Published in
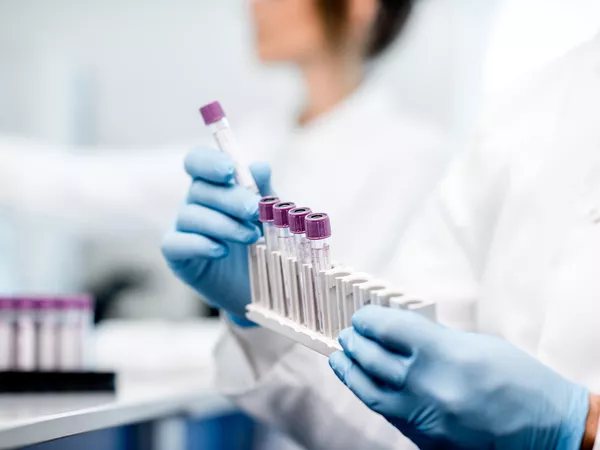
Frontiers in Medicine
Ophthalmology
1,960
Total downloads
10k
Total views and downloads
Submit your idea
Select the journal/section where you want your idea to be submitted:
Manuscripts can be submitted to this Research Topic via the main journal or any other participating journal.
Submit your idea
Select the journal/section where you want your idea to be submitted: