Published in
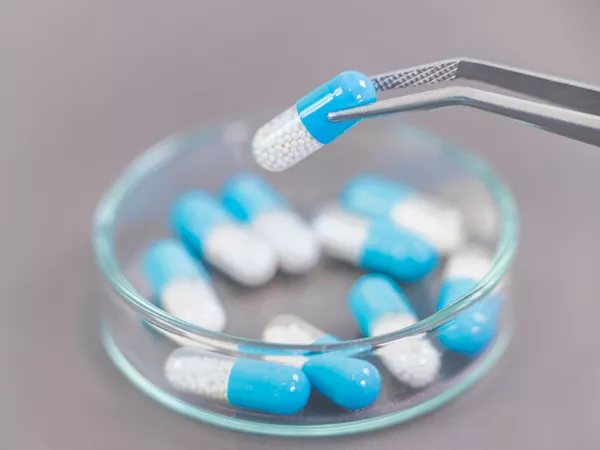
Frontiers in Drug Discovery
In silico Methods and Artificial Intelligence for Drug Discovery
720
Total views and downloads
Submit your idea
You will be redirected to our submission process.
Manuscripts can be submitted to this Research Topic via the main journal or any other participating journal.
Submit your idea
You will be redirected to our submission process.