient agreement among the group. This process led to the first version of the instrument, which was further tested during study 1 and study 2 (see Figure 1). A complete overview of the preliminary adaptions made to the instrument is available in the Supplementary material. The version of the instrument employed in Study 1 and Study 2 is found in the Appendix Table 1.
The Delphi technique can be broadly defined as “a method for structuring a group communication process so that the process is effective in allowing a group of individuals, as a whole, to deal with a complex problem” (Linstone and Turoff, 1975, p. 3). The Delphi technique has been successfully employed in educational research (Green, 2014; Helmer, 1966), including for validation purposes (e.g., Mengual-Andrés et al., 2016; Smith and Simpson, 1995). In the current study, we did not adopt the traditional approach of generating items. Instead, we ask participants to evaluate a previously developed instrument. This variation of the Delphi technique is also known as the “reactive Delphi” (McKenna, 1994).
It was agreed upon that we would recruit Norwegian subject-matter experts to evaluate the TEDS-Instruct observation instrument. To this end, we employed purposeful sampling to select Norwegian subject-matter experts (Palinkas et al., 2015). Our criteria required significant experience in teaching, involvement in teacher education, and/or research expertise in mathematics or science education. Furthermore, we aimed to include experts from various public educational institutions (1) ensure diverse perspectives and maintain anonymity, and (2) minimize the possibility that opinions would be influenced through participant interactions. Finally, we targeted a balanced representation of mathematics and science education experts.
Based on these criteria, we identified 16 experts and invited them by mail to participate and co-author the Delphi study. Of these, 12 experts (75%) confirmed their participation, representing eight governmental institutions. The participants included six experts specialized in mathematics education — four full professors, one associate professor, and one Ph.D. candidate — and six in science education, comprising two full professors and four associate professors.
Next, we started the iterative two-round Delphi process (see, e.g., Dalkey and Helmer, 1963; Linstone and Turoff, 1975; McKenna, 1994). During this stage, we aimed to obtain data about the relevance and representativeness of TEDS-Instruct by eliciting the opinions of subject-matter experts. Instead of meeting for face-to-face discussions, participants were provided with extensive online questionnaires to complete individually, ensuring participant anonymity and confidentiality of their responses. These measures were taken to reduce the impact of social-psychological influences, such as reluctance to express divergent opinions, the unwillingness to abandon publicly expressed opinions, or following what seems to be the majority’s opinion (Helmer, 1966; Ho and McLeod, 2008).
In the first questionnaire round, participants received detailed information about TEDS-Instruct and instructions on how to complete the questionnaire. They were then asked to express their opinions on two validity-related topics: (1) the relevance and (2) the representativeness of the sub-dimensions assessed through the instrument.
Mathematics experts were asked to rate the extent to which they believe the sub-dimensions of TEDS-Instruct are relevant for Norwegian Grade 6 mathematics lessons. Similarly, science experts assessed their relevance for science lessons (e.g., “Rate the extent to which you believe time-on-task is relevant to assess in Norwegian Grade 6 science lessons”). The experts were provided with a brief description of each sub-dimension and typical examples of observable behaviors (indicators). They then answered on a four-point Likert scale with strongly irrelevant (coded 1), irrelevant (coded 2), relevant (coded 3), and strongly relevant (coded 4). If experts rated a sub-dimension as strongly irrelevant or irrelevant, they were requested to provide a reason/explanation for their opinion.
All experts were asked to assess how well the sub-dimensions represent the overarching dimensions (e.g., “Rate the extent to which you believe the sub-dimensions adequately represent the dimension of classroom management”). They responded on a four-point Likert scale with not at all (coded 1), not very (coded 2), somewhat (coded 3), and to a large extent (coded 4). While providing explanations for their ratings was optional, experts were encouraged to elaborate on their responses.
The second questionnaire round was developed based on the evaluation and analysis of results obtained from the first round (see Figure 1). Initially, we assessed the extent of agreement among experts. For this purpose, consensus criteria were established (see Table 1) based on a systematic review of definitions of consensus in Delphi studies (Diamond et al., 2014). The criteria for positive agreement were set to a median score ≥ 3, and at least 75% of responses being either 3 or 4. For negative agreement, the criteria were a median ≤ 2 and less than 25% of the responses being either 3 or 4. Disagreement was defined as between 25 and 75% of the responses being either 3 or 4.
The following approach was agreed upon: If the experts demonstrated positive agreement, the relevant question would not be followed up on in the second questionnaire round. In cases where the experts showed disagreement, the reason or explanation provided by them in their open-ended responses would be summarized and provided back to them in the second round, offering an opportunity to revise their opinions. Additionally, if there were negative agreement on the relevance of a sub-dimension, it would be considered for removal from the instrument. Similarly, negative agreement on the representativeness would prompt us to consider revising the structure of the dimensions, based on the expert’s feedback. The revised structure would then be provided back to the experts in the second questionnaire round to assess if the changes enhanced representativeness (see Figure 2).
Finally, responses to the open-ended questions would be summarized and analyzed to identify any problems or recurring themes that would need to be addressed in the second questionnaire round.
Based on the evaluation of the results from the first questionnaire, the second round did not include questions on the representativeness or relevance of the sub-dimension, due to overall positive agreement. Instead, based on insights gained from the analyses of the open-ended responses, the experts were asked to assess the relevance of the indicators (e.g., “Rate the extent to which you believe these indicators are relevant for assessing time on task in Norwegian Grade 6 science lessons”). Expert agreement was again evaluated using the previously stated consensus criteria. Additionally, an open-ended question was included to solicit recommendations for potentially more suitable indicators (e.g., “Are there any alternative indicators that you believe would be more suitable for assessing time on task in Norwegian Grade 6 mathematics lessons?”).
All 12 experts who initially agreed to participate responded to both questionnaires. Of these, seven experts indicated that they had previously used observation instruments to assess teaching quality.
Analyses of the responses from the first questionnaire round revealed a high degree of consensus among experts regarding the relevance of the sub-dimensions assessed through TEDS-Instruct for Norwegian Grade 6 mathematics and science lessons (see Appendix Table 1). Based on the consensus criteria, both mathematics and science experts indicated positive agreement for all 21 sub-dimensions. In other words, all sub-dimensions were relevant to strongly relevant for assessing teaching quality in Norwegian Grade 6 mathematics and science lessons. Consequently, we concluded that it was unnecessary to continue enquiring about the relevance of the sub-dimensions in the second questionnaire round.
However, subsequent analysis of the open-ended responses revealed two main themes. Firstly, while there was broad agreement on the relevance of the sub-dimensions, several experts were critical about the relevance of the indicators, which were presented as typical examples of observable behaviors. Secondly, experts offered recommendations for clarifying and developing the instrument, often with a specific focus on refining the indicators. Based on these insights, it was determined that the second questionnaire round should focus on exploring the relevance of the indicators and soliciting recommendations for their improvement.
Results from the second questionnaire showed a large amount of positive agreement regarding the relevance of the indicators. In other words, the majority of indicators were considered relevant to strongly relevant to assess the sub-dimensions, with similar findings in both mathematics and science (see Appendix Table 1). However, there was also disagreement regarding the relevance of nine indicators in mathematics and four in science. Notably, following the consensus criteria, no indicators reached negative agreement, indicating experts did not agree on any indicators being irrelevant or strongly irrelevant. Further analysis of the open-ended responses revealed numerous suggestions from experts on how to improve the indicators to better suit the Norwegian Grade 6 mathematics and science context. These suggestions were later analyzed by the focus group to adapt the instrument and provide recommendations for its future development and use.
Analysis of the responses from the first questionnaire round (see Table 2) revealed that experts in mathematics education agreed that, across all four dimensions, the sub-dimensions were representative of the overarching dimensions. Similar results were found when analyzing the responses of science experts. However, the median reveals that science experts agreed to a lesser extent than mathematics experts regarding personal learning, support, cognitive activation, and educational structuring. A median score of 3 indicates that these dimensions are “somewhat” represented by their respective sub-dimensions.
Table 2. Expert opinions on the extent to which the sub-dimensions of the TEDS-Instruct observation instrument adequately represent the overarching dimension.
According to previously established consensus criteria, experts in this study largely agreed that the sub-dimensions and indicators provided by the TEDS-Instruct observation instrument appear relevant to strongly relevant for assessing teaching quality in Norwegian Grade 6 mathematics and science lessons. However, due to the small sample size, these findings should be interpreted as preliminary, and further validation with a larger expert group, and including teachers, could clarify whether these findings hold more broadly.
One possible hypothesis is, that this finding might be a result of validating the content of the instrument in contexts that are relatively similar, such as Germany and Norway or across mathematics and science. In line with the notion that the conceptualization of teaching quality is shaped by societal and cultural values (Luoto, 2020; Pacheco, 2009), we might expect less agreement in vastly different contexts. Nevertheless, we argue that the instrument could benefit from further development based on the recommendations provided by the experts through answering the open-ended questions. Incorporating these recommendations could improve the instrument’s relevance for assessing teaching quality in the new contexts. To this end, final adaptations and recommendations are provided at the end of this article.
However, there is also a drawback when adapting an existing instrument. While adapting an instrument can improve the relevance of the assessment, it also reduces the potential for cross-contextual and international comparisons. In the field of international comparative research, Clarke et al. (2012) refer to this trade-off as the ‘validity-comparability compromise’. They argue that “pursuing commensurability by imposing general classificatory frameworks can misrepresent valued performances, school knowledge and classroom practice as these are conceived by each community and sacrifice validity in the interest of comparability” (Clarke et al., 2012, p. 171). While initially discussed as a theoretical concern in cross-cultural research, we argue that this compromise also applies to cross-contextual comparisons, such as across grade levels and subjects. Therefore, the current approach of adapting an instrument to new contexts might lead to a more relevant assessment of teaching quality, but at the expense of comparability. A possible solution would be to develop a set of ‘core practices’ that are shown to be stable across contexts alongside flexible, context-specific ones.
Moreover, an interesting finding of the current study was that experts predominantly discussed and referred to the indicators when asked about the relevance of the instrument in the new contexts. In responding to the open-ended questions, experts provided a substantial number of recommendations for modifying the indicators. This might be due to the indicators being more concrete and easier to suggest modifications for, or it could be that the indicators are more sensitive to context. Regardless, this provides an indication that while the dimensions and sub-dimensions are applicable to the new contexts, refining the indicators might be beneficial to better reflect the typical observable behaviors of the overarching sub-dimensions in the new contexts.
Finally, experts agreed that the sub-dimensions are representative of the overarching dimensions in both mathematics and science. However, the results also indicate that for personal learning support, cognitive activation, and educational structuring, the sub-dimensions are less representative of the overarching dimension in science than mathematics. A possible cause is the instrument initially being developed for mathematics lessons and thus more geared towards the subject of mathematics. In this case, the instrument might benefit from further development aimed at increasing the representativeness of the sub-dimensions to assess teaching quality in science lessons. This seems especially so for the dimension of educational structuring.
In conclusion, while these findings provide valuable insights, future studies with a larger and more diverse expert sample are needed to confirm and expand upon these results, further informing instrument adaptation for cross-contextual applications.
Together, Study 1 and 2 provide evidence of validity and reliability in relation to using the TEDS-Instruct observation instrument in the context of Norwegian primary school (Grade 6) within science and mathematics. However, while Study 1 evaluated the validity of the content of TEDS-Instruct, the current study utilizes this instrument to score video observations to evaluate its reliability. The goal of the present study is to investigate the reliability of the scores on the four dimensions of teaching quality using TEDS-Instruct. More specifically, we employ generalizability theory (GT) to investigate whether the scores adequately reflect variation across lessons and classrooms, whether the rater bias is high, whether the reliability is sufficient, and how all this differs between mathematics and science. We address this by asking the following research questions (RQs):
RQ1: What is the psychometric quality of the scoring of the four dimensions of teaching quality in terms of:
a. the share of variance attributed by differences across classrooms, lessons, segments, and raters?
b. relative (without rater bias) and absolute (with rater bias) reliability and standard errors of measurement?
RQ2: How do these variances, relative and absolute reliabilities and standard errors of measurement differ between mathematics and science?
An important consideration when assessing teaching quality through classroom observations is how to ensure high score reliability and valid conclusions while allocating limited resources. To examine this, we employ generalizability theory (GT; Cronbach et al., 1972; Brennan, 2001) to explore to what extent scores obtained with our instrument reflect meaningful variation across classrooms and lessons and sufficient reliability. Based on these findings we provide recommendations for future use of the instrument.
GT was developed specifically for complex measurement situations with many potential sources of variation, such as classrooms, lessons, or raters. This is often encountered in research employing classroom observations, and GT has successfully been used to analyze data for these purposes (e.g., Casabianca et al., 2013; Mashburn et al., 2014). GT provides a fine-grained picture of reliability, which is done in two steps. In step one, the different sources of variation (such as rater bias or variation across classrooms) are investigated. For instruments used in classroom observations, it is useful to estimate the variance across classrooms, lessons, and raters. In studies examining teaching quality, the variance across classrooms would reflect the degree to which teaching quality differs from one classroom to the next. Large variation could reflect differences in teachers’ competence, differences in the classroom composition (some classrooms may be more difficult to teach than others), or a combination thereof. Variation across lessons within the same classrooms would reflect that teaching quality changes over time. Large variations across raters would reflect high rater bias and could indicate that more training or a higher number of raters is needed.
Step two in GT is based on the amount of variance identified from the different sources. If, for instance, the variance between raters is found to be large, one could decide to sample additional raters to control for measurement error in a follow-up study. Given the purpose of a study, one can estimate the overall reliability coefficient and standard errors of measurement with and without rater bias (i.e., some raters being stricter than others, such that they systematically assign lower scores throughout the scoring process). Rater bias can be problematic if scores are used for criterion-referenced decisions. However, it may be ignored in situations where only the rank ordering of lessons or classrooms is of interest (e.g., correlational studies). In our study, the relative standard error and reliability coefficient reflect estimates that ignore rater bias, while the absolute error includes rater bias. If there are large differences between raters, the absolute error would thus be much higher than the relative.2
The data analyzed in this study are videotaped lessons collected for the Teachers’ Effect on Student Outcome (TESO) project. Data was obtained from nine schools and 15 classrooms from the Oslo metropolitan area in Norway in the autumn of 2019 and spring of 2020. The 15 classrooms that were sampled, also participated in the large-scale assessment Trends in Mathematics and Science Study (TIMSS) in 2019. In each classroom, 1–6 mathematics and/or science lessons were videotaped over the course of several months. The length of the lessons varied between 24 and 106 min, and lessons were cut into, on average, 20-min segments for analysis (Schlesinger et al., 2018), as recommended in several studies (e.g., Mashburn et al., 2014). The sample size per subject can be found in Table 3. Nine teachers taught both mathematics and science.
Rating the videos took place during three weeks in the summer of 2023. We applied the TEDS-Instruct instrument comprising 21 items that were scored on a four-point Likert-type rating scale (ranging from 1 through 4). In the first week, raters were trained extensively by studying the rating manual, conducting video observations, and discussing the results with master raters. However, no benchmarks were applied. During the second week, the raters double-scored the first two segments of each lesson (a total of 30 segments in math, and 26 in science). After double-scoring a lesson, the raters discussed their ratings among each other, but they did not have to agree on a score. In the third week, the ratings used in the current study were obtained by having each individual lesson scored by a single rater. A total of four raters scored the videos. All raters were student teachers in their third year or later within different science, technology, engineering, and mathematics (STEM) programs. Scores were assigned using Interact software (Mangold, 2023).
In a first step, we calculated the mean scores of each dimension (i.e., classroom management, personal learning support, cognitive activation, educational structuring). Second, we estimated descriptives and correlations on the dimension level (see Appendix Table 2). We then applied GT (Cronbach et al., 1972; Brennan, 2001) to estimate measurement error and reliability in our study. GT makes use of the linear mixed model to estimate variance components for each measurement facet of interest (Brennan, 2001). We estimated variance components for classrooms, lessons (i.e., the objects of measurement), lesson segments, and raters using the REML estimator from the free R package lme4 (Bates et al., 2015). Separate GT analyses were performed for the two subjects and all teaching quality dimensions. They were compared across subject domains regarding absolute and relative error of measurement as well as reliability. The reliability coefficients were calculated correspondingly by taking either true variance over the sum of true variance and relative error variance, or true variance over the sum of true variance and absolute error variance. Reliability coefficients were interpreted similar as classical reliability coefficients: ≤ 0.5: low reliability, 0.5–0.70: moderate reliability, 0.70–0.9: good reliability, ≥ 0.90: excellent reliability (Cortina, 1993; Koo and Li, 2016).
In the following, the results are presented in the order of the research questions.
Figure 3 presents the results of a variance decomposition for mathematics and science lessons. In mathematics classrooms, only small portions of variance are due to differences between classrooms. The share of total variance across classrooms is below 7 %, except for ES (which is about 33%). This suggests that teaching quality regarding classroom management, personal learning support, and cognitive activation is similar across the observed mathematics classrooms. However, lessons within classrooms in mathematics contribute largely to the total variability. Except for cognitive activation, the variances between lessons are between 24 and 33 percent. Further sources of variance include segments and raters. We see that variability in scores attributed to segments was only substantial for classroom management (23%) and cognitive activation (10%), which suggests that personal learning support and educational structuring scores do not vary much during a lesson. Finally, we observe a large rater (main) effect for cognitive activation, which suggests that raters systematically vary in their strictness when scoring this dimension.
Figure 3. Variance components (in percentage) for the four dimensions of teaching quality in mathematics and science. CM, classroom management; PLS, personal learning support; CA, cognitive activation; ES, educational structuring.
For science lessons and classrooms, the results point in a different direction. The share of total variance that is due to variation across classrooms is substantial for all dimensions (up to 57% for classroom management), but no variation between lessons within classrooms was observed. This suggests that raters assign similar scores to a classroom independent of the specific lessons that they scored. In other words, this means that scores are stable across lessons within a science classroom. What is more, little variation was found for segments within lessons (except personal learning support, approx. 18%). This indicates that teaching quality is stable during a lesson. With regards to rater bias, only a small portion of variance was due to differences between raters in terms of classroom management and personal learning support, but the share was large for the other two dimensions. This might show that raters had more difficulties in assigning scores for cognitive activation and educational structuring.
Table 4 provides combined relative and absolute measurement error and reliabilities coefficients. The relative measures do not include rater bias, while the absolute does. We see that similar relative and absolute estimates are found for cases in which the portion of variance attributed to raters is low. For mathematics, all reliability coefficients can be considered sufficient except for cognitive activation. Which had low reliability.
For science, all dimensions reached good or even excellent reliabilities with regard to the relative estimates. The absolute estimates are sufficient but substantially lower and could be improved by, for example, having multiple raters rate the same lesson. This is especially the case for cognitive activation in both subjects, but also for educational structuring in science.
Comparing the overall reliability between mathematics and science encompasses taking all the evidence from the variance components, standard errors, and reliability coefficients into account. The variance attributed to raters in science was highest for educational structuring and cognitive activation which resulted in lower absolute reliabilities. Cognitive activation stands out as having a high amount of variance attributed to raters in both subjects. However, while the absolute reliability for this dimension was acceptable for science (0.71), it was very low for mathematics (0.35), whereas educational structuring had high absolute reliability in mathematics.
Classroom management differed substantially between the two subjects. In mathematics, most of the variance in the scores were due to variance over lessons and segments, while most of the variance in scores in science were due to differences between classrooms. In spite of no rater bias in mathematics, the reliabilities are smaller, and standard errors larger, than in science for this dimension of teaching quality. The similarities and differences pointed out, will be discussed in light of the Norwegian context and previous research in the next section.
One of the main findings in study 2, is that a very small share of variance in mathematics could be attributed to variation between classrooms. Rather, the larger portion of variance was found between lessons. This finding is different from what was found in German studies (e.g., Jentsch et al., 2021a) and also different from the findings in science found in the current study. This could imply that differences between mathematics teachers’ competence is smaller than that between science teachers. Indeed, findings from the larger, representative sample of TIMSS 2019, show that mathematics teachers have better qualifications, more specialization, and participated three times more often in professional development activities (Mullis et al., 2020). Moreover, these qualifications varied more between science teachers than mathematics teachers. Even though our sample is a sub-sample of TIMSS 2019, this could be a plausible explanation, and could also be observed in situ by those filming the lessons. In fact, the ratings of the mean overall teaching quality in mathematics for our sample was higher than those in science (see Appendix Table 2).
However, why the ratings mostly varied across lessons and segments in mathematics, in contrast to small variations across lessons and segments in science, is a more complicated question. It could be that high quality teaching is more sensitive to the composition of the classroom. In other words, it could be more difficult to maintain quality teaching over time and during a lesson in classrooms with many low SES students and students who do not speak Norwegian. Classroom management was the dimension that varied the most across lessons and segments in mathematics, and this dimension would probably also be most sensitive to the classroom composition.
It could, however, also be that mathematics lessons in the sample vary a lot in quality, and that more lessons are needed to capture more robust scores. In general, if scores mostly vary (much) between lessons within a classroom, this suggests that they are useful for giving feedback to teachers, for instance, but less so for long-term decisions, such as predicting student learning outcomes. This is because variance between classes or teachers most often is utilized when analyzing the effect of teaching quality on students’ learning outcomes. For science, a large proportion of the variance could be attributed to classrooms, whereas there was no variance that could be attributed to lessons. These findings suggest that the science scores could be useful for long-term decisions, but not necessarily to give feedback to teachers regarding a single lesson.
Finally, we experienced higher amounts of rater bias in science than in mathematics, in the sense that some raters were stricter than others. The rater bias was high in educational structuring in science, and in cognitive activation in both subjects. There were further relatively large portions of residual variance. Although this does not necessarily affect score reliability, more research is needed (1) find ways to train raters most efficiently, and (2) look at other variables or measurement facets that might affect the results, particularly regarding cognitive activation in mathematics classrooms. Overall, the reliability of the instrument is sufficient for scoring in the context of Norwegian 6th grade classrooms in science and mathematics, although cognitive activation in mathematics requires further work.
In the present investigation, both studies provided information on how to adapt and use the instrument in new contexts. In study 1, subject-matter experts provided recommendations for adapting the instrument further to be more relevant in its new contexts. Developing the instrument based on these recommendations could potentially improve the extent to which the instrument assesses teaching quality in a meaningful and relevant way in the new contexts. In addition, study 2 examined the functioning of the instrument in the new contexts. Recommendations based on these findings could potentially inform future applications of the instrument in research and practice.
To deal with this information, the first four authors of this paper came together again as a focus group to discuss the recommendations. The focus group met on six occasions for 1.5–2. hours. Based on information received through the open-ended questions in the Delphi study and information from the generalizability analysis, the focus group made adaptations to the instruments and its manual. In addition, the focus group concluded on recommendations for using and further developing TEDS-Instruct in Norwegian Grade 6 mathematics and science lessons. A full overview of the adaptations made to the instrument and the updated version of the instrument can be found in the Supplementary materials. Future research will have to test the updated instrument and possibly develop it further. To this end, collecting additional validity evidence will be necessary to form a more complete validity argument.
The current instrument assesses personal learning support, which is considered a component of a supportive classroom climate. Teaching practices related to emotional or social support are not explicitly included in the assessment of personal learning support. However, these forms of support are considered a key element in Norwegian classrooms. For further development, we would therefore recommend explicitly including such teaching practices in the instrument.
Moreover, the instrument was originally developed to assess teaching quality in mathematics lessons. Even though the current study has made several adaptions in order to use the instrument in science lessons, results from the Delphi study hinted at the need to include a more thorough assessment of subject-specific teaching practices in science. The generalizability study further confirmed these findings by providing evidence that educational structuring in science, in contrast to mathematics, was difficult to rate consistently across raters. Developing the instrument to be more directed towards subject-specific science teaching — for example, by including measures assessing the “nature of science” or “inquiry” — could provide a more meaningful and relevant assessment of teaching quality in science lessons and more reliable scores.
Since subject-specific teaching practices in science might still be underrepresented in the current instrument, it could be recommended to use the instrument together with an instrument developed for measuring subject-specific science practices (e.g., inquiry) such as ISIOP (Minner and DeLisi, 2012). Moreover, findings from the generalizability study indicate that it might be necessary to rate more lessons in mathematics than in science to obtain reliable scores. However, these findings should be confirmed by subsequent studies. In line with Praetorius et al. (2014), our results confirm that having multiple raters score cognitive activation is necessary to obtain reliable scores. Additionally, more focus on rating cognitive activation during the training might be beneficial. For example, by employing additional quality criteria, such as comparing scores in cognitive activation to a master rater score.
A major strength of the current investigation is that it considers different sources of evidence to examine the validity and reliability of TEDS-Instruct in the new contexts.
The Delphi panel in study 1 consisted of a relatively small sample of experts, comprising six mathematics and six science education experts. The limited sample size may have contributed to the large amount of agreement among experts due to accidentally sampling experts with similar value systems. On the other hand, the panel represented a range of public educational institutions from across the country, ensuring a variety of perspectives. Consequently, future research taking a similar approach could increase the sample while aiming to keep a diverse panel. Additionally, the Delphi panel consisted solely of scholars, and future research could benefit from including teachers’ as experts. However, this might also lead to more disagreement as teachers could lack the theoretical knowledge on teaching quality. Despite these potential challenges, the Delphi technique can be a powerful approach to simultaneously validate and adapt an observation instrument to new contexts. In doing so, the adapted instrument better represents the communities view on teaching and learning.
In study 2, a meaningful inter-rater reliability coefficient could not be calculated or reported. This was due to the raters being given the opportunity to discuss their scores with each other during the double scoring process in week 2, which compromised the independence of their ratings. Moreover, the small sample utilized in the generalizability study limited the statistical power. These constraints limited our ability to estimate models with more variables or interaction terms which likely would have provided more information. For example, including the time of the day the lessons were recorded as an extra variable in the GT analysis could prove insightful. Additionally, while the study focused on the four overarching dimensions of teaching quality, it is expected that variation differs between sub-dimensions as well. Furthermore, the lesson content is not standardized, and the sample of classrooms is expected to vary in several regards, e.g., background characteristics of the students, teacher qualifications. These contextual factors are not taken into account in the generalizability analysis but can have implications for the reliability estimates (White et al., 2022). Despite these limitations, we believe the results of the generalizability study to be valuable for gauging reliability and providing recommendations for the future use of the instrument in its new context. Findings from the study can increase the reliability of ratings in future studies, while decreasing costs associated with using observation instruments.
The datasets presented in this article are not readily available because they contain confidential and sensitive information, which cannot be shared due to privacy and ethical restrictions. Requests to access the datasets should be directed to Bas Senden at YmFzc2VuZGVuQGdtYWlsLmNvbQ==.
BS: Conceptualization, Data curation, Formal analysis, Investigation, Methodology, Project administration, Validation, Writing – original draft, Writing – review & editing. AJ: Conceptualization, Data curation, Formal analysis, Investigation, Methodology, Validation, Writing – original draft, Writing – review & editing. NT: Conceptualization, Data curation, Formal analysis, Investigation, Methodology, Project administration, Supervision, Validation, Writing – review & editing. TN: Conceptualization, Data curation, Formal analysis, Funding acquisition, Investigation, Methodology, Project administration, Resources, Supervision, Validation, Writing – original draft, Writing – review & editing. JF: Writing – review & editing. TF: Writing – review & editing. MM: Writing – review & editing. SM: Writing – review & editing. RM: Writing – review & editing. GN: Writing – review & editing. MO: Writing – review & editing. MS: Writing – review & editing. RLS: Writing – review & editing. AS: Writing – review & editing. RBS: Writing – review & editing. MØ: Writing – review & editing.
The author(s) declare that financial support was received for the research, authorship, and/or publication of this article. This work was supported by the FINNUT Researcher Project awarded by the Research Council of Norway under Grant number 283587 and partially supported by the Research Council of Norway through its Centers of Excellence scheme under Grant number 331640.
We would like to express our utmost gratitude to Professor Gabriele Kaiser and Professor Johannes König for their foundational work in developing the TEDS-Instruct observation instrument and their generosity in granting us access to the instrument. We would also like to thank the four raters—Helene, Neha, Sandra, and Jonathan—for their enthusiasm and devotion in rating the videos. Finally, our thanks goes to Andreas Petersen for testing, and providing feedback on, the initial Delphi questionnaires.
The authors declare that the research was conducted in the absence of any commercial or financial relationships that could be construed as a potential conflict of interest.
All claims expressed in this article are solely those of the authors and do not necessarily represent those of their affiliated organizations, or those of the publisher, the editors and the reviewers. Any product that may be evaluated in this article, or claim that may be made by its manufacturer, is not guaranteed or endorsed by the publisher.
The Supplementary material for this article can be found online at: https://www.frontiersin.org/articles/10.3389/feduc.2025.1483092/full#supplementary-material
1. ^A notable exception is the International System for Teacher Observation and Feedback (ISFOT), which was developed to ensure cross-cultural relevance (Muijs et al., 2018; Teddlie et al., 2006).
2. ^In more precise and statistical terms, the relative error (Shavelson and Webb, 1991) reflects the extent to which rank ordering of lessons is distorted (i.e., their relative standing). The absolute error is estimated if scores are compared to a certain cutoff value.
AERA, APA, NCME (2014). Standards for educational and psychological testing. Washington, DC: American Educational Research Association.
Araujo, M. C., Carneiro, P., Cruz-Aguayo, Y., and Schady, N. (2016). Teacher quality and learning outcomes in kindergarten. Q. J. Econ. 131, 1415–1453. doi: 10.1093/qje/qjw016
Bates, D., Mächler, M., Bolker, B., and Walker, S. (2015). Fitting linear mixed-effects models using lme4. J. Stat. Softw. 67, 1–48. doi: 10.18637/jss.v067.i01
Baumert, J., Kunter, M., Blum, W., Brunner, M., Voss, T., Jordan, A., et al. (2010). Teachers' mathematical knowledge, cognitive activation in the classroom, and student progress. Am. Educ. Res. J. 47, 133–180. doi: 10.3102/0002831209345157
Bell, C. A., Dobbelaer, M. J., Klette, K., and Visscher, A. (2019). Qualities of classroom observation systems. Sch. Eff. Sch. Improv. 30, 3–29. doi: 10.1080/09243453.2018.1539014
Berliner, D. C. (1987). “Simple views of effective teaching and a simple theory of classroom instruction” in Talks to teachers. eds. D. C. Berliner and B. Rosenshine (New York, NY: Random House), 93–110.
Berliner, D. C. (2005). The near impossibility of testing for teacher quality. J. Teach. Educ. 56, 205–213. doi: 10.1177/0022487105275904
Bihler, L.-M., Agache, A., Kohl, K., Willard, J. A., and Leyendecker, B. (2018). Factor analysis of the classroom assessment scoring system replicates the three domain structure and reveals no support for the bifactor model in German preschools. Front. Psychol. 9:1232. doi: 10.3389/fpsyg.2018.01232
Blazar, D., Braslow, D., Charalambous, C., and Hill, H. (2017). Attending to general and mathematics-specific dimensions of teaching: exploring factors across two observation instruments. Educ. Assess. 22, 71–94. doi: 10.1080/10627197.2017.1309274
Blikstad-Balas, M., Tengberg, M., and Klette, K. (2021). “Why – and how – should we measure instructional quality?” in Ways of analyzing teaching quality. eds. M. Blikstad-Balas, K. Klette, and M. Tengberg (Oslo: Scandinavian University Press), 9–20.
Brennan, R. L. (1992). Generalizability theory. Educ. Meas. Issues Pract. 11, 27–34. doi: 10.1111/j.1745-3992.1992.tb00260.x
Brouwers, A., and Tomic, W. (2000). A longitudinal study of teacher burnout and perceived self-efficacy in classroom management. Teach. Teach. Educ. 16, 239–253. doi: 10.1016/S0742-051X(99)00057-8
Cadima, J., Leal, T., and Burchinal, M. (2010). The quality of teacher–student interactions: associations with first graders' academic and behavioral outcomes. J. Sch. Psychol. 48, 457–482. doi: 10.1016/j.jsp.2010.09.001
Casabianca, J. M., McCaffrey, D. F., Gitomer, D., Bell, C., Hamre, B. K., and Pianta, R. C. (2013). Effect of observation mode on measures of secondary mathematics teaching. Educ. Psychol. Meas. 73, 757–783. doi: 10.1177/0013164413486987
Charalambous, C. Y., and Kyriakides, E. (2017). Working at the nexus of generic and content-specific teaching practices: an exploratory study based on TIMSS secondary analyses. Elem. Sch. J. 117, 423–454. doi: 10.1086/690221
Charalambous, C. Y., and Praetorius, A.-K. (2018). Studying mathematics instruction through different lenses: setting the ground for understanding instructional quality more comprehensively. ZDM 50, 355–366. doi: 10.1007/s11858-018-0914-8
Charalambous, C. Y., Praetorius, A.-K., Sammons, P., Walkowiak, T., Jentsch, A., and Kyriakides, L. (2021). Working more collaboratively to better understand teaching and its quality: challenges faced and possible solutions. Stud. Educ. Eval. 71:101092. doi: 10.1016/j.stueduc.2021.101092
Clarke, D., Wang, L., Xu, L., Aizikovitsh-Udi, E., and Cao, Y. (2012). “International comparisons of mathematics classrooms and curricula: the validity-comparability compromise” in PME 36: Opportunities to learn in mathematics education: Proceedings of the 36th conference of the International Group for the Psychology of mathematics education 2012 (Taipei, Taiwan: National Taiwan Normal University), 171–178.
Cohen, J., Ruzek, E., and Sandilos, L. (2018). Does teaching quality cross subjects? Exploring consistency in elementary teacher practice across subjects. AERA Open 4, 1–16. doi: 10.1177/2332858418794492
Cortina, J. M. (1993). What is coefficient alpha? An examination of theory and applications. J. Appl. Psychol. 78, 98–104. doi: 10.1037/0021-9010.78.1.98
Creemers, B. P. M., and Kyriakides, L. (2008). The dynamics of educational effectiveness: A contribution to policy, practice and theory in contemporary schools. London: Routledge.
Cronbach, L. J., Glaser, G. C., Nanda, H., and Rajaratnam, N. (1972). The dependability of behavioral measurements: Theory of generalizability for scores and profiles. Hoboken, NJ: John Wiley.
Dalkey, N., and Helmer, O. (1963). An experimental application of the Delphi method to the use of experts. Manag. Sci. 9, 458–467. doi: 10.1287/mnsc.9.3.458
Diamond, I. R., Grant, C., Feldman, M., Pencharz, P. B., Ling, S. C., Moore, A. M., et al. (2014). Defining consensus: A systematic review recommends methodologic criteria for reporting of Delphi studies. J. Clinic. Epidemiol. 67, 401–409. doi: 10.1016/j.jclinepi.2013.12.002
Doyle, W. (1985). Recent research on classroom management: implications for teacher education. J. Teach. Educ. 36, 31–35. doi: 10.1177/002248718503600307
Drollinger-Vetter, B. (2011). Verstehenselemente und strukturelle klarheit: Fachdidaktische qualität der anleitung von mathematischen verstehensprozessen im unterricht. Münster: Waxmann.
Emmer, E. T., and Stough, L. M. (2001). Classroom management: a critical part of educational psychology, with implications for teacher education. Educ. Psychol. 36, 103–112. doi: 10.1207/S15326985EP3602_5
Fenstermacher, G. D., and Richardson, V. (2005). On making determinations of quality in teaching. Teachers College Record 107, 186–213. doi: 10.1111/j.1467-9620.2005.00462.x
Ferguson, R., and Danielson, C. (2015). “How framework for teaching and tripod 7Cs evidence distinguish key components of effective teaching” in Designing teacher evaluation systems: New guidance from the measures of effective teaching project. eds. T. J. Kane, K. A. Kerr, and R. C. Pianta (Hoboken, NJ: Wiley), 98–143.
Green, R. A. (2014). The Delphi technique in educational research. SAGE Open 4, 1–8. doi: 10.1177/2158244014529773
Grossman, P., Loeb, S., Cohen, J., and Wyckoff, J. (2013). Measure for measure: the relationship between measures of instructional practice in middle school English language arts and teachers’ value-added scores. Am. J. Educ. 119, 445–470. doi: 10.1086/669901
Helmer, O. (1966). The use of the Delphi technique in problems of educational innovations (P-3499). Santa Monica, CA: The RAND Corporation.
Ho, S. S., and McLeod, D. M. (2008). Social-psychological influences on opinion expression in face-to-face and computer-mediated communication. Commun. Res. 35, 190–207. doi: 10.1177/0093650207313159
Hu, B. Y., Fan, X., Gu, C., and Yang, N. (2016). Applicability of the classroom assessment scoring system in Chinese preschools based on psychometric evidence. Early Educ. Dev. 27, 714–734. doi: 10.1080/10409289.2016.1113069
Janik, T., Seidel, T., and Najvar, P. (2009). “Introduction: on the power of video studies in investigating teaching and learning” in The power of video studies in investigating teaching and learning in the classroom. eds. T. Janik and T. Seidel (Münster: Waxmann), 7–19.
Jentsch, A., Casale, G., Schlesinger, L., Kaiser, G., König, J., and Blömeke, S. (2020). Variabilität und generalisierbarkeit von ratings zur qualität von mathematikunterricht zwischen und innerhalb von unterrichtsstunden. Unterrichtswissenschaft 48, 179–197. doi: 10.1007/s42010-019-00061-8
Jentsch, A., Heinrichs, H., Schlesinger, L., Kaiser, G., König, J., and Blömeke, S. (2021a). “Multi-group measurement invariance and generalizability analyses for an instructional quality observational instrument” in Ways of analyzing teaching quality. eds. M. Blikstad-Balas, K. Klette, and M. Tengberg (Oslo: Scandinavian University Press), 121–139.
Jentsch, A., Schlesinger, L., Heinrichs, H., Kaiser, G., König, J., and Blömeke, S. (2021b). Erfassung der fachspezifischen qualität von mathematikunterricht: Faktorenstruktur und zusammenhänge zur professionellen kompetenz von mathematiklehrpersonen. J. Math.-Didakt. 42, 97–121. doi: 10.1007/s13138-020-00168-x
Jentsch, A., and Senden, B. (n.d.). Hybrid frameworks to capture teaching quality in secondary classrooms – The case of the TEDS-Instruct observation system.
Kaiser, G., Blömeke, S., König, J., Busse, A., Döhrmann, M., and Hoth, J. (2017). Professional competencies of (prospective) mathematics teachers—cognitive versus situated approaches. Educ. Stud. Math. 94, 161–182. doi: 10.1007/s10649-016-9713-8
Kaiser, G., and König, J. (2020). “Analyses and validation of central assessment instruments of the research program TEDS-M” in Student learning in German higher education. eds. O. Zlatkin-Troitschanskaia, H. A. Pant, M. Toepper, and C. Lautenbach (Berlin: Springer).
Kane, T. J., and Cantrell, S. (2010). Learning about teaching: initial findings from the measures of effective teaching project [MET project research paper]. Bill & Melinda Gates Foundation. Available online at: https://docs.gatesfoundation.org/Documents/preliminary-findings-research-paper.pdf
Kleickmann, T., Vehmeyer, J., and Möller, K. (2010). Zusammenhänge zwischen lehrervorstellungen und kognitivem Strukturieren im unterricht am beispiel von scaffolding-maßnahmen. Unterrichtswissenschaft 38, 210–228.
Klette, K., Blikstad-Balas, M., and Roe, A. (2017). Linking instruction and student achievement. A research design for a new generation of classroom studies. Acta Didactica Norge 11, 1–19. doi: 10.5617/adno.4729
Klieme, E., Pauli, C., and Reusser, K. (2009). “The Pythagoras study: investigating effects of teaching and learning in Swiss and German mathematics classrooms” in The power of video studies in investigating teaching and learning in the classroom. eds. T. Janik and T. Seidel (Münster: Waxmann), 137–160.
Klieme, E., Schümer, G., and Knoll, S. (2001). “Mathematikunterricht in der sekundarstufe I: "aufgabenkultur" und unterrichtsgestaltung” in TIMSS-Impulse für schule und unterricht. eds. E. Klieme and J. Baumert (Bonn: Bundesministerium für Bildung und Forschung), 43–57.
Koo, T. K., and Li, M. Y. (2016). A guideline of selecting and reporting Intraclass correlation coefficients for reliability research. J. Chiropr. Med. 15, 155–163. doi: 10.1016/j.jcm.2016.02.012
Kounin, J. S. (1970). Discipline and group management in classrooms. New York: Holt, Rinehart and Winston.
Kunter, M., and Voss, T. (2013). “The model of instructional quality in COACTIV: a multicriteria analysis” in Cognitive activation in the mathematics classroom and professional competence of teachers. Results from the COACTIV project. eds. M. Kunter, J. Baumert, W. Blum, U. Klusmann, S. Krauss, and M. Neubrand (Berlin: Springer), 97–124.
Leyva, D., Weiland, C., Barata, M., Yoshikawa, H., Snow, C., Treviño, E., et al. (2015). Teacher–child interactions in Chile and their associations with prekindergarten outcomes. Child Dev. 86, 781–799. doi: 10.1111/cdev.12342
Linstone, H., and Turoff, M. (1975). “Introduction” in The Delphi method: Techniques and applications. eds. H. Linstone and M. Turoff (Boston: Addison-Wesley Publishing Company), 3–12.
Lipowsky, F., Rakoczy, K., Pauli, C., Drollinger-Vetter, B., Klieme, E., and Reusser, K. (2009). Quality of geometry instruction and its short-term impact on students' understanding of the Pythagorean theorem. Learn. Instr. 19, 527–537. doi: 10.1016/j.learninstruc.2008.11.001
Liu, S., Bell, C. A., Jones, N. D., and McCaffrey, D. F. (2019). Classroom observation systems in context: a case for the validation of observation systems. Educ. Assess. Eval. Account. 31, 61–95. doi: 10.1007/s11092-018-09291-3
Luoto, J. (2020). Exploring, understanding, and problematizing patterns of instructional quality: A study of instructional quality in Finnish–Swedish and Norwegian lower secondary mathematics classrooms [Doctoral dissertation, University of Oslo]. DUO Research Archive. Available online at: http://urn.nb.no/URN:NBN:no-88324
Luoto, J., Klette, K., and Blikstad-Balas, M. (2022). Possible biases in observation systems when applied across contexts: conceptualizing, operationalizing, and sequencing instructional quality. Educ. Assess. Eval. Account. 35, 105–128. doi: 10.1007/s11092-022-09394-y
Mangold. (2023). Interact (version 18.7.7.17) [Computer software]. Available online at: https://www.mangold-international.com/en/products/software/behavior-research-with-mangold-interact.html
Mashburn, A. J., Meyer, J. P., Allen, J. P., and Pianta, R. C. (2014). The effect of observation length and presentation order on the reliability and validity of an observational measure of teaching quality. Educ. Psychol. Meas. 74, 400–422. doi: 10.1177/0013164413515882
McKenna, H. P. (1994). The Delphi technique: a worthwhile research approach for nursing? J. Adv. Nurs. 19, 1221–1225. doi: 10.1111/j.1365-2648.1994.tb01207.x
Mengual-Andrés, S., Roig-Vila, R., and Mira, J. B. (2016). Delphi study for the design and validation of a questionnaire about digital competences in higher education. Intern. J. Edu. Technol. High. 13:12. doi: 10.1186/s41239-016-0009-y
Minner, D., and DeLisi, J. (2012). Inquiring into science instruction observation protocol (ISIOP): Codebook. Waltham, MA: Education Development Center, Inc.
Muijs, D., Kyriakides, L., van der Werf, G., Creemers, B., Timperley, H., and Earl, L. (2014). State of the art – teacher effectiveness and professional learning. Sch. Eff. Sch. Improv. 25, 231–256. doi: 10.1080/09243453.2014.885451
Muijs, D., Reynolds, D., Sammons, P., Kyriakides, L., Creemers, B. P. M., and Teddlie, C. (2018). Assessing individual lessons using a generic teacher observation instrument: how useful is the international system for teacher observation and feedback (ISTOF)? ZDM 50, 395–406. doi: 10.1007/s11858-018-0921-9
Mullis, I. V. S., Martin, M. O., Foy, P., Kelly, D. L., and Fishbein, B. (2020). TIMSS 2019 international results in mathematics and science. TIMSS & PIRLS International Study Center, Lynch School of Education and Human Development, Boston College, and International Association for the Evaluation of Educational Achievement (IEA). Available online at: https://timssandpirls.bc.edu/timss2019/international-results/
Ng, E. L., Bull, R., Bautista, A., and Poon, K. (2021). A bifactor model of the classroom assessment scoring system in preschool and early intervention classrooms in Singapore. Int. J. Early Child. 53, 197–218. doi: 10.1007/s13158-021-00292-w
Oliver, R. M., Wehby, J. H., and Reschly, D. J. (2011). Teacher classroom management practices: effects on disruptive or aggressive student behavior. Campbell Syst. Rev. 7, 1–55. doi: 10.4073/csr.2011.4
Pacheco, A. (2009). “Mapping the terrain of teacher quality” in Measurement issues and assessment for teaching quality. ed. D. H. Gitomer (Thousand Oaks, CA: SAGE Publications), 160–178.
Palinkas, L. A., Horwitz, S. M., Green, C. A., Wisdom, J. P., Duan, N., and Hoagwood, K. (2015). Purposeful sampling for qualitative data collection and analysis in mixed method implementation research. Adm. Policy Ment. Health Ment. Health Serv. Res. 42, 533–544. doi: 10.1007/s10488-013-0528-y
Pianta, R. C., La Paro, K. M., and Hamre, B. K. (2008). Classroom assessment scoring system™: Manual K-3. Towson, MD: Paul H Brookes Publishing.
Praetorius, A.-K., and Charalambous, C. Y. (2018). Classroom observation frameworks for studying instructional quality: looking back and looking forward. ZDM 50, 535–553. doi: 10.1007/s11858-018-0946-0
Praetorius, A. K., and Charalambous, C. (2023). “Creating practical theories of teaching” in Theorizing teaching: Current status and open issues. eds. A.-K. Praetorius and C. Charalambous (Berlin: Springer), 23–56.
Praetorius, A.-K., Klieme, E., Herbert, B., and Pinger, P. (2018). Generic dimensions of teaching quality: the German framework of three basic dimensions. Math. Educ. 50, 407–426. doi: 10.1007/s11858-018-0918-4
Praetorius, A.-K., Klieme, E., Kleickmann, T., Brunner, E., Lindmeier, A., Taut, S., et al. (2020). Towards developing a theory of generic teaching quality: origin, current status, and necessary next steps regarding the three basic dimensions model. Zeitschrift für Pädagogik. 66, 15–36.
Praetorius, A.-K., Pauli, C., Reusser, K., Rakoczy, K., and Klieme, E. (2014). One lesson is all you need? Stability of instructional quality across lessons. Learn. Instr. 31, 2–12. doi: 10.1016/j.learninstruc.2013.12.002
Praetorius, A.-K., Vieluf, S., Saß, S., Bernholt, A., and Klieme, E. (2016). The same in German as in English? Investigating the subject-specificity of teaching quality. Z. Erzieh. 19, 191–209. doi: 10.1007/s11618-015-0660-4
Sabornie, E. J., and Espelage, D. L. (2022). Handbook of classroom management. 3rd Edn. London: Routledge.
Scheerens, J., Luyten, J. W., Steen, R., and de Thouars, Y. C. H. (2007). Review and meta-analyses of school and teaching effectiveness. Enschede: University of Twente.
Schlesinger, L., and Jentsch, A. (2016). Theoretical and methodological challenges in measuring instructional quality in mathematics education using classroom observations. ZDM 48, 29–40. doi: 10.1007/s11858-016-0765-0
Schlesinger, L., Jentsch, A., Kaiser, G., König, J., and Blömeke, S. (2018). Subject-specific characteristics of instructional quality in mathematics education. ZDM 50, 475–490. doi: 10.1007/s11858-018-0917-5
Seidel, T., and Shavelson, R. J. (2007). Teaching effectiveness research in the past decade: the role of theory and research design in disentangling meta-analysis results. Rev. Educ. Res. 77, 454–499. doi: 10.3102/0034654307310317
Senden, B., Nilsen, T., and Blömeke, S. (2022). “5. Instructional quality: a review of conceptualizations, measurement approaches, and research findings” in Ways of analyzing teaching quality: Potentials and pitfalls. eds. M. Blikstad-Balas, K. Klette, and M. Tengberg (Oslo: Scandinavian University Press), 140–172.
Shavelson, R. J., and Webb, N. M. (1991). Generalizability theory: A primer. Thousand Oaks, CA: SAGE Publications.
Slot, P. L., Boom, J., Verhagen, J., and Leseman, P. P. M. (2017). Measurement properties of the CLASS toddler in ECEC in the Netherlands. J. Appl. Dev. Psychol. 48, 79–91. doi: 10.1016/j.appdev.2016.11.008
Smith, K. S., and Simpson, R. D. (1995). Validating teaching competencies for faculty members in higher education: a national study using the Delphi method. Innov. High. Educ. 19, 223–234. doi: 10.1007/BF01191221
Teddlie, C., Creemers, B., Kyriakides, L., Muijs, D., and Yu, F. (2006). The international system for teacher observation and feedback: evolution of an international study of teacher effectiveness constructs. Educ. Res. Eval. 12, 561–582. doi: 10.1080/13803610600874067
Thorpe, K., Houen, S., Rankin, P., Pattinson, C., and Staton, S. (2023). Do the numbers add up? Questioning measurement that places Australian ECEC teaching as ‘low quality’. Aust. Educ. Res. 50, 781–800. doi: 10.1007/s13384-022-00525-4
Turner, J. C., Meyer, D. K., Cox, K. E., Logan, C., DiCintio, M., and Thomas, C. T. (1998). Creating contexts for involvement in mathematics. J. Educ. Psychol. 90, 730–745. doi: 10.1037/0022-0663.90.4.730
Van de Pol, J., Volman, M., and Beishuizen, J. (2010). Scaffolding in teacher–student interaction: a decade of research. Educ. Psychol. Rev. 22, 271–296. doi: 10.1007/s10648-010-9127-6
Virtanen, T. E., Pakarinen, E., Lerkkanen, M.-K., Poikkeus, A.-M., Siekkinen, M., and Nurmi, J.-E. (2018). A validation study of classroom assessment scoring system–secondary in the Finnish school context. J. Early Adolesc. 38, 849–880. doi: 10.1177/0272431617699944
Westergård, E., Ertesvåg, S. K., and Rafaelsen, F. (2019). A preliminary validity of the classroom assessment scoring system in Norwegian lower-secondary schools. Scand. J. Educ. Res. 63, 566–584. doi: 10.1080/00313831.2017.1415964
White, M., Luoto, J., Klette, K., and Blikstad-Balas, M. (2022). Bringing the conceptualization and measurement of teaching into alignment. Studies Educat. Evalu. 75:101204. doi: 10.1016/j.stueduc.2022.101204
Keywords: classroom observations, teaching quality, instructional quality, reliability, validity, Delphi technique, generalizability theory, Norwegian primary education
Citation: Senden B, Jentsch A, Teig N, Nilsen T, Fauskanger J, Frågåt T, Maugesten M, Mork SM, Mosvold R, Nortvedt GA, Olufsen M, Sjøberg M, Staberg RL, Selling A, Stovner RB and Ødegaard M (2025) Context matters: adapting and validating the TEDS-instruct observation instrument assessing teaching quality for its use in Norwegian primary education. Front. Educ. 10:1483092. doi: 10.3389/feduc.2025.1483092
Received: 19 August 2024; Accepted: 27 January 2025;
Published: 13 February 2025.
Edited by:
Maria Cutumisu, McGill University, CanadaReviewed by:
Luis Alex Alzamora De Los Godos Urcia, Cesar Vallejo University, PeruCopyright © 2025 Senden, Jentsch, Teig, Nilsen, Fauskanger, Frågåt, Maugesten, Mork, Mosvold, Nortvedt, Olufsen, Sjøberg, Staberg, Selling, Stovner and Ødegaard. This is an open-access article distributed under the terms of the Creative Commons Attribution License (CC BY). The use, distribution or reproduction in other forums is permitted, provided the original author(s) and the copyright owner(s) are credited and that the original publication in this journal is cited, in accordance with accepted academic practice. No use, distribution or reproduction is permitted which does not comply with these terms.
*Correspondence: Bas Senden, YmFzc2VuZGVuQGdtYWlsLmNvbQ==
†ORCID: Bas Senden, https://orcid.org/0000-0002-1955-2249
Disclaimer: All claims expressed in this article are solely those of the authors and do not necessarily represent those of their affiliated organizations, or those of the publisher, the editors and the reviewers. Any product that may be evaluated in this article or claim that may be made by its manufacturer is not guaranteed or endorsed by the publisher.
Research integrity at Frontiers
Learn more about the work of our research integrity team to safeguard the quality of each article we publish.