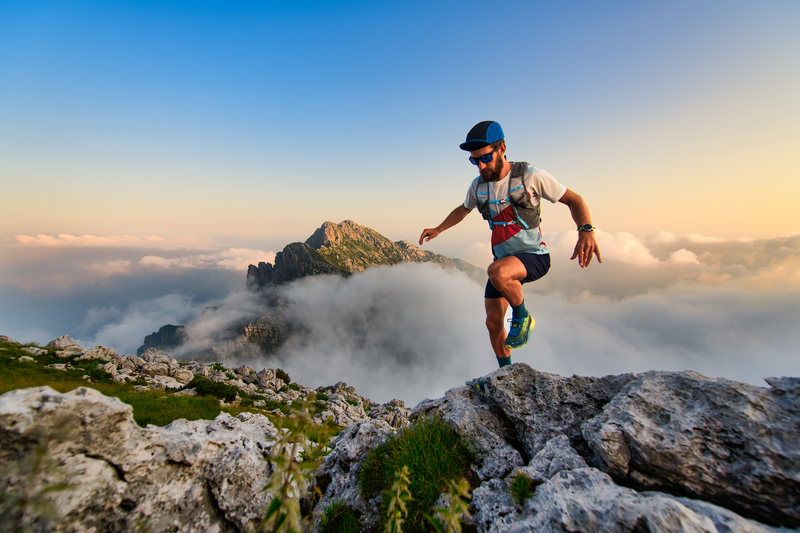
95% of researchers rate our articles as excellent or good
Learn more about the work of our research integrity team to safeguard the quality of each article we publish.
Find out more
ORIGINAL RESEARCH article
Front. Sustain. Food Syst. , 01 April 2025
Sec. Agricultural and Food Economics
Volume 9 - 2025 | https://doi.org/10.3389/fsufs.2025.1545548
This article is part of the Research Topic Harnessing Digital Innovation for Sustainable Agricultural Development View all 13 articles
There is a close symbiotic relationship among digital finance, digital rural and digital agriculture. The three promote and influence each other to jointly drive rural revitalization and agricultural modernization. Using panel data from 30 provinces in China spanning from 2015 to 2021, this paper quantitatively analyzes the dynamic characteristics, spatial patterns, and driving mechanisms of digital agriculture, digital rural and digital finance, and their symbiotic relationships through coupling coordination degree model (CCDM) and geographically and temporally weighted regression (GTWR). The study shows that symbiotic relationship between digital agriculture, digital rural and digital finance is dominated by coordinate relationship and quasi symbiotic relationship. The evolution of symbiotic indices is diversified, with emergence of a variety of patterns such as continuously declining, fluctuating upward, relatively stable, L-shaped, inverted U-shaped, W-shaped, V-shaped, tilted Z-shaped, M-shaped, and the symbiotic relationship and its changes exhibit significant spatial variability, agglomeration and correlation. The driving mechanism of symbiotic relationship is complicated, and the nature and intensity of factors are regional and time-varying. Except for fiscal self-sufficiency rate, which plays a long-term positive promotion role, most factors exert mixed effects (both positive promoting and negative inhibitory). For this reason, it is recommended that the government should change with the trend in management, balancing weak and strong interventions. And it should also accelerate the transformation of development policies toward spatial policies, scientifically delineate geographical zones, and establish spatial alliances, to enable leading regions to demonstrate their exemplary value, while also helping and assisting those underdeveloped to quickly achieve breakthrough in areas with distinctive advantages. In addition, it should design the digitalization policy portfolio according to the symbiotic mechanism, focusing on the synergistic relationship between key positive and negative factors.
Digital agriculture is a new agricultural development model that regards digital information as a new element of agricultural production, and depends on digital information to perform information management and digital research and development of agricultural production environment, objects and processes. Digital agriculture, in contrast to digital industry and digital commerce, includes digital planting, digital animal husbandry, digital fisheries, digital seed farming, digital agricultural equipment, and more. Rural digitalization is an intrinsic aspect of agricultural and rural modernization, driven by the integration of networking, informatization, and digital technologies into economic and social development, alongside the enhancement of farmers’ modern information skills (Naldi et al., 2015). Digital rural areas and digital cities (smart cities) are twin concepts. They represent not only the strategic direction of rural revitalization but also a key component of the broader initiative to build a Digital China. Digital finance represents a new generation of financial services that integrate traditional financial models with internet and information technologies. This encompasses internet payments, mobile payments, online banking, financial service outsourcing, online loans, insurance, and investment funds. Digital inclusive finance is viewed as a crucial pathway to achieving sustainable development (Tay et al., 2022). The term symbiotic originates from biology and ecology, originally referring to the close and mutually beneficial relationship between two or more different organisms. At present, the symbiosis theory is widely used in multidisciplinary fields such as agriculture, economics, management, geography, sociology, planning and engineering, to analyze the complex interactions of multiple systems (Leidner, 2018). There are complex interactive relationships among digital agriculture, digital rural and digital finance, and their collaborative symbiosis is the key to rural revitalization and development in the new era. Therefore, on the basis of analyzing the development status of digital agriculture, digital rural and digital finance, it is of great value to quantitatively evaluate the symbiotic relationship and its driving mechanism for promoting the high-quality and sustainable development of rural areas.
The essence of digital agriculture, digital rural and digital finance is the integrated application and promotion of digital technologies and devices in these fields, so developing application scenarios and building technical solutions have become priority research fields. Scholars focus on analyzing the application intentions and approaches of new generation information technologies such as blockchain, Internet of Things, machine learning, artificial intelligence, and big data in the development of agriculture, rural areas, and finance (Wu, 2022; Gong et al., 2024), and developed application scenarios such as Smart Transportation, Smart Land and Smart Governance related to agriculture and rural areas (Francini et al., 2020; Ogryzek et al., 2022). For example, Bolfe found in empirical analysis of Brazil that 84% of surveyed farmers use at least one digital technology in their agricultural production systems, and 95% hope to learn more about new technologies and are willing to apply them to the agricultural and rural development (Bolfe et al., 2020). And some scholars further discussed the difficulties and challenges faced by the application of digital technology in agriculture, rural areas, and financial development (Alabdali et al., 2023). For example, Dibbern et al. (2024) believes that economic conditions, availability of digital facilities, technical knowledge, age of farmers, organizational types, technological reliability, and concerns about security and privacy are important factors affecting the application of digital technology in agriculture. Finally, on the basis of application scenarios and technological solutions, and depending on the influencing factors, some scholars have discussed their integration into agricultural, rural, and financial development policies and spatial planning (Wojcik et al., 2021). For example, Degila et al. (2023) analyzed digital agriculture policies and innovation strategies in African countries and Nigeria, and Zhang and Zhang (2021) analyzed the characteristics of China’s digital rural strategic planning and practice.
The study focuses on analyzing the external effects incidental to the development of digital agriculture, rural digitalization, and digital finance, including economic and industrial, social and urban–rural development, food security, ecological environment, climate change, energy conservation and emission reduction, as well as comprehensive impacts involving multiple dimensions (Rolandi et al., 2021; Balasundram et al., 2023). As for economic and industrial impacts, Geng et al. (2024) believes that the development of digital agriculture reduces the labor and capital input, and improves agricultural output and quality, and that each unit increase in the intensity of digital technology adoption leads to a 30.4% increase in economic efficiency. For social impacts, Ahiase et al. (2024) argues that digital finance has a moderating effect on income inequality and a significant effect on entrepreneurial activity and risk (Konou, 2023). Wang et al. (2024), Gao et al. (2024) and Ge et al. (2022) argued in their studies that digital inclusive finance and its sub-dimensions can promote non-agricultural employment of rural labor force and play a key intermediary role in the process of improving agricultural economic resilience and industrial integration. Jamil et al. (2024) believes that digital inclusive finance is a significant contributor to the income of the rural population and plays a crucial role in the improvement of farmers’ wages, property and transfer income. In terms of urban and rural development, Jiang et al. (2022) believes that digital agriculture affects urbanization through total factor productivity improvement mechanism, agricultural division of labor acceleration mechanism, marketization process mechanism, and human capital mechanism. Guo and Zhong (2023) further pointed out that digital finance has an important impact on urban and rural migrants, especially farmers’ urban settlement. In food security, Lajoie-O’Malley et al. (2020) and Cook et al. (2022) discussed the connection between digital agriculture and food security with an attempt to integrate digital agriculture into sustainable food systems, and pointed out value creation pathways and pitfalls in the process. In regards to ecosystem and climate change, Lin et al. (2022) argued that the development of digital inclusive finance can help mitigate agricultural surface pollution, and an increase in farmers’ income, especially non-agriculturalized income, can significantly enhance the mitigation effect of digital finance on agricultural pollution. In the field of energy consumption and carbon emissions, Ma et al. (2022) and Bao et al. (2024) found that the development of digital agriculture and digital finance demonstrates obvious carbon emission reduction, and the effect is robust to different spatial weight matrices. Lee et al. (2022) further pointed out that the marginal impact of digital inclusive finance on carbon intensity has a nonlinear effect.
There are three types of interactive relationships between digital agriculture, digital finance, and digital rural, and scholars have analyzed the mutual influence between them. In the relationship between digital agriculture and digital rural, most of the focus is on the influence of the latter on the former, with less analysis of the impact of the former on the latter. Zhang and Fan (2024) believes that the digital transformation of agricultural development has significantly raised farmers’ income, and through the transmission mechanism of improving production efficiency and upgrading agricultural structure, it has promoted the achievement of digital rural and rural revitalization strategic goals. Zhao et al. (2024) found in his study based on Spatial Durbin Model that rural digitalization improves the efficiency of agricultural water use in neighboring areas. Wang and Tang (2023) analyzed the coupling relationship between China’s digital rural construction and high-quality development of green agriculture through the TOPSIS method based on entropy weight and the coupling coordination degree model, revealing their spatio-temporal distribution characteristics and influencing factors. In his study, Lu et al. (2024) found that rural digitalization significantly increases green total factor productivity in Chinese agriculture, with farmers’ entrepreneurial activities playing a key mediating role. In digital agriculture and digital finance, the main focus is on the impact of the latter on the former. Mumtaz (2024) believes that digital finance and inclusive finance have a positive impact on agricultural development, while Cao and Wang (2024) points out that digital finance further promotes the increase of agricultural output by enhancing the digitalization level of agriculture. As for digital finance and digital rural, most efforts are focused on analyzing the impact of the former on the latter. Zhang C. K. et al. (2023) believes that digital inclusive finance further enhances residents’ digital literacy by deepening technological innovation and communication infrastructure construction, and advances the construction of rural digitalization.
The academic community has conducted extensive discussions on the technological applications, external effects, and relationships between digital agriculture, digital rural, and digital finance, and has produced rich research results, providing a solid theoretical foundation for this study. However, the existing studies still have limitations, particularly in the following three areas.
Firstly, there is a complex dynamic interaction between digital agriculture, digital rural, and digital finance, but the studies often focus on pairwise relationships with fewer efforts on incorporating the three into the same analytical framework, resulting in a mismatch between theoretical analysis and real-world situations.
Secondly, digital agriculture, digital rural and digital finance are in the stage of rapid development, and most of the existing studies are based on statistical analysis and regression model analysis, with a lack of researches based on spatio-temporal econometric model, making it difficult to analyze their spatio-temporal dynamics effectively and accurately.
Thirdly, the development and management of digital agriculture, digital rural and digital finance involve multidisciplinary knowledge and technology such as agricultural economics, rural geography, informatics, finance, planning and management. However, most of the existing studies involve empirical analysis based on only single disciplinary theories and methods, and it is urgent to establish a new research framework integrating multidisciplinary theories and methods across disciplines (Klerkx et al., 2019; Shepherd et al., 2020).
This study introduces symbiosis theory and spatial econometric model in the empirical research on the complex interactions in the development process of digital agriculture, digital rural, and digital finance in China, aiming to provide a basis for sustainable agricultural development, rural revitalization, and the construction of high-quality financial service systems. This paper aims to address the following questions:
1. What are the characteristics of the development and evolution of digital agriculture, digital rural, and digital finance using time series analysis and exploratory spatial data analysis (ESDA) methods?
2. What is the symbiotic relationship between digital agriculture, digital rural, and digital finance quantitatively measured using the coupling coordination degree model (CCDM) and exploratory spatial data analysis (ESDA) methods? What are the patterns of their spatio-temporal evolution based on further analysis? These two questions are dedicated to portraying the basic picture, with a focus on analyzing the change trends, regional differences, and spatial correlation of the symbiotic relationship between digital agriculture, digital rural, and digital finance.
3. What is the impact mechanism of symbiotic environmental factors on symbiotic relationships using geographic and temporal weighted regression (GTWR) and linear regression methods, including the nature, intensity, and spatial effects of different factors?
The study area covers 30 provincial-level administrative regions in China, with a time span from 2015 to 2021 (Figure 1). Digitalization is a global development strategy in China. However, the study area does not include Tibet due to the lack of data, and does not include Hong Kong, Macau, and Taiwan due to the large differences in management systems with the mainland. In mainland China, the digital management of rural areas, agriculture, and finance is led by the government. The data is abundant and standardized. Hong Kong and Macao are small in area, neither of which has established a specialized digital management agency for agriculture or rural areas, nor can they obtain relevant data. In Taiwan region, the management entities are relatively diverse. Besides government departments, non-governmental organizations, agricultural cooperatives, etc. all play important roles. This leads to contradictions in data among different departments and entities. China attaches great importance to the digitalization of agriculture, rural areas and finance, and the process of China’s digital agriculture, digital rural and digital finance has a leading edge in the world and especially, they are typical representatives among developing countries (Liang et al., 2003). China has introduced a series of special policies to promote the development of digital agriculture, digital rural and digital finance in recent years, including the Plan for Digital Agriculture and Rural Development (2019–2025), the Outline of the Digital Rural Development Strategy, the Digital Rural Construction Guidelines 2.0, and the Implementation Opinions on Promoting the High-Quality Development of Inclusive Finance (GF [2023] No. 15). Therefore, the empirical research on the symbiosis mechanism of digital agriculture, digital rural and digital finance in China will provide beneficial enlightenment for the agricultural and rural modernization in other countries, especially developing countries.
There is a high degree of synergy between digital agriculture, digital rural and digital finance, with digital technologies playing a pivotal role in their mutual promotion and mutual influence. Digital technology eliminates the financial exclusion faced by agriculture and rural development by restructuring the financial service network (Alonso et al., 2022). By integrating resources and businesses entire agricultural pre-production—mid production—post production cycle, it enhances agricultural added value and economic benefits, and solves the problem of low efficiency in agricultural development. And in response to the challenges of rural decline and marginalization, it promotes rural revitalization through digital catalysis in production and digital sharing in life. The key to the symbiotic development of digital finance and digital agriculture is to eliminate obstacles in the resource element circulation between different industries for the integrated development between finance and agriculture. The key to the symbiotic development of digital finance and rural digitalization is to maintain a balance between capital supply and demand and to help urban funds and resources flow to rural areas to realize two-way capital flow between urban and rural areas. Digital agriculture and digital rural are ecosystems for each other, showing that the construction of rural digitalization provides a high-quality external business environment for the development of digital agriculture. The development of digital agriculture promotes the digital transformation of rural industries and governance, injecting new vitality into rural revitalization. The research on the symbiotic mechanism of digital agriculture, digital rural and digital finance is a complex systematic project. It requires the comprehensive application of information asymmetry, financial exclusion, spatial differentiation, spatial correlation, symbiosis theory, and the integration of multidisciplinary technologies and methods such as agricultural economics, rural geography, information science, finance, and management to establish an interdisciplinary research framework that integrates “symbiotic dynamics symbiotic relationships symbiotic mechanisms” (Deichmann et al., 2016; Romera et al., 2024; Figure 2).
In the field of exploring complex relationships among multiple systems, the coupling coordination degree model (CCDM) is the most commonly used empirical method in the theoretical framework of systems science. CCDM is widely used to analyze the symbiotic effects between economic development and ecological protection, industrial growth and population change, land use and ecological services, and other fields. The analysis of the symbiotic relationship between digital agriculture, digital rural and digital finance is essentially a measurement of the synergy between their digital systems. The key to analyzing the symbiotic relationship between digital agriculture, digital rural, and digital finance using CCDM is to calculate the coupling degree (), coordination degree (), and coupling coordination degree (), which are calculated as follows (Wang et al., 2023):
Where, , and represent the development indices of digital agriculture, digital rural and digital finance in the -th province in the -th year; , and represent the weights of the digitalization system of agriculture, rural and finance, respectively, which are calculated by entropy weight method. The coupling coordination degree is calculated using the SPSSPRO software with values ranging from 0 to 1. A larger value represents a better the symbiotic relationship between digital agriculture, digital rural, and digital finance, and a more significant synergistic development effect. Based on the findings of Lai et al. (2024), Xu and Tian (2022) and other scholars, the coupling coordination degree is classified into five levels in this study. Where, 0–0.2 (including 0.2) indicates that digital agriculture, digital rural and digital finance have a very low degree of symbiosis, and the three are broadly independent, marked as an Independent Relationship. A level of 0.2–0.4 (including 0.4) indicates a low degree of symbiosis among the three, only in a simple mutual coordination and coordinated development, marked as Coordinate Relationship. A range of 0.4–0.6 (including 0.6) indicates that the three are already in a synergistic stage of development, marked as Quasi Symbiotic Relationship. A value of 0.6–0.8 (including 0.8) indicates that the three are in a symbiotic development stage, with good competition and cooperation order within the system, marked as Symbiotic Relationship. At 0.8–1.0 (including 1.0), it indicates that the three have been in a high-quality symbiotic development stage, full competition and cooperation have been realized in the system, and the three are interdependent and mutually reinforcing, marking as Super Symbiotic Relationship.
With the surge of scientific and technological revolution, especially after the COVID-19 outbreak, the digitalization of agriculture, rural areas and finance is in the process of rapid development, and the state of different regions and their relationship with neighboring regions are always in the process of dynamic changes. Traditional statistical regression analysis methods often overlook the spatiotemporal non-stationary characteristics of digitalization. The spatio-temporal geographic weighted regression model (GTRW) is an improvement of the geographic weighted regression model (GWR), which integrates temporal variability into the GWR regression model and allows for analysis of the influence mechanism of factors in different spatio-temporal dimensions (Fotheringham et al., 2015). For this purpose, this study chooses to use GTRW to analyze the symbiotic mechanisms of agricultural, rural, and financial digitization, integrating geospatial effects and temporal non-stationarity. It is calculated are as follows (Mirzaei et al., 2019):
Where, represents the symbiotic index of agricultural, rural and financial digitization in the -th province; is the -th influence factor in the -th province; is a constant term, is the spatio-temporal coordinate of the -th province, is the correlation between different influence factors in the -th province, and is the error of the regression equation.
The dependent variable is coupling coordination degree index between digital agriculture, digital rural, and digital finance, later referred to as coupling coordination degree index. It is calculated from digital agriculture index (Li W. et al., 2023), digital rural index (Li and Zhang, 2024), and digital finance index (Guo et al., 2020) based on CCDM. The symbiotic environment has a significant impact on the generation and evolution of symbiotic relationships, and this article selects 10 indicators as independent variables. Data standardization, normalization, and normalization are done using the maximum and minimum value methods.
1. Fiscal self-sufficiency rate (): The digitalization of agriculture, rural areas, and finance requires large amounts of capital and financial investment, and government financial support has become an essential key point (Chandio et al., 2024). This study uses the fiscal self-sufficiency rate to measure the financial support capacity of local governments, with data sourced from the China Statistical Yearbook.
2. Growth rate of fixed assets investment (): The development of digital agriculture, digital rural and digital finance has brought immense fixed asset investment in information and digital infrastructure, including 5G base stations, broadband networks, digital equipment for farmland and water conservancy facilities, e-commerce venues, sensors and Internet of Things equipment. The application and promotion of digital technology requires large fixed assets investment. The growth rate of fixed assets investment is used to present the impact of government (including farmers) assets and investment on digitalization in this study (Huang et al., 2023). The data is from China Statistical Yearbook, China Rural Statistical Yearbook and China Agricultural Yearbook.
3. Average years of education in rural areas (): The development of digital agriculture, digital rural and digital finance involves the application of a large number of emerging information technologies and equipment, requiring users to have a matching knowledge system. Education and knowledge structure play a key intermediary role in digitalization (Liu et al., 2023). In this study, the average years of education in rural areas is used to measure the impact of farmers’ knowledge structure and digital literacy, which is calculated as (primary school education ×6 + junior high school education × 9 + senior high school education × 12 + associate degree or above ×16)/total rural population. The data is sourced from the China Statistical Yearbook and the China Education Statistical Yearbook.
4. Aging population (): The lack of acceptance and application of digital technologies and devices by the elderly population also has a negative impact on digitalization (Li X. et al., 2023). This study measures aging based on the proportion of people aged 65 and over, with the data from the samples of the national population change survey released by the National Bureau of Statistics.
5. Proportion of rural migrant workers (): Rural migrant workers are predominantly young and strong rural laborers, and they represent the loss of agricultural and rural human capital. Significant labor outflow will lead to a shortage of physical and intellectual resources in the process of digitalization in agriculture and rural areas. In this study, the proportion of rural migrant workers is used to measure their impact. It is calculated as the ratio of the rural migrant workers to the total rural laborer population. The data is sourced from the China Statistical Yearbook, China Rural Statistical Yearbook, and the Nationwide Monitoring Survey Report on Migrant Workers.
6. Agricultural new quality productivity index (): The development of agricultural new quality productivity involves a large number of innovative elements in agriculture and rural laborers, labor objects, and labor materials, which play an important role in promoting digitalization. The data is sourced from Luo and Song (2024).
7. Farmer entrepreneurship activity index (): It is calculated as the proportion of rural individual entrepreneurs and rural private enterprise employment to rural employment, with a larger value representing higher entrepreneurial activity among farmers. Digital finance reduces the financing cost for farmers’ entrepreneurship, and farmers’ entrepreneurial activities drive the development of digital agriculture and the construction of rural digitalization. In this study, the farmer entrepreneurship activity index is used to measure its mediating role on the symbiotic development of digital agriculture, digital rural and digital finance. The data is sourced from Cheng et al. (2024).
8. Rural revitalization index (): Rural revitalization is the goal of the development of digital agriculture, digital rural and digital finance, and the extent to which it is implemented greatly affects the process of digitization. The data is sourced from Xiong et al. (2024).
9. Land transfer rate (): digital technology and digital finance integrate the land transfer market and improve the efficiency of land allocation, thus accelerating the transfer of rural land (Zhang et al., 2022). Land transfer is a prerequisite for agricultural large-scale digital management and also an important part of digital rural construction. The land transfer rate is measured by the proportion of the transfer area to the total contracted arable land area of households. The data is sourced from the China Rural Management Annual Report and the China Rural Statistical Yearbook.
10. Rural power consumption (): The application of digital technology, equipment, and equipment operation largely rely on the support of electricity resources. The rapid digitalization in agriculture, rural areas, and finance has driven the expansion of rural electricity demand and had a significant impact on electricity consumption (Li, 2023). The data is sourced from the China Rural Statistical Yearbook, China Statistical Yearbook, and China Electric Power Statistical Yearbook.
From 2015 to 2021, the digital agriculture index is all in a state of growth, with Zhejiang having the highest growth rate of more than 10%, while Liaoning at the bottom with less than 3%. Nearly half of the provinces are growing at more than 5%, with the western regions like Guizhou, Qinghai, Sichuan, Xinjiang, Guangxi and Gansu leading the way. It is noteworthy that the development in Jilin, Tianjin, Liaoning, Inner Mongolia, Heilongjiang and other regions in North China, especially Northeast China, is slow, generally at 2–4%. The COVID-19 outbreak is generally regarded as a catalyst for digitalization, but its boost to digital agriculture is not significant. Only one-third of the provinces saw an increase in the development rate of the digital agriculture index after the COVID-19 outbreak, including Liaoning, Jilin, Heilongjiang, Zhejiang, Anhui, Hainan, Chongqing, Shaanxi, Guizhou, and Xinjiang. Most provinces had accelerations of around 0.5 times, but there was an explosive change in Zhejiang, where digital agriculture grew more than 5.5 times faster than before after the pandemic (Table 1).
Compared with the digital agriculture index, the digital rural index had a higher growth rate. The digital rural indices in 2015–2021 were all in growth, with Gansu showing the highest growth rate of more than 20%; Beijing, though at the bottom of the list, was also growing at nearly 8%. More than 75% of provinces had a growth rate of more than 10%, except for Chongqing, Xinjiang and Hunan exhibiting a growth rate of about 15%. It is a remarkable fact that the COVID-19 outbreak has had a huge impact on the rural digitalization, and 80% of provinces experienced a decrease in the development speed of the digital rural index after the pandemic, including Hainan, Jiangsu, Zhejiang, Fujian, and Guangdong. In particular, Inner Mongolia changed from rising to falling. After the pandemic, only six provinces of Shanxi, Liaoning, Heilongjiang, Shaanxi, Gansu, and Xinjiang showed an increase in digital rural development, especially Xinjiang and Shanxi, which doubled their growth rate (Table 1).
The development rate of the digital finance index was somewhere between the digital agriculture index and the digital rural index, neither radical nor conservative. The digital agriculture indices from 2015 to 2021 were all in growth, with Henan having the highest growth rate, and Anhui and Jiangxi not far behind, both exceeding 10%. Liaoning was at the bottom of the list, not far behind Jilin, Hainan, Heilongjiang, Beijing, Inner Mongolia, and Ningxia, all growing at around 8%. It is rather remarkable that the COVID-19 outbreak had a significant inhibitory effect on the speed of financial digitalization. After the outbreak of the epidemic, only two provinces, Inner Mongolia and Ningxia, grew faster than before, while other provinces all slowed down. The decline in Zhejiang, Beijing, Guangdong, and Shanghai was significant, only 50 to 60% of the growth rate before the outbreak. All provinces were in positive growth before and after the COVID-19 outbreak, with no negative growth (Table 1).
The coefficients of variation for the coupling coordination degree index in 2015, 2017, 2019, and 2021 were 0.43, 0.44, 0.47, and 0.45, respectively, indicating high spatial heterogeneity. The number of members in each of the five levels shows an olive-shaped structure, with the number in independent relationship and super symbiotic relationship being very small, the number in coordinate relationship and symbiotic relationship gradually expanding (especially faster for the latter), and the number in quasi symbiotic relationship being the largest, but showing a decreasing trend. Membership in the super symbiotic relationship and the symbiotic relationship remained stable for a long time, all located in the eastern and coastal regions. The former always included only Beijing, Shanghai, Zhejiang, while the latter always included only Jiangsusu, Guangdong, Tianjin, Fujian. The membership of the independent relationship was sparse and except for Qinghai, the composition of members varied in different periods, but they were all located in the western region. The members were Qinghai, Gansu and Guizhou in 2015, Gansu and Qinghai in 2017, Gansu, Xinjiang and Qinghai in 2019, and Yunan and Qinghai in 2021. The composition and membership of quasi symbiotic relationship and coordinate relationship were constantly changing, and only some members remained unchanged, including Sichuan, Shandong, Hubei, Henan, Hainan, Chongqing, Anhui in the former, and Yunan and Shanxi in the latter (Figure 3).
For quasi symbiotic relationship, the members in 2015 were Shandong, Liaoning, Hainan, Hubei, Chongqing, Inner Mongolia, Anhui, Shaanxi, Jilin, Sichuan, Ningxia, Heilongjiang, Jiangxi and Henan, concentrated in the central and northeast regions. The members in 2017 were Shandong, Hubei, Chongqing, Guangxi, Hainan, Anhui, Liaoning, Inner Mongolia, Shaanxi, Hunan, Henan, Hebei and Sichuan, with a geographic distribution similar to that of 2015, contracting in the northeast but expanding to the south. The members in 2019 were Shandong, Hubei, Chongqing, Henan, Hainan, Anhui, Hebei, Jiangxi, Sichuan and Hunan, with a rapidly shrinking geographic coverage, especially in the northeast. The members in 2021 were Shandong, Hubei, Anhui, Chongqing, Henan, Hunan, Sichuan, Shaanxi, Hainan, Hebei, and Jiangxi, with the geographical distribution pattern largely similar to that in 2019. As for coordinate relationship, the members in 2015 were Hebei, Hunan, Shanxi, Guangxi, Xinjiang and Yunnan, mostly clustered in the southwest and Beijing-Tianjin-Hebei region. The members in 2017 were Jiangxi, Heilongjiang, Jilin, Ningxia, Xinjiang, Guizhou, Shanxi and Yunnan, scattered in distribution, mostly located in border areas. The members in 2019 were Shaanxi, Liaoning, Guangxi, Inner Mongolia, Ningxia, Jilin, Heilongjiang, Shanxi, Yunnan and Guizhou, with the majority clustered in the north (including the northeast) and a small portion clustered in the southwest. The members in 2021 were Guangxi, Liaoning, Inner Mongolia, Ningxia, Heilongjiang, Xinjiang, Jilin, Guizhou, Shanxi and Gansu, mostly clustered in northern China.
The global Moran’s I value for the coupling coordination degree index in 2015, 2017, 2019, and 2021 were 0.10 (p < 0.001, Z = 4.03), 0.10 (p < 0.005, Z = 4.03), 0.11 (p < 0.003, Z = 4.29), and 0.11 (p < 0.003, Z = 4.16), respectively, all showing significant positive spatial autocorrelation. Similar to the spatial association pattern of the HH space, the L-L space was better developed, concentrated in contiguous distribution in the west, covering a large geographic area, with only minor adjustments occurring in different years. In contrast, the H-H space was clustered in a band along the eastern coast, covering a small geographical area including Anhui, Jiangsu, Zhejiang and Fujian. The L-H and H-L spaces were badly underdeveloped, scattered in isolated points, such as Chongqing, Beijing and Jiangxi.
The changes in coupling coordination degree index from 2015 to 2021 show diverse trends, with the emergence of continuously declining, fluctuating upward, relatively stable, L-shaped, inverted U-shaped, W shaped, V-shaped, tilted Z-shaped and M-shaped. Beijing, Tianjin, Fujian, Jiangxi were tilted Z-shaped, that is, they experienced the zigzagging change of first falling, then rising, then falling. Hebei, Guangxi, and Shaanxi were relatively stable, fluctuating slightly around a certain numerical value. Sichuan was violently volatile, differing from the relatively stable type in that the fluctuations were much greater. Shanxi and Qinghai were L-shaped, i.e., declining rapidly and then remaining relatively stable or fluctuating slightly up and down. Inner Mongolia, Shanghai, Jiangsu, Shandong, Henan, and Guangdong were inverted U-shaped, i.e., undergoing first upward and then downward, a type with the largest number of members. Lianing, Jilin, Heilongjiang, Hainan, and Ningxia continued to decline, the type with the second largest number of members. Zhejiang and Gansu were W-shaped, i.e., undergoing successive changes of decreasing-ascending-decreasing-ascending. Hubei, Hunan, Yunnan were M-shaped, with characteristics opposite to those of the W-shaped. Anhui and Guizhou experienced significant fluctuations and upward movements, i.e., they generally showed an overall increase in index values during the process of large fluctuations. Chongqing fluctuated sharply downward, with changes opposite to those sharply fluctuating upward. Xinjiang was V-shaped, showing a rapid decline followed by a rapid rebound (Figure 4).
According to the growth rate of the coupling coordination degree index from 2015 to 2021, most experienced a decline, with less than one-third seeing growth. The largest declines were recorded in Heilongjiang, Inner Mongolia, Liaoning, Jilin, with an average annual decline of over 5%. Guizhou, Gansu, Hunan, Henan, Anhui, Zhejiang, Hebei, Guangxi, Jiangxi recorded positive growth, with Guizhou and Gansu well ahead, the former exceeding 10% and the latter close to 5%. A comparison of the growth rates for 2015–2019 and 2019–2021 shows a diversification of trends before and after the COVID-19 outbreak, suggesting a regional role for digitalization. Beijing, Tianjin, Inner Mongolia, Shanghai, Jiangsu, Shandong, Hainan, Chongqing, Sichuan and Yunnan kept declining and more rapidly after the pandemic; Hunan and Gansu enjoyed a long-time growth and began to slow down after the pandemic. It indicates that the impact of the COVID-19 outbreak may have played a dampening role with a stronger influence on the former. Shanxi, Liaoning, and Jilin experienced a long-time decline, but it gradually slowed down and was effectively controlled after the pandemic, suggesting that the COVID-19 outbreak stimulated the digitization process, but with limited effect. The long-term growth in Anhui and Guizhou, with a faster growth after the pandemic, shows that the COVID-19 outbreak significantly accelerated digitization. Hebei, Fujian, Jiangxi, Henan, Hubei, Guangdong, and Guangxi all experienced a reversal in their trends from growth to decline before and after the pandemic, while Heilongjiang, Zhejiang, Shaanxi, Qinghai, Ningxia, and Xinjiang experienced a shift from decline to growth before and after the pandemic. It suggests that the COVID-19 outbreak plays a key role in the digitization process, with the former highlighted as inhibitory while the latter as facilitating (Figure 5).
The medium and high growth areas of the coupling coordination degree index from 2015 to 2021 were concentrated in the eastern and central regions, showing a “center-periphery” structure in the geographical distribution, with the low growth areas located in the northeast. In the same period, the global Moran’s I was 0.03 (p < 0.10, Z = 1.79), indicating that the changes in symbiotic relationships have a relaxed positive spatial autocorrelation. The spatial pattern of the growth rate of the coupling coordination degree index experienced significant changes before and after the pandemic. Before the pandemic, the high growth space was concentrated in the pan-Pearl River Delta region and expanded to the Yellow River basin; the medium growth space was in the eastern coastal area and the Chengdu-Chongqing city cluster, while the low growth space was in the west and north (including the northeast). After the pandemic, the high growth space was concentrated in the northwest, most of the medium growth space was concentrated in the lower reaches of the Yellow River Basin, while the low growth space was scattered, only relatively concentrated in the northeast. The global Moran’s I value before and after the pandemic were 0.05 (p < 0.03, Z = 2.50) and − 0.01 (p < 0.10, Z = 0.82), respectively, shifting from significant positive spatial autocorrelation to insignificant spatial negative correlation. And before the pandemic, the H-H space was clustered in the Pan-Pearl River Delta, and the L-L space in the northeast; after the pandemic, the H-H space was clustered in the northwest, and the L-L space was underdeveloped, only including Hebei, Liaoning, and Hainan (Figure 6).
According to the previous analysis, the coupling coordination degree index between digital agriculture, digital rural, and digital finance has significant spatial heterogeneity and spatial autocorrelation, and the analysis of the driving mechanism of the influencing factors requires the use of spatial econometric models. Before starting regression analysis using GTWR, it is important to ensure no significant collinearity between different factors. Table 2 shows the VIF (variance inflation factor) parameters in linear regression analysis, all less than 10, indicating weak collinearity among the 10 factors and high levels of goodness of fit and significance. These factors are fully suitable for empirical analysis using GTWR. GTWR has a goodness of fit of 0.91 with a high level of confidence.
According to the comparative analysis of the minimum and maximum values of GTWR regression coefficients in 2015, 2017, 2019 and 2021, most of the factors showed mixed effects, and none exerted a negative inhibitory effect. In 2015, only the fiscal self-sufficiency rate () and farmer entrepreneurship activity index () had a positive promoting effect. In 2017, the positive factors shifted to the fiscal self-sufficiency rate () and growth rate of fixed assets investment (). In 2019, only the fiscal self-sufficiency rate () was available. In 2021, fiscal self-sufficiency rate () and land transfer rate () were included. Overall, only the fiscal self-sufficiency rate () had a long-term positive promoting effect, while the growth rate of fixed assets investment (), farmer entrepreneurship activity index (), and land transfer rate () had a positive promoting effect in a given year (Table 3). Most factors exhibit mixed effects, indicating that the driving mechanism of factors is very complex. For different provinces, there are differences in the intensity of action, besides property reversals.
According to the comparative analysis of the mean value of GTWR regression coefficients in 2015, 2017, 2019 and 2021, it is possible to analyze the intensity of the influence of each factor as a whole and to classify the influence factors into three categories: key, important, and auxiliary. Fiscal self-sufficiency rate () and land transfer rate () have much higher influence than other factors, and their influence is increasing, so they are key influence factors. Farmer entrepreneurship activity index (), rural power consumption (), rural power consumption (), and proportion of rural migrant workers () are significant factors because their impact is high most of the time or very high in a given year, much higher than the other factors. Growth rate of fixed assets investment (), average years of education in rural areas (), and aging population () are auxiliary influence factors, and their influence is low in most years (Table 4).
The strong influence space of the fiscal self-sufficiency rate () in 2015 was concentrated in the Pan Pearl River Delta and northwest China, and its influence in the upper and middle reaches of the Yangtze River Basin should not be ignored in 2017, the strong influence space contracted in the northwest and expanded in the northeast, while basically remaining unchanged in the Pan Pearl River Delta and the middle reaches of the Yangtze River Basin. The strong influence space in 2019 was clustered mainly in the northeast and the Pan Pearl River Delta region. In 2021, the strong influence space expanded from northeast to northwest, extending from the Pan Pearl River Delta to the Yangtze River Delta (Figure 7).
Figure 7. Spatial effect of symbiotic environment influence for coupling coordination degree index in China.
The strong positive influence space of the growth rate of fixed assets investment () was clustered in Fujian and Jiangxi in 2015 and expanded to the north. The strong negative influence space was concentrated in the southern coast, expanding from Hainan and the Pan-Pearl River Delta to the Chengdu-Chongqing urban agglomeration. The weak negative influence space was concentrated in the northern coast, expanding from Jiangsu to Liaoning and Heilongjiang in 2017, the strong influence area playing a positive role was concentrated in the Yangtze River Basin and gradually decreased to the peripheral areas, forming a “center periphery” spatial pattern. In 2019, the strong positive influence space was concentrated in the south, while the strong negative influence space was concentrated in the north. The geographical pattern of positive and strong negative influence space in 2021 did not change much, with the former still centered around the south and the latter gradually forming a cluster area centered in the Beijing-Tianjin-Hebei region (Figure 7).
In 2015, most of the strong positive influence space of the average years of education in rural areas () was concentrated in the southwest and formed a small cluster in the northeast. It had a strong negative influence on Xinjiang and a moderate negative influence on the Yangtze River Delta urban agglomeration. In 2017, the strong positive influence spaces were still centered in the southwest and expanded to the northwest. The strong negative influence spaces were distributed in the lower reaches of the Yangtze River and the Yellow River, and extended to the middle reaches. In 2019, the strong positive influence spaces basically remained unchanged, but the strong negative influence spaces were clustered in the northeast and expanded to the east along the coast. In 2021, most of the strong positive influence spaces were clustered in the southwest and the weak positive influence spaces were in the northeast. The strong negative influence spaces were located in Xinjiang, while the weak negative influence spaces were concentrated in the eastern coastal areas (Figure 7).
The strong negative influence spaces of the aging population () in 2015 were located in the northwest and expanded to the east and southwest Most of the strong negative influence spaces were concentrated in the northeast, with a small portion in the Beibu Gulf region. In 2017, the strong positive influence spaces were concentrated in the east and northwest, and strung together by the Yellow River Basin. The weak positive influence spaces were concentrated in the northeast, while the strong negative influence spaces were in the Beibu Gulf and its surrounding areas. In 2019, the strong positive influence spaces were concentrated in the eastern coast and gradually decreased and expanded toward the inland, while the strong negative influence spaces were in the Beibu Gulf region. In 2021, the strong positive influence spaces were concentrated in the Beijing-Tianjin-Hebei region and the lower reaches of the Yellow River, while the weak influence spaces were concentrated in the central region and expanded to the west and northeast. The strong negative influence was found only in Xinjiang, while the weak influence was in the Beibu Gulf region (Figure 7).
The positive influence spaces of the proportion of rural migrant workers () in 2015, 2017, 2019 and 2021 were nearly the same, all clustered in the east. In 2015 and 2017, the positive influence spaces were centered on Fujian, Zhejiang, and Jiangsu, gradually decreasing from coastal to inland, forming a gradient spatial pattern. In 2019 and 2021, the spatial coverage of positive influence shrank significantly, and high influence centers degraded and transitioned. In 2021, Xinjiang became an enclave with strong positive influence. Most of the negative influence spaces were clustered in bands in the south-western and north-western regions, with the location of the strong influence poles shifting across the region. The poles of the early strong negative influence spaces were located in the Yunnan-Sichuan-Qinghai region, and then shifted to Xinjiang and finally to Xinjiang and Ningxia (Figure 7).
The agricultural new quality productivity index () had a positive promoting effect on most regions in a long time, except a negative inhibitory effect on some areas such as Yunnan, Hainan, and Xinjiang in a given year. The strong positive influence spaces were in a “center periphery” structure, and the position of the strong influence center was constantly changing. In both 2015 and 2017, there were dual centers, located in the northwest and central regions for the former, and in the northwest and eastern coastal areas for the latter. There was a single center in 2019 and 2021, located in the north, covering an increasingly larger geographic area (Figure 7).
The rural entrepreneurship activity index () had a positive promoting effect on most regions, but the coverage of negative inhibitory effects was gradually expanding. In 2015, the positive influence spaces gradually decreased from west to east, exhibiting a gradient decay trend. The spatial pattern of influence in 2017 was similar to that in 2015, but the coverage of strong influence spaces significantly expanded, with negative influence spaces emerging in the southern coastal areas. In 2019, the strong positive influence spaces were clustered in the southwest, while the weak influence spaces were clustered in the central region and expanded to the northeast. Most of the negative influence spaces were concentrated in the southern coastal area, and the negative influence was stronger in Guangdong, Fujian and Xinjiang. In 2021, the coverage of the strong positive influence spaces further shrank toward the southwest, and the influence of the factor on the central region further decreased, facing the risk of a shift from positive promoting effects to negative inhibitory effects. The negative influence spaces expanded northward in coastal areas, but the influence decreased significantly (Figure 7).
The positive and negative influence spaces of the rural revitalization index () were roughly equivalent in the early stage, while the negative influence was dominant in the later stage. The geographical distribution of positive and negative influence spaces in the early stage was characterized by east–west separation and agglomeration, which shifted into north–south separation and aggregation in the middle stage, and then into a north–south gradient change in the later stage in 2015, the positive influence spaces were concentrated in the west, with two centers in the southwest and northwest. Most of the negative influence spaces were concentrated in the east, centered on the middle and lower reaches of the Yangtze River and Yellow River, in a “center-periphery” structure. The strong positive influence space in the southwest remained relatively stable in 2017, while the influence shifted from positive to negative in the northwest (mainly Xinjiang). Most of the negative influence spaces were still concentrated in eastern China, but the coverage area, especially the strong influence area, shrank significantly. In 2019, the strong positive influence spaces were clustered in the south (Hainan, Guangdong and Guangxi) and the strong negative influence spaces were in the north. In 2021, the negative influence decreased in a gradient from north to south, and the strong influence spaces expanded from north to southwest (Figure 7).
The land transfer rate () had a long-term positive promoting effect on most regions, while the negative effect was mainly found in some areas in the early stages, which gradually decreased in the later period. In 2015, 2017 and 2019, the strong positive influence spaces were concentrated in the north, with an expanding geographic coverage. In 2015, the negative influence spaces were concentrated in the southwest, while in 2017 and 2019, they mainly had a strong influence on Xinjiang and Yunnan, with little influence on other regions. In 2021, all factors had a positive influence, and the strong influence center was still located in the north, but gradually declining toward other regions (Figure 7).
In 2015, the rural power consumption () had a negative inhibitory effect on most regions, with strong negative influence spaces concentrated in the north and strong positive influence spaces in the southwest. In 2017, the positive and negative influence spaces were roughly equivalent, with strong positive influence spaces clustered in the southwestern and northwestern regions and strong negative influence spaces clustered in the north. In 2019, the positive influence spaces significantly expanded, but the intensity of influence decreased relatively. During the same period, the negative influence spaces were still concentrated in the north, but the coverage area significantly shrank. The spatial pattern of influence in 2021 was similar to that in 2019, but the positive influence space agglomerations in the south began to shrink (Figure 7).
The change trend shows that digital agriculture in western China develops rapidly and has great growth potential, but it does not change the overall development lag; the east is leading in the development of digital agriculture, but its growth capacity is gradually weakening, and it needs to innovate the development pattern in the future, or else it will be caught up or even overtaken by the central and western regions; Hubei, Shaanxi, Anhui and Sichuan will become the most promising challengers to the existing order. The digital rural is growing at a very fast pace, but is also being hit hard by the pandemic and is seeing a shift from growth to decline in some areas. Digital finance is somewhere between digital agriculture and digital rural in development speed, and the COVID-19 outbreak has a significant inhibitory effect on it. The changes in the symbiotic relationship between digital agriculture, digital rural, and digital finance are increasingly diverse, giving rise to a variety of patterns such as continuously declining, fluctuating upward, relatively stable, L-shaped, inverted U-shaped, W-shaped, V-shaped, tilted Z-shaped, and M-shaped. It is worthwhile to note that the empirical analysis finds that the impact of the COVID-19 outbreak is time-varying, inconsistent with expectations and the analysis results of other scholars (Zhang et al., 2023). The possible reason for this case is that previous studies by other scholars mainly focused on case analysis or qualitative analysis, while this study is a large-scale analysis covering the entire domain. The finding of coexisting inhibitory and promoting effects of the COVID-19 outbreak in this study precisely suggests that there may be a survivor bias in the earlier case studies, which led to differences in the analytical results due to case selection. In general, digital agriculture, digital rural and digital finance and their symbiotic relationship are changing rapidly, with diversified trends, and the impact of the COVID-19 outbreak is variable. Therefore, management policy design should comply with the change trend, and countercyclical intervention is needed to incorporate hard management and soft management strategies into the same strategic planning framework (Kulikov et al., 2019). Hard management and soft management are two different yet complementary management strategies. The former is a rigid management system centered around digital rural areas, digital agriculture, and digital finance, composed of a series of rules and regulations, organizational structures, administrative orders, etc., highlighting compulsion and restrictiveness. The latter is a flexible and supplementary management system constructed by applying rural culture, farmers’ values and emotions, and various incentive measures, aiming to stimulate the enthusiasm, initiative, and creativity of those being managed. Symbiotic development requires the coordinated changes of different elements. If the development of digital rural areas is significantly ahead of that of digital agriculture and digital finance, damaging the coordinated symbiosis, the government should adopt counter—cyclical intervention in rural digital development. Through a combination of hard and soft measures, the development resources of digital rural areas should be moderately transferred to the development fields of digital agriculture and digital finance, promoting the coordination among the three.
According to spatial distribution, the coupling coordination degree index of digital agriculture, digital rural and digital finance and its changes showed high spatial heterogeneity and positive spatial autocorrelation, and the L-L space was better developed, concentrated in the western region, covering a large geographical range. Overall, digital agriculture, digital rural and digital finance and their symbiotic relationship, exhibit significant spatial heterogeneity and spatial autocorrelation. Their enlightening value lies in accelerating the transformation of development policies toward spatial policies and from inclusive policies to distinctive policies. The current policies and plans regarding digital agriculture, digital rural, and digital finance are all development oriented, with the same measures and strategies for all regions, and the relevant laws, technologies, facilities, educational support services and guarantee systems are inclusive (Sadjadi and Fernández, 2023). In the future, government decision-making should change from policy design dominated by development planning, implementation opinions and action plans to spatial planning. It is necessary to delineate spatial policy zoning according to geographical distribution characteristics, and establish regional development alliances according to neighborly relations (Zhang et al., 2023). For example, for regions with a high degree of spatial autocorrelation, the government, agricultural enterprises, e—commerce platforms, telecommunications operators, financial institutions, scientific research institutions and universities should jointly establish a regional development alliance. They should jointly analyze the current situation and existing problems of the symbiotic development of digital rural areas, digital agriculture, and digital finance within the region, and investigate the demands and participation methods of all parties involved in the construction. Based on this, they can design management policies, build a resource—sharing platform, and establish a cooperation mechanism.
The quantity structure of the symbiotic relationship between digital agriculture, digital rural, and digital finance is characterized by an olive shape, with most members in a transitional period of quasi symbiotic relationship. Few areas are already in symbiotic relationship and super symbiotic relationship, with large gaps between them and the intended objectives of the relevant plans and policies. And very few areas are in independent relationship, indicating that local governments have realized the necessity of symbiotic development, but still face many challenges and dilemmas in the process of practice without achieving the expected goals. Most members are in coordinate relationship and quasi symbiotic relationship. It is particularly noteworthy that the number of formers is expanding, indicating that the forward evolution of the symbiotic critical state of digital agriculture, digital rural and digital finance still faces great challenges. Besides, the driving mechanism of symbiotic relationship is complex, and the influence nature and intensity of different factors vary greatly in different regions. Currently, the central policy has adopted symbiosis as the new concept of sustainable development for digital agriculture, digital rural and digital finance, but local governments have no sufficient basis and means to drive their symbiotic development. This study maps out the current characteristics and change trends of symbiotic relationship in different regions while identifying the key influence factors that affect the generation and evolution of symbiotic relationship in each region, which is the greatest innovative academic contribution. The government may, in the future, designate regions in symbiotic relationship and super symbiotic relationship as national pilot projects for symbiotic development of digital agriculture, digital rural and digital finance, and take regions in independent relationship as the target of key assistance, to drive the symbiotic development of more regions through demonstration and external assistance. When designing policies, each region should create a more accurate policy combination to stimulate digitalization based on the operating characteristics of its own driving mechanism and around key positive promoting and negative inhibitory factors (Latino et al., 2024). For example, in Xinjiang and the eastern coastal areas, the growth rate of fixed—assets investment plays a positive role, indicating that it is beneficial to continuously increase investment in fixed assets such as digital infrastructure in the future. However, in regions such as Inner Mongolia, Hebei, and Beijing, its effect turns negative. Therefore, future policy design should focus on cultivating new—quality agricultural productivity rather than fixed—assets investment.
Compared with existing literature, the findings of this study are consistent or similar to the views of some scholars, except for some new perspectives and discoveries. Scholars have in the past paid more attention to social inequalities in agricultural, rural and financial digitalization, such as analyzing differences in digital technological innovations and the distribution of their added value among different groups, and then differences in digital literacy (digital skills and knowledge), digital problem definition and problem-solving abilities among different groups (Hackfort, 2021). For spatial disparities, more focus has been placed on the national scale at the macro level, while empirical studies at the meso-micro scale at the provincial level are just emerging. Due to significant differences in development conditions and environments across different regions, different countries and regions have different development attitudes, performance and change trends toward digital agriculture, digital rural and digital finance. In most cases, digitalization is regarded as a new round of major innovation and advanced development model in the fields of agriculture, rural areas, and finance (Runck et al., 2022; Rodino et al., 2023), and a new approach to sustainable development (Adamowicz and Zwolinska-Ligaj, 2020). However, some countries and regions (such as Australia, India and Africa) think that the technical systems of digital agriculture, digital rural and digital finance are inherently immature, resulting in unsatisfactory performance and often degenerating into temporary strategies for doers (Nitturkar, 2021; Hansen et al., 2023). In addition, the development level of digitalization in agriculture, rural areas and finance in different countries varies greatly. Puntel et al. (2023) believes that Brazil and Argentina are much higher than Uruguay and Chile. Compared to countries on a global scale, different provinces in China are not weak in terms of differences in development stages and environmental complexity. This study finds large differences in the development performance and change trends of digital agriculture, digital rural and digital finance, which is similar to the inter-country heterogeneity at the global scale, and is consistent with the analysis results of Zhu et al. (2023) and Meng et al. (2024), who found large spatial variations with positive spatial autocorrelation in digital agriculture in China. Different from the previous research, this study focuses on the spatial heterogeneity and autocorrelation of the symbiotic relationship between digital agriculture, digital rural and digital finance, which is a further expansion of them. In addition, scholars used to pay more attention to the analysis of the influence factors of digital agriculture, digital rural and digital finance, and lacked the research on their symbiotic mechanism. Goswami et al. (2023) and Kitole et al. (2024) argued the advantages of smallholder farmers, diversity of production systems, advantages of commodity crops, proximity to urban markets, public policies, credit support, extension services, and education as the main factors affecting the development of digital agriculture in India and Tanzania. Li et al. (2022), Cao et al. (2023) and Liu et al. (2024) found that financial investment, villagers’ income, investment in rural infrastructure, agricultural development, and population density have important effects on the level of digitalization. Nizam and Rashidi (2024) believes that dependency, illiteracy, lack of trust, cost, and stability are key obstacles to the development of digital finance. This study extends the analysis of influence mechanism to the field of symbiotic relationship generation and evolution, and emphasizes that the analysis of the symbiotic relationship between digital agriculture, digital rural and digital finance and its driving mechanism is the marginal contribution.
Based on the analysis of the development trend of digital agriculture, digital rural and digital finance, this paper quantitatively analyzes their symbiotic relationship and driving mechanism based on CCDM and spatio-temporal geographic weighted regression model, reaching the following conclusions. First, digital agriculture, digital rural and digital finance are in a rapid development stage, and the impact of the COVID-19 outbreak is regional. Second, coordination relationships and quasi symbiotic relationships dominate the symbiotic relationship between digital agriculture, digital rural, and digital finance. The evolution of the symbiotic index (coupling coordination index) is diverse, with a variety of patterns emerging such as continuously declining, fluctuating upward, relatively stable, L-shaped, inverted U-shaped, W-shaped, V-shaped, tilted Z-shaped, M-shaped, and the symbiotic relationship and its changes exhibit significant spatial variability, agglomeration, and autocorrelation. Third, the driving mechanism of symbiotic relationship is complicated. Most of the factors play a mixed role, with the effect nature and influence intensity varying over different regions. Notably, the fiscal self-sufficiency rate has played a positive role in the long run, growth rate of fixed assets investment, farmer entrepreneurship activity index and land transfer rate can all play a positive promoting role in a given environment.
The main inspirations of this study are listed below. First, the development and management policy design of digital agriculture, digital rural and digital finance should comply with the actual situation, trying to balance weak intervention and strong intervention, to ensure achievement of planning goals and strategic visions. Second, a shift should be encouraged in the management of digital agriculture, digital rural and digital finance from an inclusive development policy to a distinctive spatial policy, by delineating geographical zonings and establishing spatial development alliances. Third, the symbiotic development of digital agriculture, digital rural and digital finance is in the ascendant. It is necessary to apply benchmarking management methods and select leading regions to build regional and even national pilot projects to stimulate overall development. It is also necessary to increase external assistance and support to areas that are seriously lagging behind, and help them to achieve breakthroughs early in areas of strength with pairwise symbiosis (digital agriculture and digital rural, digital agriculture and digital finance, digital finance and digital rural). Fourth, the driving mechanism for the generation and evolution of the symbiotic relationship between digital agriculture, digital rural and digital finance is complex, and the influence of most factors is time-varying and regional. Therefore, it is necessary to design a combination of digitalization policies according to local conditions.
The marginal contribution of this study is the establishment of an interdisciplinary symbiotic research framework through the integration of information asymmetry theory, financial exclusion theory, spatial differentiation theory, spatial interaction theory, and symbiotic theory. The research framework integrating “symbiotic dynamics-symbiotic relationship-symbiotic mechanism” in digital agriculture, digital rural and digital finance, and further applies coupling coordination degree model and geographically and temporally weighted regression to the empirical research of China. The emerging research framework constructed in this study, as well as the introduction of spatial econometric model, provides a new perspective and new tool for scholars’ follow-up academic research. In addition, the analysis results of this study are of great reference value for countries similar to China, especially developing countries, and provide a basis for them to make symbiotic planning of digital agriculture, digital rural and digital finance. It should be noted that this study also has some shortcomings. For example, the late start, short time and immature development of digital agriculture, digital rural and digital finance in China, and the difficulty in accessing required data lead to a relatively short span of time in this study.
The original contributions presented in the study are included in the article/supplementary material, further inquiries can be directed to the corresponding author.
XY: Data curation, Formal analysis, Funding acquisition, Investigation, Methodology, Resources, Supervision, Validation, Writing – original draft. KL: Data curation, Investigation, Methodology, Software, Writing – original draft. WL: Conceptualization, Formal analysis, Funding acquisition, Project administration, Resources, Software, Supervision, Validation, Visualization, Writing – review & editing.
The author(s) declare that financial support was received for the research and/or publication of this article. This research was funded by “Research Project for Philosophy and Social Science Planning of Guangxi Zhuang Autonomous Region in 2024 (24GLF026)” and “Research Project Approved by Guangxi Vocational University of Agriculture in 2024 (XSK2409).”
The authors declare that the research was conducted in the absence of any commercial or financial relationships that could be construed as a potential conflict of interest.
The author(s) declare that no Gen AI was used in the creation of this manuscript.
All claims expressed in this article are solely those of the authors and do not necessarily represent those of their affiliated organizations, or those of the publisher, the editors and the reviewers. Any product that may be evaluated in this article, or claim that may be made by its manufacturer, is not guaranteed or endorsed by the publisher.
Adamowicz, M., and Zwolinska-Ligaj, M. (2020). The “Smart Village” as a way to achieve sustainable development in rural areas of Poland. Sustain. For. 12:6503. doi: 10.3390/su12166503
Ahiase, G., Nugraha, N., Andriana, D., and Sari, M. (2024). Digital financial inclusion and environmental sustainability in Africa: the moderating effect of income inequality. Management of Environmental Quality. Early Access 35, 1671–1690. doi: 10.1108/MEQ-11-2023-0382
Alabdali, S. A., Pileggi, S. F., and Cetindamar, D. (2023). Influential Factors, Enablers, and barriers to adopting smart Technology in Rural Regions: a literature review. Sustain. For. 15:7908. doi: 10.3390/su15107908
Alonso, S. L. N., Jorge-Vazquez, J., Fernández, M. A. E., Kolegowicz, K., and Szymla, W. (2022). Financial exclusion in rural and urban contexts in Poland: a threat to achieving SDG eight? Landscape 11:539. doi: 10.3390/land11040539
Balasundram, S. K. K., Shamshiri, R. R. R., Sridhara, S., and Rizan, N. (2023). The role of digital agriculture in mitigating climate change and ensuring food security: an overview. Sustain. For. 15:5325. doi: 10.3390/su15065325
Bao, B. F., Fei, B. X., Ren, G. G., and Jin, S. T. (2024). Study on the impact of digital finance on agricultural carbon emissions from a spatial perspective: an analysis based on provincial panel data. Int. J. Low-Carbon Technol. 19, 497–507. doi: 10.1093/ijlct/ctae019
Bolfe, E. L., Jorge, L. A. D., Sanches, I. D., Luchiari, A., da Costa, C. C., Victoria, D. D., et al. (2020). Precision and digital agriculture: adoption of technologies and perception of Brazilian farmers. Agriculture 10:653. doi: 10.3390/agriculture10120653
Cao, L., and Wang, G. S. (2024). Impact of digital finance on agricultural output: from the perspective of digital development of agriculture. Financ. Res. Lett. 66:105698. doi: 10.1016/j.frl.2024.105698
Cao, X. Z., Yan, M. Y., and Wen, J. (2023). Exploring the level and influencing factors of Digital Village development in China: insights and recommendations. Sustain. For. 15:10423. doi: 10.3390/su151310423
Chandio, A. A., Ozdemir, D., Gokmenoglu, K. K., Usman, M., and Jiang, Y. S. (2024). Digital agriculture for sustainable development in China: the promise of computerization. Technol. Soc. 76:102479. doi: 10.1016/j.techsoc.2024.102479
Cheng, C. M., Gao, Q., Ju, K. X., and Ma, Y. T. (2024). How digital skills affect Farmers’ agricultural entrepreneurship? An explanation from factor availability. J. Innov. Knowl. 9:100477. doi: 10.1016/j.jik.2024.100477
Cook, S., Jackson, E. L., Fisher, M. J., Baker, D., and Diepeveen, D. (2022). Embedding digital agriculture into sustainable Australian food systems: pathways and pitfalls to value creation. Int. J. Agric. Sustain. 20, 346–367. doi: 10.1080/14735903.2021.1937881
Degila, J., Sodedji, F. A. K., Avakoudjo, H. G. G., Tahi, S. P. G., Houetohossou, S. C. A., Honfoga, A. C., et al. (2023). Digital agriculture policies and strategies for innovations in the Agri-food systems - cases of five west African countries. Sustain. For. 15:9192. doi: 10.3390/su15129192
Deichmann, U., Goyal, A., and Mishra, D. (2016). Will digital technologies transform agriculture in developing countries? Agric. Econ. 47, 21–33. doi: 10.1111/agec.12300
Dibbern, T., Romani, L. A. S., and Massruhá, S. M. F. S. (2024). Main drivers and barriers to the adoption of digital agriculture technologies. Smart Agricul. Technol. 8:100459. doi: 10.1016/j.atech.2024.100459
Fotheringham, A. S., Crespo, R., and Yao, J. (2015). Geographical and temporal weighted regression (GTWR). Geogr. Anal. 47, 431–452. doi: 10.1111/gean.12071
Francini, M., Chieffallo, L., Palermo, A., and Viapiana, M. F. (2020). Estimation of the smart land index: application to the rural context of the Crati Valley. Eur. Plan. Stud. 28, 749–770. doi: 10.1080/09654313.2019.1648384
Gao, Q., Sun, M. Y., and Chen, L. (2024). The impact of digital inclusive finance on agricultural economic resilience. Financ. Res. Lett. 66:105679. doi: 10.1016/j.frl.2024.105679
Ge, H. P., Li, B. W., Tang, D. C., Xu, H., and Boamah, V. (2022). Research on digital inclusive finance promoting the integration of rural three-industry. Int. J. Environ. Res. Public Health 19:3363. doi: 10.3390/ijerph19063363
Geng, W. X., Liu, L. P., Zhao, J. Y., Kang, X. R., and Wang, W. L. (2024). Digital technologies adoption and economic benefits in agriculture: a mixed-methods approach. Sustain. For. 16:4431. doi: 10.3390/su16114431
Gong, W., Ma, R. Y., and Zhang, H. X. (2024). Digital agricultural technology services and Farmers’ willingness to choose digital production Technology in Sichuan Province. China. Front. Sustain. Food Syst. 8:1401316. doi: 10.3389/fsufs.2024.1401316
Goswami, R., Dutta, S., Misra, S., Dasgupta, S., Chakraborty, S., Mallick, K., et al. (2023). Whither digital agriculture in India? Crop Pasture Sci. 74, 586–596. doi: 10.1071/CP21624
Guo, F., Wang, J. Y., Wang, F., Kong, T., Zhang, X., and Cheng, Z. Y. (2020). Measuring China’s digital financial inclusion: index compilation and spatial characteristics. China Econ. Q. 19, 1401–1418. doi: 10.13821/j.cnki.ceq.2020.03.12
Guo, X., and Zhong, S. (2023). Unintended consequences: how does digital inclusive finance affect Migrants’ urban settlement intentions? Int. Rev. Financ. Anal. 89:102766. doi: 10.1016/j.irfa.2023.102766
Hackfort, S. (2021). Patterns of inequalities in digital agriculture: a systematic literature review. Sustain. For. 13:12345. doi: 10.3390/su132212345
Hansen, B. D., Leonard, E., Mitchell, M. C., Easton, J., Shariati, N., Mortlock, M. Y., et al. (2023). Current status of and future opportunities for digital agriculture in Australia. Crop Pasture Sci. 74, 524–537. doi: 10.1071/CP21594
Huang, Y., Zhao, J. Y., and Yin, S. (2023). Does digital inclusive finance promote the integration of rural industries? Based on the mediating role of financial availability and agricultural digitization. PLoS One 18:e0291296. doi: 10.1371/journal.pone.0291296
Jamil, S. A., Hamid, I., Alam, M. S., and Ahmad, S. (2024). Digitizing prosperity: how digital finance transforms agricultural incomes in China. Asia-Pacific financial markets. Early Access. doi: 10.1007/s10690-024-09465-8
Jiang, S., Zhou, J., and Qiu, S. (2022). Digital agriculture and urbanization: mechanism and empirical research. Technol. Forecast. Soc. Chang. 180:121724. doi: 10.1016/j.techfore.2022.121724
Kitole, F. A., Mkuna, E., and Sesabo, J. K. (2024). Digitalization and agricultural transformation in developing countries: empirical evidence from Tanzania agriculture sector. Smart Agricul. Technol. 7:100379. doi: 10.1016/j.atech.2023.100379
Klerkx, L., Jakku, E., and Labarthe, P. (2019). A review of social science on digital agriculture, smart farming and agriculture 4.0: new contributions and a future research agenda. NJAS-Wageningen J. Life Sci. 90-91:100315, 1–16. doi: 10.1016/j.njas.2019.100315
Konou, C. M. (2023). Entrepreneurial risk and digital financial inclusion: a cross-country analysis. Econ. Pap. 42, 267–281. doi: 10.1111/1759-3441.12399
Kulikov, I. M., Semin, A. N., Kislitskiy, M. M., and Strelka, E. A. (2019). Digital solutions of management issues and strategic planning for the development of agriculture and rural areas. Int. Transaction J. Eng. Manag. Appl. Sci. Technol. 10:10A18F. doi: 10.14456/ITJEMAST.2019.200
Lai, M., Li, W. W., Gao, Z. Y., and Xing, Z. P. (2024). Evaluation, mechanism and policy implications of the symbiotic relationship among rural digitization, agricultural development and farmer enrichment: evidence from Digital Village pilots in China. Front. Environ. Sci. 12:1361633. doi: 10.3389/fenvs.2024.1361633
Lajoie-O’Malley, A., Bronson, K., van der Burg, S., and Klerkx, L. (2020). The future(s) of digital agriculture and sustainable food systems: an analysis of high-level policy documents. Ecosyst. Serv. 45:101183. doi: 10.1016/j.ecoser.2020.101183
Latino, M. E., Menegoli, M., and Corallo, A. (2024). Agriculture digitalization: a global examination based on bibliometric analysis. IEEE Trans. Eng. Manag. 71, 1330–1345. doi: 10.1109/TEM.2022.3154841
Lee, C., Wang, F., and Lou, R. (2022). Digital financial inclusion and carbon neutrality: evidence from non-linear analysis. Res. Policy 79:102974. doi: 10.1016/j.resourpol.2022.102974
Leidner, D. E. (2018). Review and theory Symbiosis: an introspective retrospective. J. Assoc. Inf. Syst. 19, 552–567. doi: 10.17705/1jais.00501
Li, F. (2023). Measurement of digital agriculture level and spatial location distribution in the context of Chinese-style modernization. Feed Res. 46, 186–192. doi: 10.13557/j.cnki.issn1002-2813.2023.19.037
Li, X. J., Chandel, R. B. S., and Xia, X. L. (2022). Analysis on regional differences and spatial convergence of Digital Village development level: theory and evidence from China. Agriculture-Basel 12:164. doi: 10.3390/agriculture12020164
Li, X., Lepour, D., Heymann, F., and Marechal, F. (2023). Electrification and digitalization effects on sectoral energy demand and consumption: a prospective study towards 2050. Energy 279:127992. doi: 10.1016/j.energy.2023.127992
Li, X., and Zhang, Y. L. (2024). Digital economy, rural labor migration and common prosperity of farmers and rural areas. Statistics & Decision 40, 10–15. doi: 10.13546/j.cnki.tjyjc.2024.11.002
Li, W., Zhang, P., Zhao, K., Chen, H., and Zhao, S. (2023). The evolution model of and factors influencing digital villages: evidence from Guangxi. China. Agriculture-Basel 13:659. doi: 10.3390/agriculture13030659
Liang, Y., Lu, X. S., Zhang, D. G., Liang, F., and Ren, Z. B. (2003). Study on the framework system of digital agriculture. Chin. Geogr. Sci. 13, 15–19. doi: 10.1007/s11769-003-0078-4
Lin, Q. H., Cheng, Q. W., Zhong, J. F., and Lin, W. H. (2022). Can digital financial inclusion help reduce agricultural non-point source pollution? An empirical analysis from China. Front. Environ. Sci. 10:1074992. doi: 10.3389/fenvs.2022.1074992
Liu, Y., Deng, Y., and Peng, B. Y. (2023). The impact of digital financial inclusion on green and low-carbon agricultural development. Agriculture-Basel 13:1748. doi: 10.3390/agriculture13091748
Liu, H. X., Zhang, Y. Q., Wang, S. M., and Zhao, H. (2024). Comprehensive evaluation of Digital Village development in the context of rural revitalization: a case study from Jiangxi Province of China. PLoS One 19:e0303847. doi: 10.1371/journal.pone.0303847
Lu, S., Zhuang, J. C., Sun, Z. N., and Huang, M. (2024). How can rural digitalization improve agricultural green Total factor productivity: empirical evidence from counties in China? Heliyon 10:e35296. doi: 10.1016/j.heliyon.2024.e35296
Luo, G. Q., and Song, X. Y. (2024). The production mechanisms, spatial and temporal characteristics, and regional differences of agricultural new quality productivity in China. Chinese J. Agricul. Resources Regional Plan. 6, 1–14.
Ma, S. L., Li, J. F., and Wei, W. T. (2022). The carbon emission reduction effect of digital agriculture in China. Environmental science and pollution research. Early Access. doi: 10.1007/s11356-022-24404-8
Meng, J. J., Zhao, B. Y., Song, Y. X., and Lin, X. M. (2024). Research on the spatial dynamic evolution of digital agriculture-evidence from China. Sustain. For. 16:735. doi: 10.3390/su16020735
Mirzaei, M., Amanollahi, J., and Tzanis, C. G. (2019). Evaluation of linear, nonlinear, and hybrid models for predicting PM2.5 based on a GTWR model and MODIS AOD data. Air Qual. Atmos. Health 12, 1215–1224. doi: 10.1007/s11869-019-00739-z
Mumtaz, M. Z. (2024). Financial inclusion, digital finance and agricultural participation. Agricultural finance review. Early Access. 84, 93–113. doi: 10.1108/AFR-10-2023-0132
Naldi, L., Nilsson, P., Westlund, H., and Wixe, S. (2015). What Is Smart Rural development? J. Rural. Stud. 40, 90–101. doi: 10.1016/j.jrurstud.2015.06.006
Nitturkar, H. (2021). A practical tool to enhance the chances of success of digital agriculture interventions for sustainable development in Africa and India. J. Crop Improv. 35, 890–914. doi: 10.1080/15427528.2021.1879335
Nizam, K., and Rashidi, M. Z. (2024). Barriers to digital financial inclusion and digital financial services (DFS) in Pakistan: a phenomenological approach. Qualitative Res. Financial Markets 17, 251–274. doi: 10.1108/QRFM-11-2023-0271
Ogryzek, M., Krupowicz, W., and Sajnog, N. (2022). Public participation as a tool for solving socio-spatial conflicts of smart cities and smart villages in the sustainable transport system. Remote Sens. 13:4821. doi: 10.3390/rs13234821
Puntel, L. A., Bolfe, É. L., Melchiori, R. J. M., Ortega, R., Tiscornia, G., Roel, A., et al. (2023). How digital is agriculture in a subset of countries from South America? Adoption and limitations. Crop Pasture Sci. 74, 555–572. doi: 10.1071/CP21759
Rodino, S., Butu, M., Butu, A., Lazar, C., Ciornei, L., and Simion, P. S. (2023). Challenges of digital transformation in agriculture from Romania. Romanian Agricul. Res. 40, 713–721. doi: 10.59665/rar4066
Rolandi, S., Brunori, G., Bacco, M., and Scotti, I. (2021). The digitalization of agriculture and rural areas: towards a taxonomy of the impacts. Sustain. For. 13:5172. doi: 10.3390/su13095172
Romera, A. J., Sharifi, M., and Charters, S. (2024). Digitalization in agriculture: towards an integrative approach. Comput. Electron. Agric. 219:108817. doi: 10.1016/j.compag.2024.108817
Runck, B. C., Joglekar, A., Silverstein, K. A. T., Chan-Kang, C., Pardey, P. G., and Wilgenbusch, J. C. (2022). Digital agriculture platforms: driving data-enabled agricultural innovation in a world fraught with privacy and security concerns. Agron. J. 114, 2635–2643. doi: 10.1002/agj2.20873
Sadjadi, E. N., and Fernández, R. (2023). Challenges and opportunities of agriculture digitalization in Spain. Agronomy-Basel 13:259. doi: 10.3390/agronomy13010259
Shepherd, M., Turner, J. A., Small, B., and Wheeler, D. (2020). Priorities for science to overcome hurdles thwarting the full promise of the ‘Digital Agriculture’ revolution. J. Sci. Food Agric. 100, 5083–5092. doi: 10.1002/jsfa.9346
Tay, L. Y., Tai, H. T., and Tan, G. S. (2022). Digital financial inclusion: a gateway to sustainable development. Heliyon 8:e09766. doi: 10.1016/j.heliyon.2022.e09766
Wang, Y., Huang, Y. F., and Zhang, Y. H. (2023). Coupling and Coordinated Development of digital economy and rural revitalisation and analysis of influencing factors. Sustain. For. 15:3779. doi: 10.3390/su15043779
Wang, Y., Qi, Y., and Li, Y. (2024). How does digital inclusive finance influence non-agricultural employment among the rural labor force? Evidence from Micro-data in China. Heliyon 10:e33717. doi: 10.1016/j.heliyon.2024.e33717
Wang, H., and Tang, Y. T. (2023). Spatiotemporal distribution and influencing factors of coupling coordination between Digital Village and green and high-quality agricultural development-evidence from China. Sustain. For. 15:8079. doi: 10.3390/su15108079
Wojcik, M., Dmochowska-Dudek, K., and Tobiasz-Lis, P. (2021). Boosting the potential for GeoDesign: digitalisation of the system of spatial planning as a trigger for smart rural development. Energies 14:3895. doi: 10.3390/en14133895
Wu, Y. Z. (2022). The path of agricultural policy finance in smart Service for Rural Revitalization under big data technology. Mob. Inf. Syst. 1:9113683. doi: 10.1155/2022/9113683
Xiong, Z. B., Huang, Y. Z., and Yang, L. Y. (2024). Rural revitalization in China: measurement indicators, regional differences and dynamic evolution. Heliyon 10:E29880. doi: 10.1016/j.heliyon.2024.e29880
Xu, X. M., and Tian, X. H. (2022). Research on the relationship of coupling coordination between digitalization and agricultural sustainable development. Mob. Inf. Syst. 2022, 1–10. doi: 10.1155/2022/5643893
Zhang, F. W., Bao, X. L., Guo, S. L., Deng, X., Song, J. H., and Xu, D. D. (2022). Internet use and land transfer in: empirical evidence from China’s rural panel data. Environ. Sci. Pollut. Res. doi: 10.1007/s11356-022-21917-0
Zhang, G. J., Chen, Y., Wang, G. N., and Zhou, C. S. (2023). Spatial-temporal evolution and influencing factors of digital financial inclusion: county-level evidence from China. Chin. Geogr. Sci. 33, 221–232. doi: 10.1007/s11769-023-1333-5
Zhang, X. F., and Fan, D. C. (2024). Can agricultural digital transformation help farmers increase income? An empirical study based on thousands of farmers in Hubei Province. Environ. Dev. Sustain. 26, 1–27. doi: 10.1007/s10668-023-03200-5
Zhang, C. K., Li, Y., Yang, L. L., and Wang, Z. (2023). Does the development of digital inclusive finance promote the construction of digital villages? -an empirical study based on the Chinese experience. Agriculture-Basel 13:1616. doi: 10.3390/agriculture13081616
Zhang, P., Li, W., Zhao, K., Zhao, Y., Chen, H., and Zhao, S. (2023). The impact factors and management policy of Digital Village development: a case study of Gansu Province. China. Land 12:616. doi: 10.3390/land12030616
Zhang, X. J., and Zhang, Z. G. (2021). How Do Smart Villages Become a way to achieve sustainable development in rural areas? Smart Village planning and practices in China. Sustain. For. 12:10510. doi: 10.3390/su122410510
Zhao, L., Chen, H. N., Ding, X. H., and Chen, Y. F. (2024). Does Digital Village construction empower the green allocation of agricultural water resources? System 12:214. doi: 10.3390/systems12060214
Keywords: digital agriculture, digital rural, digital finance, coupling coordination degree, China
Citation: Yang X, Liu K and Li W (2025) Symbiotic relationship and attribution analysis of digitalization in agriculture, rural, and finance: evidence from China. Front. Sustain. Food Syst. 9:1545548. doi: 10.3389/fsufs.2025.1545548
Received: 15 December 2024; Accepted: 26 February 2025;
Published: 01 April 2025.
Edited by:
Rose Nyikal, University of Nairobi, KenyaReviewed by:
Yiorgos Gadanakis, Cyprus University of Technology, CyprusCopyright © 2025 Yang, Liu and Li. This is an open-access article distributed under the terms of the Creative Commons Attribution License (CC BY). The use, distribution or reproduction in other forums is permitted, provided the original author(s) and the copyright owner(s) are credited and that the original publication in this journal is cited, in accordance with accepted academic practice. No use, distribution or reproduction is permitted which does not comply with these terms.
*Correspondence: Weiwei Li, d3dsaTIwMjFAMTYzLmNvbQ==
Disclaimer: All claims expressed in this article are solely those of the authors and do not necessarily represent those of their affiliated organizations, or those of the publisher, the editors and the reviewers. Any product that may be evaluated in this article or claim that may be made by its manufacturer is not guaranteed or endorsed by the publisher.
Research integrity at Frontiers
Learn more about the work of our research integrity team to safeguard the quality of each article we publish.