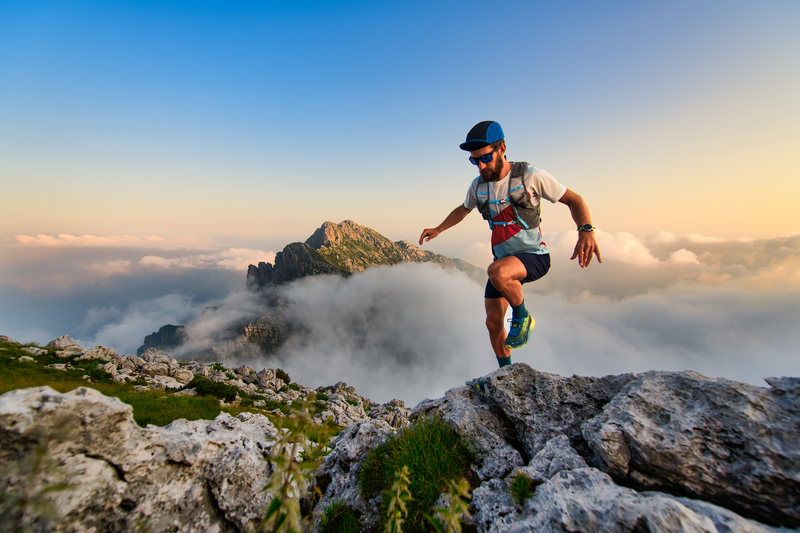
94% of researchers rate our articles as excellent or good
Learn more about the work of our research integrity team to safeguard the quality of each article we publish.
Find out more
ORIGINAL RESEARCH article
Front. Sustain. Food Syst.
Sec. Land, Livelihoods and Food Security
Volume 9 - 2025 | doi: 10.3389/fsufs.2025.1573149
The final, formatted version of the article will be published soon.
You have multiple emails registered with Frontiers:
Please enter your email address:
If you already have an account, please login
You don't have a Frontiers account ? You can register here
Many rural areas regard the tourism livelihood strategy as an essential breakthrough to overcome poverty and improve the lives of farmers. Studies have explored the income increasing effect of rural tourism on farmers' families, but few have focused on the impact of rural tourism on farmers' livelihood resilience under the requirements of rural sustainable development. The purpose of this paper is to explore the impact of rural tourism development on the livelihood resilience of rural households, using 987 households from the China Rural Revitalization Survey as case studies. The urban-rural gap in farmers' livelihood resilience was found to be narrowing. The rural tourism development has a significant promoting effect on enhancing the livelihood resilience of farmers. Specifically, the enhancement effect is mainly reflected in strengthening the livelihood buffering capacity, and the enhancement effect is most potent among high livelihood resilience farmers, non-poverty-stricken farmers, and farmers in the eastern region. The results of the mediation effect model indicate that livelihood factor mobility and livelihood strategy diversity are critical variables in the improvement process, with mediation effects accounting for 12.3% and 30.2%, respectively. The results of the moderation effect model further indicate that in enhancing farmers' livelihood resilience through rural tourism development, subjective well-being and perceived fairness play a positive moderating role. The findings contribute to a deeper understanding of whether and how rural tourism development can enhance farmers' livelihood resilience. We emphasize the need to develop rural tourism according to local conditions and implement precise policies to guide farmers in scientifically participating in rural tourism.
Keywords: Livelihood strategy diversity, Rural Development, Quality of Life, deep learning, subjective perception
Received: 08 Feb 2025; Accepted: 25 Feb 2025.
Copyright: © 2025 Niu and Zhou. This is an open-access article distributed under the terms of the Creative Commons Attribution License (CC BY). The use, distribution or reproduction in other forums is permitted, provided the original author(s) or licensor are credited and that the original publication in this journal is cited, in accordance with accepted academic practice. No use, distribution or reproduction is permitted which does not comply with these terms.
* Correspondence:
Yuxi Zhou, Shandong Agricultural University, Taian, China
Disclaimer: All claims expressed in this article are solely those of the authors and do not necessarily represent those of their affiliated organizations, or those of the publisher, the editors and the reviewers. Any product that may be evaluated in this article or claim that may be made by its manufacturer is not guaranteed or endorsed by the publisher.
Research integrity at Frontiers
Learn more about the work of our research integrity team to safeguard the quality of each article we publish.