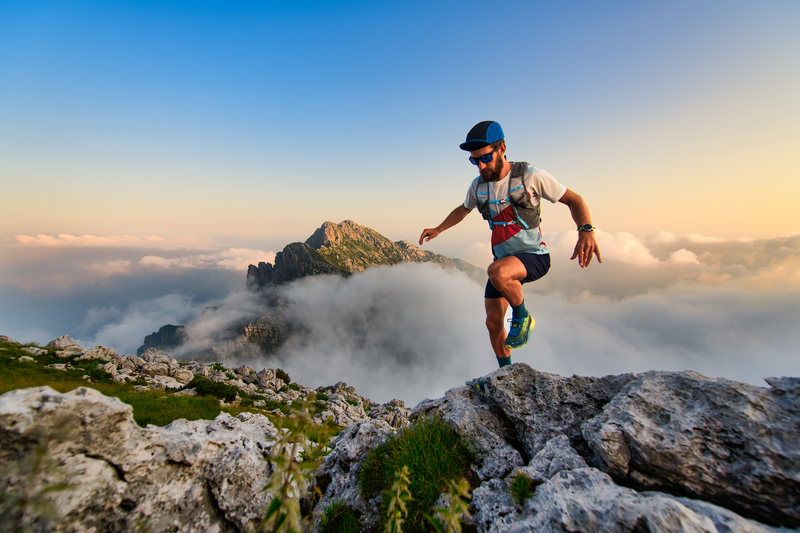
94% of researchers rate our articles as excellent or good
Learn more about the work of our research integrity team to safeguard the quality of each article we publish.
Find out more
ORIGINAL RESEARCH article
Front. Sustain. Food Syst.
Sec. Climate-Smart Food Systems
Volume 9 - 2025 | doi: 10.3389/fsufs.2025.1548223
This article is part of the Research Topic Measurements and Modelling of Methane Emissions from Agricultural Cropping Systems View all articles
The final, formatted version of the article will be published soon.
You have multiple emails registered with Frontiers:
Please enter your email address:
If you already have an account, please login
You don't have a Frontiers account ? You can register here
Ruminant livestock production depends on microorganisms to ferment forages into valuable dairy and meat products. However, this process also generates enteric methane emissions, a significant contributor to anthropogenic greenhouse gases. Despite various strategies aimed at reducing methane emissions, success has been limited. In previous work, we developed an AI-driven model based on deep microbiome sequencing, which predicts the effect of feed additives on methane emissions. The model uses sequenced rumen samples from a given herd to construct microbiome networks to identify biomarkers associated with feed additive effectiveness in the reduction of methane emissions. In this study, we validated the model supplying a commercial methane-mitigating feed additive and performing hundreds of in-situ methane measurements across several commercial dairy farms. The results highlight the model's robustness and precision, demonstrating its effectiveness in predicting enteric methane reductions and enhancing feed additive performance. Additionally, the model serves as a critical tool for data-driven decision-making, playing a pivotal role in advancing precision agriculture practices.
Keywords: Enteric methane emissions, Dairy, AI model, predictive model, precision agriculture, feed additive
Received: 19 Dec 2024; Accepted: 11 Mar 2025.
Copyright: © 2025 Altshuler, Calvao Chebach, Cohen and Gatica. This is an open-access article distributed under the terms of the Creative Commons Attribution License (CC BY). The use, distribution or reproduction in other forums is permitted, provided the original author(s) or licensor are credited and that the original publication in this journal is cited, in accordance with accepted academic practice. No use, distribution or reproduction is permitted which does not comply with these terms.
* Correspondence:
Yaniv Altshuler, Massachusetts Institute of Technology, Cambridge, United States
Disclaimer: All claims expressed in this article are solely those of the authors and do not necessarily represent those of their affiliated organizations, or those of the publisher, the editors and the reviewers. Any product that may be evaluated in this article or claim that may be made by its manufacturer is not guaranteed or endorsed by the publisher.
Research integrity at Frontiers
Learn more about the work of our research integrity team to safeguard the quality of each article we publish.