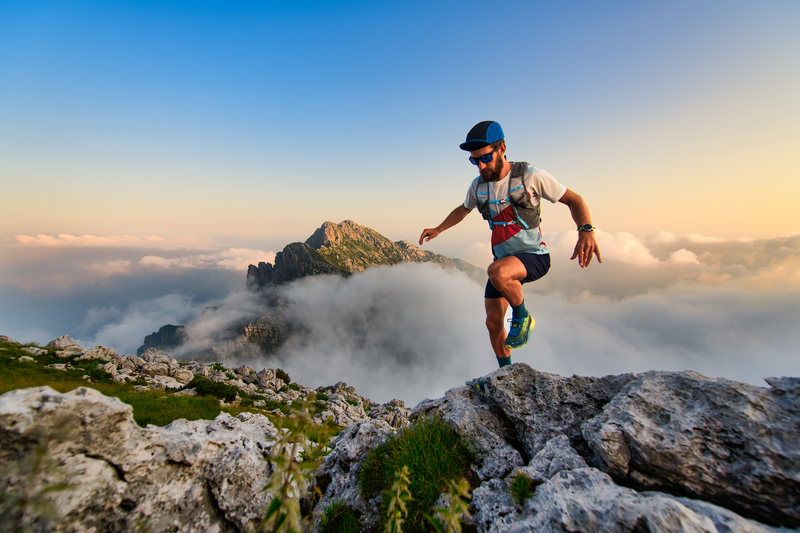
95% of researchers rate our articles as excellent or good
Learn more about the work of our research integrity team to safeguard the quality of each article we publish.
Find out more
ORIGINAL RESEARCH article
Front. Sustain. Food Syst.
Sec. Agricultural and Food Economics
Volume 9 - 2025 | doi: 10.3389/fsufs.2025.1568041
This article is part of the Research Topic Agricultural Innovation in the Age of Climate Change: A 4.0 Approach View all 4 articles
The final, formatted version of the article will be published soon.
You have multiple emails registered with Frontiers:
Please enter your email address:
If you already have an account, please login
You don't have a Frontiers account ? You can register here
As an essential part of daily life, the drastic fluctuations in agricultural commodity prices significantly impact producers' motivation and consumers' quality of life, further exacerbating market uncertainty and unsustainability. The ability to scientifically and effectively predict agricultural commodity prices is of great significance for the rational deployment of market mechanisms, the timely adjustment of supply chains, and the promotion of food policy adjustments. This paper proposes a sustainable hybrid model SV-PSO-BiLSTM which integrates Seasonal-Trend decomposition procedure based on Loess (STL), Variational Mode Decomposition (VMD), Particle Swarm Optimization (PSO), and Bidirectional Long Short-Term Memory (BiLSTM) neural networks. This innovative approach first performs seasonal decomposition of the original data using the STL method, then applies the VMD method for double decomposition of the residual components, reconstructs the data based on sample entropy, and finally predicts agricultural commodity market prices using the BiLSTM network model optimized by the PSO algorithm. This paper investigates the market price dynamics of four agricultural commodities (chili, garlic, ginger, and pork) and one agricultural financial derivative (soybean futures). The experimental results indicate that the proposed SV-PSO-BiLSTM hybrid model achieves average values of 0.2241 for root mean square error (RMSE), 0.1665 for mean absolute error (MAE), 0.0207 for mean absolute percentage error (MAPE), and 0.9851 for the coefficient of determination (R² ). These results surpass those of other comparative models, demonstrating stronger generalization, reliability, and stability. The research findings can provide effective guidance for the reasonable regulation of agricultural commodity market prices and further promote the healthy and sustainable development of the agricultural commodity industry.
Keywords: Agricultural commodity, Price Prediction, Dual decomposition, multiple hybrid model, BiLSTM, Supply Chains
Received: 28 Jan 2025; Accepted: 26 Mar 2025.
Copyright: © 2025 Zhang, Wang, Wang, Wang, Chen and Liu. This is an open-access article distributed under the terms of the Creative Commons Attribution License (CC BY). The use, distribution or reproduction in other forums is permitted, provided the original author(s) or licensor are credited and that the original publication in this journal is cited, in accordance with accepted academic practice. No use, distribution or reproduction is permitted which does not comply with these terms.
* Correspondence:
Chao Wang, College of Information Science and Technology, Hebei Agricultural University, Baoding, China
Disclaimer: All claims expressed in this article are solely those of the authors and do not necessarily represent those of their affiliated organizations, or those of the publisher, the editors and the reviewers. Any product that may be evaluated in this article or claim that may be made by its manufacturer is not guaranteed or endorsed by the publisher.
Research integrity at Frontiers
Learn more about the work of our research integrity team to safeguard the quality of each article we publish.