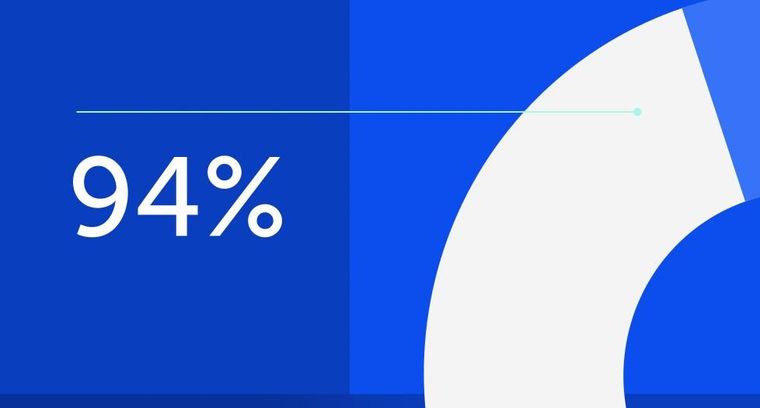
94% of researchers rate our articles as excellent or good
Learn more about the work of our research integrity team to safeguard the quality of each article we publish.
Find out more
ORIGINAL RESEARCH article
Front. Sustain. Food Syst., 15 April 2025
Sec. Agroecology and Ecosystem Services
Volume 9 - 2025 | https://doi.org/10.3389/fsufs.2025.1570678
This article is part of the Research TopicInnovative Approaches in Soil, Water, and Crop Management for Sustainable Agricultural SystemsView all 8 articles
Introduction: Promoting agricultural low-carbon transformation are essential for achieving the “dual carbon” targets and advancing high-quality development.
Methods: This study employed evolutionary game theory to construct a tripartite model involving local governments, agricultural enterprises, and consumers. It stimulated their decision-making processes and explored the mechanisms of the government and market. Furthermore, taking into account regional technological differences, this study examined the differentiated incentive effects of two mechanisms, and developed a comprehensive analytical framework for agricultural low-carbon transformation.
Results: (1) The model revealed three evolutionarily stable states, with the system evolving toward the optimal equilibrium of (1,1,1) under specific constraint conditions. (2) To achieve the system's Pareto optimality, the optimal intervals for subsidy coefficients and carbon emission penalties were (0.2, 0.25) and (1.5, 1.75), respectively. The optimal intervals for publicity coefficients and low-carbon consumption preferences were (0.3, 0.35) and (0.65, 0.7), respectively. (3) Considering regional technological differences, western regions with lower levels of low-carbon technology should prioritize government regulation, supplemented by market adjustment. Central regions should strike a balance between two methods, whereas eastern regions with more advanced low-carbon technologies should focus on market adjustment, with government regulation as a supplement.
Discussion: This study enhances the understanding of the mechanisms underlying the government and market, holding both theoretical and practical significance for advancing agricultural low-carbon transformation.
Since the reform and opening-up, China has achieved tremendous accomplishments in economic development. However, the extensive economic growth model has also given rise to severe environmental pollution issues, particularly climate change induced by carbon emissions, which poses a grave threat to the sustainable development of China's economy and society (Stocker, 2014; Hu et al., 2023). As a major agricultural country, agricultural production is an important source of carbon emissions, and deep involvement in the agricultural sector is essential for achieving low-carbon transformation (Tian and Chen, 2021). To this end, the Chinese government has introduced a series of policies to guide and support agriculture toward a low-carbon, environmentally friendly, and sustainable direction.1 Nevertheless, constrained by the current high-input, high-pollution, and extensive agricultural production methods, Chinese agricultural production remains highly dependent on energy, fertilizers, and other production factors, resulting in considerable pressure on carbon emission control and ineffective containment of environmental problems (Gao and Zhang, 2022).
The promotion of agricultural low-carbon transformation relies on the combined application of government policies and market mechanism (Sun P. et al., 2024). Theoretically, the “Porter Hypothesis” suggests that reasonable environmental policies can help drive technological innovation, achieving a win–win situation for economic development and environmental protection (Huang et al., 2022; Shao and Li, 2022; Hu et al., 2020; Porter, 1996). The government directly influences agricultural enterprises' decisions on emission reduction through multidimensional policy tools: Command-and-control policies set strict emission reduction targets and clear technical standards to limit enterprises' pollution emissions, forcing the withdrawal of backward production capacity (Lanoie et al., 2008; Zhang et al., 2011). Market-based environmental policies mainly provide economic incentives for enterprises' green innovation behaviors through market-oriented means, such as subsidies and taxes, internalizing their environmental costs (Lee et al., 2011). Voluntary environmental policies cultivate enterprises' environmental responsibility through mechanisms, such as information disclosure and environmental certification (Xu, 2020; 徐佳 and 崔静波, 2020). Meanwhile, market mechanisms play a pivotal role in promoting agricultural green transformation on the demand side. If the market is efficient, the potential improvement in agricultural product quality from low-carbon production will lead to price spillovers through the pricing mechanism, thereby providing economic incentives for low-carbon production (Stavins, 2010; Malkiel, 1989; Norse, 2012; Zhu and Tian, 2012).
The decision of agricultural enterprises to adopt low-carbon technologies is influenced by multiple stakeholders. As rule-makers and representatives of public interests, governments must balance the economic and environmental benefits of low-carbon transformation, and their regulatory decisions directly impact the costs of other entities. Consumers primarily influence the demand side, providing market momentum for low-carbon transformation through their consumption behaviors. Agricultural enterprises are the direct executors, with their production decisions influenced by regulatory policies, market risks, and consumer demand. Meanwhile, there is a interactive influence among the three parties: government promotion can reduce consumers' information acquisition costs, whereas green consumption preferences can enhance the marginal effectiveness of policy tools. Enterprises' green transformation, while saving energy and reducing emissions, also provides feasibility space for government regulatory policies. Essentially, agricultural low-carbon transformation operates as a closed-loop cycle of “policy driven—production response—market feedback (Norse, 2012).” Neglecting the role of government may lead enterprises into “race to the bottom” due to a lack of constraints. If consumers are overlooked, policy effects remain confined to the production side, making it difficult to verify their actual impact. Although other stakeholders are also involved in agricultural low-carbon transformation, their roles can be indirectly reflected through the actions of core entities. For instance, financial institutions' funding support can be embodied in policy tools such as green credit, while technology suppliers' support is internalized as adoption costs for enterprises. The key to resolving the current transformation dilemma lies in achieving incentive compatibility among the three parties through institutional innovation (He et al., 2021; Nyambuu and Semmler, 2020; Xu, 2022).
Based on these practical considerations, this study aimed to address the following questions:
• How do the decisions of local governments, agricultural enterprises, and consumers interact and affect the evolution of system stability?
• How do government regulation and market adjustment operate in agricultural low-carbon transformation?
• Given regional technological differences, how can we devise appropriate low-carbon development models for different regions?
Evolutionary game theory can provide a robust framework for analyzing behavior and strategic evolution within complex systems (Zhu and Tian, 2012). To address the aforementioned questions, this study adopted evolutionary game theory to analyze agricultural low-carbon transformation. It constructed an evolutionary game model that incorporates government regulation and market adjustment, analyzing possible equilibrium points, the stability conditions for the three stakeholders to reach equilibrium, and the sensitivity of key variables to explore the evolutionary dynamics of the system. Building on this, the study further analyzed the incentive effects of two mechanisms across different regions, and developed an analytical framework for agricultural low-carbon transformation.
Compared with existing literature, this study makes the following three contributions:
First, most existing literature focused on a two-party game between local governments and enterprises. This study extended the analysis by incorporating another key stakeholder—the consumer—and constructed a tripartite evolutionary game model, aiming to offer a more comprehensive perspective on promoting agricultural low-carbon transformation.
Second, existing literature often emphasizes either government regulation or market adjustment in isolation. This study constructed an analytical framework that integrates both mechanisms, comprehensively examining their synergistic effects and differentiated impacts.
Finally, this study considered regional technological differences and analyzed the incentive effects of government regulation and market adjustment. Based on these analyses, targeted policy recommendations are offered for each region.
The remainder of this paper is organized as follows: Section 2 presents the literature review. Sections 3 introduces the materials and methods. Section 4 presents the results. Section 5 further discusses the effects of the two mechanisms. Section 6 explains the conclusions and implications.
Low-carbon agriculture originated from the idea of a low-carbon economy, initially taking the form of low-carbon agricultural economics, which focuses on achieving maximum benefits from agricultural production and operations while minimizing greenhouse gas emissions (Wang, 2008). As research progressed, the scope of low-carbon agricultural economics expanded, leading to the concept of low-carbon agriculture—a comprehensive approach aimed at achieving low-carbon emissions throughout the entire food production process by fully leveraging agricultural carbon sequestration (Nyambuu and Semmler, 2020; Xu, 2022). Low-carbon agriculture represents the future direction of modern agricultural development, helping to address challenges, such as climate deterioration and resource scarcity (Xie et al., 2018). It is also an inevitable path toward realizing modern agriculture with Chinese characteristics and advancing agricultural ecological civilization (Wang and Yang, 2021). To transform agricultural production methods, the Chinese government has focused on the improvement of resource utilization efficiency and ecological protection, actively implementing ecological compensation and incentive policies (Wang et al., 2010), promoting and innovating low-carbon agricultural production technologies, and developing a low-carbon agricultural technology system centered on five core techniques: no-tillage and reduced tillage, deep tillage and loosening, pest control, organic fertilization, and straw return (Zheng et al., 2022). While low-carbon agriculture shows promising prospects and has achieved initial success, it still faces several challenges and obstacles. On the one hand, current incentive policies tend to benefit only large-scale agricultural enterprises, whereas small and medium-sized enterprises face considerable liquidity constraints during the initial stages of transformation (Luo and Xu, 2010). On the other hand, insufficient consumer awareness of low-carbon consumption, coupled with high market risks for low-carbon agricultural products, leads to considerable instability in profit expectations for operators, thereby discouraging the adoption of low-carbon technologies (Freibauer et al., 2004).
In summary, existing research has extensively investigated the origins, developmental significance, and practical challenges of low-carbon agriculture but lacks a systematic analytical framework that incorporates multiple stakeholders. It has also neglected a thorough analysis of the conflicts of interest among stakeholders and the evolution of their behaviors, leading to an incomplete understanding of the complex interactive mechanisms involved in agricultural low-carbon transformation.
This study mainly examined the interactions among local governments, agricultural enterprises, and consumers. Building on existing literature, we specifically analyzed the impact of individual characteristics, government regulation, and market adjustment on agricultural low-carbon transformation.
Existing studies have predominantly focused on the adoption of low-carbon technologies from the perspective of farmers. Subjective factors such as farmers' gender, age, and educational level significantly influence their willingness to adopt these technologies (Li and Wang, 2010; Zhang et al., 2024; Yang et al., 2020). Household endowments, including income level and the proportion of agricultural income, also affect farmers' willingness to adopt low-carbon technologies (Barham et al., 2011). Low income and a small proportion of agricultural income reduce farmers' demand for low-carbon technologies. With the research advancement, some scholars have explored the impact of psychological factors, such as risk perception and cognitive norms, on farmers' decision-making from the perspective of behavioral economics. For instance, risk aversion leads farmers to prefer traditional production methods that are familiar and provide stable returns (Schulte et al., 2022), whereas attitudes, cognitive norms, and perceived benefits significantly positively influence farmers' willingness to adopt low-carbon technologies (Zhao and Zhou, 2021).
Both the government and market are key drivers of agricultural low-carbon transformation. Scholars generally agree that reasonable environmental regulatory policies contribute to the promotion of agricultural low-carbon transformation. Policy tools, such as carbon taxes and carbon emission caps, can effectively increase the cost of carbon emissions and guide agricultural producers to adopt low-carbon technologies (Zheng et al., 2022; Stewart, 2022). Concurrently, the effectiveness of environmental regulation not only depends on the enforcement efforts of regulatory agencies but is also closely related to farmers' environmental awareness (Hui et al., 2023; Ren and Zhong, 2022). In developed countries, such as Germany and the Netherlands, the combination of environmental regulatory policies with publicity and education has promoted the widespread application of low-carbon technologies. However, some scholars pointed out that excessive restrictions in environmental regulation may have a negative impact on low-carbon agriculture and, in some cases, may even sacrifice certain economic growth (Luo et al., 2024). With economic development and the upgrading of consumption concepts, the role of market mechanisms in the development of low-carbon agriculture has gradually gained attention. Numerous studies have proved that market incentive mechanisms, such as carbon trading markets and low-carbon subsidies, have become important tools for promoting agricultural low-carbon transformation (Zheng et al., 2022; Ke and Huang, 2024). There is limited research in China on the application of carbon markets, but some scholars have begun to focus on the potential of carbon trading for agricultural emission reductions. By establishing agricultural carbon trading platforms, the ecological benefits of low-carbon agriculture can be effectively converted into economic benefits (Guo et al., 2022). In addition to the direct effects of governments and market mechanisms, the synergistic effect between them is also noteworthy. Studies have reported that the optimal combination of environmental regulation and market incentives can effectively balance low-carbon goals and economic benefits (Zheng et al., 2022). Single environmental regulation may be constrained by cost pressures, whereas the introduction of market mechanisms can effectively alleviate this issue. Changes in market demand are also among the driving forces for low-carbon agriculture. From a neoclassical economic perspective, the key to agricultural low-carbon transformation lies in the recognition of the market value of low-carbon agricultural products (Sneeringer, 2009). With the increase in consumers' income levels, heightened environmental awareness, and concerns regarding food safety, the demand for low-carbon agricultural products in China has significantly increased (Lyu et al., 2020), and consumers are more willing to pay for low-carbon development, thereby driving agricultural enterprises to adopt low-carbon technologies from the demand side (Norse, 2012; Zhang et al., 2024; Hou and Hou, 2019; Wang and Wang, 2010; Jin et al., 2020; Echeverría et al., 2014; Chen et al., 2018; Kong et al., 2017; Zhang and Xu, 2022).
Existing literature mainly focuses on the adoption of low-carbon technologies from the perspective of farmers, with limited attention paid to business entities, such as agricultural enterprises. In contrast to farmers, agricultural enterprises benefit from advantages in intensification, specialization, organization, and socialization, enabling them to efficiently allocate production factors and thereby significantly influence agricultural carbon emissions (Wang and Wang, 2010). In the context of large-scale and intensive operations, discussing the low-carbon transformation of agricultural enterprises is more practically significant. Furthermore, although the aforementioned studies have investigated the impact of government regulation and market adjustment on agricultural low-carbon transformation, there remains a lack of comprehensive exploration that considers the interactions and coupling effects of various factors. In addition, much of the related research adopted a static perspective using qualitative and descriptive statistical methods to study low-carbon agriculture, overlooking the long-term and dynamic nature of the low-carbon transformation (Xie et al., 2018; Zhao et al., 2024; Coninx et al., 2018; Sun P. et al., 2024; Feng and Ge, 2024; Liu et al., 2022; He and Liao, 2024; Hu and Wang, 2022).
In summary, existing literature mainly discusses the origins, significance, development challenges, and influencing factors of low-carbon agriculture. It examines the impact of government regulation and market adjustment on agricultural low-carbon transformation from a singular perspective. However, there is a lack of a comprehensive analysis involving multiple stakeholders, and the roles of the government and market, along with their dynamic effects on low-carbon agriculture, have not been fully elucidated. To bridge this gap, this study constructed an evolutionary game model to systematically describe the strategic evolution process of local governments, agricultural enterprises, and consumers. It offers a differentiated analysis of the incentive effects of government regulation and market adjustment, providing a more comprehensive and dynamic perspective for advancing low-carbon agriculture.
Traditional game theory focuses on static equilibrium analysis, assuming that all participants make instantaneous decisions based on complete information. However, such static models often overlook the dynamic nature of decision-making and the constantly evolving interactions among participants. This is particularly relevant in complex systems, where decision-makers frequently face incomplete information and bounded rationality. Evolutionary game theory is a generalized approach for studying agent interactions and strategic decisions. It develops an evolutionary game model based on replicator dynamics and evolutionarily stable states, which represent the stable states of evolutionary games and the process of dynamically converging to these states, respectively (Jin et al., 2020). Compared with the static equilibrium of traditional game theory, evolutionary game theory addresses the issues of bounded rationality and learning mechanisms. By incorporating replicator dynamic equations and evolutionarily stable states, it captures the strategic evolution of game participants over long-term interactions. As a result, it serves as a powerful tool for analyzing individual behavior and strategy evolution in complex systems.
Agricultural low-carbon transformation is critical for achieving the coordinated development of the economy and environment, necessitating cooperation among local governments, agricultural enterprises, and consumers. Local governments facilitate low-carbon transformation by implementing ecological compensation systems, reward and penalty mechanisms, as well as policy promotion. However, the implementation of these policies often incurs substantial regulatory costs. When the outcomes of incentive policies fail to meet expectations, local governments may resort to passive regulation, which can undermine their credibility and, consequently, affect policy effectiveness and social stability. For agricultural enterprises, low-carbon agricultural products are theoretically more valuable in the market, and leveraging policy incentives for transformation can yield greater economic benefits. However, enterprises often face liquidity constraints and uncertainty regarding whether consumers will recognize the market value of low-carbon products. They need to make production decisions by considering both the policy environment and market demand. Consumers, may perceive additional benefits in low-carbon agricultural products, but these benefits are offset by higher purchasing costs. In addition to the aforementioned endogenous factors, external factors, such as low-carbon technology level, considerably influence the evolution of the system.
The information asymmetry and interest misalignment among local governments, agricultural enterprises, and consumers complicate their interactions. As representatives of public interests, local governments must balance environmental protection and economic growth, with their core regulatory goal being to maximize social welfare under limited resource conditions. Agricultural enterprises, as the direct executors of low-carbon transformation, pursue profit maximization, but there is a contradiction between the long-term benefits of low-carbon production and short-term investment costs. Consumers, acting on the demand side, reshape the value system of agricultural products through their purchasing behavior. However, due to information asymmetry, they struggle to directly assess the quality of agricultural products, making their choices susceptible to bias and potentially leading to a “lemon market” problem. The interest misalignment among the three parties easily results in behavioral conflicts, such as insufficient subsidies prompting enterprises to engage in strategic emission reductions, rigid policies hindering low-carbon transformation, and distorted market signals causing consumer trust crises. Any deviation from equilibrium by one party can disrupt the entire system. Given bounded rationality, local governments, agricultural enterprises, and consumers are unlikely to find an optimal strategy in a single game. Instead, they must adjust their decisions based on past experiences and environmental changes, iterating through multiple rounds of interaction to gradually identify the optimal strategy. The process of finding the optimal strategy involves multi-agent, multi-level interactions, aligning with the analytical framework of evolutionary game theory. Based on this, this paper constructed an evolutionary game theoretical framework for analyzing agricultural low-carbon transformation, as shown in Table 1.
Table 1. Evolutionary game-theoretic analysis framework for Low carbon transformation of agriculture.
The principles of evolutionary game theory encompass three key dimensions: bounded rationality, differentiated strategies, and group dynamic adjustment. First, bounded rationality suggests that participants cannot fully predict the consequences of all strategies, and their decisions are based on experiential imitation, local information, or simple rules. Second, differentiated strategies emphasize that variations in individual characteristics lead to multidimensional strategy diversification. Finally, group dynamic adjustment reflects the evolutionary process of strategies through a “trial-error-learning-diffusion” mechanism, where high-reward strategies gradually increase in proportion within the group, while low-reward strategies are progressively eliminated. These three dimensions reveal the evolutionary logic of evolutionary game theory, from individual bounded rationality to an evolutionarily stable state. When constructing an evolutionary game model, it is essential to follow these principles and extract key parameters based on real-world contexts, thereby simulating the behavioral logic and interaction mechanisms of participants. Therefore, this paper proposes the following assumptions:
Assumption 1 (rational participant assumption). One of the central principles of evolutionary game theory is that agents participate in repeated games under the assumption of bounded rationality until the game system evolves toward a stable state over time (Li). This model involves three primary stakeholders: local governments, agricultural enterprises, and consumers. Each of these stakeholders operates under bounded rationality, continuously learning from one another throughout the game process to make decisions that best align with the evolving environment and their own interests.
Assumption 2 (strategy selection assumption). For ease of analysis, scholars typically employ binary strategies to analyze agents' strategies (Zhao et al., 2024; Sun Y. et al., 2024). Specifically, the strategy space for local governments is (active regulation, passive regulation) = (x, 1 − x), the strategy space for agricultural enterprises is (low-carbon production, traditional production) = (y, 1 − y), and the strategy space for consumers is (low-carbon consumption, traditional consumption) = (z, 1 − z).
Assumption 3 (government behavior assumption). When local governments opt for active regulation, they must implement a series of incentive policies to guide agricultural enterprises and consumers toward low-carbon transformation. Specifically, local governments provide low-carbon production subsidies, denoted as αCl (where α denotes the subsidy coefficient and Cl denotes the cost of low-carbon technologies), to agricultural enterprises while imposing carbon penalties, denoted as P, on enterprises that exceed carbon emission thresholds. In addition, local governments seek to increase consumer demand for low-carbon products through policy promotion, with the promotion cost represented as (1 + β)Cp (where β denotes the promotion coefficient and Cp denotes the promotion cost). Moreover, local governments must cover air pollution control costs, denoted as Ca, to mitigate excessive carbon emissions from traditional production methods. In contrast, when local governments adopt passive regulation, they incur a credibility loss, denoted as L, which exceeds the costs associated with active regulation (Mei et al., 2024; Sun P. et al., 2024), i.e., L > αCt + (1 + β)Cp + Ca.
Assumption 4 (agricultural enterprise behavior assumption). The prices and sales volumes of traditional agricultural products and low-carbon agricultural products are denoted as Pt, Dt and Pl, Dl, respectively, with production costs represented by Ct and Cl. Government promotion can enhance consumer awareness, thereby increasing the sales of low-carbon agricultural products. Consequently, the revenues of agricultural enterprises from traditional production and low-carbon production are given by Pt ∗ Dt and λ ∗ Pl ∗ (1 + β) ∗Dl, respectively, where λ denotes low-carbon consumption preference. However, the information asymmetry between agricultural enterprises and consumers can lead to adverse selection, thereby increasing the market risks (Feng and Ge, 2024; Liu et al., 2022). The risk cost is denoted as Cm. In addition, our field research found that the application for low-carbon subsidies is cyclical, and agricultural enterprises that transform earlier can occupy a larger market share owing to first-mover advantages (Kerin et al., 1992). Many enterprises regret missing the opportunity, so opportunity cost Co is introduced to describe this aspect.
Assumption 5 (consumers' behavior assumption). Low-carbon consumption can provide consumers with additional perceived benefits, such as health and environmental benefits as well as social reputation, with these perceived benefits being linked to government promotion, denoted as (1 + β)U. However, a mismatch between supply and demand may arise: when agricultural enterprises engage in low-carbon production but consumers opt for traditional consumption, consumers miss out on the additional benefits, resulting in an opportunity cost, denoted as V. Conversely, when agricultural enterprises choose traditional production while consumers lean toward green consumption, consumers incur a search cost, denoted as Cs.
Assumption 6 (external driver assumption). The maturity of low-carbon technology considerably influences the cost structure of agricultural enterprises, making it a critical external factor in their decision-making. Consequently, this study introduces the concept of the low-carbon technology level (d) to quantify the impact of technological maturity on the strategic choices of agricultural enterprises.
The diagram of the variable relationships is presented in Figure 1.
Based on the above assumptions, the payoff matrix for local governments, agricultural enterprises, and consumers is presented in Table 2.
Let the expected payoffs for local governments choosing active regulation and passive regulation be E11 and E12, respectively, with the average payoff denoted as . Then:
Let the expected payoffs for agricultural enterprises choosing low-carbon production and traditional production be E21 and E22, respectively, with the average payoff denoted as . Then:
Let the expected payoffs for consumers choosing low-carbon consumption and traditional consumption be E31 and E32, respectively, with the average payoff denoted as . Then:
The replication dynamic equations for local governments, agricultural enterprises, and customers are as follows:
Let:
According to the stability conditions of the replicator dynamic equation, when F(x) = 0 and F′(x) < 0, the point is an evolutionary stable point for local governments. When , G(y) = 0, and for any value of x, F(x) ≡ 0. At this point, the strategy choice of local governments does not change over time, and the stable strategy is any arbitrary strategy. When , let F(x) = 0, and we get two possible equilibrium solutions, x1 = 0 and x2 = 1.
(1) When , G(y) < 0, , and . In this case, x1 = 0 is the evolutionary stable strategy for local governments.
(2) When , and , G(y) > 0, , and . In this case, x2 = 1 is the evolutionary stable strategy for local governments.
The evolutionary dynamic phase diagram of local governments is illustrated in Figure 2.
Let:
According to the stability conditions of the replicator dynamic equation, when F(y) = 0 and F′(y) < 0, the point is an evolutionary stable point for agricultural enterprisers. When , G(z) = 0, and for any value of y, F(y) ≡ 0. At this point, the strategy choice of agricultural enterprisers does not change over time, and the stable strategy is any arbitrary strategy. When , let F(y) = 0, and we get two possible equilibrium solutions, y1 = 0 and y2 = 1.
(1) When , and , G(z) < 0, , and . In this case, y1 = 0 is the evolutionary stable strategy for agricultural enterprisers.
(2) When , G(z) > 0, , and . In this case, y2 = 1 is the evolutionary stable strategy for agricultural enterprisers.
The evolutionary dynamic phase diagram of agricultural enterprisers is illustrated in Figure 3.
Let:
According to the stability conditions of the replicator dynamic equation, when F(z) = 0 and F′(z) < 0, the point is an evolutionary stable point for consumers. When , G(x) = 0, and for any value of z, F(z) ≡ 0. At this point, the strategy choice of consumers does not change over time, and the stable strategy is any arbitrary strategy. When , let F(z) = 0, and we get two possible equilibrium solutions, z1 = 0 and z2 = 1.
(1) When , and , G(x) < 0, , and . In this case, z1 = 0 is the evolutionary stable strategy for consumers.
(2) When , G(x) > 0, , and . In this case, z2 = 1 is the evolutionary stable strategy for consumers.
The evolutionary dynamic phase diagram of consumers is illustrated in Figure 4.
When local governments, agricultural enterprises, and consumers engage in repeated games and no longer alter their strategies, the strategy combinations of all agents converge to an evolutionarily stable state (ESS). To identify the local stationary points and system stable points in the dynamic system, set Equation (10) equal to zero, which indicates that the strategies of the agents no longer change over time, and each participant's choice represents the optimal strategy. At this point, we obtain nine local equilibrium points: E1(0, 0, 0), E2(1, 0, 0), E3(0, 1, 0), E4(0, 0, 1), E5(1, 1, 0), E6(1, 0, 1), E7(0, 1, 1), E8(1, 1, 1), and .
The equilibrium point can be called an ESS if and only if it is a strict Nash equilibrium and a pure strategy Nash equilibrium (He and Liao, 2024; Hu and Wang, 2022). Therefore, the mixed strategy E9 is not a stable strategy. To determine the ESSs, the partial derivatives of Equation (10) with respect to x, y, and z are solved to obtain the Jacobian matrix. The eigenvalues of the local stationary points are presented in Table 3.
According to Lyapunov's stability theory, an equilibrium point can be considered an ESS of the entire system only if all its eigenvalues are negative. Consequently,E1(0, 0, 0), E3(0, 1, 0), E4(0, 0, 1), E6(1, 0, 1), and E7(0, 1, 1) cannot be ESS. The possible ESSs for the model include E2(1, 0, 0), E5(1, 1, 0), and E8(1, 1, 1). Considering the real situation of low-carbon transformation of Chinese agriculture, these three equilibrium points reflect three typical evolutionary processes of agricultural carbon reduction to some extent. Therefore, this study will discuss these three scenarios in detail; the system evolution process is illustrated in Figure 5.
Ct − (1 − α)(1 − d)Cl − Cm + P + Co < 0. The constraints of the initial stage indicate that under active regulation, the cost of low-carbon production for agricultural enterprises is higher than that of traditional production, leading them to prefer traditional production. The market is dominated by traditional agricultural products, and consumers face high search costs, making them inclined toward traditional consumption. At this point, the system evolves toward (1, 0, 0).
Climate change caused by excessive greenhouse gas emissions has already posed a considerable threat to agricultural development. Without appropriate measures, Chinese agriculture may face the risk of reduced yields or even food shortage (Weibull, 1997). As the main institutional provider, the government plays an important guiding role in developing low-carbon agriculture through ecological compensation reward and penalty systems as well as policy promotion. However, due to the immaturity of low-carbon agricultural production technologies and consumers' reluctance to pay for low-carbon agricultural products affected by factors, such as income levels and consumption concepts, the cost of low-carbon production remains high, leading agricultural enterprises to resist the adoption of low-carbon technologies. Moreover, the limited market share of low-carbon agricultural products further diminishes consumers' willingness to engage in low-carbon consumption. Therefore, this stage corresponds to E2(1, 0, 0), in which local governments choose active regulation, but agricultural enterprises and consumers do not opt for low-carbon transformation. It is noteworthy that although (1, 0, 0) represents an evolutionarily stable state in theory, if incentive policies fail to achieve the expected results, it may lead local governments to choose passive regulation or even withdraw from regulation owing to financial burden, which would significantly hinder the process of agricultural low-carbon transformation.
(1 − α)(1 − d)Cl + Cm − Ct − Co − P < 0, (1 + β)U − λPl(1 + β)Dl + V + PtDt < 0. The constraints of the transitional stage indicate that under active regulation, the cost of low-carbon production for agricultural enterprises is lower than that of traditional production, making them inclined toward low-carbon production. However, the benefits of low-carbon consumption for consumers are relatively low, making them inclined toward traditional consumption. Therefore, the system evolves toward (1, 1, 0).
As agricultural low-carbon production technologies mature, agricultural enterprises are generally able to achieve low-carbon transformation through government subsidies. Concurrently, the government actively promotes the health benefits and environmental value of low-carbon agricultural products. However, as the transformation in consumer attitudes takes a relatively long time, consumers are still unwilling to pay for low-carbon products. At this stage, government subsidies directly increase the disposable income of agricultural enterprises, providing a more immediate incentive effect compared with policy promotion. Therefore, this stage corresponds to E5(1, 1, 0), in which local governments choose active regulation, agricultural enterprises choose low-carbon production, and consumers choose traditional consumption. However, subsidy policies may increase the financial burden (Xing et al., 2023) and lead to “subsidy dependency,” resulting in the awkward situation of “using when subsidies are available and stopping when they are not” (Zhang and Wang, 2022; Sun et al., 2023; Zhang, 2022). Solely relying on government regulation without market adjustment makes it difficult to truly achieve agricultural low-carbon transformation.
PtDt + Cm + (1 − α)(1 − d)Cl − λPl(1 + β)Dl − Co − P − Ct < 0, λPl(1 + β)Dl − PtDt − V − (1 + β)U < 0. The constraints of the mature stage indicate that under active regulation, the net income from low-carbon production is higher than that from traditional production, and consumers have fully recognized the health benefits and environmental value of low-carbon agricultural products. Consequently, agricultural enterprises and consumers are inclined toward low-carbon transformation, leading the system to evolve toward (1, 1, 1).
Long-term policy promotion has significantly improved consumption attitudes, ensuring the economic benefits of low-carbon production for agricultural enterprises. The agricultural low-carbon transformation no longer depends solely on government subsidies, with market mechanisms now serving as an important incentive. Financial burdens are reduced, and resource allocation is further optimized, fostering the sustainable development of agricultural production and the environment. Under the combined influence of government regulation and market adjustment, the three core stakeholders achieve mutual benefits and a win–win situation. Therefore, this stage corresponds to E8(1, 1, 1), where local governments choose active regulation and both agricultural enterprises and consumers choose low-carbon transformation.
The data source and parameter calibration in this study are mainly based on the following principles:
(1) Based on field research conducted by our team on multiple agricultural enterprises in Zhejiang Province. The rich survey data provide valuable insights into key factors, such as market information, consumer preferences, and risk expectations during the modeling process. Our study found that the market price of low-carbon agricultural products is approximately four times that of traditional agricultural products, whereas the sales volume is < 50% of that of traditional products. To minimize data bias, we surveyed 92 agricultural enterprises and conducted in-depth interviews with their leaders regarding critical information, including product prices, sales volumes, and production costs. In addition, by combining these findings with publicly available data from the “Compilation of National Agricultural Product Cost and Income Data” and agricultural product information platforms (Li and Ying, 2004), we calculated the prices of traditional and low-carbon agricultural products to be 0.004 and 0.016, respectively, with corresponding sales volumes of 2,250 and 1,000. The production costs for traditional and low-carbon products are 4 and 14, respectively. In addition, we conducted a survey on 105 consumers using a questionnaire, and 85 of them preferred low-carbon consumption. Based on this, we assumed that the low-carbon consumption preference is 0.8.
(2) Based on public documents, such as policies and government reports. According to relevant policy documents, the low-carbon subsidy ratio generally ranges between 10% and 30%; therefore, the subsidy coefficient in this study was set to α = 0.3. To determine the parameters related to government regulatory costs, we mainly referred to the annual report information disclosed by local governments in various regions of Zhejiang Province. After simplification, we determined the promotion cost to be 3; initial value of promotion coefficient, 0.5; and air pollution control cost, 1. Moreover, following the methodology of Wickramarathne, Premaratne (Akerlof, 1970), the loss of credibility can be roughly estimated by the increase in annual administrative management cost for regional agriculture. Based on annual reports from different regions of Zhejiang Province, we calculated the loss of credibility to be 7.5.
(3) Based on previous literature. Due to the inherently vulnerable nature of agricultural production, the intensity of carbon emission penalties is generally lower than low-carbon production subsidies (Kerin et al., 1992). Following the method of Moraga-González, Sándor (Ritzberger and Weibull, 1995), consumers' search cost can be simply considered as the employment cost of workers within a certain period of time. Based on the above analysis, this study sets the carbon emission penalty and consumers' search cost as 2 and 1.5, respectively.
(4) Based on expert opinions. For controversial data, such as opportunity cost and perceived utility of low-carbon agricultural products, we consulted 10 experts from universities, research institutions, and government departments to determine the corresponding parameters.
The specific parameter settings are illustrated in Table 4.
To further validate the precision of the model analysis and probe into the impact of government regulation and market adjustment on agricultural low-carbon transformation alongside its sensitivity, MATLAB simulation was employed (China Statistics Press, 2021; Wickramarathne et al., 2012; Gu, 2014; Moraga-González et al., 2013).
Based on the stability analysis in Section 3.5, this model has three possible ESSs: E2(1, 0, 0), E5(1, 1, 0), and E8(1, 1, 1). However, owing to the considerable instability of E2(1, 0, 0) and E5(1, 1, 0), this study took E8(1, 1, 1) as the final target state. Using the constraints of (1, 1, 1), we conducted sensitivity analysis on key parameters, including the subsidy coefficient, promotion coefficient, carbon emission penalties, and low-carbon consumption preference.
The initial probability settings for this study are as follows: As the primary institutional provider, local governments are responsible for implementing central policies and actively introducing relevant measures to guide agricultural enterprises and consumers toward low-carbon transformation. The willingness of local government is set as x = 0.5. In addition, based on our research findings, low-carbon production technologies are predominantly adopted by large enterprises with strong financial resources, whereas small and medium-sized enterprises mainly rely on traditional production methods. In a market dominated by traditional agricultural products, most consumers tend to prefer traditional consumption. Therefore, the willingness of agricultural enterprises and consumers to choose low-carbon transformation is set at y = z = 0.3. The simulation period is set as t = 10.
The subsidy coefficient, denoted by α, has a value range of [0, 1]. To explore under what conditions the subsidy coefficient achieves system Pareto optimality, this section first sets α = 0, 0.1, 0.3, 0.5, 0.7, where α = 0 indicates no subsidy, keeping other parameters unchanged. The simulation results are illustrated in Figures 6a–c. According to the results, the optimal subsidy coefficient for local governments lies within the range of [0.1, 0.3].
Figure 6. The impact of subsidy coefficient on system evolution. (a–c) Represent the impact of subsidies on local governments, agricultural enterprises, and farmers, respectively. (d–f) Represent the influence of subsidies on these entities after narrowing the range.
When α ≤ 0.1, local governments maintain high willingness to subsidize, but the willingness of agricultural enterprises to adopt low-carbon production and consumers to engage in low-carbon consumption remains at 0. For agricultural enterprises, owing to the inherent vulnerability of agricultural production and the uncertainty of expected returns, low-carbon transformation requires considerable financial subsidies to offset market operational costs (Selten and Selten, 1988). During this period, the market is dominated by traditional agricultural products, and consumers tend to choose traditional consumption to avoid search cost (Zheng, 2001; Geng et al., 2022). When 0.3 ≤ α < 0.7, the willingness of agricultural enterprises and consumers for low-carbon transformation markedly increases, but the willingness of local governments to subsidize begins to decline. This is because although increased subsidies ease liquidity constraints for agricultural enterprises, they also impose heavier financial burden on local governments (Matsuo and Schmidt, 2017). When α ≥ 0.7, the financial pressure on local governments further intensifies, resulting in fluctuations in regulatory willingness. Even if consumers are inclined to choose low-carbon consumption, the willingness of agricultural enterprises for low-carbon production becomes unstable due to the insufficient subsidies provided.
To further refine the optimal range for the subsidy coefficient, this study adjusts the step size to 0.05 within the interval [0.1, 0.3], ensuring that the conclusion falls within a reasonable margin of error of 5%. The simulation results are presented in Figures 6d–f. Based on the results, when the subsidy coefficient α ∈ (0.2, 0.25), the system achieves Pareto optimality.
The intensity of promotion can be quantified as the ratio of actual promotion expenditure to the promotion budget, with its value ranging between [0, 1]. To determine under what conditions the promotion coefficient causes the system to evolve into the optimal state, this study initially assigned values to the promotion coefficient within the range, with a step size of 0.2, and kept other parameters unchanged. The simulation results are illustrated in Figures 7a–c.
Figure 7. The impact of promotion coefficient on system evolution. (a–c) Represent the impact of promotion on local governments, agricultural enterprises, and farmers, respectively. (d–f) Represent the influence of promotion on these entities after narrowing the range.
According to the results, when β ≤ 0.3, local governments maintain high willingness to promote, but the willingness of agricultural enterprises to adopt low-carbon production and the consumers to engage in low-carbon consumption remains at 0. On the one hand, in the absence of a low-carbon consumption mindset, consumers find it difficult to perceive the additional benefits of low-carbon agricultural products. Moreover, the market price of low-carbon agricultural products is considerably higher than that of conventional products (Cai and Wang, 2023), making high prices a major obstacle to low-carbon consumption. On the other hand, as the market value of low-carbon agricultural products is not recognized by consumers, agricultural enterprises cannot obtain market returns and therefore prefer the more stable traditional production mode. When 0.5 ≤ β < 0.9, the willingness of agricultural enterprises and consumers for low-carbon transformation increases with the promotion coefficient. This is because a social consensus on low-carbon consumption has largely formed, and consumers are willing to pay for the health benefits and environmental value of low-carbon agricultural products, ensuring the production benefits of agricultural enterprises. Further analysis of the simulation results revealed that as promotion coefficient increases, the marginal effect of government promotion on incentivizing agricultural enterprises and consumers gradually decreases, and when β ≥ 0.9, the government's willingness declines due to excessively high costs. Therefore, there is an optimal range for promotion coefficient, which lies between 0.3 and 0.5.
To further refine the optimal range for promotion coefficient, this study adjusts the step size to 0.05 within the interval [0.3, 0.5], ensuring that the conclusion falls within a reasonable margin of error of 5%. The simulation results are presented in Figures 7d–f. According to the results, when the promotion coefficient β ∈ (0.3, 0.35), the system achieves Pareto optimality.
To improve the ecological compensation system for carbon emissions, in recent years, the Chinese government has actively performed pilot reforms of the reward and penalty mechanism, transitioning from a single ecological subsidy to a management model that combines rewards and penalties.2 To explore the impact of carbon emission penalties on system evolution, this section scales the baseline value of carbon emission penalties (P = 2) by certain proportions (P = 0, 1, 2, 3, 4) while keeping other parameters unchanged. The simulation results are illustrated in Figures 8a–c. According to the results, the optimal range for carbon emission penalties lies between (Stocker, 2014; Hu et al., 2023).
Figure 8. The impact of penalties for exceeding carbon emissions on system evolution. (a–c) Represent the impact of penalties on local governments, agricultural enterprises, and farmers, respectively. (d–f) represent the influence of penalties on these entities after narrowing the range.
For local governments, imposing carbon emission penalties does not lead to increased regulatory costs; thus, they consistently maintain high willingness for regulation. However, as the penalty intensity increases, the speed of local governments evolving to a state of 1 decreases. The possible reason is that due to the dispersed and vulnerable nature of agricultural production, high-intensity penalties may increase the survival pressure on small and medium-sized agricultural enterprises (Zhang and Luo, 2022; Acs et al., 1997). For large enterprises, they may transfer penalty costs to consumers by concealing information or raising product prices (China Statistics Press, 2021; Yang and Xu, 2016), which increases the difficulty of regulation for local governments to some extent. For agricultural enterprises, when P ≤ 1, they do not need to bear the high cost of carbon emission control (Wickramarathne et al., 2012) and therefore tend to prefer traditional production methods to secure stable returns and avoid the high investment associated with low-carbon production. When P ≥ 2, the penalty mechanism raises production costs for agricultural enterprises, forcing high-emission, inefficient agricultural enterprises to either exit the market or undergo low-carbon transformation to avoid carbon emission penalties (Chen et al., 2023). At this point, the market is dominated by low-carbon agricultural products, and consumers tend to prefer low-carbon consumption.
To further refine the optimal range for carbon emission penalties, this study adjusts the step size to 0.25 within the interval (Stocker, 2014; Hu et al., 2023). The simulation results are illustrated in Figures 8d–f. According to the results, when the carbon emission penalty P ∈ (1.5, 1.75), the system achieves Pareto optimality.
Low-carbon consumption preference can be simply expressed as the proportion of consumers who choose low-carbon consumption among all consumers, with its value ranging between [0, 1]. To explore under what conditions low-carbon consumption preference causes the system to evolve into the optimal state, this study initially assigned values to low-carbon consumption preference within the range, with a step size of 0.2, and kept other parameters unchanged. The simulation results are illustrated in Figures 9a–c. According to the results, low-carbon consumption preference has no considerable effect on the strategy evolution of local governments, but it significantly affects the decisions of agricultural enterprises and consumers.
Figure 9. The impact of green consumption preference on system evolution. (a–c) Represent the impact of consumption preference on local governments, agricultural enterprises, and farmers, respectively. (d–f) Represent the influence of consumption preference on these entities after narrowing the range.
The willingness of agricultural enterprises for low-carbon production is positively correlated with low-carbon consumption preference. Within the interval [0.6, 0.8], the willingness shifts from 0 to 1. However, for consumers, the positive stimulation of low-carbon consumption preference turns into negative stimulation within the interval [0.8, 1]. Low-carbon consumption preference is an essential psychological factor for consumption upgrading and an important market driver for the low-carbon transformation of agricultural enterprises. On the one hand, when the low-carbon consumption preference is too low, the market returns for agricultural enterprises cannot be guaranteed. On the other hand, under limited market resource conditions, excessively high consumption preferences can easily lead to malicious consumer behavior, such as price gouging (Zwolinski, 2008). Therefore, the optimal range for low-carbon consumption preference is [0.6, 0.8].
To further refine the optimal range for low-carbon consumption preference, this study adjusts the step size to 0.05 within the interval [0.6, 0.8], ensuring that the conclusion falls within a reasonable margin of error of 5%. The simulation results are presented in Figures 9d–f. According to the results, when the low-carbon consumption preference λ ∈ (0.65, 0.7), the system achieves Pareto optimality.
The sensitivity analysis in Section 4 revealed that changes in government and market parameters can influence the production decisions of agricultural enterprises. However, considering the significant regional technology differences in China, technology levels also have an important impact on low-carbon transformation (Huang et al., 2024). To further analyze the effects of government regulation and market adjustment on low-carbon transformation in different regions, this section alters the low-carbon technology level (d) to simulate different regional technology environments. Accordingly, the relevant parameters are adjusted.
Considering the regional development realities in China, low-carbon agricultural production technologies are more advanced in the eastern region, followed by the central region, whereas the western region lags behind. Therefore, let d = 0.3, 0.5, 0.7 represent the three major regions of western, central, and eastern China, respectively. On this basis, the subsidy coefficient (α) and promotion coefficient (β) are adjusted, and policy combinations are constructed based on the complementary principle3 to analyze the differentiated effects of various policy combinations on the agricultural low-carbon transformation. The simulation results are presented in Figure 10.
According to the results, when the low-carbon technology level is low (d = 0.3), relying on market adjustment alone cannot achieve low-carbon transformation in agricultural production, and government subsidies are the main driving factor. As low-carbon technologies mature (d = 0.5), the marginal utility of subsidy policies weakens, and market adjustment mechanisms begin to take effect. In the eastern region, where low-carbon agricultural production technologies are most advanced (d = 0.7), the willingness of agricultural enterprises to engage in low-carbon production rapidly rises under all policy combinations, with almost the same rate of change. This suggests that at higher levels of technology, the influence of government regulation and market adjustment weakens, and the system possesses a form of “automatic stability” (Sahin, 2006).
Given that government regulation and market adjustment have different effects at different stages, promoting the agricultural low-carbon transformation requires the formulation of differentiated policy combinations tailored to actual development needs. On the one hand, low-carbon agriculture generates considerable positive externalities. While the environmental benefits it provides can maximize social welfare, they do not directly translate into increased enterprise income. The transformation cost for enterprises may far exceed the direct benefits they can obtain (Lin and Xu, 2019), leading agricultural enterprises to not voluntarily adopt low-carbon production technologies in the absence of external incentives. Given the relatively low level of agricultural low-carbon technology, even with government promotion encouraging consumer preference for low-carbon agricultural products, agricultural enterprises may struggle to quickly respond to market demand, thereby weakening the incentive effect of market adjustment. Therefore, in the western region, an incentive model of “government regulation as the main driver, supplemented by market adjustment” should be used.
On the other hand, as low-carbon agricultural production technologies mature, agricultural enterprises do not have to bear excessive pioneer costs, and the liquidity risk associated with low-carbon transformation becomes more manageable, thereby reducing the reliance on government subsidies to some extent. However, this does not mean that low-carbon transformation no longer requires government subsidies. According to the simulation results, in the central region, when α ≤ 0.1, agricultural enterprises still tend to prefer traditional production. Concurrently, the widespread adoption of low-carbon agricultural production technologies also facilitates the production of low-carbon agricultural products. Under effective market conditions, agricultural enterprises can increase revenue through product premiums (Ma and Ma, 2023). Based on this, enterprises are increasingly turning to market adjustment mechanisms for low-carbon transformation (Nee, 1992). However, in China, consumers tend to prefer traditional consumption, and the demand in the mid-to-high-end market is weak, making it difficult to obtain sufficient economic returns through market premiums. Therefore, the market adjustment mechanism still needs to be improved further. Considering the marginal utility of government subsidies and the prospects of market incentives, the central region should gradually increase promotion efforts, supported by appropriate subsidies.
Moreover, according to the simulation results, while low-carbon agriculture in the eastern region is less dependent on government regulation and market adjustment, this does not imply complete withdrawal of both mechanisms. They still play pivotal roles in the long-term sustainable development of low-carbon agriculture. At the mature stage of low-carbon agricultural production technology, the government's role should shift from being a “subsidizer” to a “supporter,” focusing on supporting technology innovation and institution improvement (Bian, 2023), whereas market incentives remain the core element for enterprises to maintain competitiveness. Therefore, the eastern region should adopt a “market adjustment as the main driver, supplemented by government regulation” combination model.
In summary, government regulation and market adjustment are indispensable incentive factors for the development of low-carbon agriculture, with both playing different incentive roles at various stages. To further promote management innovation and improve market mechanisms, this study, based on the simulation results and practical development of low-carbon agriculture in China, proposed the following analytical framework (Figure 11). The framework comprises four key stages: defining policy objective, identifying the development stage, formulating governance strategy, and achieving transformation effect. Among these stages, formulating governance strategy is crucial for achieving the agricultural low-carbon transformation. This requires comprehensive consideration of the synergistic effects of government regulation and market adjustment, along with stakeholder participation mechanisms, to formulate differentiated policies that ensure the efficient advancement and continued deepening of agricultural low-carbon transformation.
To analyze the differentiated impacts of government regulation and market adjustment on the agricultural low-carbon transformation, this study constructed an evolutionary game model based on bounded rationality to explore the strategic interactions and dynamic evolution among local governments, agricultural enterprises, and consumers. On this basis, this study further discussed the effects of policy combinations on the agricultural low-carbon transformation in different regions and proposed targeted policy recommendations. The main research conclusions are as follows:
First, through replicator dynamic analysis, this study identified three possible ESSs, (1, 0, 0), (1, 1, 0), and (1, 1, 1), which correspond to different stages of low-carbon agriculture in China. When the constraints PtDt + Cm + (1 − α)(1 − d)Cl − λPl(1 + β)Dl − Co − P − Ct < 0 and Pl(1 + β)Dl − PtDt − V − (1 + β)U < 0 are met, the system evolves to the optimal stable equilibrium of (1, 1, 1).
Second, this study demonstrated the key influencing factors of the agricultural low-carbon transformation considering the interactions among local governments, agricultural enterprises, and consumers. There are threshold values for the relevant parameters of government regulation and market adjustment. The optimal ranges for the subsidy and promotion coefficients are (0.2, 0.25) and (0.3, 0.35), respectively. Excessive subsidies and promotion increase the financial burden on local governments. The optimal range for carbon emission penalties is (1.5, 1.75). Too low penalties fail to achieve effective emission reduction, whereas excessively high penalties may increase the survival pressure on enterprises and even result in a “negative incentive effect.” When the low-carbon consumption preference is within (0.65, 0.7), the system achieves Pareto optimality. An excessively high low-carbon consumption preference can easily lead to malicious market behaviors.
Third, considering the regional technology differences in China, promoting the agricultural low-carbon transformation requires formulating differentiated policy combinations based on actual development needs. The western region, where agricultural low-carbon production technologies are underdeveloped, should use a combination model of “government regulation as the main driver, supplemented by market adjustment.” The central region should gradually increase promotion efforts based on appropriate subsidies, whereas the eastern region, where low-carbon production technologies are relatively advanced, should mainly rely on market adjustment, with government regulation as a supplement.
Based on the research findings of this study and practical experiences from the agricultural low-carbon transformation in China, we proposed the following policy recommendations:
First, the government policy formulation should follow the principle of moderation. According to the simulation results in this study, there are optimal ranges for reward and penalty policies as well as government promotion. Excessive fiscal spending not only leads to resource misallocation but may also hinder the agricultural low-carbon transformation. Therefore, before issuing policies, local governments should conduct comprehensive market research and scientifically calculate the reward and penalty amounts to avoid formalism and excessive force.
Second, the agricultural low-carbon transformation should emphasize the role of market adjustment mechanisms. On the one hand, local governments should strengthen policy promotion within a reasonable scope to form a social consensus on low-carbon consumption, thereby increasing consumer preference for low-carbon agricultural products and driving agricultural enterprises toward low-carbon transformation through market demand. On the other hand, the government should improve the unified standards and certification systems for the quality inspection of low-carbon agricultural products, reflecting the market principle of “high quality, high price.” This would help avoid the “lemon problem” caused by information asymmetry, enhance consumer trust in low-carbon agricultural products, and thereby promote the sustainable development of low-carbon agriculture.
Finally, given the regional technology differences in China, a classified and regional approach must be employed to promote the transformation. In the eastern region, where economic levels are high, technological development is mature, and market mechanisms are relatively well established, the market mechanism should be fully adopted to guide agricultural enterprises in the low-carbon transformation. In the central region, the role of the market mechanism should gradually be increased on the basis of government incentives. In the western region, where the technological foundation is relatively weak, the government should take the lead at this stage by providing financial subsidies and technical support to promote the initial development of low-carbon agriculture. Once stability is achieved, market mechanisms can then be gradually introduced.
The theoretical importance of this study lies in providing a comprehensive theoretical framework for understanding the agricultural low-carbon transformation, analyzing the effectiveness of government regulation, the role of market adjustment, and the regional differences in policy combinations. In terms of practical importance, this study proposes policy recommendations for promoting low-carbon agriculture. However, there are certain limitations: (1) The analysis of low-carbon transformation in this study is limited to the perspective of evolutionary game theory, neglecting alternative theoretical frameworks, such as innovation systems, decision support systems, and institutional economics. Future research could consider integrating multiple theoretical perspectives, such as innovation systems and institutional economics, to gain a more comprehensive understanding of the mechanisms by which government and market influence the agricultural low-carbon transformation. (2) The analysis of the evolutionary game model requires more accurate data to predict the behavior of stakeholders. Future research should further improve the model, consider other variable factors, and conduct empirical testing to enhance the model's accuracy.
The original contributions presented in the study are included in the article/supplementary material, further inquiries can be directed to the corresponding author.
FL: Methodology, Software, Writing – original draft, Writing – review & editing. YZ: Methodology, Supervision, Writing – original draft, Writing – review & editing. XW: Resources, Writing – original draft, Writing – review & editing. LX: Validation, Writing – original draft, Writing – review & editing.
The author(s) declare that financial support was received for the research and/or publication of this article. This research was funded by the National Social Science Fund of China (22BGL112), the Key Project of Zhejiang Soft Science Research Program (2024C25028), the Wenzhou Science and Technology Specialist Program (X2023093), and the Yongjia County Modern Agricultural Industry Enhancement Project (2024YJ008).
The authors declare that the research was conducted in the absence of any commercial or financial relationships that could be construed as a potential conflict of interest.
The author(s) declare that no Gen AI was used in the creation of this manuscript.
All claims expressed in this article are solely those of the authors and do not necessarily represent those of their affiliated organizations, or those of the publisher, the editors and the reviewers. Any product that may be evaluated in this article, or claim that may be made by its manufacturer, is not guaranteed or endorsed by the publisher.
1. ^In 2020, the “14th Five-Year Plan” emphasized ecological restoration in agriculture and the development of low-carbon agricultural industry chains to achieve the dual-carbon targets. In 2021, the National Energy Administration and other agencies issued the “Implementation Opinions on Accelerating the Transformation and Development of Rural Energy to Support Rural Revitalization,” proposing to increase the proportion of renewable energy. In 2022, the National Development and Reform Commission and other institutions jointly issued the “Implementation Plan for Carbon Emission Reduction and Carbon Sequestration in Agriculture and Rural Areas,” elucidating key tasks for emission reduction and carbon sequestration. In 2024, the Ministry of Agriculture and Rural Affairs issued the “Guiding Opinions on Accelerating the Comprehensive Green Transformation of Agricultural Development to Promote Ecological Revitalization in Rural Areas,” setting green development goals for agriculture.
2. ^For example, Shandong Province, based on the principles of “making continuous improvement in ecological environment quality a binding requirement for regional development” and “those who protect, benefit; those who pollute, pay,” took the lead in implementing the ecological compensation reward and penalty mechanism reform across the province in 2014. After the reform, Shandong's environmental quality showed considerable improvement, and the average annual GDP growth rate of cities increased accordingly.
3. ^Such as the “high subsidy—low promotion” or “low subsidy—high promotion” combination models. The “low subsidy—low promotion” combination often fails to produce significant policy effects, and there may be concerns on passive regulation by local governments. Meanwhile, the “high subsidy—high promotion” combination can easily lead to financial burden, which is not conducive to the sustainable development of agricultural carbon emission reduction. Therefore, these two combination models are not considered in this section.
Acs, Z. J., Morck, R., Shaver, J. M., and Yeung, B. (1997). The internationalization of small and medium-sized enterprises: a policy perspective. Small Bus. Econ. 9, 7–20. doi: 10.1023/A:1007991428526
Akerlof, G. A. (1970). The market for ‘lemons': quality uncertainty and the market mechanism. Market Fail. Success 66, 488–500. doi: 10.2307/1879431
Barham, B. L., Callenes, M., Gitter, S., Lewis, J., and Weber, J. (2011). Fair trade/organic coffee, rural livelihoods, and the “agrarian question”: Southern Mexican coffee families in transition. World Dev. 39, 134–145. doi: 10.1016/j.worlddev.2010.08.005
Bian, A. (2023). Policy simulation analysis on accelerating research and development of green and low-carbon technology based on the carbon dioxide emissions peak and carbon neutrality. Soft Sci. 37, 94–102. doi: 10.13956/j.ss.1001-8409.2023.08.13
Cai, J., and Wang, Q. (2023). Analysis of factors influencing farmers' adoption behavior of low-carbon agricultural technology. Sci. Technol. Manag. Res. 43, 255–266. doi: 10.3969/j.issn.1000-7695.2023.23.028
Chen, H., Zhou, R., and Lei, H. (2023). Incentives and penal[ties:the lmpact of pilot carbon emissions trading on industrial structure. J. Yunnan Univ. Financ. Econ. 39, 18−34. doi: 10.16537/j.cnki.jynufe.000870
Chen, R., Deng, Y., and Jiang, Z. (2018). Spatial and temporal pattern of regional agricultural carbon compensation based on the modified carbon measurement. Econ. Geogr. 38, 168–1177.
China Statistics Press (2021). Compilation of Cost-Benefit Data on Agricultural Products. Beijing: Nationwide.
Coninx, K., Deconinck, G., and Holvoet, T. (2018). Who gets my flex? An evolutionary game theory analysis of flexibility market dynamics. Appl. Energy 218, 104–113. doi: 10.1016/j.apenergy.2018.02.098
Echeverría, R., Hugo Moreira, V., Sepúlveda, C., and Wittwer, C. (2014). Willingness to pay for carbon footprint on foods. Br. Food J. 116, 186–196. doi: 10.1108/BFJ-07-2012-0292
Feng, N., and Ge, J. (2024). How does fiscal policy affect the green low-carbon transition from the perspective of the evolutionary game? Energy Econ. 134:107578. doi: 10.1016/j.eneco.2024.107578
Freibauer, A., Rounsevell, M. D., Smith, P., and Verhagen, J. (2004). Carbon sequestration in the agricultural soils of Europe. Geoderma 122, 1–23. doi: 10.1016/j.geoderma.2004.01.021
Gao, M., and Zhang, Z. X. (2022). Positioning and policy suggestions of China's agricultural green development under the targets of carbon peaking and carbon neutrality. J. Huazhong Agric. Univ. 2022, 24–31. doi: 10.13300/j.cnki.hnwkxb.2022.01.003
Geng, N., Liu, Z., Han, X., and Zhang, X. (2022). Influencing factors and group differences of urban consumers' willingness to pay for low-carbon agricultural products in China. Int. J. Environ. Res. Public Health 20:358. doi: 10.3390/ijerph20010358
Gu (2014). Research on the compensation mechanism for low-carbon agriculture: a case study of grain cropping. J. Humanities 12, 125–1128. doi: 10.15895/j.cnki.rwzz.2014.12.018
Guo, Z., Chen, X., and Zhang, Y. (2022). Impact of environmental regulation perception on farmers' agricultural green production technology adoption: a new perspective of social capital. Technol. Soc. 71:102085. doi: 10.1016/j.techsoc.2022.102085
He, P., Zhang, J., and Li, W. (2021). The role of agricultural green production technologies in improving low-carbon efficiency in China: necessary but not effective. J. Environ. Manag. 293:112837. doi: 10.1016/j.jenvman.2021.112837
He, Y., and Liao, P. (2024). Carbon insurance, low-carbon fiscal and tax policies, and agricultura carbon reduction: an evolutionary game analysis based on carbon emission trading. Soft Sci. 38, 107–1117. doi: 10.13956/j.ss.1001-8409.2024.05.15
Hou, J., and Hou, B. (2019). Farmers' adoption of low-carbon agriculture in China: an extended theory of the planned behavior model. Sustainability 11:1399. doi: 10.3390/su11051399
Hu, J., Fang, Q., and Long, W. (2023). Carbon emission regulation, corporate emission reduction incentive and total factor productivity: a natural experiment based on China's carbon emission trading system. Econ. Res. J. 58, 77–94.
Hu, J., Huang, N., and Shen, H. T. (2020). Can market-incentive environmental requlation promote corporate innovation? A natural experiment based on China's carbon emissions trading mechanism. J. Financ. Res. 1, 171–189.
Hu, Z.-H., and Wang, S.-W. (2022). An evolutionary game model between governments and manufacturers considering carbon taxes, subsidies, and consumers' low-carbon preference. Dyn. Games Appl. 12, 513–551. doi: 10.1007/s13235-021-00390-3
Huang, J., Su, L., and Wang, Y. (2024). Digital technologies facilitate agricultural and rural development: opportunities, challenges, and future directions. China Rural Econ. 1, 21–40. doi: 10.20077/j.cnki.11-1262/f.2024.01.019
Huang, W., Wu, F., Han, W., Li, Q., Han, Y., Wang, G., et al. (2022). Carbon footprint of cotton production in China: composition, spatiotemporal changes and driving factors. Sci. Total Environ. 821:153407. doi: 10.1016/j.scitotenv.2022.153407
Hui, M., Quan, Y.-R., and Yong, F. (2023). Risk preferences and the low-carbon agricultural technology adoption: evidence from rice production in China. J. Integr. Agric. 22, 2577–2590. doi: 10.1016/j.jia.2023.07.002
Jin, S. Q., Niu, K. Y., and Han, D. M. (2020). The path of agricultural green development and lts orientation in the 14th five-year plan period. Reform 2, 30–39.
Ke, C., and Huang, S.-Z. (2024). The effect of environmental regulation and green subsidies on agricultural low-carbon production behavior: a survey of new agricultural management entities in Guangdong Province. Environ. Res. 242:117768. doi: 10.1016/j.envres.2023.117768
Kerin, R. A., Varadarajan, P. R., and Peterson, R. A. (1992). First-mover advantage: a synthesis, conceptual framework, and research propositions. J. Mark. 56, 33–52. doi: 10.1177/002224299205600404
Kong, D., Li, M., and Jin, L. (2017). Research on the performance evaluation and incentive mechanism for transfer payments to national key ecological function zones. Inq. Econ. Issues 7, 81–87.
Lanoie, P., Patry, M., and Lajeunesse, R. (2008). Environmental regulation and productivity: testing the porter hypothesis. J. Product. Anal. 30, 121–128. doi: 10.1007/s11123-008-0108-4
Lee, J., Veloso, F. M., and Hounshell, D. A. (2011). Linking induced technological change, and environmental regulation: evidence from patenting in the US auto industry. Res. Policy 40, 1240–1252. doi: 10.1016/j.respol.2011.06.006
Li, G., and Ying, R. (2004). “Lemon market” and institutional arrangements: an analytical framework for ensuring agricultural product quality and safety. J. Agrotech. Econ. 3, 15–20.
Li, X., and Wang, B. (2010). Low-carbon agriculture: the path of agricultural development in response to climate change. Rural Econ. 3, 10–12.
Lin, B., and Xu, M. (2019). Good subsidies or bad subsidies? Evidence from low-carbon transition in China's metallurgical industry. Energy Econ. 83, 52–60. doi: 10.1016/j.eneco.2019.06.015
Liu, F., Wei, Y., Du, Y., and Lv, T. (2022). Mechanism and influencing factors of low-carbon coal power transition under China's carbon trading scheme: an evolutionary game analysis. Int. J. Environ. Res. Public Health. 20:463. doi: 10.3390/ijerph20010463
Luo, J., Huang, M., and Bai, Y. (2024). Promoting green development of agriculture based on low-carbon policies and green preferences: an evolutionary game analysis. Environ. Dev. Sustain. 26, 6443–6470. doi: 10.1007/s10668-023-02970-2
Luo, J., and Xu, L. (2010). On production, connotation and development strategy of low carbon agriculture. Res. Agric. Modern. 31, 701–703+728. doi: 10.3969/j.issn.1000-0275.2010.06.014
Lyu, X., Shi, A., and Wang, X. (2020). Research on the impact of carbon emission trading system on low-carbon technology innovation. Carbon Manag. 11, 183–193. doi: 10.1080/17583004.2020.1721977
Ma, X., and Ma, L. (2023). Research on the compatibility strategy of farmers' income growth and green management of agricultural products market system experiment based on single substitution and network externality. Tax. Econ. 5, 66–74.
Malkiel, B. G. (1989). “Efficient market hypothesis,” in Finance (Springer: New York) 127–134. doi: 10.1007/978-1-349-20213-3_13
Matsuo, T., and Schmidt, T. S. (2017). Hybridizing low-carbon technology deployment policy and fossil fuel subsidy reform: a climate finance perspective. Environ. Res. Lett. 12:14002. doi: 10.1088/1748-9326/aa5384
Mei, L., Zheng, Y., Tian, M., and Wu, Y. (2024). Driven by the policy or bent by the market? Cracking the digital transformation code of farmer cooperatives. Technol. Forecast. Soc. Change 208:123659. doi: 10.1016/j.techfore.2024.123659
Moraga-González, J. L., Sándor, Z., and Wildenbeest, M. R. (2013). Semi-nonparametric estimation of consumer search costs. J. Appl. Econom. 28, 1205–1223. doi: 10.1002/jae.2290
Nee, V. (1992). Organizational dynamics of market transition: hybrid forms, property rights, and mixed economy in China. Adm. Sci. Q. 37, 1–27. doi: 10.2307/2393531
Norse, D. (2012). Low carbon agriculture: objectives and policy pathways. Environ. Dev. 1, 25–39. doi: 10.1016/j.envdev.2011.12.004
Nyambuu, U., and Semmler, W. (2020). Climate change and the transition to a low carbon economy–Carbon targets and the carbon budget. Econ. Model. 84, 367–376. doi: 10.1016/j.econmod.2019.04.026
Ren, Z., and Zhong, K. (2022). Driving mechanism of subjective cognition on farmers' adoption behavior of straw returning technology: evidence from rice and wheat producing provinces in China. Front. Psychol. 13:922889. doi: 10.3389/fpsyg.2022.922889
Ritzberger, K., and Weibull, J. W. (1995). Evolutionary selection in normal-form games. Econometrica 63, 1371–1399. doi: 10.2307/2171774
Sahin, I. (2006). Detailed review of Rogers' diffusion of innovations theory and educational technology-related studies based on Rogers' theory. Turk. Online J. Educ. Technol. 5, 14–23.
Schulte, E., Scheller, F., Pasut, W., and Bruckner, T. (2022). Product traits, decision-makers, and household low-carbon technology adoptions: moving beyond single empirical studies. Energy Res. Soc. Sci. 83:102313. doi: 10.1016/j.erss.2021.102313
Selten, R., and Selten, R. (1988). A Note on Evolutionarily Stable Strategies in Asymmetric Animal Conflicts. Springer: New York. doi: 10.1007/978-94-015-7774-8_3
Shao, S., and Li, X. (2022). Can market-oriented low-carbon policies promote high-quality economic development? evidence from the carbon emissions trading pilots. Soc. Sci. Guangdong. 214, 33–45.
Sneeringer, S. E. (2009). Effects of environmental regulation on economic activity and pollution in commercial agriculture. BE J. Econ. Anal. Policy 9. doi: 10.2202/1935-1682.2248
Stavins, R. N. (2010). “Market-based environmental policies,” in Public Policies for Environmental Protection (London: Routledge), 31–76.
Stewart, F. (2022). Friends with benefits: how income and peer diffusion combine to create an inequality “trap” in the uptake of low-carbon technologies. Energy Policy. 163:112832. doi: 10.1016/j.enpol.2022.112832
Stocker, T. (2014). Climate Change 2013: the Physical Science Basis: Working Group I Contribution to the Fifth Assessment Report of the Intergovernmental Panel on Climate Change. Cambridge: Cambridge University Press.
Sun, P., Jianming, W., and Xueshuang, J. (2024). “Ecological curse” or “ecological well-being”: on the carbon effects of agroecological carrying capacity. J. Nanjing Agric. Univ. 24, 172–186.
Sun, Y., Liu, B., Sun, Z., and Yang, R. (2023). Inter-regional cooperation in the transfers of energy-intensive industry: an evolutionary game approach. Energy 282:128313.
Sun, Y., Miao, Y., Xie, Z., and Wu, R. (2024). Drivers and barriers to digital transformation in agriculture: An evolutionary game analysis based on the experience of China. Agric. Syst. 221:104136. doi: 10.1016/j.agsy.2024.104136
Tian, Y., and Chen, C. (2021). Research on the compensation mechanism of agricultural carbon emission reduction in China from the perspective of combination of market and government. Issues Agric. Econ. 2021, 120–12136.
Wang, D., and Wang, J. (2010). Research on the upgrade of Chinese food consuming structure under the new situation. Issues Agric. Econ. 31, 75–79+112. doi: 10.13246/j.cnki.iae.2010.06.007
Wang, G., and Yang, Y. (2021). To make decision on adopting organic fertilizer instead of chemical fertilizer: market-driven or government incentives? Rural Econ. 4, 102–1110.
Wang, S. L., Caldwell, C. D., and Zhu, W. (2010). Low carbon agriculture: origins, principles and strategies. Res. Agric. Modern. 31, 604–607. doi: 10.3969/j.issn.1000-0275.2010.05.021
Wang, Y. (2008). “A brief discussion on low-carbon agricultural economy,” in The Seventh Member Representative Conference of the Chinese Society of Ecological Economics and the Seminar on Ecological Economy and Harmonious Society (Nanjing).
Wickramarathne, T. L., Premaratne, K., and Murthi, M. N. (2012). “Consensus-based credibility estimation of soft evidence for robust data fusion,” in Belief Functions: Theory and Applications: Proceedings of the 2nd International Conference on Belief Functions, Compiègne, France (Springer, Ney Work), 9–11. doi: 10.1007/978-3-642-29461-7_35
Xie, H., Wang, W., and Zhang, X. (2018). Evolutionary game and simulation of management strategies of fallow cultivated land: a case study in Hunan province, China. Land Use Policy 71, 86–97. doi: 10.1016/j.landusepol.2017.11.050
Xing, X.-H., Hu, Z.-H., and Luo, W.-P. (2023). Using evolutionary game theory to study governments and logistics companies' strategies for avoiding broken cold chains. Ann. Oper. Res. 329, 127–155. doi: 10.1007/s10479-020-03599-4
Xu, A. (2022). Study on the lmpact of green low-carbon transformation on enterprise profitability under the 'double carbon' target. Macroeconomics 1, 161–175. doi: 10.16304/j.cnki.11-3952/f.2022.01.013
Yang, J., and Xu, J. (2016). The inequity of environmental benefit distribution and its transfer mechanism. Econ. Res. J. 51, 155−167.
Yang, X., Qi, Z., Chen, X., and Yang, C. (2020). Influence of social capital on farmers' adoption of ecological agriculture technology decision-making behavior: taking rice and shrimp co-culture technology as an example. J. China Agric. Univ. 25, 1183–1198.
Zhang (2022). The logic of chemical use reduction in agriculture: an analyzing structure. Agric. Econ. 15–26.
Zhang, C., Lu, Y., Guo, L., and Yu, T. (2011). The intensity of environmental regulation and technological progress of production. Econ. Res. J. 46, 113–124.
Zhang, H., Shen, K., Liang, R., and Yang, J. (2024). Research on the Advantageous Effects of the Reform of the Ecological Compensation Reward and Punishment Mechanism on Air Pollution Control. J. Manag. World 40, 114–133. doi: 10.19744/j.cnki.11-1235/f.2024.0072
Zhang, L., and Luo, B. L. (2022). The logic of chemical use reduction in agriculture: an analyzing structure. Issues Agric. Econ. 4, 15–126. doi: 10.13246/j.cnki.iae.2022.04.002
Zhang, M., and Wang, Y. (2022). Low carbon policy pilot, regional lmbalance and urban wealth growth. J. Shanxi Univ. Financ. Econ. 44,1–16. doi: 10.13781/j.cnki.1007-9556.2022.08.001
Zhang, X., and Xu, F. (2022). The influence of environmental regulation on green technology innovation: regional heterogeneity analysis from the perspective of government intervention. Urban Prob. 9, 55–64. doi: 10.13239/j.bjsshkxy.cswt.220906
Zhao, D., and Zhou, H. (2021). Livelihoods, technological constraints, and low-carbon agricultural technology preferences of farmers: analytical frameworks of technology adoption and farmer livelihoods. Int. J. Environ. Res. Public Health 18:13364. doi: 10.3390/ijerph182413364
Zhao, R., Peng, L., Zhao, Y., and Feng, Y. (2024). Coevolution mechanisms of stakeholder strategies in the green building technologies innovation ecosystem: an evolutionary game theory perspective. Environ. Impact Assess. Rev. 105:107418. doi: 10.1016/j.eiar.2024.107418
Zheng, H., Ma, J., Yao, Z., and Hu, F. (2022). How does social embeddedness affect farmers' adoption behavior of low-carbon agricultural technology? Evidence from Jiangsu province, China. Front. Environ. Sci. 10:909803. doi: 10.3389/fenvs.2022.909803
Zheng, W. (2001). Impacts of global change on China's food security. Geogr. Res. 20, 282–2289. doi: 10.3969/j.issn.1560-8999.2004.01.024
Zhu, H., and Tian, Z. (2012). Analysis on dilemma of low carbon agriculture technology in rice production. J. China Agric. Univ. 29, 153–160. doi: 10.13240/j.cnki.caujsse.2012.04.001
Zwolinski, M. (2008). The ethics of price gouging. Bus. Ethics Q. 18, 347–378. doi: 10.5840/beq200818327
Keywords: low-carbon agriculture, government regulation, market adjustment, technology differences, evolutionary game
Citation: Liao F, Zheng Y, Wang X and Xiong L (2025) Government-market synergy in China's agricultural low-carbon transformation: policy adaptation to regional divides. Front. Sustain. Food Syst. 9:1570678. doi: 10.3389/fsufs.2025.1570678
Received: 04 February 2025; Accepted: 21 March 2025;
Published: 15 April 2025.
Edited by:
Anas Tallou, University of Bari Aldo Moro, ItalyReviewed by:
Min Xu, Chinese Academy of Fishery Sciences, ChinaCopyright © 2025 Liao, Zheng, Wang and Xiong. This is an open-access article distributed under the terms of the Creative Commons Attribution License (CC BY). The use, distribution or reproduction in other forums is permitted, provided the original author(s) and the copyright owner(s) are credited and that the original publication in this journal is cited, in accordance with accepted academic practice. No use, distribution or reproduction is permitted which does not comply with these terms.
*Correspondence: Xiaofeng Wang, d2FuZ3hpYW9mZW5nQHd6dmNzdC5lZHUuY24=
Disclaimer: All claims expressed in this article are solely those of the authors and do not necessarily represent those of their affiliated organizations, or those of the publisher, the editors and the reviewers. Any product that may be evaluated in this article or claim that may be made by its manufacturer is not guaranteed or endorsed by the publisher.
Research integrity at Frontiers
Learn more about the work of our research integrity team to safeguard the quality of each article we publish.