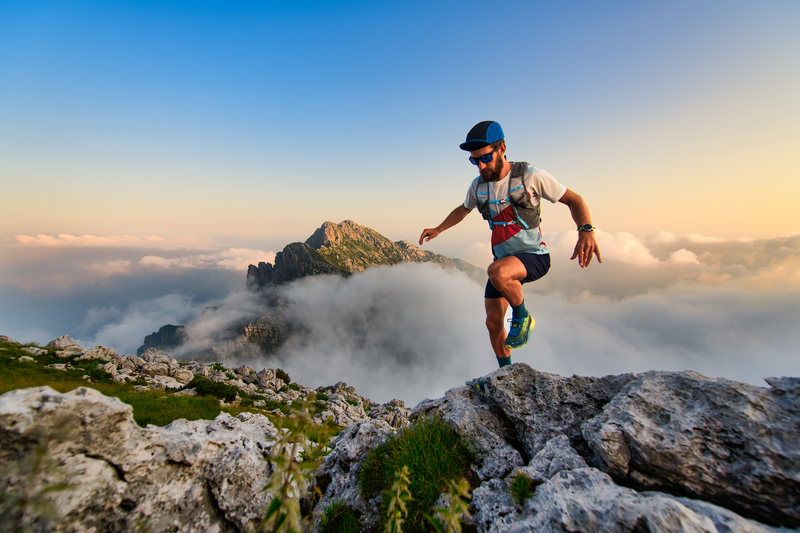
95% of researchers rate our articles as excellent or good
Learn more about the work of our research integrity team to safeguard the quality of each article we publish.
Find out more
ORIGINAL RESEARCH article
Front. Sustain. Food Syst.
Sec. Climate-Smart Food Systems
Volume 9 - 2025 | doi: 10.3389/fsufs.2025.1565776
This article is part of the Research Topic Conservation Agriculture For Food Security And Climate Resilience View all 8 articles
The final, formatted version of the article will be published soon.
You have multiple emails registered with Frontiers:
Please enter your email address:
If you already have an account, please login
You don't have a Frontiers account ? You can register here
Agricultural carbon emission reduction is the meaning of realizing the goal of dual carbon, and Sichuan province, as one of the main grain producing areas in China, it is urgent to realize agricultural carbon reduction. Based on the data of 18 cities in Sichuan province from 2000 to 2022, this paper calculates the total agricultural carbon emission and carbon emission intensity in Sichuan province by using IPCC guidelines, and measures its temporal, spatial evolution trend and regional differences, and further evaluates the driving factors by using fixed-effect model. The results show that: (1) The total quantity of agricultural carbon emissions in Sichuan province has increased, but the carbon intensity has decreased, among which agricultural carbon emissions caused by agricultural land planting and residents' life are the main carbon sources; (2) The regional differences of agricultural carbon emissions in Sichuan province are narrowing, among which the gap between groups is the root of the regional differences of agricultural carbon emissions, which shows that the agricultural carbon emissions in eastern Sichuan and western Sichuan, eastern Sichuan and southern Sichuan, western Sichuan and southern Sichuan, are quite different; (3) Agricultural carbon emissions in Sichuan province are characterized by agglomeration and spatial spillover, mainly showing a High-High agglomeration mode, but a few cities have changed their agglomeration modes; (4) The agricultural carbon intensity in Sichuan province is influenced by multiple factors. Population density, industrial structure, social wealth, agricultural mechanization and technological progress have negative effects on agricultural carbon intensity, while macro-control has increased agricultural carbon intensity. The results of this paper have important implications for the green development of agriculture in Sichuan province.
Keywords: Agricultural carbon emission, Agricultural carbon intensity, Sichuan province, Region difference, Driver factors
Received: 23 Jan 2025; Accepted: 28 Mar 2025.
Copyright: © 2025 Zhang and Shen. This is an open-access article distributed under the terms of the Creative Commons Attribution License (CC BY). The use, distribution or reproduction in other forums is permitted, provided the original author(s) or licensor are credited and that the original publication in this journal is cited, in accordance with accepted academic practice. No use, distribution or reproduction is permitted which does not comply with these terms.
* Correspondence:
Yang Shen, Institute of Quantitative Economics,Huaqiao University, Xiamen, China
Disclaimer: All claims expressed in this article are solely those of the authors and do not necessarily represent those of their affiliated organizations, or those of the publisher, the editors and the reviewers. Any product that may be evaluated in this article or claim that may be made by its manufacturer is not guaranteed or endorsed by the publisher.
Research integrity at Frontiers
Learn more about the work of our research integrity team to safeguard the quality of each article we publish.