- Science News
- Featured news
- New technique predicts risk of plant disease and infestation
New technique predicts risk of plant disease and infestation
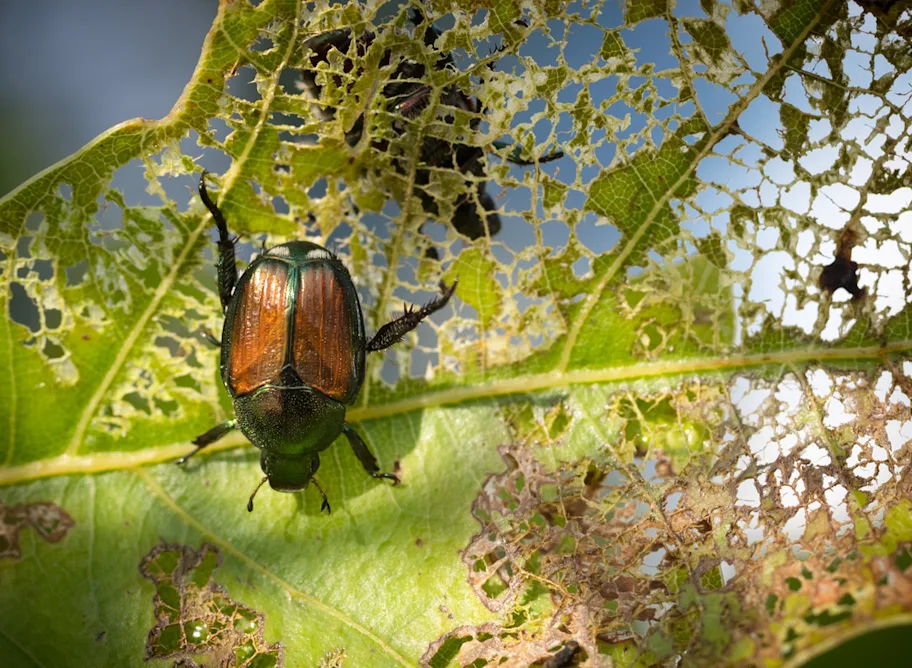
The technique is based on the principle that closely related plants that grow near each other are prone to infection or infestation by the same pathogens or pests. Image: Shutterstock
Researchers develop algorithms to predict the risk of disease or infestation in plants anywhere in the world
— By Conn Hastings
A newly developed technique can predict the risk of plant disease or infestation across the globe. Described in open-access journal Frontiers in Applied Mathematics and Statistics, the modeling technique considers the geographical distribution of vulnerable plants to provide maps of potential disease hotspots. This could help governments to understand the risk of outbreaks before they happen.
Diseases and pests can have a devastating impact on plants and the surrounding ecosystem. When outbreaks affect food crops, food shortages or even famine can result. The effects of plant infection or infestation can be particularly damaging when a pest or pathogen invades a new territory, in which native plants have little natural resistance and the destructive invader has few native predators or competitors.
Government agencies try to restrict pests and pathogens by controlling the movement of plants and animals between countries and regions. However, with international trade and travel, it can be difficult or impossible to stop pests and pathogens from spreading.
One way to get a head start in preventing outbreaks involves analyzing where known pests and pathogens are currently located, and then looking at the distribution of plants that might be vulnerable to attack, but this type of in-depth analysis can be time-consuming, given the huge array of plant, pathogen and pest species.
To address this, researchers in Mexico developed a series of algorithms to help predict these types of outbreaks. The technique is based on the principle that closely related plants that grow near each other are prone to infection or infestation by the same pathogens or pests. By studying the geographical distribution of closely-related plants, the research team generated maps of potential disease hotspots.
To test their algorithms, the team applied them to an invasive pest present in North America, the redbay ambrosia beetle. This invasive beetle transmits Laurel Wilt Disease, which can be deadly for plants of the laurel family. The researchers consulted online databases to find a group of ambrosia beetles that are closely related to the redbay ambrosia beetle, and a group of plant species that are associated with these beetles.
Using known beetle/plant interactions as a starting point, and then using their algorithms to estimate the probability that closely related plants would be similarly impacted, the researchers calculated the probability of each plant being affected by a particular beetle species.
The team then incorporated data about the known geographical distribution of each plant. If plants are found over large areas, then they are at higher risk for contracting and spreading an outbreak. Using their algorithms, the researchers calculated the probability of multiple plant species being infested by a beetle when the plants are present at the same site.
Using the technique, the team created maps showing regions of the world most likely to suffer infestation, or interaction between the beetles and plants. The maps accurately reflected the native territories of the beetles, along with the recent invasive behavior of some beetles, including the southward advance of one beetle across the United States. Worryingly, the model indicated that similar plants in Central and South America could be vulnerable to invasion next.
These types of maps could be very helpful for government agencies and ecologists in understanding and predicting outbreaks, by highlighting current or potential disease hotspots, but the team need further data from fieldwork to check the system’s accuracy.
However, these algorithms are not just applicable to plant infestations. “The method provides easy-to-use computer tools, which can be applied to understand and predict interactions between any group of organisms,” says Andrés Lira-Noriega, a researcher involved in the study.
The article is part of the Frontiers Research Topic Data Mining and Methods for Early Detection, Horizon Scanning, Modelling, and Risk Assessment of Invasive Species
Original research article: Combining Phylogenetic and Occurrence Information for Risk Assessment of Pest and Pathogen Interactions with Host Plants
Corresponding author: Andrés Lira-Noriega
REPUBLISHING GUIDELINES: Open access and sharing research is part of Frontier’s mission. Unless otherwise noted, you can republish articles posted in the Frontiers news blog — as long as you credit us with a link back to the original research. Selling the articles is not allowed.