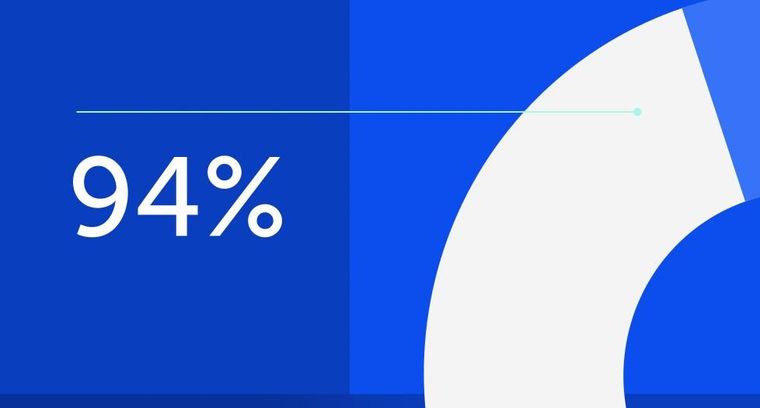
94% of researchers rate our articles as excellent or good
Learn more about the work of our research integrity team to safeguard the quality of each article we publish.
Find out more
ORIGINAL RESEARCH article
Front. Sustain. Food Syst., 16 April 2025
Sec. Agricultural and Food Economics
Volume 9 - 2025 | https://doi.org/10.3389/fsufs.2025.1564443
This article is part of the Research TopicHarnessing Digital Innovation for Sustainable Agricultural DevelopmentView all 13 articles
Purpose: The purpose of this study is to explore the relationship between the transportation industry and agricultural resilience, focusing on how the digital economy promotes synergistic resilience so as to promote the improvement of agricultural industry resilience. The research goal is to provide policy recommendations for regional agricultural development, with particular attention to how to make more effective use of industry synergies to enhance agricultural resilience in the context of digital transformation.
Design/methodology/approach: Based on the panel data of 31 provinces from 2012 to 2022, this study first uses the coupling coordination model to analyze the relationship between industries. Then, the benchmark regression and mediating effect model are used to study how the digital economy promotes the coordinated development of the industry. Finally, the spatial Durbin model is used to test the spatial differences in the synergy between the two industries.
Findings: The results reveal prevalent “high coupling but low coordination” between agricultural and transportation resilience, with coupling degrees mostly above 0.85 yet coordination degrees below 0.4. Digital transformation significantly enhances industry synergy resilience (β = 0.569, p < 0.01), with regional resource allocation mediating nearly half of this effect. Spatial analysis further confirms notable positive spillover effects (β = 0.483, p < 0.01), especially in eastern coastal provinces.
Originality/value: This paper proposes a synergistic effect analysis framework combining digitization and regional differences, which provides a new perspective and method for academic research in related fields. The research results provide policymakers with regional differentiation results and different reference suggestions based on regional characteristics.
As one of the basic industries of China’s economy, agriculture has always occupied an important position in the national development strategy (Li et al., 2024). The National Rural Revitalization Strategic Plan (2021–2025) emphasizes modernizing the agricultural industry and enhancing the resilience of the agricultural supply chain, which is vital for food security and sustainable agricultural growth. The agricultural supply chain’s stability relies heavily on an efficient transportation system, particularly logistics, which ensures timely delivery from production to consumers. The “14th Five-Year Plan for the Development of the Transportation Industry” highlights improving transportation modernization as essential for the efficient allocation of agricultural resources and reducing costs, thereby supporting smooth agricultural supply chain circulation.
In the context of global climate change and the low-carbon transformation, the coordinated, green development of agriculture and transportation is vital for sustainable growth. Reducing carbon emissions and improving energy efficiency are central to the green transformation of these industries (Lee et al., 2022). Digital transformation has been considered to play an important role in promoting the green synergy between agriculture and transportation by many studies (Li et al., 2021). According to the “Outline of Digital Economy Development Plan,” accelerating digital transformation and integrating information technology across industries is essential for high-quality development. Digital technologies not only improve agricultural production efficiency but also promote the green, coordinated development of agriculture and transportation by optimizing resource allocation and energy utilization, ultimately enhancing resilience and reducing carbon emissions.
However, despite extensive literature emphasizing the transformative potential of digitalization in promoting green development, existing studies predominantly focus on isolated industry impacts rather than cross-industry synergies. Systematic insights into how digital transformation concurrently enhances the sustainable synergy and resilience between agricultural and transportation sectors remain scarce. Addressing this critical gap, this study aims to: (1) analyze the current coupling and coordination patterns between agricultural and transportation resilience across Chinese provinces; (2) empirically explore how digital transformation fosters cross-sector resilience synergy, including identifying key mediating mechanisms; and (3) examine the spatial spillover characteristics and regional heterogeneities of this synergy.
This study contributes distinctively to existing literature in three aspects. First, it proposes an innovative analytical framework integrating digital transformation with regional heterogeneity to enrich theoretical understandings of cross-sector resilience synergy. Second, it empirically identifies regional resource allocation as a crucial mediator, elucidating the mechanisms through which digital transformation influences synergistic resilience. Third, it utilizes a spatial Durbin model (SDM) to rigorously assess spatial spillover effects, capturing regional differentiation and providing precise, policy-relevant implications.
The rapid advancement of the digital economy has significantly promoted cross-sectoral collaboration between agriculture and transportation industries. Digital technologies, through intelligent decision-making systems and real-time data sharing platforms, have enhanced operational efficiency and encouraged cross-industry integration (Bi et al., 2023; Wang et al., 2024). In the field of transportation, many scholars have verified the importance of digital technology in improving the resilience of transportation systems by quantifying the resilience model of railway transportation systems, microscopic traffic flow simulation, and the introduction of time-evolving demand bridge reconstruction planning (Adjetey-Bahun et al., 2016; Hou et al., 2019; Wu and Chen, 2023; Yang, 2024; Tubis et al., 2024). In agriculture, digital transformation has also markedly improved rural household resilience, mitigating the adverse impacts caused by rural labor aging. It has narrowed the urban–rural divide by increasing production efficiency and market competitiveness (Xu et al., 2024; Zhang et al., 2023; Li et al., 2021). Moreover, digital technologies have modernized agricultural supply chains, boosting their flexibility and adaptability. Nonetheless, existing literature highlights that digitization’s effects on resilience exhibit nonlinear characteristics and significant spatial spillover effects, underscoring notable regional disparities in digital economic maturity across China’s provinces. Typically, eastern provinces exhibit high levels of digital maturity, whereas central and western provinces lag behind (Luo and Zhou, 2022; Yang et al., 2022; Adve et al., 2024).
In the process of sustainable development in all walks of life, the synergistic effect of green development and the digital economy has become a topic of widespread concern. Many scholars have discussed how the digital economy promotes the realization of green development from different perspectives. Some studies use spatial models and structural decomposition models to analyze how the digital economy promotes green, sustainable development by reducing carbon emissions. The research shows that there is an inverted U-shaped relationship between the digital economy and carbon emission reduction, and there is significant regional heterogeneity (Cheng et al., 2023; Du and Wang, 2024; Hong et al., 2024; Chang et al., 2023). Another type of research explores the role of the digital economy in promoting the green total factor productivity of the manufacturing industry from the perspective of improving green productivity. Studies have shown that the digital economy can not only enhance local green productivity but also drive the growth of green productivity in surrounding areas (Liu et al., 2024; Deng et al., 2022).
However, previous research also suggests that digitization’s positive impacts on green development become apparent only beyond a certain threshold of digital maturity. Furthermore, enhancing industry linkage effects can additionally amplify green sustainable development outcomes (Zhang and Yin, 2023; Jin et al., 2024; Han et al., 2023; Yang et al., 2024). Although existing literature recognizes the critical role of the digital economy in promoting regional green development, its emphasis remains predominantly confined to individual sectors, overlooking cross-sectoral collaboration mechanisms in promoting resilience and green sustainability.
Regional difference is an important factor affecting the realization of digital integration and collaborative resilience of rural and transportation industries. The existing research has discussed the impact of regional differences on the development of industry resilience from the perspectives of coupling synergy, spatial distribution characteristics and driving mechanisms. First of all, there are obvious spatial differences in agricultural resilience and land use efficiency. The differences in infrastructure construction, talent flow and policy support among different provinces directly affect the process and effect of digital transformation (Lamine, 2015; Maria et al., 2021). Secondly, the spatial resilience of suburban rural settlements usually shows strong long-term stability, and the coordinated development of urban and rural ecological resilience is gradually strengthened (Zhou et al., 2024; Zhou and Hou, 2021); in the Yangtze River Delta region, the digital economy and tourism economy of Shanghai and its surrounding cities show obvious high–high agglomeration characteristics, and the synergy effect is strong. However, in the central and marginal regions, the integration level of the digital economy and local industries is low, which restricts the coordinated development of the region (Yang et al., 2024; Su et al., 2022). In the transportation industry, regional differences also show a significant impact, especially in terms of logistics green resilience, the eastern region is generally better than the central and western regions. With a better spatial correlation network, higher connectivity and accessibility, the eastern region has obvious advantages in achieving green logistics resilience. However, the overall tightness and stability still need to be improved (Urruty et al., 2016). In the development of green logistics in the Yangtze River Economic Belt, the imbalance of development levels in different regions is more prominent, and there are significant differences in the speed and quality of green logistics. This requires that all regions should fully consider the local economic structure, resource endowment and development needs when formulating green logistics strategies so as to enhance the synergy resilience of the overall transportation industry (Ariken et al., 2021).
Despite extensive analyses of digital transformation impacts on agriculture and transportation individually, existing studies rarely address the synergistic resilience across these industries comprehensively. Previous literature predominantly adopts single-sector perspectives, inadequately explaining the mechanisms of digital integration in cross-sectoral resilience and failing to effectively capture the spatial dynamics inherent to regional development. This research addresses these critical gaps by proposing an integrative framework explicitly combining digital transformation with cross-industry synergy and regional heterogeneity. Specifically, the study innovatively employs the coupling coordination model, mediating effect analysis, and spatial Durbin model (SDM) to empirically investigate regional variations, clarify digital transformation’s mediating mechanisms via regional resource allocation, and rigorously examine spatial spillover characteristics.
In this paper, the coupling coordination degree model is used to measure the coordinated development level between agricultural toughness and transportation toughness. The coupling degree and coordination degree are calculated, respectively, in the following two steps:
The first step: coupling degree calculation.
The coupling degree is used to measure the interaction strength between agricultural resilience and transportation resilience. An improved coupling function usually calculates it. The formula is as follows (Equation 1):
represents the level of agricultural resilience, represents the level of transportation resilience. The coupling degree C is in the range of [0, 1], When C approaches 1, it indicates a high degree of industry interaction; When C approaches 0, it indicates that the development of the industry is more independent.
The second step: coordination degree calculation.
On the basis of the coupling degree, the coordination degree of the industry is further calculated to measure its coordinated development level. The coordination index model is as follows (Equation 2):
T is the comprehensive effect index, which is used to reflect the overall level of agricultural resilience and transportation resilience. It is generally defined as (Equation 3):
Among them, α and β represent the weight of agricultural and transportation resilience in the comprehensive effect, respectively, and are usually equal (α = β = 0.5). The value range of coordination degree D is [0, 1], When D approaches 1, it shows that the coordinated development of agriculture and transportation resilience is better. When D is low, it shows that the industry has shortcomings in coordinated development.
From the empirical point of view, this paper constructs the following double fixed effect panel regression model to explore the direct impact of digitalization of different industries on agricultural resilience, transportation resilience and industry synergy resilience. The benchmark regression model expression is as follows (Equation 4):
Where I denotes province and t denotes years; represents the industry synergy resilience index in the model; is the level of digital economy development in various industries; represents each control variable; α is the intercept term, β and γ are regression coefficients; is an individual fixed effect, which controls the heterogeneity between provinces; is a time fixed effect, which controls the common influencing factors in the time dimension; is a random error term.
In order to further analyze the path mechanism of the impact of digitization on the resilience of various industries, this paper introduces the intermediary effect model on the basis of the benchmark regression model to explore the indirect effect of digital integration on industrial resilience through intermediary variables. The mediating effect model expression is as follows:
The first step is to test the impact of digitization on mediating variables (Equation 5):
The second step is to test the impact of mediating variables on resilience (under the control of digitization) (Equation 6):
Among them represents the coordinated development of the region in the model, θ is the regression coefficient of the intermediary variable, and the explanation of other variables is the same as above. This study confirms the mediating effect based on the results of both the Sobel and Bootstrap tests.
The SDM combines the characteristics of the spatial error model and the spatial lag model. It contains the spatial lag term of the explained variable and the explanatory variable, so it is widely used in empirical research. Its expression is (Equation 7):
Among them, ρ is the spatial effect coefficient, W is the spatial weight matrix, WY and WX are the spatial lag terms of the dependent variable and the explanatory variable respectively, β is the regression coefficient of the explanatory variable to the dependent variable, θ is the regression coefficient of the spatial lag term of the explanatory variable to the dependent variable; ϵ is the error term.
On the basis of the existing research results of rural economic resilience, the comprehensive index system of green agriculture industry resilience is further explored and constructed. Learn from the findings of relevant researchers (Zhou et al., 2024; Li et al., 2022; Li, 2023; Yu et al., 2023); in this paper, the resilience of the green agriculture industry is divided into three parts: economic resilience, social resilience and engineering resilience. Economic resilience reflects the economic output and production efficiency of rural industries. Social resilience reflects the service level, education level and social participation of rural society. Engineering resilience reflects the coping ability and recovery ability of rural industries in the face of natural disasters. Add ecological and environmental indicators related to green development, such as agricultural carbon emissions and waterlogging areas (Tables 1, 2).
Transportation resilience refers to the ability of transportation systems to maintain basic functions and quickly return to normal or new equilibrium in the face of natural disasters, human factors or other emergencies. It covers three aspects: resistance, adaptability and resilience (Dong et al., 2022; Ilalokhoin et al., 2023). Resistance reflects the ability of the system to maintain its structure and function when it encounters interference, mainly through the scale and quality of infrastructure, such as the operating mileage of railways and highways. Adaptability refers to the ability of the system to adjust and reorganize in the face of changes, ensuring that the service level is not reduced, and indicators such as freight volume and cargo turnover can reflect the flexibility and efficiency of the system. Resilience emphasizes the rapid recovery ability of the system after interference. Key indicators such as the length of the cable line reflect the smoothness of information flow, and carbon emissions are used as negative indicators to measure the impact of system recovery on the environment. Based on the past literature review, this paper constructs the transportation resilience index system as follows.
With reference to relevant literature (Liu et al., 2023; Yi et al., 2022; Wang and Xue, 2024; Liang et al., 2024), this study constructs an index system with industry digital linkage as the independent variable, industry collaborative index as the dependent variable and regional collaborative level index as an intermediary variable. Through infrastructure construction density, information sharing index, rural e-commerce penetration, Internet penetration rate and average R&D investment intensity, these five dimensions jointly reflect the degree of digital integration development. Information sharing index and rural e-commerce penetration reflect the degree of digitization of information circulation and market expansion. Digital infrastructure density, Internet penetration rate, R&D investment and logistics integration measure the role of infrastructure support in promoting synergy. The industry synergy development index is used as the dependent variable to evaluate the synergy effect of agriculture and transportation comprehensively. In contrast, the regional synergy development analyzes the synergy effect between regions through factors such as industrial output, infrastructure and environmental protection. In order to eliminate the influence of external interference factors, control variables such as technology R&D investment are set to ensure that the research focuses on the core impact of digitization; the detailed index construction is shown in Table 3.
The synergy index of the agriculture and transportation industry is increasing year by year, and the data of each province in the past 11 years are shown in Figure 1. However, the synergy index varies greatly among provinces. In 2022, the top three are Shanghai, Guangdong and Zhejiang, and the last three are Hainan, Ningxia and Tibet. In addition, there is a positive correlation between industry resilience and digital integration, but there are some outliers when the digital level is high (Figure 2).
The primary data utilized in this study consists of panel data for 31 provinces from 2012 to 2022, sourced from the Guo Tai database, the China Economic Statistics Database, and the EPS database. Carbon emission data for the transportation sector are obtained from the OECD database. Missing values are addressed using linear interpolation, where each missing observation is replaced by the average of its preceding and succeeding values. This method, commonly applied in time-series analysis, mitigates the potential bias caused by missing data and ensures the continuity and consistency of the dataset.
After using the entropy method to obtain two indexes of green agricultural industry resilience and transportation resilience, the coupling coordination degree model is calculated to obtain the following results.
The test results of the coupling coordination degree model of agricultural resilience and transportation resilience are shown in the figure. The grading reference standard of coupling degree and coupling coordination degree the standard in reference (Chen et al., 2024). Figure 3 shows the degree of coupling between agriculture and transportation toughness, ranging from 0.7 to 1, with colors varying from blue to red. Figure 4 reflects the degree of coordination between the two industries, ranging from 0.25 to 0.73, with the color gradient from blue to red. By comparing the two, it is found that most provinces show the characteristics of high coupling and low coordination, such as Hainan and Tianjin, where the coupling degree is more than 0.85, but the coordination degree is lower than 0.4. Only a few provinces, such as Jiangsu and Shandong, show the characteristics of high coupling and high coordination, which is more than 0.9 coupling degree and 0.7 coordination degree.
Based on the analysis of the coupling coordination degree model of the resilience of the rural industry and the resilience of the transportation industry, it is found that there is a phenomenon of ‘high coupling and low coordination’ between the two industries, which indicates that the industry has strong links but insufficient coordination. It is necessary to strengthen the coordinated development of the industry further. Therefore, the index system model based on the industry’s collaborative indicators is constructed. The research results are as follows.
The regression results (Table 4) reveal a significant positive impact of the digital economy (ED) on industry synergy resilience (BC) with coefficients significant at p < 0.01. Notably, patent applications (RD) and technology market turnover (CT) positively influence industry synergy, whereas excessive technology market turnover (CX) negatively impacts it, suggesting possible resource misallocation.
How does the degree of digital integration of the industry promote the degree of industry collaboration, and whether the level of regional collaborative configuration can become a bridge between digitization and industry collaboration? This still needs to be further tested for the mediating effect. The test results are as follows Table 5.
The mediating effect analysis (Table 5) confirms regional coordination (ZC) as a partial mediator between ED and BC. In Model (1), ED directly impacts BC (coefficient = 0.537, p < 0.01). Model (2) shows that ED also positively affects ZC (coefficient = 1.172, p < 0.01), suggesting that digitization improves regional coordination. In Model (3), when ZC is included as a mediator, the direct effect of ED on BC decreases to 0.271 (p < 0.05), confirming that regional coordination partially mediates the relationship between digital integration and industry synergy. The positive impact of ZC on BC (coefficient = 0.227, p < 0.01) further supports this mediating role.
To address potential endogeneity concerns arising from reverse causality and unobserved confounders, this study employs a multi-methodological approach to ensure estimator consistency and inferential validity. Specifically, the analysis utilizes the first lag of digital economy development (L_ED) as an instrumental variable, leveraging its temporal precedence to satisfy exclusion restrictions while maintaining strong relevance with current digitalization measures. To enhance estimation robustness, we adopt a two-pronged strategy: (1) systematically screening observations at 1% thresholds to mitigate outlier distortion, and (2) applying weighted least squares regression to account for cross-sectional heterogeneity. Results from Tables 6, 7 consistently confirm that L_ED significantly and positively affects industry resilience, validating the robustness of the causal findings.
Based on the dual fixed-effect model and the intermediary model, the above study analyzed the influence mechanism of digital integration on industrial synergy, and controlled for individual and temporal heterogeneity. However, these models fail to fully account for interregional spatial dependencies. In order to further explore the spillover effect and spatial interaction of digitalization on regional synergy, this paper introduces a spatial econometrics model to capture the impact of spatial dependence on synergy more comprehensively. Specifically, this paper uses the economic geography nested matrix as the spatial weight matrix, which can better reflect the internal relationship of inter-provincial traffic, industrial layout and resource allocation than the traditional matrix, and effectively reveal the transmission and feedback mechanism of inter-regional cooperation. Therefore, the spatial Durbin model (SDM) will be used to further analyze the spatial dynamics of regional coordinated development.
In this study, the synergy resilience of the agriculture and transportation industry is selected as the core index, and the spatial econometric model is used to verify its spatial dependence between regions. Through the analysis of the global Moran’s I index, combined with the definition of the spatial weight matrix, this study systematically tests the industry’s integration synergy effect and its spatial autocorrelation characteristics. Spatial autocorrelation analysis (Table 8 and Figure 5) confirms a significant positive spatial correlation in industrial synergy resilience, with clear regional clustering patterns.
In the two-year data, most provinces are clustered in the first and third quadrants, indicating clear high–high and low–low spatial agglomeration, suggesting positive spatial dependence between regions. Eastern coastal provinces like Jiangsu, Shanghai, and Zhejiang are mainly in the first quadrant, showing high synergy and driving surrounding areas through spatial spillover. In contrast, western provinces like Sichuan, Xinjiang, and Gansu are mostly in the third quadrant, reflecting low synergy resilience and strong regional spatial clustering (Table 9).
From a trend perspective, by 2022, compared to 2012, the high-high agglomeration in the eastern region has strengthened, while the low-low agglomeration in the western region has become more concentrated. This indicates that the eastern region’s advantages in digital integration and infrastructure are expanding, while the western region’s low-level synergy remains stable. As digital technology and transportation facilities continue to improve nationwide, the eastern region’s advanced experiences are gradually spreading westward, though regional resource and infrastructure differences mean this process will take time.
In practical empirical studies, the selection of models usually depends on the Lagrange multiplier (LM) test, which is a widely used model selection method. According to the LM test results in Table 10 of this study, although the traditional LM test is not significant, the robust LM-Error and robust LM-Lag are both significant (p < 0.05), indicating that the spatial error effect and the spatial lag effect are significant after controlling the other effect. Although this result does not conform to the traditional judgment rules, it has been theoretically and empirically supported in spatial econometric research. In this context, the choice of SDM is a reasonable choice because the SDM model can simultaneously deal with spatial errors and spatial lag effects and capture the complex spatial correlation in the data (Elhorst, 2010; Anselin et al., 1996).
After the LM test, the LR test is performed to test whether the SDM model degenerates into the SAR model or the SEM model. The LR test results showed that for the LR test, the LR chi2 value was 28.16, and the p value was 0.0031. Since the p value is less than 0.05, the null hypothesis can be rejected, which indicates that the model, both including individuals and ID FE, is more appropriate than the model containing only individual effects. Similarly, the LR chi2 value of the LR test is 626.10, and the p value is 0.0000, which is far less than 0.01. Therefore, the null hypothesis is also rejected, indicating that the model containing individual and ID FE can better fit the data than the model containing only ID FE. Therefore, this paper chooses the double fixed effect space Durbin model.
Based on previous analysis, the spatial Durbin model (SDM) was employed to examine the effects of digital integration in agriculture and transportation on collaborative resilience. Table 11 presents the SDM results, including both direct effects and spatial spillovers, while Table 12 further dissects these effects, illustrating how regional and adjacent factors influence the synergic resilience of agriculture and transportation.
Table 13 displays the regression results for both OLS and SDM models. The OLS model indicates that ED and HZ have significant positive impacts on the dependent variables. After incorporating spatial factors, the SDM model reveals a spatial spillover effect. The positive impact of ED remains consistent in the SDM model (0.260), while the coefficient of ZC increases significantly (0.381), suggesting that spatial interactions enhance its positive effect. RD exhibits a negative effect (−0.000), indicating spatial competition. The spatial lag term (WX) shows that ED in neighboring areas has a significant positive spillover effect (0.483), whereas ZC and RD exhibit negative spillover effects (−0.495 and −0.000, respectively). The spatial autocorrelation coefficient (rho = 0.160) is significant, confirming spatial dependence in the model.
Table 11 details the direct, indirect, and total effects of each factor. ED has a positive direct effect within this province (0.274) and a significant positive spillover effect on neighboring provinces (0.609), resulting in a total effect of 0.884, which demonstrates that its influence extends beyond provincial boundaries. ZC has a positive direct effect within the province, but its negative spillover effect leads to a negative total effect. RD’s direct effect is close to zero, with a weak negative spillover effect, keeping the total effect negative. HZ exhibits a small positive spillover effect, contributing to an overall positive total effect.
In order to ensure the robustness of the model results, this paper considers the robustness test of the replacement space weight matrix and explanatory variables lagging one stage, and the specific results are as follows.
In this paper, the spatial economic distance matrix is used, which reflects the strength of economic ties between regions, but may ignore the spatial correlation caused by pure geographical proximity. In order to verify the robustness of the model, the spatial distance matrix is replaced to reflect the influence of geographical proximity on the dependent variable more directly. The regression results after replacement show that the coefficient of ED is 0.352 at the significance level of 1%, which is consistent with the above results, indicating that the model estimation results are robust and not significantly affected by the weight matrix replacement (Table 14).
In order to verify the stability of the model, the robustness test was performed with a one-stage lag. The results show that both the direct effect and the spatial spillover effect of the ED lag term are significantly positive, which indicates that the driving effect on the province and its neighboring areas is stable. The direct effect of ZC is positive, while the spatial spillover effect is negative, which indicates that its cross-regional negative effect persists. The spatial autocorrelation parameter (rho) is significantly positive, which further supports the stability of the spatial structure. The error variance of the model is small, indicating that the lag processing does not significantly affect the estimation accuracy.
The study identifies a prevalent pattern of “high coupling yet low coordination” between agricultural and transportation resilience. Despite the strong interdependence, practical synergy remains limited due to disparities in digital infrastructure, ineffective resource allocation, and fragmented policy frameworks. Addressing these issues requires targeted investments in digital infrastructure, improvements in regional digital literacy, and integrated policy frameworks. Such strategies would effectively translate strong coupling into genuine coordination, thereby facilitating sustainable synergy between agriculture and transportation industries.
The empirical analysis confirms the significant positive impact of digital transformation on the resilience synergy between agricultural and transportation sectors. Notably, the digital economy significantly enhances resilience by improving resource allocation efficiency. However, unregulated rapid growth may lead to resource misallocation, underscoring the importance of robust policy management. Policymakers should therefore adopt strategies that balance rapid digital infrastructure growth with regulatory mechanisms to ensure efficient resource allocation and optimal synergy outcomes.
The mediating effect analysis further demonstrates that regional resource coordination substantially mediates the influence of digital transformation on industry resilience. Approximately half of the effect of digitalization on resilience is realized through improved regional resource coordination. Consequently, enhancing mechanisms for regional resource coordination and optimizing the efficiency of resource distribution through digital technology applications should be central to policy efforts. This approach would effectively strengthen the foundational linkages between agriculture and transportation, promoting comprehensive and sustainable development.
The spatial analysis highlights significant regional disparities in digital development and its spillover effects. Advanced eastern provinces exhibit robust positive spatial spillovers due to their strong digital infrastructure, positively influencing neighboring provinces’ resilience. In contrast, central and western regions show limited spillover effects, reflecting inadequate digital integration and infrastructure deficits. Policies should target enhanced regional connectivity, digital infrastructure upgrades in less-developed regions, and interregional collaborative initiatives to bridge digital and spatial gaps effectively.
Based on the panel data of 31 provinces in China from 2012 to 2022, this study constructed a comprehensive index system of green rural industry resilience, green transportation infrastructure resilience and industrial synergy, and analyzed the relationship between digital economy development and agricultural transportation industry synergy using multiple models. The results show that digital transformation markedly strengthens synergistic resilience between agriculture and transportation industries primarily through enhanced resource allocation efficiency and improved regional coordination. Nonetheless, considerable regional disparities persist, with eastern regions outperforming central and western areas significantly due to advanced digital infrastructure, effective resource integration, and proactive policy support. These disparities are compounded by limited spatial spillover effects in less-developed regions, underscoring the necessity of differentiated regional policy strategies.
Eastern provinces: Empirical evidence reveals substantial positive spatial spillover effects, particularly pronounced in the eastern provinces. Consequently, targeted policy measures for these regions should emphasize further investments in advanced digital infrastructure, specifically by enhancing intelligent logistics networks and promoting comprehensive digitalization in agriculture. To effectively implement these improvements, provincial governments should establish clear industry-specific digital standards and regulatory frameworks to prevent resource misallocation and inefficient investments. Additionally, structured knowledge-sharing platforms should be developed to systematically transfer best practices and technological advancements to neighboring provinces, thereby facilitating a broader regional transformation.
Central and western provinces: In light of the identified weaknesses in digital infrastructure and insufficient regional coordination in central and western provinces, initial policy measures should focus on addressing rural broadband infrastructure gaps and systematically enhancing the development of intelligent logistics networks. Furthermore, establishing clear regional coordination mechanisms—such as interprovincial digital resource sharing platforms and structured policy communication channels—can improve resource allocation efficiency. To practically harness technological spillovers, these provinces should proactively implement policies that facilitate direct collaboration and structured technology transfer arrangements with digitally advanced eastern provinces, thus effectively addressing spatial disparities and fostering resilient cross-sectoral synergy.
National digital governance and integration strategy: To address the observed regional differences in digital transformation, investments in digital infrastructure should be standardized to ensure balanced growth across provinces. Targeted financial incentives, including public-private partnerships, smart agriculture subsidies, and digital infrastructure grants, should be established to support less developed regions. Existing national initiatives, such as digital economy development plans, should be leveraged to enhance regional coordination and ensure that digital transformation policies effectively promote cross-sectoral resilience.
This study highlights regional differences in the digital transformation of agriculture and transportation industries. Future research should focus on the long-term effects of digital transformation, especially in balancing digital progress with environmental protection to ensure sustainable industry development. The mediating effect analysis emphasizes the role of regional collaboration in enhancing industry resilience, and future studies can explore other mediators, such as technological innovation and market access, to fully understand the digitization mechanism in industry coordination.
The original contributions presented in the study are included in the article/supplementary material, further inquiries can be directed to the corresponding author.
GZ: Conceptualization, Funding acquisition, Methodology, Supervision, Validation, Visualization, Writing – review & editing. JC: Conceptualization, Data curation, Formal analysis, Investigation, Methodology, Project administration, Resources, Software, Writing – original draft, Writing – review & editing. JX: Conceptualization, Methodology, Resources, Supervision, Visualization, Writing – review & editing.
The author(s) declare that no financial support was received for the research and/or publication of this article.
The authors declare that the research was conducted in the absence of any commercial or financial relationships that could be construed as a potential conflict of interest.
The author(s) declare that no Gen AI was used in the creation of this manuscript.
All claims expressed in this article are solely those of the authors and do not necessarily represent those of their affiliated organizations, or those of the publisher, the editors and the reviewers. Any product that may be evaluated in this article, or claim that may be made by its manufacturer, is not guaranteed or endorsed by the publisher.
Adjetey-Bahun, K., Birregah, B., Châtelet, E., and Planchet, J. L. (2016). A model to quantify the resilience of mass railway transportation systems. Reliabil. Eng. Syst. Saf. 153, 1–14. doi: 10.1016/j.ress.2016.03.015
Adve, V. S., Wedow, J. M., Ainsworth, E. A., Chowdhary, G., Green-Miller, A., and Tucker, C. (2024). AIFARMS: artificial intelligence for future agricultural resilience, management, and sustainability. AI Mag. 45, 83–88. doi: 10.1002/aaai.12152
Anselin, L., Bera, A. K., Florax, R., and Yoon, M. J. (1996). Simple diagnostic tests for spatial dependence. Reg. Sci. Urban Econ. 26, 77–104. doi: 10.1016/0166-0462(95)02111-6
Ariken, M., Zhang, F., Chan, N. W., and Kung, H. T. (2021). Coupling coordination analysis and spatio-temporal heterogeneity between urbanization and eco-environment along the silk road Economic Belt in China. Ecol. Indic. 121:107014. doi: 10.1016/j.ecolind.2020.107014
Bi, W., MacAskill, K., and Schooling, J. (2023). Old wine in new bottles? Understanding infrastructure resilience: foundations, assessment, and limitations. Transp. Res. Part D: Transp. Environ. 120:103793. doi: 10.1016/j.trd.2023.103793
Chang, H., Ding, Q., Zhao, W., Hou, N., and Liu, W. (2023). The digital economy, industrial structure upgrading, and carbon emission intensity——empirical evidence from China’s provinces. Energ. Strat. Rev. 50:101218. doi: 10.1016/j.esr.2023.101218
Chen, X., An, Y., Pan, W., Wang, Y., Chen, L., Gu, Y., et al. (2024). Dynamic transfer and driving mechanisms of the coupling and coordination of agricultural resilience and rural land use efficiency in China. J. Geogr. Sci. 34, 1589–1614. doi: 10.1007/s11442-024-2262-7
Cheng, Y., Zhang, Y., Wang, J., and Jiang, J. (2023). The impact of the urban digital economy on China’s carbon intensity: spatial spillover and mediating effect. Resour. Conserv. Recycl. 189:106762. doi: 10.1016/j.resconrec.2022.106762
Deng, H., Bai, G., Shen, Z., and Xia, L. (2022). Digital economy and its spatial effect on green productivity gains in manufacturing: evidence from China. J. Clean. Prod. 378:134539. doi: 10.1016/j.jclepro.2022.134539
Dong, B. X., Shan, M., and Hwang, B. G. (2022). Simulation of transportation infrastructures resilience: a comprehensive review. Environ. Sci. Pollut. Res. 29, 12965–12983. doi: 10.1007/s11356-021-18033-w
Du, C., and Wang, C. (2024). Digitization and carbon emissions: how does the development of China’s digital economy affect carbon intensity? Environ. Sci. Pollut. Res. 31, 26895–26915. doi: 10.1007/s11356-024-32758-4
Elhorst, J. P. (2010). “Spatial panel data models” in Handbook of applied spatial analysis. eds. M. M. Fischer and A. Getis (Berlin Heidelberg: Springer), 377–407.
Han, X., Fu, L., Lv, C., and Peng, J. (2023). Measurement and spatio-temporal heterogeneity analysis of the coupling coordinated development among the digital economy, technological innovation and ecological environment. Ecol. Indic. 151:110325. doi: 10.1016/j.ecolind.2023.110325
Hong, J., Huang, H., Wang, X., Dockerill, B., Ye, J., and Zhang, S. (2024). Structural effects of provincial digital economy on carbon emissions within China: a multi-region input-output based structural decomposition analysis. Sci. Total Environ. 934:173140. doi: 10.1016/j.scitotenv.2024.173140
Hou, G., Chen, S., and Han, Y. (2019). Traffic performance assessment methodology of degraded roadway links following hazards. J. Aerosp. Eng. 32:04019055. doi: 10.1061/(ASCE)AS.1943-5525.0001050
Ilalokhoin, O., Pant, R., and Hall, J. W. (2023). A model and methodology for resilience assessment of interdependent rail networks – case study of Great Britain’s rail network. Reliabil. Eng. Syst. Saf. 229:108895. doi: 10.1016/j.ress.2022.108895
Jin, M., Feng, Y., Wang, S., Chen, N., and Cao, F. (2024). Can the development of the rural digital economy reduce agricultural carbon emissions? A spatiotemporal empirical study based on China’s provinces. Sci. Total Environ. 939:173437. doi: 10.1016/j.scitotenv.2024.173437
Lamine, C. (2015). Sustainability and resilience in Agrifood systems: reconnecting agriculture, food and the environment. Sociol. Rural. 55, 41–61. doi: 10.1111/soru.12061
Lee, C., Yuan, Y., and Wen, H. (2022). Can digital economy alleviate CO2 emissions in the transport sector? Evidence from provincial panel data in China. Nat. Res. Forum 46, 289–310. doi: 10.1111/1477-8947.12258
Li, Y. (2023). A systematic review of rural resilience. CAER 15, 66–77. doi: 10.1108/CAER-03-2022-0048
Li, J., Dou, K., Wen, S., and Li, Q. (2021). Monitoring index system for sectors’ digital transformation and its application in China. Electronics 10:1301. doi: 10.3390/electronics10111301
Li, H., Jin, X., Liu, J., Feng, D., Xu, W., and Zhou, Y. (2022). Analytical framework for integrating resources, morphology, and function of rural system resilience—an empirical study of 386 villages. J. Clean. Prod. 365:132738. doi: 10.1016/j.jclepro.2022.132738
Li, Y., Song, C., and Huang, H. (2021). Rural resilience in China and key restriction factor detection. Sustain. For. 13:1080. doi: 10.3390/su13031080
Li, Y., Wang, S., Zhang, Y., and Du, G. (2024). The measurement of rural community resilience to natural disaster in China. Sci. Rep. 14:20322. doi: 10.1038/s41598-024-70719-6
Liang, C., Chen, X., and Di, Q. (2024). Path to pollution and carbon reduction synergy from the perspective of the digital economy: fresh evidence from 292 prefecture-level cities in China. Environ. Res. 252:119050. doi: 10.1016/j.envres.2024.119050
Liu, B., Luo, J., Zheng, K., Wu, F., and Zhao, X. (2023). How will industrial collaborative agglomeration affect the efficiency of regional green development? Front. Ecol. Evol. 11:11. doi: 10.3389/fevo.2023.1179004
Liu, L., Wang, H., Wang, Z., Ding, T., and Wu, M. (2024). The coupling coordination between digital economy and Green high-quality development of Industries in China’s the Yangtze River Economic Belt. Pol. J. Environ. Stud. 33, 3799–3812. doi: 10.15244/pjoes/178322
Luo, R., and Zhou, N. (2022). Dynamic evolution, spatial differences, and driving factors of China’s provincial digital economy. Sustain. For. 14:9376. doi: 10.3390/su14159376
Maria, M. C., Chereji, A. I., Smaranda, M. O., Camelia, M. D., and Alexandru, G. M. (2021). Challenges and opportunities for a sustainable agriculture in the context of the green and digital twin transition. How can we increase the resilience of the agriculture in the current context? Analele Universitatii din Oradea, Fascicula Ecotoxicologie, Zootehnie si Tehnologii în Industria Alimentara 20:43. doi: 10.1016/j.jhep.2021.11.018
Su, J., Su, K., and Wang, S. (2022). Evaluation of digital economy development level based on multi-attribute decision theory. PLoS One 17:e0270859. doi: 10.1371/journal.pone.0270859
Tubis, A. A., Koliński, A., and Poturaj, H. (2024). Digital maturity of logistics processes assessed in the areas of technological support for performance measurement, employees, and process management. Appl. Sci. 14:7893. doi: 10.3390/app14177893
Urruty, N., Tailliez-Lefebvre, D., and Huyghe, C. (2016). Stability, robustness, vulnerability and resilience of agricultural systems. A review. Agron. Sustain. Dev. 36:15. doi: 10.1007/s13593-015-0347-5
Wang, F., Wang, H., and Xiong, L. (2024). Does the digital economy exhibit multiplier effects? A case study on the optimization of agricultural production structure in rural digital economy. Nature Food. 22, 550–556. doi: 10.1038/s43016-024-01014-w
Wang, L., and Xue, W. (2024). Research on synergistic development between environment and industry in the Yellow River Basin. Pol. J. Environ. Stud. 33, 5303–5321. doi: 10.15244/pjoes/176558
Wu, Y., and Chen, S. (2023). Transportation resilience modeling and bridge reconstruction planning based on time-evolving travel demand during post-earthquake recovery period. Sustain. For. 15:12751. doi: 10.3390/su151712751
Xu, J., Wang, C., Yin, X., and Wang, W. (2024). Digital economy and rural household resilience: evidence from China. Agric. Econ. (Zemědělská ekonomika) 70, 244–263. doi: 10.17221/317/2023-AGRICECON
Yang, Z. (2024). Assessing the impacts of rainstorm and flood disaster for improving the resilience of transportation system. J. Adv. Transp. 2024:6687438. doi: 10.1155/2024/6687438
Yang, M., Jiao, M., and Zhang, J. (2022). Spatio-temporal analysis and influencing factors of rural resilience from the perspective of sustainable rural development. Int. J. Environ. Res. Public Health 19:12294. doi: 10.3390/ijerph191912294
Yang, Z., Sang, S., and Zhu, Y. (2024). Analysis of coupled coordination and spatial interaction effects between digital and tourism economy in the Yangtze River Delta region. PLoS One 19:e0307756. doi: 10.1371/journal.pone.0307756
Yi, Y., Cheng, R., Wang, H., Yi, M., and Huang, Y. (2022). Industrial digitization and synergy between pollution and carbon emissions control: new empirical evidence from China. Environ. Sci. Pollut. Res. 30, 36127–36142. doi: 10.1007/s11356-022-24540-1
Yu, J., Zhang, J., Zhou, M., and Cai, W. (2023). Impact of COVID-19 on the comprehensive resilience of rural areas—a case study of Jilin Province of China. Sustain. For. 15:3152. doi: 10.3390/su15043152
Zhang, H., Li, J., and Quan, T. (2023). Strengthening or weakening: the impact of an aging rural workforce on agricultural economic resilience in China. Agriculture 13:1436. doi: 10.3390/agriculture13071436
Zhang, M., and Yin, S. (2023). Can China’s digital economy and green economy achieve coordinated development? Sustain. For. 15:5666. doi: 10.3390/su15075666
Zhou, J., and Hou, Q. (2021). Resilience assessment and planning of suburban rural settlements based on complex network. Sustain. Prod. Consum. 28, 1645–1662. doi: 10.1016/j.spc.2021.09.005
Keywords: agricultural industry resilience, transportation resilience, digital economy, mediating effect, coupling coordination degree model, spatial Durbin model
Citation: Zhang G, Chai J and Xie J (2025) Digital transformation and agricultural transportation synergy resilience: path and spatial effects. Front. Sustain. Food Syst. 9:1564443. doi: 10.3389/fsufs.2025.1564443
Received: 21 January 2025; Accepted: 21 March 2025;
Published: 16 April 2025.
Edited by:
Siphe Zantsi, Agricultural Research Council of South Africa (ARC-SA), South AfricaReviewed by:
Feng Hu, Zhejiang Gongshang University, ChinaCopyright © 2025 Zhang, Chai and Xie. This is an open-access article distributed under the terms of the Creative Commons Attribution License (CC BY). The use, distribution or reproduction in other forums is permitted, provided the original author(s) and the copyright owner(s) are credited and that the original publication in this journal is cited, in accordance with accepted academic practice. No use, distribution or reproduction is permitted which does not comply with these terms.
*Correspondence: JunJie Chai, MjMyMDIwOTcwMThAc3R1Lnh1c3QuZWR1LmNu
Disclaimer: All claims expressed in this article are solely those of the authors and do not necessarily represent those of their affiliated organizations, or those of the publisher, the editors and the reviewers. Any product that may be evaluated in this article or claim that may be made by its manufacturer is not guaranteed or endorsed by the publisher.
Research integrity at Frontiers
Learn more about the work of our research integrity team to safeguard the quality of each article we publish.