Published in
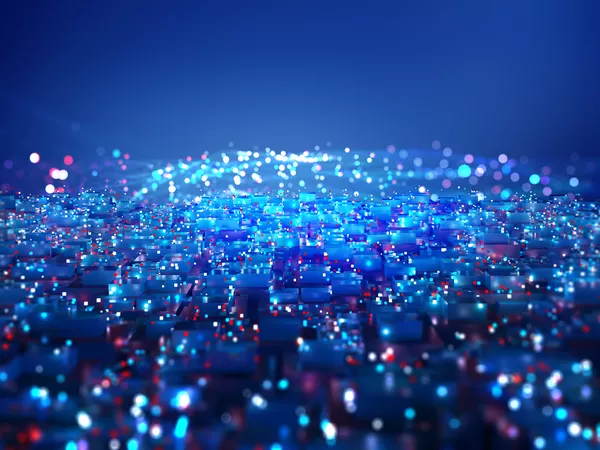
Frontiers in Artificial Intelligence
- Medicine and Public Health
1,109
Total views and downloads
Select the journal/section where you want your idea to be submitted:
Frontiers in Big Data
Manuscripts can be submitted to this Research Topic via the main journal or any other participating journal.
Select the journal/section where you want your idea to be submitted: