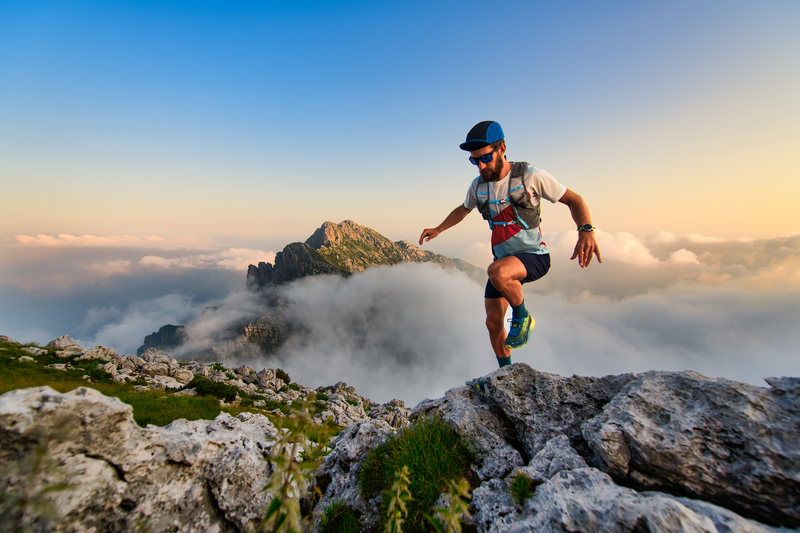
94% of researchers rate our articles as excellent or good
Learn more about the work of our research integrity team to safeguard the quality of each article we publish.
Find out more
REVIEW article
Front. Sustain. Food Syst.
Sec. Agro-Food Safety
Volume 9 - 2025 | doi: 10.3389/fsufs.2025.1571317
The final, formatted version of the article will be published soon.
You have multiple emails registered with Frontiers:
Please enter your email address:
If you already have an account, please login
You don't have a Frontiers account ? You can register here
This paper summarizes the existing methods of milk powder detection, and classifies them according to the direct and indirect characteristics of the detection methods, mainly introducing the detection methods of milk powder nutrition, recombination characteristics, transportation convenience and sensory characteristics. The direct detection methods of milk powder include traditional chemical analysis and modern instrument technology, most of which are based on the International Dairy Federation (IDF) standard method and powder detection instrument method. These methods can give accurate quantitative results, but often require complex sample preparation processes and long experimental operations. The indirect detection methods of milk powder mainly use microscopic imaging, spectral analysis, electronic nose system, environmental parameter monitoring and other technologies to establish complex mathematical models and provide a fast and non-destructive alternative. In addition, this paper summarizes the development of milk powder quality detection in three main directions: first, the traditional chemical detection method to environmental protection indirect analysis technology; Secondly, the development direction of multidisciplinary comprehensive evaluation; Finally, there is the wider use of artificial intelligence (AI) and automation. Future developments in the field are expected to focus on innovation across disciplines, combining technologies such as spectroscopy, high-definition microscopic imaging, digital twin with modern technologies such as AI and the Internet of Things. These advances are expected to improve the efficiency, sustainability and intelligence of milk powder quality assessment systems, while ensuring their accuracy and reliability.
Keywords: Milk powder, Quality detection, artificial intelligence, Rapid detection, spectrum, indirect detection
Received: 05 Feb 2025; Accepted: 05 Mar 2025.
Copyright: © 2025 Song, Shen, Dong, Ding, Xie, Wang and Cheng. This is an open-access article distributed under the terms of the Creative Commons Attribution License (CC BY). The use, distribution or reproduction in other forums is permitted, provided the original author(s) or licensor are credited and that the original publication in this journal is cited, in accordance with accepted academic practice. No use, distribution or reproduction is permitted which does not comply with these terms.
* Correspondence:
Haohan Ding, Jiangnan University, Wuxi, China
Disclaimer: All claims expressed in this article are solely those of the authors and do not necessarily represent those of their affiliated organizations, or those of the publisher, the editors and the reviewers. Any product that may be evaluated in this article or claim that may be made by its manufacturer is not guaranteed or endorsed by the publisher.
Research integrity at Frontiers
Learn more about the work of our research integrity team to safeguard the quality of each article we publish.