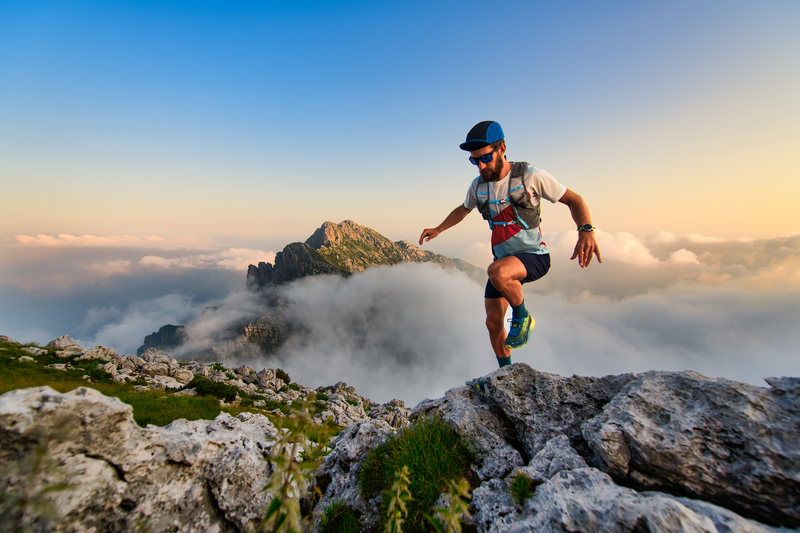
94% of researchers rate our articles as excellent or good
Learn more about the work of our research integrity team to safeguard the quality of each article we publish.
Find out more
ORIGINAL RESEARCH article
Front. Sustain. Food Syst.
Sec. Land, Livelihoods and Food Security
Volume 9 - 2025 | doi: 10.3389/fsufs.2025.1533423
This article is part of the Research Topic Technologies To Assess Soil Quality Towards Sustaining Food Security View all 7 articles
The final, formatted version of the article will be published soon.
You have multiple emails registered with Frontiers:
Please enter your email address:
If you already have an account, please login
You don't have a Frontiers account ? You can register here
Introduction: Most farmers in Nigeria lack knowledge of their farmland's nutrient content, often relying on intuition for crop cultivation. Even when aware, they struggle to interpret soil information, leading to improper fertilizer application, which can degrade soil and ground water quality. Traditional soil nutrient analysis requires field sample collection and laboratory analysis; a tedious and time-consuming process. Digital Soil Mapping (DSM) leverages Machine Learning (ML) to create detailed soil maps, helping mitigate nutrient depletion. Despite its growing use, existing DSM-based ML methods face challenges in prediction accuracy and data representation. Aim: This study presents GeaGrow, an innovative mobile app that enhances agricultural productivity by predicting soil properties and providing tailored fertilizer recommendations for yam, maize, cassava, upland rice, and lowland rice in southwest Nigeria using Artificial Neural Networks (ANN).The presented method involved the collection of soil samples from six states in southwest Nigeria which were analysed in the laboratory to compile the primary dataset mapped to the coordinates. A secondary dataset was compiled using iSDAsoil's API for data augmentation and validation. The two sets of data were preprocessed and normalized using Python, and an ANN was employed to predict soil properties such as NPK, Organic Carbon, Soil Textural Composition and pH levels through regressive analysis while building a composite model for Soil Texture Classification based on the predicted soil composition. The model's performance yielded a Mean Absolute Error (MAE) of 1.9750 for NPK and Organic Carbon prediction, 3.5461 for Soil Textural Composition prediction, and 0.1029 for pH prediction. For the classification of the soil texture, the results showed a high accuracy value of 99.9585%.The results highlight the effectiveness of combining soil texture with water retention, NPK, and Organic Carbon to predict pH and optimize fertilizer application. The GeaGrow app provides farmers with accessible, locationbased soil insights and personalized crop recommendations, marking a significant advancement in agricultural technology. The GeaGrow app also provides smallholder farmers with scalable, ease of adoption and use of the developed mobile application.This research demonstrates the potential of ML to transform soil nutrient management and improve crop yields, contributing to sustainable farming practices in Nigeria.
Keywords: Precision farming, machine learning, soil data analytics, Fertilizer optimization, GeaGrow mobile application
Received: 23 Nov 2024; Accepted: 24 Feb 2025.
Copyright: © 2025 Folorunso, Ojo, Busari, Adebayo, Adejumobi, Folorunso, Ayo, Alabi and OLABANJO. This is an open-access article distributed under the terms of the Creative Commons Attribution License (CC BY). The use, distribution or reproduction in other forums is permitted, provided the original author(s) or licensor are credited and that the original publication in this journal is cited, in accordance with accepted academic practice. No use, distribution or reproduction is permitted which does not comply with these terms.
* Correspondence:
Olusegun Folorunso, Federal University of Agriculture, Abeokuta, Abeokuta, 110282, Ogun, Nigeria
Disclaimer: All claims expressed in this article are solely those of the authors and do not necessarily represent those of their affiliated organizations, or those of the publisher, the editors and the reviewers. Any product that may be evaluated in this article or claim that may be made by its manufacturer is not guaranteed or endorsed by the publisher.
Research integrity at Frontiers
Learn more about the work of our research integrity team to safeguard the quality of each article we publish.