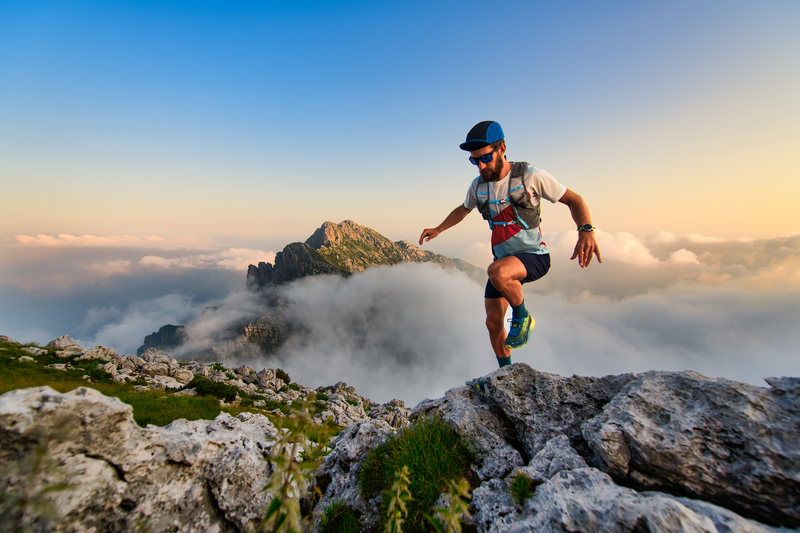
95% of researchers rate our articles as excellent or good
Learn more about the work of our research integrity team to safeguard the quality of each article we publish.
Find out more
ORIGINAL RESEARCH article
Front. Sustain. Food Syst. , 19 March 2025
Sec. Agricultural and Food Economics
Volume 9 - 2025 | https://doi.org/10.3389/fsufs.2025.1552637
Introduction: Technological change is a mega trend that drives sustainable development in the agrifood sector globally. The introduction of BanQu, a blockchain-enabled platform, aimed to address challenges like lack of transparency, side-selling, and unfair pricing in Uganda's barley value chain, but its acceptance has been slow. While blockchain adoption has thrived in developed countries and large supply chains, empirical evidence on its uptake among smallholder farmers in Sub-Saharan Africa, especially Uganda, remains limited. This study investigates determinants of smallholder barley farmers' intentions to accept blockchain technology (BCT) in Uganda.
Methods: The study utilized the second extension of the Technology Acceptance Model (TAM2), customized to fit Uganda's context. Quantitative data were gathered from 245 farmers in Bukwo and Kween, the two leading barley-producing districts in eastern Uganda. The data were analyzed using Partial Least Squares Structural Equation Modeling (PLS-SEM).
Results and discussion: The study showed that perceived usefulness (PU) significantly influenced behavioral intention (BI) and shaped perceived ease of use (PEU). Subjective norms (SUN) and voluntariness (BV) enhanced PU, while perceived behavioral control (PBC) improved PEU. Notably, BCT relevance (BR) directly influenced BI, bypassing PU. These findings provide fresh insights into rural technology adoption, highlighting PU's influence on PEU and BV's role in shaping PU. The study recommends emphasizing BCT benefits such as reducing transaction costs, leveraging social networks, and addressing resource gaps to boost acceptance. This study advances understanding of BCT adoption among smallholder farmers in emerging economies like Uganda.
Technological innovations are critical drivers of sustainable development in the agrifood sector, reshaping productivity, market access, and income security for smallholder farmers (Yuan and Sun, 2024). Among these innovations, blockchain technology (BCT) stands out for enhancing transparency, traceability, and trust in agricultural value chains (Kamilaris et al., 2019). In Uganda's eastern barley value chain, as in other countries, smallholder farmers face multiple challenges in executing their value chain operations, including limited access to markets, exploitation by middlemen, opaque pricing systems, and small gross margins (Piabuo et al., 2020). Existing interventions, such as the formation of farmer groups, producer associations, and contract farming, have had limited success in effectively addressing these challenges. To tackle these persistent issues, a collaborative effort involving Company B (pseudo name), the Ugandan government, and other stakeholders introduced BanQu (BankYou), a blockchain-enabled platform, in 2019 (Kagorora et al., 2021).
Blockchain's capabilities of secure, decentralized, and immutable record-keeping addresses challenges like information asymmetry and distrust in farmer-buyer transactions (Panwar et al., 2023). BanQu operates by having smallholder farmers connect their phones to the BanQu blockchain software app and allows them to register and store their value chain activities' data, and receive short message services (SMSs) upon each transaction. The SMSs received contain immutable information on prices offered to farmers at the end of season, input costs (seeds, fertilizers, packing materials), Company B field staff extension days as per farmers' location, payment details after supply (African News Agency, 2019). BanQu BCT allows farmers lacking financial resources to access production inputs on credit, with payment deferred until they deliver produce to Company B's buying centers. The platform provides multiple payment options, including cash, mobile money, or bank transfers, and records all transactions on an immutable, globally accessible ledger (Further Africa, 2019; Kumarathunga et al., 2022). These records can be printed and used to secure credit or apply for financial support, such as Uganda's parish development model (Tripoli and Schmidhuber, 2020). By reducing transaction costs, connecting farmers with buyers, and ensuring transparent, immutable records, BanQu promotes fair trade and loyalty in the value chain (Bhusal, 2021; Lin et al., 2020). It also secures farmers' economic identities and transaction histories, enhancing global visibility (Kshetri, 2021).
However, blockchain's widespread acceptance remains limited. For instance, since the introduction of BanQu BCT in 2019, only one of Uganda's four regions (eastern) has adopted it, and its future use remains uncertain. Even within this region, not all farmers produce and supply barley through the BanQu BCT platform, and the factors influencing this adoption pattern remain unknown. While BCT adoption has been successful in developed countries and within the supply chains of large firms (Ballantyne and Addison, 2020), there is limited empirical evidence on its adoption among smallholder farmers in Sub-Saharan Africa (SSA), particularly in Uganda. This study addresses this gap by examining the determinants of smallholder barley farmers' behavioral intentions to adopt BanQu BCT in eastern Uganda. Additionally, prior studies, such as Molina-Maturano et al. (2021), have primarily focused on BCT adoption in its later stages, once the technology has already gained significant traction. However, little attention has been given to the critical role of farmers' initial intentions in adopting BCT, despite early acceptance being a key factor for widespread adoption.
Acceptance of agricultural technologies among farmers has been reportedly low due to challenges such as high production costs, limited digital literacy, skepticism about long-term benefits, and technological complexity (Akella et al., 2023). These challenges are further compounded by social and behavioral factors, particularly in rural smallholder farming communities in Sub-Saharan Africa. When the costs of adoption outweigh the perceived benefits, many farmers hesitate to embrace new technologies. For instance, financial constraints have been found to reduce the adoption of inorganic fertilizers and improved seeds in most Sub-Saharan African countries (Yaghi et al., 2023). Likewise, low digital literacy has been found to negatively impact smallholder farmers' perceptions of mobile-based extension services and digital marketplaces in Tanzania (Hassani et al., 2023), while perceived complexity has been identified as a barrier to adopting most internet based agricultural innovations (Managa et al., 2023).
Blockchain platforms like BanQu have shown potential to strengthen farmer-buyer relationships and reduce side-selling (Bedford, 2018; Choruma et al., 2024). Research on blockchain applications in agriculture highlights its ability to enhance transparency and traceability in supply chains (Giganti et al., 2024), fostering greater trust between farmers and buyers (Sendros et al., 2022). This increased trust helps discourage side-selling by ensuring that all transactions are securely recorded and verifiable. Moreover, as the demand for sustainable and inclusive agrifood systems grows globally, blockchain technology plays a crucial role in ensuring product provenance (Thejaswini and Ranjitha, 2020) while also bridging the digital divide that often excludes smallholder farmers from participating in and gaining visibility within global value chains (Kos and Kloppenburg, 2019).
This paper addresses this knowledge gap by examining the determinants of smallholder barley farmers' behavioral intentions to adopt BanQu BCT in eastern Uganda. The study integrates the Theory of Planned Behavior (TPB) with the second extension of the Technology Acceptance Model (TAM2) to provide an in-depth understanding of the factors influencing acceptance behavior among these farmers. This study's novelty lies in its unique contribution to BCT adoption literature in a developing country context, such as Uganda, and its focus on last-mile stakeholders—smallholder farmers—in a globally significant emerging value chain like barley. Furthermore, incorporating contextualized constructs, such as farmers' perception of their image in relation to blockchain technology adoption, offers a valuable theoretical contribution to TAM research. While this construct has been examined in other fields like education (Izuagbe et al., 2019) and business (Bashir et al., 2022), its role in agriculture has received little attention. In previous agricultural studies, image has often been treated as a component of social influence (Mishra et al., 2024), rather than being explored for its direct impact on farmers' perceived usefulness of a technology, especially in rural settings where self-image holds significant value.
The Theory of Planned Behavior (TPB) informs this study. Developed by Ajzen in 1985 (Ajzen, 1985), TPB posits that, intentions, shaped by attitudes, subjective norms, and perceived behavioral control (PBC), predict behavior (Ajzen, 1991). While TPB has been widely applied across domains, its use in studying BCT acceptance among smallholder farmers in developing countries is limited. To gain an in-depth understanding of the smallholder farmers' behavioral intentions, the Technology Acceptance Model (TAM) and its second extension, TAM2, provide a robust framework for analyzing technology acceptance (Davis, 1989; Venkatesh and Davis, 2000).
The TAM is more suitable for this study's context than recent models like Unified Theory of Acceptance and Use of Technology (UTAUT) due to its simplicity, focus on individual decision-making, and adaptability. TAM's core constructs, that is, Perceived Usefulness (PU) and Perceived Ease of Use (PEU), provide a clear framework for analyzing how farmers evaluate blockchain's benefits and usability (Davis, 1989). Unlike UTAUT, which emphasizes organizational and social influences, TAM is more applicable in contexts where technology adoption is voluntary and individually driven, such as smallholder farming (Venkatesh and Bala, 2008). Furthermore, TAM provides flexibility for incorporating factors like farmers' image and result demonstrability, which are particularly relevant in understanding how visible benefits and self-image in society influence blockchain acceptance (Moore and Benbasat, 1991). Given blockchain's complexity and its nascent application in agriculture, a streamlined and adaptable model like TAM offers a better fit for understanding farmers' behavioral intentions.
The traditional TAM suggests that behavioral intention (BI) to accept a technology is influenced by perceived usefulness (PU) and perceived ease of use (PEU). However, due to novelty and emergingt nature of BCT, PU and PEU may not necessarily underpin farmers' behavioral intention to accept, or later on predict potential for adoption of BCT (Marangunić and Granić, 2015). There is need therefore to understand the antecedents of farmers' perceptions, especially regarding BCT usefulness and ease of use. The TAM2 expands this model by integrating external factors like subjective norms (SUN), farmer image (FI), attitudes (FA), result demonstrability (BD), BCT relevance to the barley value chain (BR), barley output quality (BQ), and voluntariness (BV), which shape PU (Ngubelanga and Duffett, 2021; Salloum et al., 2019). Ease of use is less relevant to this study, as farmers enrolled in the BCT app primarily interact with it through SMS notifications after transactions. Thus, only perceived behavioral control (PBC) is used to underprop PEU. By integrating TAM2's tailored insights into technology acceptance, this study addresses gaps in understanding BCT acceptance within Uganda's barley value chain, offering theoretical and practical implications for rural technology adoption.
Subjective norms refer to the social pressure individuals feel to perform or not perform a specific behavior, influenced by significant others like family, peers, or community leaders (Ajzen, 1991). For farmers, subjective norms involve influences from peers, agricultural advisors, or local authorities, shaping acceptance decisions. Advocacy by respected peers may motivate acceptance to align with social expectations. Bashir et al. (2022) found subjective norms indirectly influenced intentions through PU. Similarly, Ren and Zhong (2022) observed that SUN directly impacted Chinese farmers' intentions to adopt straw returning technology. Other studies (Dissanayake et al., 2022; Massoro and Adewale, 2019) highlighted SUN's significant influence on smallholders' technology acceptance in rural contexts. This study examines both direct and indirect effects of SUN on farmer intentions, proposing:
H1a: Subjective norm significantly and positively influences behavioral intention to accept BCT.
H1b: Subjective norm significantly and positively influences perceived usefulness.
Attitudes (FA), reflecting an individual's positive or negative evaluation of a technology, significantly acceptance intentions (Davis et al., 1989). While TAM often links attitudes to BI, for new technologies like BCT, initial beliefs may instead shape PU (Suresh et al., 2022). Hence:
H2: Farmer attitudes significantly and positively affect perceived usefulness of BCT.
Moore and Benbasat (1991) define image as the extent to which using an innovation enhances one's social status. For blockchain, this refers to farmers perceiving adoption as elevating their societal standing, influencing PU and acceptance. While much research emphasizes the technology's image (Izuagbe et al., 2019), few studies explore user image (farmers' status) and its influence on PU. This study hypothesizes:
H3: Perceived farmer image (FI) has a positive and significant influence on PU of BCT.
Result demonstrability (BD), reflecting the tangible outcomes of a technology, is a key determinant of PU. This study considers farmers' perceptions of BCT results in barley value chain activities. Soodan et al. (2024) found BD positively influenced PU, influencing acceptance intentions. Similarly, Dai and Cheng (2022) identified a positive relationship between BD and PU. This study hypothesizes:
H4: Barley value chain result demonstrability (BD) from using BCT positively PU of BCT.
Perceived usefulness is influenced by output quality (BQ), reflecting the user's belief that a technology enhances outcome quality. In TAM2, Venkatesh and Davis (2000) proposed a direct relationship between BQ and PU, supported by four field studies. Similarly, Moreno et al. (2017) applied TAM2 to e-learning adoption and hypothesized a direct positive effect of BQ on PU, though their findings did not support this. In TAM3, Venkatesh and Bala (2008) introduced a moderating role for BQ, suggesting it strengthens the link between job relevance (BR) and PU when output quality is high. Zaineldeen et al. (2020) also emphasized BQ in evaluating BR and perceived gains from technology. Building on this, the current study hypothesizes:
H5: Barley output quality (BQ) has a significant and positive moderating effect on the relationship between BCT relevance and perceived usefulness of BCT.
In reference to Venkatesh and Davis's (2000) definition, job relevance (in this case BCT relevance to the value chain, BR) is the extent to which barley farmers perceive BCT as applicable to their value chain activities, from input sourcing to output delivery. Zarafshani et al. (2020) found BR significantly influenced PU in technology acceptance among agricultural teachers in Iran. Similarly, Kemp et al. (2019) defined relevance as job-fit, or a technology's ability to enhance user performance, confirming its positive effect on PU in educational technologies. While these studies highlight the importance of BR, its application in the agrifood sector is limited, and few studies explore its direct effect on BI. This study addresses these gaps by hypothesizing that:
H6: BCT relevance to the barley value chain (BR) has a positive and significant effect on perceived usefulness.
H7: BCT relevance to the barley value chain has a positive and significant effect on behavioral intention.
Perceived behavioral control (PBC), from the TPB, refers to individuals' perceived ability to control technology use, influenced by factors like access to resources, skills, and support (Ajzen, 1988). PBC affects PEU; when users feel more control, they face fewer challenges, making technology easier to use. PBC is shaped by self-efficacy (confidence in one's abilities) and supporting resources, such as mobile phone ownership, land control, and network stability. This study hypothesizes:
H8: Perceived behavioral control (PBC) significantly and positively affects PEU of BCT.
Perceived usefulness (PU) refers to the extent to which users believe a technology enhances their performance (Davis, 1989). In this study, PU reflects barley farmers' belief that BCT improves productivity, gross margin, reduces transaction costs, and other production costs. PU significantly influences PEU and BI. While traditional TAM suggests PEU influences PU (Davis, 1989; He et al., 2018), this study explores the inverse relationship due to rural farmers' skepticism toward disruptive technologies like blockchain. When farmers find a technology useful, they are more likely to perceive it as easy to use and adopt. Therefore, the study hypothesizes:
H9: Perceived usefulness of BCT (PU) significantly and positively influences (PEU) of BCT.
H10: Perceived usefulness of BCT positively and significantly influences BI to accept BCT.
Perceived Ease of Use (PEU) is the extent to which an individual believes using a technology requires little effort (Ajzen, 2002). In this study, PEU reflects barley farmers' perception of BCT's simplicity, usability, and learnability, which significantly affects their acceptance intentions. Previous studies have shown PEU's direct influence on attitudes (Mishra et al., 2024) or its effect on BI mediated through PU (Widiar et al., 2023). However, this study posits that PEU directly affects BI, as farmers who find BCT easy to use are more likely to form favorable adoption intentions.
H11: Perceived ease of use (PEU) has a positive and significant effect on behavioral intention (BI) to accept BCT.
Voluntariness (BV) is the perception of technology use as optional rather than mandatory (Moore and Benbasat, 1991). While TAM3 typically views voluntariness as a moderator between SUN and BI (Marikyan and Papagiannidis, 2023), Park et al. (2022) found it directly affects BI in the health sector. When individuals feel free to choose acceptance, they are more likely to perceive the technology as useful, influencing their acceptance intentions. Therefore, this study treats voluntariness as both a moderator, as per TAM3, and a direct influencer of PU, proposing that:
H12: Voluntariness to accept BCT (BV) significantly and positively PU of BCT.
H13: Voluntariness to accept BCT positively and significantly moderates the relationship between SUN and BI.
The hypotheses and their proposed relationships are illustrated in the research model shown in Figure 1.
As highlighted by Xiong et al. (2020), a notable gap in the literature is the limited application of the TAM to “last-mile” stakeholders, such as smallholder farmers, within most crop value chains. Additionally, there is a lack of empirical research addressing the specific context of Uganda's barley farming sector.
This study was conducted in the Sebei sub-region of eastern Uganda, which comprises three districts, 54 sub-counties, and 295 parishes. The study focused on Bukwo and Kween districts, which are located along the Mount Elgon slopes on the eastern border with Kenya. These two districts were selected due to their status as the leading barley producers in the region (Cheptoyek, 2018). Bukwo district has 21 sub-counties and 107 parishes, while Kween district has 19 sub-counties and 101 parishes. Given the large number of sub-counties and parishes, a multistage sampling approach was employed, combining both purposive and systematic random sampling techniques to ensure representative data collection while maintaining feasibility (Rahman et al., 2022; Ramanujan et al., 2022).
A quantitative research approach was adopted, and a cross-sectional survey design was used. Data were collected through structured questionnaires administered by trained enumerators. The multistage sampling procedure involved four key stages. In the first stage, purposive sampling was used to select Bukwo and Kween, the two highest barley-producing districts. In the second stage, two sub-counties from each district were purposively selected based on their high barley output and large farming populations. Specifically, Cheminy and Kwosir sub-counties were chosen in Bukwo district, while Kortek and Amanang sub-counties were selected in Kween district. In the third stage, two parishes were purposively selected from each sub-county, again based on their significant barley production and farmer population. The selected parishes were Chesimat and Kubobei in Cheminy sub-county; Kubulwo and Sosho in Kwosir sub-county; Serere and Kamwam in Kortek sub-county; and Kere and Tuikat in Amanang sub-county.
In the fourth and final stage, probability proportional to size systematic random sampling was used to select farmers while accounting for differences in parish sizes. According to records from Company B, district and sub-county officers, the total population of barley farmers in the two districts was estimated at 1,600, with 900 in Kween and 700 in Bukwo. However, eligibility for the study was limited to farmers who had interacted with blockchain technology (BCT) since its introduction in 2019, which reduced the population to 800 farmers. Additionally, farmers cultivating more than five hectares were excluded, as they did not meet the FAO (2017) definition of smallholder farmers. To ensure comparability of experiences before and after BCT introduction, only farmers with at least 5 years of barley-growing experience were included, further reducing the target population to 630 farmers.
With the eligible target population ascertained, Yamane's (1967) formula was employed to calculate the sample size as: where; n is sample size, N is the population size (630), and e is the margin of error (±5%). Substituting these values, the sample was calculated as: = 244.66 ~ 245. To ensure randomization in the final selection of respondents, systematic random sampling was used to distribute the sample across the selected parishes (Mostafa and Ahmad, 2018). Farmer lists from Company B field officers provided the number of eligible farmers per parish. Then, the sampling interval (K) was calculated as K = N/n, where N is the number of eligible farmers in a given parish and n is the sample size assigned to that parish. Given the variability in parish sizes, the sampling interval differed across parishes. Finally, a random starting point was selected within each parish using simple random sampling, and then every K-th farmer was chosen until all 245 questionnaires were administered.
The research underwent a thorough ethical review and was approved by the ethics committees of two universities—one from Kenya (Egerton University, Approval No. EUISERC/APP/355/2024), and one from Uganda (Uganda Christian University, Approval No. UCUREC-2024-1000). Furthermore, the study received approval from Uganda's National Council for Science and Technology to be conducted in the designated region. To uphold voluntary participation, farmers selected for the study were provided with consent forms before each interview, enabling them to formally consent to their involvement.
The dependent variable was behavioral intention to accept BCT while the explanatory variables were perceived usefulness (PU) and perceived ease (PEU) of use of BCT. But since PU and PEU have their antecedents, they are also regarded as dependent variables when they are not affecting BI. The moderating variables of perceived barley output quality (BQ) and voluntariness in accepting BCT (BV) were also explored. All the independent variables were hypothesized to have a positive influence on the dependent variables, as stated in Section 2. Due to multiple variables and antecedents affecting the variables, this study modeled the second extension of TAM, using partial least squares structural equation modeling (PLS-SEM) to determine farmers' behavioral intention to accept BCT. The PLS-SEM was preferred because of its wide applicability in empirical studies of human and social behavior, higher prediction capacity and ability to use reflection on observed constructs (Manley et al., 2021; Shmueli et al., 2019).
Prior to data collection, the questionnaire consisted of 53 items that were based on both dependent and independent variables, which were measured on a 5-point Likert scale. The questionnaire was validated through experts review at the faculty of Agriculture, Egerton University, to eliminate ambiguity and enhance understanding. The items were further validated through a pilot study in Kitawoi Parish, Kween District, involving 30 farmers with sociodemographic characteristics similar to those in the main sample. However, the pilot sample was not included in the final study. Pilot data was then statistically tested using factor analysis to eliminate the items that loaded below 0.5 as recommended by Hair et al. (2017). As such, some items that loaded extremely lower were eliminated on constructs like SUN, BD, BR, BQ, PEU and PU. At this stage, care was taken not to remove the items that loaded below 0.7 but above 0.5 as we were not sure how the elimination would affect the reliability and validity of the construct. Thus, these were left to be validated during the assessment of the measurement model using PLS-SEM, which forms part of the analysis of this study. The questionnaire indicating the items is presented in Appendix 1.
The respondents' demographic characteristics offer valuable insights into the study population. A detailed summary is presented in Table 1. The sampled farmers primarily consisted of middle-aged farmers, with an average age of 42.6 years. These farmers had an average of 9.9 years of schooling, equivalent to Senior Three under Uganda's education standards, suggesting they were sufficiently literate to utilize BCT. On average, farmers had 8 years of barley farming experience, with farm sizes ranging from 0.5 to 4 acres (mean of 1.2 acres), confirming that barley farming is largely small-scale (FAO, 2017). Households were relatively large, averaging eight members, higher than Uganda's typical five to seven members (IFAD, 2023). This larger size provides labor advantages. Most household heads were male (87.35%), aligning with evidence that male farmers typically have better access to resources like land and information which are essential for technology adoption (Dwomoh et al., 2023). Additionally, 95.92% of respondents were doing farming as their primary occupation, emphasizing the timeliness and relevance of BCT application to improve their farm performance. Technology readiness was notable, with almost all farmers owning phones, though most were keypad models. Solar power was the dominant electricity source (91.84%), underscoring the limited access to national grid electricity in rural areas (Peters et al., 2019). Connectivity challenges, especially during the rainy season, and network instability (reported by 45.71% of farmers) pose barriers to effective BCT use.
All the sampled farmers reported having been members of farmer groups for an average period of 3.1 years. This underlines the role of farmer groups in facilitating social influence and peer learning. Through group interactions, farmers were likely able to gain insights and share experiences, which helped reduce uncertainty and build confidence in accepting BCT (Manda et al., 2020). The sampled farmers sourced most inputs from Company B (88.6%) and sold outputs exclusively back to it (100%). This indicates that blockchain facilitated smoother transactions between farmers and Company B through transparent pricing and secured market access, which likely contributed to the acceptance and adoption of the technology. The findings align with those of Gandhi Maniam et al. (2024) who reported that blockchain is a transformative tool for smallholder farmers who benefit from increased transparency, efficiency, and market access.
Regarding the need for credit to finance barley value chain operations, only 29.4% of the sampled barley farmers reported a need for credit, while the majority (70.6%) did not. This suggests that farmers relied more on Company B for financing their barley values chain rather than securing credit from financial institutions, which would typically charge high interest rates. A likely explanation for this unexpected finding is that adopting BCT initially provided farmers with access to inputs at no cost, with payment due upon delivering barley to Company B's buying centers. This supports the role of blockchain in reducing transaction costs for barley farmers (Ahluwalia et al., 2020). Additionally, 88.2% of the sampled farmers reported receiving blockchain-related training before enrolling in the BanQu blockchain technology app. This implies that training was provided to all farmers before enrollment, ensuring that the decision to adopt the technology was purely voluntary. It also highlights the importance of blockchain-specific training in enhancing farmers' knowledge during implementation (Leung et al., 2024).
The results presented in Figure 2 and Table 2 show that all the indicators that were retained after running PLS-SEM algorithm had loadings above 0.5 as recommended by Hair et al. (2017) for most social science-based research. Construct reliability measures how consistently the findings hold true across constructs. This study adopted two measures of assessing reliability namely Cronbach's alpha, and Jöreskog's (1971) composite reliability (rho_c). The values of the two measures should be over a threshold level of 0.70 for internal consistency reliability to be established in most scientific research (Hair et al., 2021). The higher the values the higher the reliability, however, rho_c values higher than 0.95 indicate that indicators are redundant which reduces their reliability and consequent validity (Hair et al., 2021). Results in Table 2 indicate that all the values of Cronbach's alpha, and rho_c are above 0.70 but below 0.95 which means that internal consistency reliability was established across all the constructs.
Construct validity evaluates whether the data accurately measures the intended constructs. It was assessed statistically through convergent and discriminant validity in the measurement model. Convergent validity examines the extent to which indicators align to measure a specific construct, typically using the Average Variance Extracted (AVE). An AVE value above 0.50 indicates sufficient convergent validity, meaning that indicators effectively converge to represent the construct (Hair et al., 2017). In this study's results in Table 2, all constructs had AVE values exceeding 0.50, confirming adequate convergent validity.
Discriminant validity was assessed using the Fornell-Larcker criterion, HTMT ratio, and cross-loadings. The Fornell and Larcker (1981) criterion confirms discriminant validity when the square root of a construct's AVE exceeds its correlations with other constructs. This condition was met in this study, as shown in Table 2. The HTMT ratio, proposed by Henseler et al. (2015) as a superior method, confirms discriminant validity when HTMT values are ≤ 0.85 for similar constructs or ≤ 0.90 for distinct constructs. All HTMT values in this study met the threshold, confirming adequate discriminant validity (Table 3). Cross-loadings assess how indicators load on their intended construct compared to other constructs. According to Hair et al. (2017), discriminant validity is established when indicators load significantly higher on their own construct than on others. The analysis results confirmed this criterion, as all indicators loaded substantially higher on their respective constructs than on others.
The variance inflation factor (VIF) measures multicollinearity among explanatory variables in regression models. A VIF >5 indicates multicollinearity, while values below 5 are recommended (Latif et al., 2020). In this study, all outer model VIF values were below 5, confirming no multicollinearity issues. Further, common method bias (CMB), a common issue in behavioral studies, was assessed using VIF values from the inner model. VIF values exceeding 3.3 indicate potential CMB or pathological collinearity (Kock, 2015). In this study, all VIF values were below 3.3, confirming the model was free of CMB.
The structural model was assessed using PLS bootstrapping with 5,000 samples, as recommended by Hair et al. (2017), to test path significance. Figure 3 provides the graphical output, while Table 4 summarizes the results.
As shown in Table 4, out of the 14 hypotheses tested through PLS-SEM bootstrapping, 9 were supported, while 5 were not. The results showed that in H1a, subjective norm had a positive but insignificant direct effect on behavioral intention (B = 0.103, t = 1.408, p = 0.159), but in H1b, subjective norm had a significant positive effect on perceived usefulness (B = 0.135, t = 2.520, p = 0.012). This implies that the effect of subjective norm on farmers' intention was indirect through perceived usefulness rather than direct. As a core construct in the theory of planned behavior, this finding may suggest that when barley farmers perceive that important others (e.g., peers, family, or social groups like farmer groups) believe they should accept BCT, it enhances their perception of BCT usefulness. This is consistent with previous research by Zhang et al. (2023) that suggested subjective norms indirectly influence acceptance behavior of pre-service teachers by altering perceptions of usefulness of artificial intelligence-enabled educational applications.
Additionally, farmer attitude, in H2, had a positive and significant effect on perceived usefulness (B = 0.177, t = 2.231, p = 0.026), implying that barley farmers' positive feelings toward BCT enhanced their perception of its usefulness in barley value chain activities. This is likely because favorable attitude may have influenced the barley farmers to see the broader advantages of BCT such as transparency and efficiency. This in turn may have reduced their skeptism and made them more receptive to seeing the technology as useful. This finding aligns with the acceptance study in China by Dong et al. (2022) where farmers' attitudes were found to positively influence perception toward usefulness of environmentally friendly agricultural technologies.
Perceived farmer image, in H3,also had a positive and significant effect on perceived usefulness (B = 0.193, t = 2.610, p = 0.009). This implies that farmers believed that using BCT enhances their social image and thus were more likely to perceive it as useful. As anticipated, this finding highlights how barley farmers' perceptions of their social standing or reputation influence their views on the benefits and practicality of adopting new technologies like blockchain. Consistent with Mishra et al. (2024), barley farmers who see themselves or are regarded by others as innovative and forward-thinking were more likely to perceive BCT as useful. Such farmers believe that adopting BCT enhances their reputation and aligns with their self-image as progress-oriented individuals. They associate BCT adoption with improved farm productivity, social recognition, and economic benefits, which further reinforces the perceived usefulness of the BCT.
The results further showed in H4 that BCT results demonstrability had a positive, significant effect on perceived usefulness (B = 0.261, t = 4.354, p = 0.001), implying that the more farmers were able to see and understand the tangible benefits of BCT, the more they perceived it as useful. Blockchain result demonstrability (BD), defined as the observability and communicability of a technology's results, may have enhanced perceived usefulness by making BCT outcomes such as increased gross margins, and productivity, tangible and shareable among barley farmers, them to perceive it as useful. This finding conforms with that of Izuagbe et al. (2022), who reported that the visible benefits of blockchain technology, such as increased efficiency, higher productivity, and lower transaction costs in crop value chain operations, enhance the users' perception of its usefulness.
Regarding H5, the results revealed a negative and insignificant effect of barley output quality on the relationship between BCT relevance and perceived usefulness (B = −0.021, t = 0.414, p = 0.679). The finding indicates that variations in barley quality did not meaningfully influence how farmers perceived the usefulness of BCT in the value chain. Alternatively, it may indicate that farmers did not perceive a direct connection between improved barley quality and the relevance or benefits of BCT. This finding may be partly explained by the high-quality standards required by Company B, for barley crop output. Barley grain must meet various physiological and biochemical quality parameters, such as hectoliter weight, protein and carbohydrate content, specific moisture levels, grain uniformity, etcetera, before being accepted for malt production and brewing (Rani and Bhardwaj, 2021). However, not all of these quality parameters are directly influenced or achieved through blockchain technology use. Additionally, barley is highly sensitive to various postharvest handling processes, for which many farmers lack the necessary skills. As a result, lower-quality barley produced by farmers is often classified as grade two, fetching lower prices at Company B despite BCT's potential to enhance value chain performance. This finding contrasts with other TAM studies, such as those by Al-Gahtani (2016) and Venkatesh and Davis (2000), where output quality positively moderated the relationship between BCT relevance and perceived usefulness.
Equally, in H6, BCT relevance to the barley value chain had a negative and non-significant effect on perceived usefulness (B = −0.039, t = 0.449, p = 0.654). The implication here is that farmers do not necessarily perceive BCT as useful solely based on its relevance to crop value chains; other factors may also influence their perception. The possible explanations for this finding are as follows. First, since the technology relevance construct is a cognitive instrumental process, as conceptualized by Venkatesh and Davis (2000), rural barley farmers may have been unable to apply a mental representation to connect the goals of barley value chain to BCT, in order to form a clear perception of its usefulness. Second, a lack of technical knowledge about how BCT works and how it could improve their operations in the initial stages of adoption may have further contributed to this result. Third, barley farmers might have associated BCT with external control, monitoring, or additional compliance burdens imposed by Company B rather than viewing it as a tool that would directly benefit them. All these factors could weaken perceived usefulness of BCT, even if it is considered relevant in the broader value chain. This study presents contradictory findings to previous research by Okcu et al. (2019) and Wu and Chen (2017), where task relevance was found to have a positive and significance influence on perceived usefulness. The possible reason for this contradiction could be attributed to differences in user-specific contexts between the present study and the aforementioned studies.
Nevertheless, in H7, BCT relevance was found to have a positive and significant effect on farmers' behavioral intention (B = 0.213, t = 2.261, p = 0.024), indicating that BCT relevance had rather a direct effect on behavioral intention than indirectly through perceived usefulness. This finding implies that when farmers recognize the relevance of BCT in crop value chains, they are more likely to express an intention to adopt or engage with it. The rationale behind this could be that BCT was seen as highly relevant to barley farmers' BCT operations, directly motivating their intentions to accept it, regardless of other factors like perceived usefulness. Further, the findings may reflect the early stages of BCT adoption, where relevance is recognized but perceived usefulness has not yet fully materialized. As farmers gain experience and witness concrete benefits, their perception of usefulness may evolve, potentially strengthening adoption in the long run. These contrasting findings suggest a disconnect between farmers' recognition of BCT's relevance and their early perception of its usefulness. While they acknowledge its role in the value chain, which encourages acceptance intentions, they may not yet see clear personal benefits. This highlights the need for contextual and tailored interventions, such as farmer education, demonstration projects, and incentives, to bridge the gap between relevance and perceived usefulness, ultimately fostering stronger adoption of BCT in the barley value chain. While previous studies have examined the effect of technology relevance on perceived usefulness (Kemp et al., 2019; Zarafshani et al., 2020), this study, to our knowledge, is the first to provide evidence of a direct relationship between technology relevance and behavioral intention.
Correspondingly, results for H8 showed that farmers' perceived behavioral control had a positive and significant effect on perceived ease of use of BCT (B = 0.495, t = 7.845, p = 0.001). This finding suggests that when farmers feel they have the necessary resources, knowledge, and ability to use BCT, they are more likely to perceive it as easy to use. This is probably because barley farmers believed they could accept BCT based on resources like possession of mobile phones, land, skills, and self-efficacy, which enhanced their perception of BCT's ease of use. Additionally, the flexibility of BCT to function on both sensory and keypad phones may have contributed to farmers perceiving it as easy to use. This finding aligns with Lopes et al. (2024), who noted that e-commerce shoppers who have control over the necessary resources are more likely to perceive AI enabled platforms as easy to use.
Also, H9 results showed a positive and significant effect of perceived usefulness on perceived ease of use (B = 0.289, t = 4.225, p = 0.001), implying that when farmers recognize the benefits of BCT, they are more likely to perceive it as easy to use. The plausible explanation for this is that barley farmers' positive perceptions of blockchain's usefulness in areas like transparency, efficiency, or market access, may have superseded their initial skepticism of its ease of use, motivating them to invest more effort and time into learning and using it, which in turn shaped their view of its ease of use. Instead of ease of use leading to usefulness, as often suggested in technology adoption models (Davis, 1989; He et al., 2018), this finding indicates that usefulness itself may shape how easy farmers perceive the technology to be. This may also highlight that promoting the tangible benefits of BCT could indirectly improve its acceptance by making it seem more easier to use. Other studies have examined the role of perceived usefulness in shaping user attitudes, often finding a positive effect (Toraman, 2022). However, to our knowledge, this is the first study to explore its direct effect on perceived ease of use.
The results in H10 further indicated that perceived usefulness had a positive and significant effect on behavioral intention (B = 0.395, t = 3.959, p = 0.001). This implies that barley farmers who perceived BCT as beneficial for enhancing productivity and addressing value chain challenges were more likely to show a stronger intention to accept it. This could be because farmers may associate BCT with tangible benefits such as better contract enforcement, reduced side-selling, and improved market access. If they perceive BCT as a tool that fosters trust, reduces fraud, and enhances financial gains through transparency, their intention to adopt it is likely to increase. When technology directly addresses real challenges, farmers become more motivated to embrace it. Consistent with the hypothesis, this finding aligns with the TAM framework and previous research, where perceived usefulness has been found to positively influence technology acceptance intentions. For example, Victor et al. (2021) found that perceived usefulness significantly influenced Nigerian farmers' behavioral intentions to accept mobile applications. Similarly, Adnan et al. (2019) reported that perceived usefulness positively influenced Malaysian farmers' behavioral intentions to accept green fertilizer technology.
In H11, the study results surprisingly found that perceived ease of use had a positive but insignificant effect on behavioral intention (B = 0.019, t = 0.250, p = 0.803). This implies that in this smallholder farming context, how easy farmers perceive BCT to be may not be a strong predictor of their intention to accept it. Although perceived ease of use is a key construct in the TAM and TPB for positively and significantly predicting behavior (Davis, 1989; Salloum et al., 2019), farmers may have prioritized the perceived usefulness of BCT over its ease of use. Barley farmers might have been more focused on how BCT can directly benefit them in terms of increasing productivity, elimination of exploitation from middlemen, or solving input sourcing challenges, rather than how simple it is to use. Another possible explanation could be that Company B's BCT tailored training and support systems, including on-farm visits and regular group training sessions, may have alleviated barley farmers' concerns about the technology's ease of use. As a result, ease of use became less critical in influencing acceptance intention compared to factors like perceived usefulness and relevance. Our findings align with Al-Adwan et al. (2023), who reported that perceived ease of use did not significantly affect students' intentions to use metaverse-based learning platforms in Jordan.
Voluntariness to accept BCT, in H12, had a positive and significant effect on perceived usefulness (B = 0.275, t = 4.433, p = 0.001), implying that when farmers feel they are adopting BCT by choice rather than obligation, they are more likely to perceive it as useful. This study hypothesized a direct effect of voluntariness on perceived usefulness on the grounds that when individuals accept a technology voluntarily, they are intrinsically motivated to explore and utilize its capabilities. This idea is well supported in the literature on self determination theory and intrinsic motivation in human behavior (Deci and Ryan, 1985; Ryan and Deci, 2000; Deci and Ryan, 2013). This intrinsic motivation can lead to a more favorable perception of the technology's usefulness. In this context, farmers likely viewed their decision to accept BCT as voluntary, free from external pressures, which encouraged them to explore BCT benefits in barley value chain operations, thereby enhancing their perception of its usefulness. While previous studies have primarily examined the direct effect of voluntariness on behavioral intention, often finding significant positive relationships (Bervell and Arkorful, 2020; Park et al., 2022), or its role as a moderator, as discussed in H13, this study provides novel insights into the relationship between voluntary technology acceptance and perceived usefulness among small-scale farmers, who are key stakeholders in agricultural value chains.
H13 results indicated that voluntariness had no significant moderating effect on the subjective norm–behavioral intention relationship (B = −0.072, t = 1.164, p = 0.244). This implies that farmers' decision to accept BCT was not significantly influenced by social pressures (subjective norms), regardless of whether the acceptance was voluntary or not. In other words, even if influential figures (such as, peers, extension officers, or company B staffs) encouraged BCT adoption, voluntariness did not strengthen or weaken the effect of this social influence on farmers' behavioral intentions. It is possible that barley farmers' acceptance decisions might have been more driven by personal and economic considerations (like, perceived benefits, trust, or financial benefits), rather than social expectations or normative pressures. The voluntary nature of adoption may have led farmers to rely more on individual assessments or intrinsic motivation rather than conforming to social influence. This finding contrasts that of Venkatesh and Davis (2000), who found that voluntariness moderates the influence of subjective norms on behavioral intention. The reason for this contrast could be that unlike workplace environments, where employees may feel obligated to conform to social norms (as seen in Venkatesh and Davis, 2000), smallholder farmers may operate independently and base decisions on tangible benefits rather than peer pressure.
The structural model explained 49.9% (R2 = 0.499) of the variance in farmers' BI to accept BCT, 61.6% (R2 = 0.616) in PU, and 46.6% (R2 = 0.466) in PEU, all above the 10% threshold, making them acceptable (Hair et al., 2017; Sarstedt et al., 2014). F2, indicating effect sizes, showed that only BR had a small effect on PU (F2 = 0.001), while other explanatory variables had medium to large effects. PBC had the largest effect on PEU (F2 = 0.352), and PEU had a small effect on BI (F2 = 0.015). Effect sizes met Cohen (1988) and Hair et al. (2021) criteria (0.02–0.35). Q2 values, all above zero, confirmed the model's predictive relevance (Hair and Alamer, 2022). The SRMR, measuring the average differences between observed and model-implied correlations, is the most common evaluator of model residuals. A threshold of ≤ 0.08 is recommended (Hair et al., 2021; Henseler et al., 2016). The obtained SRMR values of 0.068 (saturated model) and 0.073 (estimated model) indicate a good model fit.
The model was also assessed for out of sample predictive power using the PLS predict approach in Smart PLS-SEM to ascertain the generalizability and practicability of the findings. Based on the results in Table 5, the Q2 predict values for the dependent variables were all above the threshold of zero. Similarly, as shown in Table 6, Column 1, the Q2 predict values for the independent variables also exceeded this threshold, indicating that the model's constructs have strong out-of-sample predictive power.
Further, the prediction errors in the PLS-SEM model, specifically the root mean squared error (PLS-SEM_RMSE) and mean absolute error (PLS-SEM_MAE) of the dependent variables' indicators, were compared against the benchmark values from a naïve linear model (LM), denoted as LM_RMSE and LM_MAE, respectively. As shown in Table 6, all but one PLS-SEM_RMSE value were lower than the corresponding LM_RMSE values, while all except two PLS-SEM_MAE values were lower than their respective LM_MAE values. According to Shmueli et al. (2019), this indicates that the model demonstrated medium to high predictive power. To address the two indicators with lower predictive power (PEU3 and BI2), the researchers examined potential data issues such as distribution anomalies, outliers, and indicator loadings. However, attempts to improve their predictive power through outlier treatment as recommended by Mooi et al. (2018) compromised the overall quality of the measurement model. Given that both indicators exhibited strong loadings on their respective constructs and retained medium predictive power, they were ultimately retained in the model.
This study intended to determine the key factors influencing barley farmers' acceptance of BCT within the barley value chain in a rural farming community. It thus highlights that while subjective norms positively impacted perceived usefulness, farmer attitudes, image, and result demonstrability were strong predictors of BCT's perceived usefulness. Additionally, the relevance of BCT in the barley value chain and farmers' perceived control were important drivers of adoption intentions. Surprisingly, perceived ease of use did not significantly influence adoption intentions, indicating that farmers prioritized perceived usefulness over ease of use, potentially due to tailored training and support systems. Voluntariness positively influenced perceived usefulness, suggesting that intrinsic motivation plays a key role in technology acceptance. However, voluntariness did not moderate the influence of subjective norms on behavioral intention, suggesting that farmers were more driven by personal and economic factors than social pressures. These findings contribute to the literature by providing novel insights into the factors influencing smallholder farmers' adoption of complex technologies like BCT, emphasizing the importance of relevance, perceived benefits, and intrinsic motivation in technology adoption.
This study extends the TAM and TPB models by revealing new dynamics in rural farmers' acceptance of BCT. Unlike traditional TAM, where PEU influences PU, this study finds that PU significantly shapes PEU in rural farming. Farmers' initial perceptions of a technology's utility drive their later beliefs about its ease of use, challenging established TAM assumptions and encouraging further exploration of reciprocal relationships in technology acceptance. The study further highlights voluntariness (BV) and social influence as critical factors. BV positively affects PU, reflecting intrinsic motivation, particularly relevant for disruptive technologies like BCT in rural areas with limited external pressures.
Moreover, subjective norm positively affects perceived usefulness, emphasizing the role of social influence in collectivist, rural settings. A novel finding is the direct influence of BCT's relevance on behavioral intention, bypassing perceived usefulness. This underscores the importance of task-specific factors, suggesting that direct applicability to tasks can motivate acceptance independently of perceived usefulness. Lastly, the study confirms the significance of perceived behavioral control in shaping perceived usefulness. Farmers' confidence in their ability to use BCT, based on resource availability (such as, mobile phones, land, network stability), reinforces perceived ease of use, aligning with TPB's emphasis on behavioral control as a key determinant of behavioral intention.
The finding that perceived usefulness is a stronger determinant of behavioral intention than ease of use suggests that training programs should emphasize the tangible benefits of BCT, such as productivity improvements and enhanced value chain operations. Communicating these benefits clearly is crucial, as they play a greater role in shaping acceptance decisions than concerns about ease of use. The positive influence of subjective norm and voluntariness on perceived usefulness underscores the importance of fostering voluntary, socially-supported acceptance. Extension services and agricultural companies should leverage farmers' social networks, peer groups, and community leaders to encourage BCT acceptance. Social influence can effectively motivate technology acceptance. The direct effect of BCT relevance on farmers' intentions highlights the need for acceptance strategies to demonstrate how BCT specifically improves barley farming operations. Aligning BCT's benefits with farmers' daily practices can boost acceptance by showing its direct impact on productivity.
Additionally, the role of perceived behavioral control in shaping PEU suggests that governments and agricultural organizations should provide resources like mobile phones, affordable internet, and training to empower farmers. Policies addressing infrastructure gaps and offering practical support can enhance farmers' ability to adopt BCT. The influence of farmers' image on perceived usefulness suggests that implementers should target farmers who value innovation and social recognition. Marketing strategies should highlight how accepting BCT can improve their reputation and social standing within the community.
This study's cross-sectional design limits conclusions about causal relationships over time; a longitudinal approach could better capture how perceptions evolve and influence adoption. The focus on barley farmers may restrict generalizability to other sectors, and reliance on self-reported data introduces potential biases, despite mitigation efforts. Future research could use objective measures or mixed methods for a broader understanding of adoption behaviors. Exploring voluntariness in technology acceptance, particularly its effect on intrinsic motivation and PU in various agricultural contexts, is recommended. This study found voluntary adoption boosts motivation, but further research could assess how external pressures and cultural factors shape decisions across regions and technologies.
The reversed relationship between PU and PEU observed in this study also warrants investigation in other rural settings. Again, the mediating role of PU on SUN-BI and BR-BI relationships requires further study. While SUN influenced PU, its direct effect on BI was insignificant. Future work could examine how social structures and norms shape technology adoption in agricultural communities. Finally, PBC, which significantly influenced PEU, should be explored further, focusing on factors like digital literacy, resource access, and external support to address barriers for smallholder farmers.
The raw data supporting the conclusions of this article will be made available by the authors, without undue reservation.
The studies involving humans were approved by Egerton University, Approval No. EUISERC/APP/355/2024), and Uganda Christian University, Approval No. UCUREC-2024-1000. The studies were conducted in accordance with the local legislation and institutional requirements. The participants provided their written informed consent to participate in this study.
RN: Conceptualization, Data curation, Formal analysis, Funding acquisition, Investigation, Methodology, Resources, Writing – original draft, Writing – review & editing. PM: Supervision, Writing – review & editing. DO: Supervision, Writing – review & editing.
The author(s) declare that financial support was received for the research and/or publication of this article. This study received funding from Regional Universities Forum for Capacity Building in Agriculture (RUFORUM) Grant No. #RU/2024/GTA/CCNY/23, and Kyambogo University Research Grants and Publications Committee.
The authors would like to thank the smallholder barley farmers in eastern Uganda for their time and participation during field data collection.
The authors declare that the research was conducted in the absence of any commercial or financial relationships that could be construed as a potential conflict of interest.
The author(s) declare that no Gen AI was used in the creation of this manuscript.
All claims expressed in this article are solely those of the authors and do not necessarily represent those of their affiliated organizations, or those of the publisher, the editors and the reviewers. Any product that may be evaluated in this article, or claim that may be made by its manufacturer, is not guaranteed or endorsed by the publisher.
The Supplementary Material for this article can be found online at: https://www.frontiersin.org/articles/10.3389/fsufs.2025.1552637/full#supplementary-material
Adnan, N., Nordin, S. M., Bahruddin, M. A., and Tareq, A. H. (2019). A state-of-the-art review on facilitating sustainable agriculture through green fertilizer technology adoption: assessing farmers behavior. Trends Food Sci. Technol. 86, 439–452. doi: 10.1016/j.tifs.2019.02.040
African News Agency (2019). AB InBev Empowers Farmers across Africa through Blockchain Technology. Available online at: https://www.iol.co.za/business-report/companies/ab-inbev-empowers-farmers-across-africa-through-blockchain-technology-30110632 (accessed October 9, 2024).
Ahluwalia, S., Mahto, R. V., and Guerrero, M. (2020). Blockchain technology and startup financing: a transaction cost economics perspective. Technol. Forecast. Soc. Change 151, 1–6. doi: 10.1016/j.techfore.2019.119854
Ajzen, I. (1985). “From intentions to actions: a theory of planned behavior,” in Action Control, eds. J. Kuhl, J. Beckmann (Berlin; Heidelberg: Springer), 11–39.
Ajzen, I. (1988). Attitudes, Personality, and Behavior. New York, NY: McGraw-Hill; U.S. ed. Dorsey Press.
Ajzen, I. (1991). The theory of planned behavior. Org. Behav. Hum. Dec. Proc. 50, 179–211. doi: 10.1016/0749-5978(91)90020-T
Ajzen, I. (2002). Attitudes, Personality, and Behavior. McGraw-Hill Education; Open University Press.
Akella, G. K., Wibowo, S., Grandhi, S., and Mubarak, S. (2023). A systematic review of blockchain technology adoption barriers and enablers for smart and sustainable agriculture. Big Data Cogn. Comput. 7, 1–22. doi: 10.3390/bdcc7020086
Al-Adwan, A. S., Li, N., Al-Adwan, A., Abbasi, G. A., Albelbisi, N. A., and Habibi, A. (2023). Extending the technology acceptance model (TAM) to predict university students' intentions to use metaverse-based learning platforms. Educ. Inform. Technol. 28, 15381–15413. doi: 10.1007/s10639-023-11816-3
Al-Gahtani, S. S. (2016). Empirical investigation of E-learning acceptance and assimilation: a structural equation model. Appl. Comput. Inform. 12, 27–50. doi: 10.1016/j.aci.2014.09.001
Ballantyne, P., and Addison, C. (2020). Communicating Digital Agriculture Innovations - A Blockchain Exchange. UN Innovation Network. Available online at: https://europa.eu/capacity4dev/public-ict/documents/communicating-digital-agriculture-innovations-blockchain-exchange (accessed November 14, 2024).
Bashir, T., Zhongfu, T., Sadiq, B., Niaz, U., Anjum, F., and Mahmood, H. (2022). An assessment of influential factors developing the intention to use social media sites: a technology acceptance model-based approach. Front. Psychol. 13:983930. doi: 10.3389/fpsyg.2022.983930
Bedford, L. (2018). Blockchain Technology Will Improve Farmers' Ability to Market and Sell Crops. Successful Farming. Available online at: https://www.agriculture.com/technology/data/blockchain-technology-will-improve-farmers-ability-to-market-and-sell-crops (accessed August 9, 2024).
Bervell, B., and Arkorful, V. (2020). LMS-enabled blended learning utilization in distance tertiary education: establishing the relationships among facilitating conditions, voluntariness of use and use behavior. Int. J. Educ. Technol. Higher Educ. 17, 1–16. doi: 10.1186/s41239-020-0183-9
Bhusal, C. S. (2021). Blockchain technology in agriculture: a case study of blockchain start-up companies. Int. J. Comput. Sci. Inform. Technol. 13, 31–48. doi: 10.5121/ijcsit.2021.13503
Cheptoyek, S. (2018). The Relationship Between Agronomic Practices and Productivity of Barley in Kwosir Sub-County Kween District (Undergraduate dissertation). Makerere University Library. Available online at: http://dissertations.mak.ac.ug/handle/20.500.12281/5666 (accessed October 15, 2024).
Choruma, D. J., Dirwai, T. L., Mutenje, M. J., Mustafa, M., Chimonyo, V. G. P., Jacobs-Mata, I., et al. (2024). Digitalisation in agriculture: a scoping review of technologies in practice, challenges, and opportunities for smallholder farmers in Sub-Saharan Africa. J. Agric. Food Res. 18, 1–10. doi: 10.1016/j.jafr.2024.101286
Cohen, J. (1988). Statistical Power Analysis for the Behavioral Sciences, 2nd Edn. Hillsdale, NJ: Lawrence Erlbaum Associates, Publishers.
Dai, Q., and Cheng, K. (2022). What drives the adoption of agricultural green production technologies? An extension of TAM in agriculture. Sustainability 14, 1–18. doi: 10.3390/su142114457
Davis, F. D. (1989). Perceived usefulness, perceived ease of use, and user acceptance of information technology. MIS Quart. 13, 319–340. doi: 10.2307/249008
Davis, F. D., Bagozzi, R. P., and Warshaw, P. R. (1989). User acceptance of computer technology: a comparison of two theoretical models. Manag. Sci. 35, 982–1003. doi: 10.1287/mnsc.35.8.982
Deci, E. L., and Ryan, R. M. (1985). Intrinsic Motivation and Self-Determination in Human Behavior. New York, NY: Springer.
Deci, E. L., and Ryan, R. M. (2013). Intrinsic Motivation and Self-Determination in Human Behavior. New York, NY: Springer Science and Business Media.
Dissanayake, C. A. K., Jayathilake, W., Wickramasuriya, H. V. A., Dissanayake, U., Kopiyawattage, K. P., and Wasala, W. M. (2022). Theories and models of technology adoption in agricultural sector. Hum. Behav. Emerg. Technol. 2022, 1–15. doi: 10.1155/2022/9258317
Dong, H., Wang, H., and Han, J. (2022). Understanding ecological agricultural technology adoption in China using an integrated technology acceptance model—theory of planned behavior model. Front. Environ. Sci. 10:927668. doi: 10.3389/fenvs.2022.927668
Dwomoh, D., Agyabeng, K., Tuffour, H. O., Tetteh, A., Godi, A., and Aryeetey, R. (2023). Modeling inequality in access to agricultural productive resources and socioeconomic determinants of household food security in Ghana: a cross-sectional study. Agric. Food Econ. 11, 1–21. doi: 10.1186/s40100-023-00267-6
FAO (2017). Productivity and Efficiency Measurement in Agriculture: Literature Review and Gaps Analysis. Available online at: https://openknowledge.fao.org/server/api/core/bitstreams/dcd4edfc-f7d5-4872-8996-5612c87446d6/content (accessed March 17, 2024).
Fornell, C., and Larcker, D. F. (1981). Structural equation models with unobservable variables and measurement error: algebra and statistics. J. Market. Res. 18, 382–388. doi: 10.1177/002224378101800313
Further Africa (2019). AB InBev and BanQu Empowering Farmers through Blockchain Technology. Available online at: https://furtherafrica.com/2019/08/20/ab-inbev-banqu-empowering-farmers-through-blockchain-technology/ (accessed October 9, 2024).
Gandhi Maniam, P. S., Acharya, N., Sassenberg, A.-M., and Soar, J. (2024). Determinants of blockchain technology adoption in the Australian agricultural supply chain: a systematic literature review. Sustainability 16, 1–22. doi: 10.3390/su16135806
Giganti, P., Borrello, M., Falcone, P. M., and Cembalo, L. (2024). The impact of blockchain technology on enhancing sustainability in the agri-food sector: a scoping review. J. Clean. Prod. 456, 1–18. doi: 10.1016/j.jclepro.2024.142379
Hair, J., and Alamer, A. (2022). Partial least squares structural equation modelling (PLS-SEM) in second language and education research: guidelines using an applied example. Res. Methods Appl. Linguis. 1, 1–17. doi: 10.1016/j.rmal.2022.100027
Hair, J. F., Hult, G. T. M., Ringle, C. M., and Sarstedt, M. (2017). A Primer on Partial Least Squares Structural Equation Modeling (PLS-SEM), 2nd Edn. Los Angeles, CA: SAGE.
Hair, J. F., Hult, G. T. M., Ringle, C. M., Sarstedt, M., Danks, N. P., and Ray, S. (2021). Partial Least Squares Structural Equation Modeling (PLS-SEM) Using R: A Workbook. Springer International Publishing. doi: 10.1007/978-3-030-80519-7
Hassani, M. S., Mohamed, H. I., Urassa, J. K., and Muhanga, M. I. (2023). Digital literacy and crop productivity: evidence from cross-sectional data in Hai and Moshi Districts, Kilimanjaro-Tanzania. Tanzania J. Agric. Sci. 22, 264–279. Available online at: https://www.ajol.info/index.php/tjags/article/view/266572
He, Y., Chen, Q., and Kitkuakul, S. (2018). Regulatory focus and technology acceptance: perceived ease of use and usefulness as efficacy. Cogent Bus. Manag. 5, 1–52. doi: 10.1080/23311975.2018.1459006
Henseler, J., Hubona, G., and Ray, P. A. (2016). Using PLS path modeling in new technology research: updated guidelines. Indust. Manag. Data Syst. 116, 2–20. doi: 10.1108/IMDS-09-2015-0382
Henseler, J., Ringle, C. M., and Sarstedt, M. (2015). A new criterion for assessing discriminant validity in variance-based structural equation modeling. J. Acad. Market. Sci. 43, 115–135. doi: 10.1007/s11747-014-0403-8
IFAD (2023). Smallholders, Food Security, the Environment. Available online at: https://www.ifad.org/documents/38714170/39135645/smallholders_report.pdf/133e8903-0204-4e7d-a780-bca847933f2e (accessed September 26, 2024).
Izuagbe, R., Ifijeh, G., Izuagbe-Roland, E., Olawoyin, O. R., and Ogiamien, L. O. (2019). Determinants of perceived usefulness of social media in university libraries: subjective norm, image and voluntariness as indicators. J. Acad. Librar. 45, 394–405. doi: 10.1016/j.acalib.2019.03.006
Izuagbe, R., Olawoyin, O. R., Nkiko, C., Ilo, P. I., Yusuf, F., Iroaganachi, M., et al. (2022). Impact analysis of e-databases' job relevance, output quality and result demonstrability on faculty research motivation. Library Hi Tech. 40, 1402–1421. doi: 10.1108/LHT-03-2020-0050
Jöreskog, K. G. (1971). Simultaneous factor analysis in several populations. Psychometrika 36, 409–426. doi: 10.1007/BF02291366
Kagorora, J. P. K., Kansiime, M. K., Owuor, C., and Tumwine, J. (2021). A Review of Some Aspects of Uganda's Crop Agriculture: Challenges and Opportunities for Diversified Sector Output and Food Security. Working paper 26. CABI. doi: 10.1079/CABICOMM-62-8161
Kamilaris, A., Fonts, A., and Prenafeta-Boldú, F. X. (2019). The rise of blockchain technology in agriculture and food supply chains. Trends Food Sci. Technol. 91, 640–652. doi: 10.1016/j.tifs.2019.07.034
Kemp, A., Palmer, E., and Strelan, P. (2019). A taxonomy of factors affecting attitudes towards educational technologies for use with technology acceptance models. Br. J. Educ. Technol. 50, 2394–2413. doi: 10.1111/bjet.12833
Kock, N. (2015). Common method bias in PLS-SEM: a full collinearity assessment approach. Int. J. e-Collab. 11, 1–10. doi: 10.4018/ijec.2015100101
Kos, D., and Kloppenburg, S. (2019). Digital technologies, hyper-transparency and smallholder farmer inclusion in global value chains. Curr. Opin. Environ. Sustain. 41, 56–63. doi: 10.1016/j.cosust.2019.10.011
Kshetri, N. (2021). Blockchain and sustainable supply chain management in developing countries. In. J. Inform. Manage. 60, 1–13. doi: 10.1016/j.ijinfomgt.2021.102376
Kumarathunga, M., Calheiros, R. N., and Ginige, A. (2022). Smart agricultural futures market: blockchain technology as a trust enabler between smallholder farmers and buyers. Sustainability 14, 1–20. doi: 10.3390/su14052916
Latif, K. F., Pérez, A., and Sahibzada, U. F. (2020). Corporate social responsibility (CSR) and customer loyalty in the hotel industry: a cross-country study. Int. J. Hosp. Manag. 89, 1–13. doi: 10.1016/j.ijhm.2020.102565
Leung, A. C., Liu, D. Y., Luo, X., and Au, M. H. (2024). A constructivist and pragmatic training framework for blockchain education for IT practitioners. Educ. Inform. Technol. 29, 15813–15853. doi: 10.1007/s10639-024-12505-5
Lin, W., Huang, X., Fang, H., Wang, V., Hua, Y., Wang, J., et al. (2020). Blockchain technology in current agricultural systems: from techniques to applications. IEEE Access 8, 143920–143937. doi: 10.1109/ACCESS.2020.3014522
Lopes, J. M., Silva, L. F., and Massano-Cardoso, I. (2024). AI meets the shopper: psychosocial factors in ease of use and their effect on E-commerce purchase intention. Behav. Sci. 14, 1–22. doi: 10.3390/bs14070616
Managa, L. R., Zaca, F. N., Uchele, O., and Nemathithi, A. (2023). A review of technological barriers to climate-smart agriculture implementation in Sub-Saharan Africa: prospects for smallholder farmers. Africa Insight 53, 18–33. Available online at: https://hdl.handle.net/10520/ejc-afrins_v53_n2_a2
Manda, J., Khonje, M. G., Alene, A. D., Tufa, A. H., Abdoulaye, T., Mutenje, M., et al. (2020). Does cooperative membership increase and accelerate agricultural technology adoption? Empirical evidence from Zambia. Technol. Forecast. Soc. Change 158, 1–12. doi: 10.1016/j.techfore.2020.120160
Manley, S. C., Hair, J. F., Williams, R. I., and McDowell, W. C. (2021). Essential new PLS-SEM analysis methods for your entrepreneurship analytical toolbox. Int. Entrep. Manag. J. 17, 1805–1825. doi: 10.1007/s11365-020-00687-6
Marangunić, N., and Granić, A. (2015). Technology acceptance model: a literature review from 1986 to (2013). Univ. Access Inform. Soc. 14, 81–95. doi: 10.1007/s10209-014-0348-1
Marikyan, D., and Papagiannidis, S. (2023). “Unified theory of acceptance and use of technology: a review,” in TheoryHub Book, ed. S. Papagiannidis (Newcastle upon Tyne: Newcastle University), 1–16.
Massoro, Z. Z., and Adewale, N. T. (2019). Influence of attitude, subjective norms and personal innovativeness on intention to use open access journals: a case of agricultural research institutes. Library Philos. Pract. 1–13. Available online at: https://digitalcommons.unl.edu/libphilprac/2484
Mishra, N., Bhandari, N., Maraseni, T., Devkota, N., Khanal, G., Bhusal, B., et al. (2024). Technology in farming: unleashing farmers' behavioral intention for the adoption of agriculture 5.0. PLoS ONE 19:0308883. doi: 10.1371/journal.pone.0308883
Molina-Maturano, J., Verhulst, N., Tur-Cardona, J., Güereña, D. T., Gardeazábal-Monsalve, A., Govaerts, B., et al. (2021). Understanding smallholder farmers' intention to adopt agricultural apps: the role of mastery approach and innovation hubs in Mexico. Agronomy 11, 1–23. doi: 10.3390/agronomy11020194
Mooi, E., Sarstedt, M., and Mooi-Reci, I. (2018). Market Research. Singapore: Springer. doi: 10.1007/978-981-10-5218-7
Moore, G. C., and Benbasat, I. (1991). Development of an instrument to measure the perceptions of adopting an information technology innovation. Inform. Syst. Res. 2, 192–222. doi: 10.1287/isre.2.3.192
Moreno, V., Cavazotte, F., and Alves, I. (2017). Explaining university students' effective use of E-learning platforms. Br. J. Educ. Technol. 48, 995–1009. doi: 10.1111/bjet.12469
Mostafa, S. A., and Ahmad, I. A. (2018). Recent developments in systematic sampling: a review. J. Stat. Theory Pract. 12, 290–310. doi: 10.1080/15598608.2017.1353456
Ngubelanga, A., and Duffett, R. (2021). Modeling mobile commerce applications' antecedents of customer satisfaction among millennials: an extended TAM perspective. Sustainability 13, 1–29. doi: 10.3390/su13115973
Okcu, S., Hancerliogullari Koksalmis, K., Basak, E., and Calisir, F. (2019). “Factors affecting intention to use big data tools: an extended technology acceptance model,” in Industrial Engineering in the Big Data Era, eds. F. Calisir, E. Cevikcan, and H. Camgoz Akdag (Springer International Publishing), 401–416. doi: 10.1007/978-3-030-03317-0_33
Panwar, A., Khari, M., Misra, S., and Sugandh, U. (2023). Blockchain in agriculture to ensure trust, effectiveness, and traceability from farm fields to groceries. Fut. Intern. 15, 1–28. doi: 10.3390/fi15120404
Park, I., Kim, D., Moon, J., Kim, S., Kang, Y., and Bae, S. (2022). Searching for new technology acceptance model under social context: analyzing the determinants of acceptance of intelligent information technology in digital transformation and implications for the requisites of digital sustainability. Sustainability 14, 1–29. doi: 10.3390/su14010579
Peters, J., Sievert, M., and Toman, M. A. (2019). Rural electrification through mini-grids: challenges ahead. Energy Policy 132, 27–31. doi: 10.1016/j.enpol.2019.05.016
Piabuo, S. M., Yakan, H. B., Puatwoe, J. T., Nonzienwo, V. Y., and Mamboh, T. R. (2020). Effect of rural farmers' access to information on price and profits in cameroon. Cogent Food Agric. 6:1799530. doi: 10.1080/23311932.2020.1799530
Rahman, M. M., Tabash, M. I., Salamzadeh, A., Abduli, S., and Rahaman, M. S. (2022). Sampling techniques (probability) for quantitative social science researchers: a conceptual guidelines with examples. SEEU Rev. 17, 42–51. doi: 10.2478/seeur-2022-0023
Ramanujan, P., Bhattacharjea, S., and Alcott, B. (2022). A multi-stage approach to qualitative sampling within a mixed methods evaluation: some reflections on purpose and process. Can. J. Prog. Eval. 36, 355–364. doi: 10.3138/cjpe.71237
Rani, H., and Bhardwaj, R. D. (2021). Quality attributes for barley malt: the backbone of beer. J. Food Sci. 86, 3322–3340. doi: 10.1111/1750-3841.15858
Ren, Z., and Zhong, K. (2022). Driving mechanism of subjective cognition on farmers' adoption behavior of straw returning technology: evidence from rice and wheat producing provinces in China. Front. Psychol. 13:922889. doi: 10.3389/fpsyg.2022.922889
Ryan, R. M., and Deci, E. L. (2000). Self-determination theory and the facilitation of intrinsic motivation, social development, and well-being. Am. Psychol. 55, 68–78. doi: 10.1037//0003-066X.55.1.68
Salloum, S. A., Alhamad, Q. M., Al-Emran, M., Abdel Monem, A., and Shaalan, K. (2019). Exploring students' acceptance of E-learning through the development of a comprehensive technology acceptance model. IEEE Access 7, 128445–128462. doi: 10.1109/ACCESS.2019.2939467
Sarstedt, M., Ringle, C. M., Smith, D., Reams, R., and Hair, J. F. (2014). Partial least squares structural equation modeling (PLS-SEM): a useful tool for family business researchers. J. Fam. Bus. Strat. 5, 105–115. doi: 10.1016/j.jfbs.2014.01.002
Sendros, A., Drosatos, G., Efraimidis, P. S., and Tsirliganis, N. C. (2022). Blockchain applications in agriculture: a scoping review. Appl. Sci. 12, 1–37. doi: 10.3390/app12168061
Shmueli, G., Sarstedt, M., Hair, J. F., Cheah, J.-H., Ting, H., Vaithilingam, S., et al. (2019). Predictive model assessment in PLS-SEM: guidelines for using PLS predict. Eur. J. Mark. 53, 2322–2347. doi: 10.1108/EJM-02-2019-0189
Soodan, V., Jamwal, M., Rana, N. P., Sharma, D., and Chakraborty, S. (2024). Modelling the adoption of agro-advisory mobile applications: a theoretical extension and analysis using result demonstrability, trust, self-efficacy and mobile usage proficiency. J. Agribus. Dev. Emerg. Econ. 14, 749–768. doi: 10.1108/JADEE-05-2022-0087
Suresh, K., Narmilan, A., Kariapper, K. A. R. R., Nawaz, S. S., and Suresh, J. (2022). Farmers' Perception on Precision Farming Technologies: A Novel Approach. Mumbai: Indian Society of Agricultural Economics, 77, 1–24.
Thejaswini, S., and Ranjitha, R. K. (2020). “Blockchain in agriculture by using decentralized peer to peer networks,” in 2020 Fourth International Conference on Inventive Systems and Control (ICISC) (IEEE), 600–606. doi: 10.1109/ICISC47916.2020.9171083
Toraman, Y. (2022). Interest-free finance model by using blockchain-based company tokens: research on digital Turkish Lira (DTL) and Borsa Istanbul with technology acceptance model (TAM). EMAJ 12, 56–66. doi: 10.5195/emaj.2022.275
Tripoli, M., and Schmidhuber, J. (2020). Emerging Opportunities for the Application of Blockchain in the Agri-food Industry. Rome; Geneva: FAO; ICTSD. Available online at: https://www.fao.org/3/ca9934en/CA9934EN.pdf (accessed November 11, 2024).
Venkatesh, V., and Bala, H. (2008). Technology acceptance model 3 and a research agenda on interventions. Decis. Sci. 39, 273–315. doi: 10.1111/j.1540-5915.2008.00192.x
Venkatesh, V., and Davis, F. D. (2000). A theoretical extension of the technology acceptance model: four longitudinal field studies. Manag. Sci. 46, 186–204. doi: 10.1287/mnsc.46.2.186.11926
Victor, O., Nic, J. L., and Xiaomeng, L. (2021). Factors affecting the adoption of mobile applications by farmers: an empirical investigation. Afr. J. Agric. Res. 17, 19–29. doi: 10.5897/AJAR2020.14909
Widiar, G., Yuniarinto, A., and Yulianti, I. (2023). Perceived ease of use's effects on behavioral intention mediated by perceived usefulness and trust. Interdiscipl. Soc. Stud. 2, 1829–1844. doi: 10.55324/iss.v2i4.397
Wu, B., and Chen, X. (2017). Continuance intention to use MOOCs: integrating the technology acceptance model (TAM) and task technology fit (TTF) model. Comput. Hum. Behav. 67, 221–232. doi: 10.1016/j.chb.2016.10.028
Xiong, H., Dalhaus, T., Wang, P., and Huang, J. (2020). Blockchain technology for agriculture: applications and rationale. Front. Blockchain 3, 1–7. doi: 10.3389/fbloc.2020.00007
Yaghi, T., Arslan, A., Saeed, H., and Elmouhammad, A. (2023). “The response of Nigella Sativa plants to different natural and chemical fertilizers under Mediterranean conditions,” in Scientific Papers Series Management, Economic Engineering in Agriculture & Rural Development, 1–12. Available online at: https://managementjournal.usamv.ro/pdf/vol.23_4/Art93.pdf (accessed September 12, 2024).
Yamane, T. (1967). Statistics: An Introductory Analysis, 2nd Edn. New York, NY; Evanston, IL; London; Tokyo: Harper and Row; John WeatherHill, Inc.
Yuan, Y., and Sun, Y. (2024). Practices, challenges, and future of digital transformation in smallholder agriculture: insights from a literature review. Agric. Basel 14, 1–19. doi: 10.3390/agriculture14122193
Zaineldeen, S., Hongbo, L., Koffi, A. L., and Hassan, B. M. A. (2020). Technology acceptance model' concepts, contribution, limitation, and adoption in education. Univ. J. Educ. Res. 8, 5061–5071. doi: 10.13189/ujer.2020.081106
Zarafshani, K., Solaymani, A., D'Itri, M., Helms, M. M., and Sanjabi, S. (2020). Evaluating technology acceptance in agricultural education in Iran: a study of vocational agriculture teachers. Soc. Sci. Human. Open 2, 1–8. doi: 10.1016/j.ssaho.2020.100041
Keywords: BanQu blockchain, barley value chain, intentions to adopt, smallholder farmers, Technology Acceptance Model, Uganda
Citation: Ninsiima R, Mshenga P and Okello D (2025) Determinants of smallholder barley farmers' intentions to adopt blockchain technology: a Technology Acceptance Model approach in Uganda. Front. Sustain. Food Syst. 9:1552637. doi: 10.3389/fsufs.2025.1552637
Received: 28 December 2024; Accepted: 24 February 2025;
Published: 19 March 2025.
Edited by:
Yari Vecchio, University of Bologna, ItalyReviewed by:
Francesco Bozzo, University of Bari Aldo Moro, ItalyCopyright © 2025 Ninsiima, Mshenga and Okello. This is an open-access article distributed under the terms of the Creative Commons Attribution License (CC BY). The use, distribution or reproduction in other forums is permitted, provided the original author(s) and the copyright owner(s) are credited and that the original publication in this journal is cited, in accordance with accepted academic practice. No use, distribution or reproduction is permitted which does not comply with these terms.
*Correspondence: Racheal Ninsiima, bnJhY2hlYWxAa3l1LmFjLnVn
Disclaimer: All claims expressed in this article are solely those of the authors and do not necessarily represent those of their affiliated organizations, or those of the publisher, the editors and the reviewers. Any product that may be evaluated in this article or claim that may be made by its manufacturer is not guaranteed or endorsed by the publisher.
Research integrity at Frontiers
Learn more about the work of our research integrity team to safeguard the quality of each article we publish.