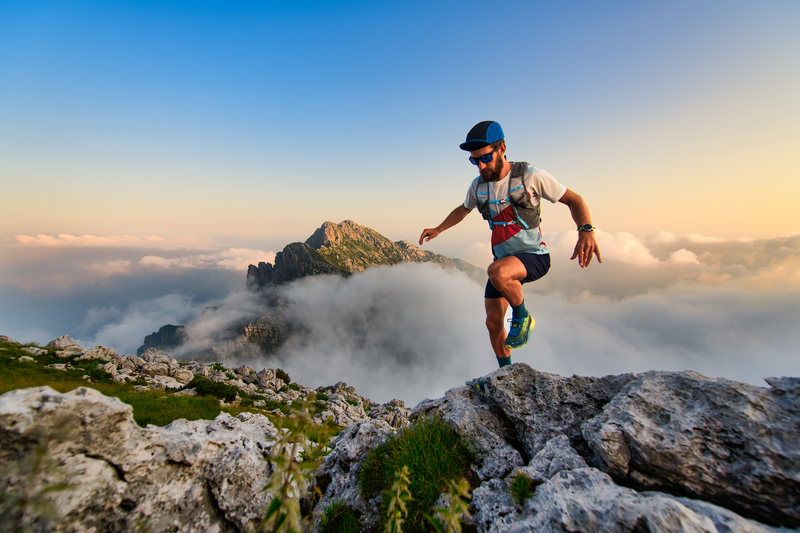
95% of researchers rate our articles as excellent or good
Learn more about the work of our research integrity team to safeguard the quality of each article we publish.
Find out more
ORIGINAL RESEARCH article
Front. Sustain. Food Syst.
Sec. Sustainable Food Processing
Volume 9 - 2025 | doi: 10.3389/fsufs.2025.1565247
This article is part of the Research Topic Machine Learning and Predictive Microbiology: Enhancing Food Safety Models View all articles
The final, formatted version of the article will be published soon.
You have multiple emails registered with Frontiers:
Please enter your email address:
If you already have an account, please login
You don't have a Frontiers account ? You can register here
Salmonella Infantis is a top human clinical isolate that is found at low levels in chicken liver after primary processing. However, temperature abuse of chicken liver during secondary processing can lead to growth of Salmonella and higher risk of salmonellosis. Therefore, a three-phase linear, polynomial regression, tertiary model (TMPR) and a multiple layer feedforward neural network with two nodes in the hidden layer, tertiary model (TMNN) for growth of Salmonella Infantis in chicken liver as a function of dose (10 1 to 10 6 ), time (0 to 8 h), and temperature (18 to 30°C) were constructed, validated, and compared using the criteria of the Acceptable Prediction Zones (APZ) method. When the proportion of residuals in the APZ or pAPZ was ≥ 0.7, predictions were considered acceptable. The pAPZ for the dependent data (n = 360) was 0.979 for the TMPR and 0.976 for the TMNN, whereas the pAPZ for the independent data for interpolation (n = 72) was 0.968 for the TMPR and 0.964 for the TMNN. Thus, both the TMPR and TMNN were validated for interpolation, had similar performance, and can be used with confidence to predict the growth of Salmonella Infantis in chicken liver during a secondary processing deviation of temperature abuse.However, construction of the TMPR involved three steps, whereas construction of the TMNN involved one step. Thus, the TMNN was easier to construct and validate. Nonetheless, the final TM included the TMPR and TMNN because the TMPR predicted lag time and growth rate, whereas the TMNN did not.
Keywords: Salmonella Infantis, Chicken liver, Growth, Tertiary model, Validation, predictive microbiology
Received: 22 Jan 2025; Accepted: 26 Mar 2025.
Copyright: © 2025 Oscar. This is an open-access article distributed under the terms of the Creative Commons Attribution License (CC BY). The use, distribution or reproduction in other forums is permitted, provided the original author(s) or licensor are credited and that the original publication in this journal is cited, in accordance with accepted academic practice. No use, distribution or reproduction is permitted which does not comply with these terms.
* Correspondence:
Thomas P. Oscar, United States Department of Agriculture (USDA), Washington, United States
Disclaimer: All claims expressed in this article are solely those of the authors and do not necessarily represent those of their affiliated organizations, or those of the publisher, the editors and the reviewers. Any product that may be evaluated in this article or claim that may be made by its manufacturer is not guaranteed or endorsed by the publisher.
Research integrity at Frontiers
Learn more about the work of our research integrity team to safeguard the quality of each article we publish.