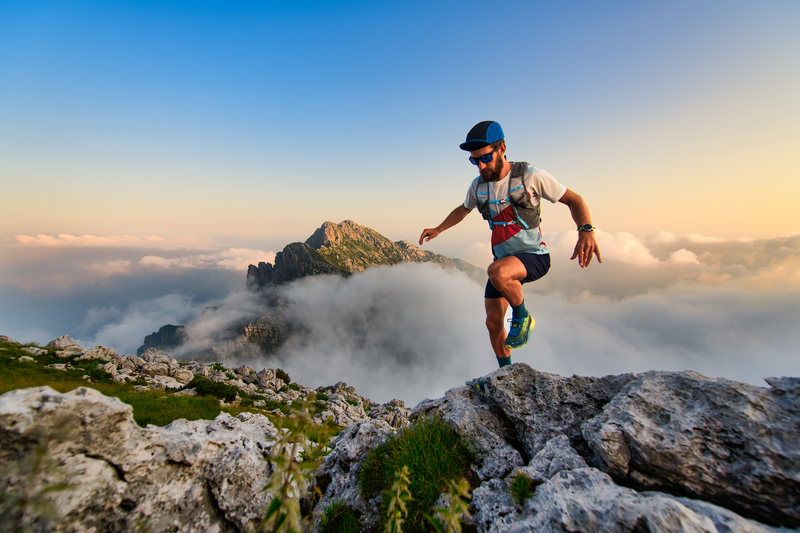
94% of researchers rate our articles as excellent or good
Learn more about the work of our research integrity team to safeguard the quality of each article we publish.
Find out more
ORIGINAL RESEARCH article
Front. Sustain. Food Syst. , 22 January 2025
Sec. Agricultural and Food Economics
Volume 9 - 2025 | https://doi.org/10.3389/fsufs.2025.1501600
Most farmers in China show an strange attitude towards food sale channels:they prefer low priced channel rather than high priced channel. This paper examines the mechanism by which food price affect farmers’ choices of sale channels and the role played by risk aversion, based on the 2022 China Land Economic Survey. The results indicate that higher prices are more likely to lead farmers to choose dealers who are private buyers offering flexible terms, compared with depots which are government procurement centers ensuring stable prices and other channels. It reveals that the underlying reason for farmers’ choices is the certainty effect, which causes farmers to prefer dealers offering certain profits over depots with uncertain profits, despite the high prices. It is further found that risk aversion has a mediating effect on the relationship between food price and farmers’ choices of sale channels.
Agriculture is a sensitive sector subject to various risks with a low comparative advantage, and almost every government in the world supports and protects its agriculture to varying degrees. Compared to developed countries such as the United States and the European Union, China still subsidizes food price in circulation despite the constraints of the amber box policy (Springmann and Freund, 2022; Sharma and Shajahan, 2024). At harvest, China’s state-owned food depots are open for buying from farmers at higher prices than those offered by dealers and factories, which provides opportunities for farmers to obtain higher income.
Even so, it seems that farmers are either unable or unwilling to take advantage of such opportunities. Food price are crucial for farmers to realize their production value, especially in recent years while yields are stagnant despite high agricultural costs. However, most farmers show an indifferent attitude towards food sale channels; they ignore the price difference and sell directly to the dealers.
Explanations for the puzzling choice focus on storage, quality, and transaction cost (Aggarwal et al., 2018; Burke et al., 2019; Brunt and Cannon, 2022). Selling to food depots requires facilities for dewatering, high yields with low impurity rates, and ease of trading. Other influencing factors include planting size (Zulu et al., 2007), liquidity (Stephens and Barrett, 2011), and technology (Channa et al., 2019).”We address a new insight that farmers’ indifference to sale channels is based on the preference for certain price. Although the purchase price of depots seems higher, farmers have to calculate the net benefit after removing costs such as dewatering, substandard quality, transportation, etc. Compared with depots where the real price is unknown, farmers tend to choose dealers for certain profits, besides the profits of selling to depots are insufficient due to the fragmented farmland with small scale in China. Moreover, in a normal year, the higher the food price, the greater the risk. For farmers who are mostly risk averse, the risk of flat or declining prices after harvest may induce them to quick sale to lock in profits.
There has been extensive and in-depth research contributing to the rich literature regarding the impact of price volatility on producers in low-income countries (Stiglitz, 1969; Sandmo, 1971; Deaton and Laroque, 1992; FAO et al., 2011; Bellemare et al., 2013; Gilbert et al., 2017). For example, Barrett (1996) show that price uncertainty reduces the incentive to store among poor farmers in Madagascar. Tripathi (2024) describes how the Indian government’s net purchases prevent low market prices for wheat but can result in price spikes. Yet, studies on the impact of price volatility on farmers’ choice of food sale channels have not been found. Our point is that farmers’ preference for certain prices is a certainty effect, which was proposed in prospect theory (Kahneman and Tversky, 1979). The certainty effect refers to the fact that decision-makers tend to give more weight to certain outcomes, while assigning lower weights to probable outcomes. In the context of food sales, farmers prefer dealers with actual certain prices, rather than food depots with uncertain profits despite higher prices. It helps to explain the perplexing problem in reality: why farmers sell their food to low-priced dealers rather than high-priced depots.
The possible modest contributions of this research are summarized as follows. First, it reveals that the crucial reason why farmers choose to sell directly to dealers lies in their preference for certain profits, and our results break the long-standing assumption of price primacy in relevant research. Second, we quantify the magnitude of food price on farmers’ food sale channels and demonstrate how food price can affect sale channels choice. Third, we find that psychological preference has a mediating effect on farmers’ sale channels choice. This finding provides important policy implications to reduce risk for farmers. Moreover, the mediating effect of risk aversion is common and practical in China and other developing countries regarding farmers’ food sales.
The remainder of the article is organized as follows: the second part constructs the theoretical model and the mechanism analysis; the third part presents the data sources and variable selection; the fourth part reports and analyzes the estimation results; the fifth part further discusses the implications based on farmers’ characteristics; and the last part concludes the full article.
We consider a simple model in which a farmer decides whether to sell food to a depot or a dealer. Farmers who sell food encounter both income and price risk, and the decision relies on price and risk preference, as shown in Barrett (1996). We draw on Cardell and Michelson's (2022) analysis to develop a theoretical model of the relationship between price and farmers’ choice for sale channels.
First, we assume that the farmer is rational, risk-averse, and a price taker in both input and output markets, and operates in a competitive market with identical storage and credit conditions.
Considering that any one farmer, all the outputs can be sold to dealer and depot. is the price of dealer, and is the price of depot. is the quantity of food sold. is transaction cost of depot while the transaction cost of dealer is approximately zero. We calculate the net benefit :
We need to calculate the net benefit per unit because there will be amount deduction for the imperfect quality of food sold to the depot. Here we assume the quantity is the same, and later we relax this assumption. The net benefit per unit can be approximated as:
The transaction cost is mainly concerned with transport, labor and time costs, etc., and is basically exogenous from . In Equation 2, an increase in implies an increase in , which means that the net benefit per unit sold to depot increase with sales quantity. This leads to the research hypothesis H1:
H1: The larger planting size, the more likely it is that the farmer will choose sale to depot compared to dealer.
However, in the actual sale of food, the quantity usually will be a deduction while sold to depot due to the imperfect quality. We set is the quantity of harvest, is the quantity after deduction by depots. The Equation 1 is rewritten as:
By taking a partial derivative of Equation 3, we can find that the elasticity of net benefit to price is much greater than that of quantity. Even if the transaction cost is calculated, the value of is more likely to be greater than zero, given the profit margin of the dealer. Why do most farmers still sell to the dealers? How do farmers make these decisions? What strategies do they use to mitigate their exposure to output price risk? We draw on Cardell and Michelson's (2022) analysis to continue the discussion.
Based on the cost–benefit analysis in the previous section, the farmer still chooses to sell to a dealer, even though the revenue from selling to a depot is likely to be greater. At harvest time, the price offered by the dealer is certain, but the transaction price of the depot is uncertain. If the net profit is always positive, then the food should be sold to the depot, but the randomness of actual transaction price exposes farmers to price risk. We assume that farmers have a Von Neumann-Morgenstern (VNM) utility function for calculating the certain equivalent rate of return for different sale channels. The risk premium equation is as follows:
Where represents the farmer’s wealth, represents risk–return ratio of sale to depot, and is the certainty equivalent rate of return that the farmer is willing to forgo sale to depot. Assuming that the utility function is second-order continuously derivable, a second-order Taylor expansion of the left side of the equal sign of Equation 4 at the mean yields:
In Equation 5, = = . Taking the expectation of Equation 5 yields:
In Equation 6, is the risk–return variance of farmers’ choice for sale to depot, representing the price uncertainty. The higher food price, the more farmers tend to sell quickly to dealers to get rid of risk and lock in profits. Since , Equation 6 reduces to:
Also, a first-order Taylor expansion of the right side of the equality sign of Equation 7 at yields:
Joining the two Equations 7 and 8, we obtain:
In Equations 9, we set the absolute risk aversion coefficient , denoting that a farmer is willing to give up in order to avoid the risk of losing 1 unit quantity of wealth; relative risk aversion coefficient , denoting that a farmer is willing to give up in order to avoid the risk of a 1 percentage loss of wealth. Logically, varies greatly with the amount of individual wealth and does not completely portray the risk preference of farmers. The degree of aversion to the risk of proportional loss of wealth, , is more reflective of the inherent attitude of farmers to risk. Therefore, we use to refer to the risk preference of farmers. The higher the value of implies that the more averse to risk. The certainty equivalent return can be expressed as:
There are two scenarios here: (i) and (ii) . We concern about (i) because if , the price will be close to dealer’s price and the farmer is willing to forgo sale to depot. From the theoretical mechanism, an increase in and in Equation 10 implies a decrease in , that is, the value of sale to depot decreases with the increase of farmer’s risk aversion and food price, thus reducing the likelihood that the farmer will choose sale to depot. This leads to the following research hypotheses:
H2: The higher food price, the less likely it is that the farmer will choose sale to depot or factory compared to dealer.
In the framework of expected utility theory and its variants, risk preference is only a descriptive label that technically refers to the curvature of the utility function. Risk aversion is explained at the core of psychology as “risk taking demands a premium return” (Winterfeldt and Edwards, 1986), while the choice of sale channels by farmers is based on the pursuit of premium returns. When farmers are mostly risk averse and it is considered to be a consistent and invariant psychological trait (Stigler and Becker, 1977), then , can be approximated as a positive constant, the certainty-equivalent rate of return actually determined by food price .
Although there is little consensus as to whether covariate shocks induce individuals to become more or less risk averse, empirical studies find evidence that price uncertainty would increase individuals’ risk aversion (Sandmo, 1971; Barrett, 1996; Peng and Xu, 2022; Liebenehm et al., 2024). As a consequence, risk averse farmers are less likely to choose depots since they involve uncertain returns. That is, farmers’ choice of sales channels is not only directly affected by food price, but also it’s mediated by food price on risk aversion.
The derivation of with respect to in Equation 10 gives:
From Equation 11, we can see that the marginal effect of price on farmers’ sales channels is related to risk aversion: As food prices rise , the likelihood that a farmer will choose depot for sale decreases with increasing risk aversion . From this we derive the following hypothesis:
H3:The higher food price, the more risk averse farmers, and the less likely they will choose sale to depots or factories compared to dealers.
Figure 1 shows the mechanism of food price, risk aversion on farmer’s sales channels.
This research uses data from the 2022 China Land Economic Survey (CLES) of Nanjing Agricultural University, China, and rainfall data from the Jiangsu Statistical Yearbook 2022. The China Land Economic Survey was founded by the Division of Humanities and Social Sciences of Nanjing Agricultural University in 2020, with the assistance of the Jinshanbao Institute of Agricultural Modernisation (JIAM) in the implementation of the survey. The construction of the CLES database was based on the concept of retracing the path of John Lossing Buck, with the sampling area covering the regions where Professor Lossing Buck conducted his research. The research area starts from Jiangsu and gradually expands to the Yangtze River Delta region and the whole country. The research data will be compared with Buck’s research data to illustrate the changes in China’s rural landscape over the past century. We exclude data on food sale prices and channels that are missing or outliers, resulting in 475 observations in the sample.
To examine the effect of food price on farmer’s choice of sale channels, let denote the sale channel chosen by farmer, and the explanatory variable varies only with farmer and not with group . It is a multivariate unordered choice problem requiring a control group, we use multinomial logit model for empirical estimation. The general form of the model can be expressed as follows in Equation 12:
The dependent variable in this paper is the choice of sale channels by farmer. The choice of dealer1 is assigned a value of 1, the choice other than dealer and depot2is assigned a value of 2, and the choice of depot is assigned a value of 3. The distribution of choices of the sample farmers is reported in Table 1.
Table 1 shows that 82.53% of the sample farmers chose sale to dealer, while only 6.74% of the farmers chose depot, indicating that most of the farmers are not concerned about chances of higher prices but certain returns.
The core independent variable in this paper is food price. Food price have the most significant and direct impact on farmer’s production returns. The histogram of food price is shown in Figure 2. It can be seen that food price are approximately normally distributed.
To capture price level variability more effectively, we include a table presenting the mean, standard deviation, and results of the Jarque-Bera normality test for price levels across dealers, depots, and other marketing channels in Table 2. An ANOVA test was also performed to determine whether price levels significantly differ among these three groups.
The mediating variable in this paper is the risk aversion of farmers. In the questionnaires of CLES, farmers’ risk aversion level is measured by the following question: “If you have a sum of money to invest, what kind of investment program are you most willing to choose?” If farmers choose option 1 “high risk and high return,” it means that farmers’ risk aversion level is low. Similarly, if farmers choose option 2 “medium risk and medium return” or option 3 “low risk and low return,” it means that farmers’ risk aversion level is medium and high, respectively. Table 3 reports the distribution of sample farmers’ risk aversion.
The results of the survey shows that only 6.11% of the 475 farmers are low level risk aversion, while 76.42% are high level risk aversion, indicating that most of the farmers are highly risk averse.
We select other factors affecting the decision of farmers’ sales channels as control variables, including the characteristics of the head of the household (health, whether or not a village official), the characteristics of the family (household size, labor, deposit) and the characteristics of production (planting size, farm machinery, disease and pest training).
Table 4 shows the definition of each variable and the results of descriptive statistics. The average physical condition of the sample households is good, and 18.9% of the sample households have had the experience of village officials. The average household size of the sample households is about 3 persons, which is more than the average household laborers. The household deposit is around 6.27 thousand USD dollars on average. The average planting size is 2.467 hectares. Each household has 0.59 tractors and receives 0.86 trainings on average.
Table 5 reports the estimated results from the multinomial logit model, which demonstrates the impact of food price on farmers’ choice of sale channels. Compared with dealers, food price has a negative effect on depots and other channels at 1 and 5% significance level, respectively. The term relative risk ratio (RRR) refers to the odds ratio (OR). After adding control variables, for each unit increase in food price compared with dealers, the odds of farmers choosing others and depots decreases to 0.002 and 0.007 times the original ratio, respectively. Our findings indicate that the higher food price, the more likely farmers are to choose dealers over depots and other channels. The hypothesis H2 is verified.
Among the control variables, the household labor has a significant and positive coefficient on other channels compared to the dealer, which may be due to the fact that laborers could help with food production and realize the transaction to other channels such as factories more easily, while household labor is not helpful in selling to the depot. Also, the planting size has a positive effect on farmers’ choosing the depot compared to the dealer at the 1% level of significance, which means that the larger the planting size, the more likely it is that the farmer will choose sale to the depot compared to the dealer. The hypothesis H1 is verified.
Results from the average marginal effect in Table 6 show that food price have a significant impact on farmers’ sale channel choice. For each unit increase in food price, the probability of farmers choosing dealers increases by 69.2%, while the probability of choosing other channels decreases by 47.9%, and the probability of choosing depots decreases by 21.4%, respectively, validating the results of the baseline regression.
From the average marginal effect of control variables, household labor has a significant impact on other channels, while no significant impacts on dealers or depots. The reason may lie in the fact that the availability of sufficient labor gives farmers the possibility to choose a wider range of marketing channels. For planting size, planting size has a negative impact on choosing dealers, and positive impact on choosing depots. It’s possibly because that large-scale farmers are choosing depots with high unit prices. Also, farm machinery has a negative effect on choosing dealers, similarly because highly mechanized farmers are more reluctant to deal with dealers.
The model estimation has endogenous problems between food price and sale channels mainly because of reverse causality. Different sale channels may affect the transaction price. The reality is that food transaction prices in depots tend to be higher than those of dealers. To solve the endogenous problems, we estimate the inversion model, referring to Berry (1994). The altitude of the village is employed to serve as the Instrumental Variable (IV). The IV is expected to be effective in food price but ineffective in sale channels’ choices (Kolko, 2012). When using the inversion model, the corresponding estimation method can be expressed with the following in Equation 13:
Where indicates the difference of sale channels probabilities. Since multivariate logit model can be viewed as a simultaneous estimation of multiple binary logit models that are composed of selection behaviour, we denote in terms of the average marginal effect of food price.
We employ the IV-2SLS model to mitigate endogenous problems between food price and farmers’ sale channels’ choices. The results of Table 7 indicate that the weak IV test rejects the presence of a weak IV. Both the first-stage and the IV regressions show that the coefficients are significant, implying a negative effect of food price on depots and other channels compared with dealers.
In order to test the reliability of the results, this paper replaces the core independent variable with the reciprocal of rainfall during the harvest season and uses the same control variables to estimate the impact of rainfall on farmers’ channel choice. Logically, the less rainfall there is at harvest, the higher the food price. The results in Table 8 show that the reciprocal of rainfall negatively affects farmers’ choice of depot and other channels at the 1% level of significance. In summary, the article’s treatment of the independent variable does not seriously interfere with the robustness of the findings.
In order to test the robustness of the model, we conduct a robustness test of the baseline regression by changing the model form. Since we want to observe the difference between dealers and other sale channel choices, the choice of dealer is assigned a value of 0, and other choice is assigned a value of 1. The binary logit model is used for replacement. Table 9 shows that food price negatively affect farmers’ food sales choices at the 1% level of significance. That is, the higher the food price, the more farmers tend to give up other channel sales and depot sales.
The phenomenon that farmers sell their harvest grain through multiple simultaneously does exist. And the multinomial logit model’s suitability would come into question. We also take a multivariate probit model, which empirically measures the correlation coefficients among the three marketing channels, for the robustness test. The results in Table 9 show that food price have significant negative impacts on Others and Depot channels, which validated the empirical analysis.
There may be significant heterogeneity in the effect of food price on farmers’ food sale channels across different planting scales and levels of non-farm income. We attempt to reclassify the sample based on the characteristics of farmers’ planting scale and non-farm income, allowing us to analyze the heterogeneity in the effect on farmers’ food sales channels.
Planting scale is a crucial sales factor for farmers. There can be significant heterogeneity in the impact of food price on farmers’ sale channels at different planting sizes. We divide the sample farmers into two groups based on the mean of planting size. Table 10 shows the effects of food price on farmers’ sale channels with different planting scales.
The regression results show that smallholder farmers are significantly affected by food price, and food price are no longer significant in the channel choice of large-scale farmers. The pursuit of revenue and the management of risk differ in the two groups. For small-scale farmers, the revenue of food sales is low, and they are often risk-averse, so they tend to sell to dealers. Instead, farmers with large-scale planting focus on sales revenue and are not likely to give up on profitability easily. The regression results indicate that heterogeneity in planting size significantly differentiates farmers’ choices of sale channels, thus reinforcing the practical basis of the relevant discussion in this paper.
As non-farm income becomes increasingly important to households, the level of non-farm income will affect farmers’ choice of food sale channels. We categorize the sample into two groups: a low-level non-farm income and a high-level non-farm income, based on the median, and run separate economic regressions. Table 11 reports the effect of food price on farmers’ sale channels’ choices with different levels of non-farm income.
Compared to the total sample, the regression results show that farmers with high-level non-farm income would not sell to depots, and farmers with low-level non-farm income would not sell to other channels. In the case of farmers with high-level non-farm income, food sales are not as important for total household income. As a result, they often choose to sell directly to dealers. On the contrary, based on the importance of revenue from food sales, farmers with low-level non-farm income will pay more attention to the choice of food sale channels. To summarize, unlike the attitude of farmers who value food sale channels with low-level non-farm income, a high-level non-farm income is an important factor that induces farmers to sell to dealers.
We also explore the mechanisms for the linkage between farmers’ risk aversion and sale channel choices. Based on our findings in baseline regression, the increase in food price has reduced the probability of farmers choosing other channels and depots compared with dealers. Table 12 represents the estimated mediating effect of risk aversion on farmers’ sale channel choice. Relative to dealers, food price has a positive effect on risk aversion for both other channels and depots at the 1% level of significance, which indicates that the higher the price, the more risk-averse farmers are, and the less likely they are to choose sale to depots or other channels compared to dealers. The hypothesis H3 is verified.
In addition to the significant impact of food price on farmers’ sales channels, the influence of control variables cannot be ignored (Qiu et al., 2020). From the baseline regression results in Table 4, we can see that household labor has a significant impact on other sale channels compared with dealers. This is in line with the reality of farmers’ food sales situation. While it is difficult to sell food into depots and the dealers’ purchase price is low, those with surplus labor households sell their food to other channels such as factories, cooperatives, etc. Likewise, planting size positively influences farmers’ choice to sell to depots compared with dealers. As the planting scale increases, the elasticity of returns to prices gradually increases, and farmers strive to sell to depots for the sake of more profit. This is consistent with the findings of Xu et al. (2018).
Meanwhile, the heterogeneity of key factors in farmers’ food sale channels should be discerned. Based on the fact of characterization, we focus on the heterogeneity in planting size and non-farm income, which significantly differentiate farmers’ choices of sale channels. For planting size heterogeneity, it is proved by results presented in Table 10. In addition to considerations of uncertain risk and low revenue from food sales, small-scale farmers tend to sell to dealers other than depots. The effect is significant as can be seen in the right part of Table 10. Conversely, the impact of food price on large-scale farmers’ sale channels is not significant. Due to their large size, large-scale farmers have to take more factors into consideration: water removal, funds liquidity, storage space, etc. Farmers often cannot afford to forgo any opportunity for profitability due to the critical role of food sales in their livelihoods. For non-farm income heterogeneity, non-farm income of Chinese farmers has gradually increased. The ones with high non-farm income will not pay much attention to food sales as before, which is confirmed in the left part of Table 11. Compared with sale to dealers, farmers with high non-farm income tend to forgo the chance to sell to depots. Meanwhile, the attitude of those with low non-farm income has changed. They would not like to sell to other channels, but the option to drop sale to depots becomes not significant, which is statistically different from the total sample. It reveals that farmers with low non-farm income pay more attention to sale channels choice.
We also check the mediating mechanism of risk aversion between food price and farmers’ food sale channels. Table 13 shows that farmers’ level of risk aversion elevates with food price increasing. The mediating mechanism gives an explanation of farmers’ choice of food sale channels from the perspective of risk. In addition, we assume that farmers are risk-averse, and this assumption is supported by a number of scholars (Cotty et al., 2019; Cardell and Michelson, 2022; Tian et al., 2024). However, this viewpoint is facing challenges and is criticized as too generalized. Still, many Chinese farmers have just been lifted out of poverty, they do not have the ability or strength to take risks and are indeed risk-averse.
In addition, we develop a “Theory of Change” model to illustrate how small farmers, particularly those without non-farm income, can increase their earnings and mitigate risks. Due to farmers’ risk perception, small farmers tend to sell their food to dealers. This reduces risk but does not contribute to increased farm incomes. In order to change this situation, government involvement is needed to reduce the external risk to farmers, so that they are willing to choose more profitable ways of selling their grain. Farmers’ risk attitude changes when the government policy mitigates the external risk, and the risk seeking attitude drives farmers to seek higher returns and turns to sales towards depots (Figure 3).
The assumption of rationality for farmers has led many researchers to ignore the price risk of farmers when making sales decisions. We address a new insight that farmers’ indifference to sale channels is based on the preference for certain profits. For risk-averse farmers, they are more concerned with fixed profits, while they do not seek high prices.
We demonstrate that high food price induces farmers to choose dealers for sales compared with depots and other channels. We also find that risk aversion would mediate the effect of food price on sale channels choice and plausibly contribute to the farmers’ decision to choose dealers. In fact, the inclusion of constraints on farmers not assumed in the actual food sales would only strengthen our results.
In particular, our findings also suggest the potential importance of experimenting with and evaluating policies that address farmers’ risk perceptions on sales to depots. Farmers who are exposed to price risk and unprotected, dysfunctional market and government institutions are more risk-averse towards uncertain returns. In turn, chances of higher profits are forgone, and the likelihood of remaining poor is increasing. Improvements in agricultural services, credit support, and price mechanisms to break the constraints are needed (Reddy, 2021). Relevant policies that might increase farmers’ income would help to ensure food security in a macro sense.
The original contributions presented in the study are included in the article/Supplementary material, further inquiries can be directed to the corresponding author.
TT: Conceptualization, Data curation, Formal analysis, Funding acquisition, Investigation, Methodology, Project administration, Resources, Software, Validation, Visualization, Writing – original draft, Writing – review & editing. YS: Writing – review & editing, Resources. PC: Validation, Writing – review & editing.
The author(s) declare that financial support was received for the research, authorship, and/or publication of this article. This study was supported by National Social Science Major Fund Project (22ZDA117).
The authors declare that the research was conducted in the absence of any commercial or financial relationships that could be construed as a potential conflict of interest.
The author(s) declare that no Gen AI was used in the creation of this manuscript.
All claims expressed in this article are solely those of the authors and do not necessarily represent those of their affiliated organizations, or those of the publisher, the editors and the reviewers. Any product that may be evaluated in this article, or claim that may be made by its manufacturer, is not guaranteed or endorsed by the publisher.
The Supplementary material for this article can be found online at: https://www.frontiersin.org/articles/10.3389/fsufs.2025.1501600/full#supplementary-material
1. ^Although cooperatives and large farmers also buy food, the vast majority of them end up sales still to depots, so we classify them as dealers.
2. ^Other channels include factories and consumers, etc.
Aggarwal, S., Francis, E., and Robinson, J. (2018). Food today, gain tomorrow: evidence from a storage experiment with savings clubs in Kenya. J. Dev. Econ. 134, 1–15. doi: 10.1016/j.jdeveco.2018.04.001
Barrett, C. B. (1996). On price risk and the inverse farm size-productivity relationship. J. Dev. Econ. 51, 193–215. doi: 10.1016/S0304-3878(96)00412-9
Bellemare, M. F., Barrett, C. B., and Just, D. R. (2013). The welfare impacts of commodity price volatility: evidence from rural Ethiopia. Am. J. Agric. Econ. 95, 877–899. doi: 10.1093/ajae/aat018
Berry, S. T. (1994). Estimating discrete-choice models of product differentiation. RAND J. Econ. 25, 242–262. doi: 10.2307/2555829
Brunt, L., and Cannon, E. (2022). English farmers' wheat storage and sales in the late eighteenth and early nineteenth centuries. Econ. Hist. Rev. 75, 932–959. doi: 10.1111/ehr.13133
Burke, M., Bergquist, L. F., and Miguel, E. (2019). Sell low and buy high: arbitrage and local Price effects in Kenyan markets. Q. J. Econ. 134, 785–842. doi: 10.1093/qje/qjy034
Cardell, L., and Michelson, H. (2022). Price risk and small farmer maize storage in sub-Saharan Africa: new insights into a long-standing puzzle. Am. J. Agric. Econ. 105, 1–23. doi: 10.1111/ajae.12343
Channa, H., Chen, A. Z., Pina, P., Gilbert, J., and Stein, D. (2019). What drives smallholder farmers’ willingness to pay for a new farm technology? Evidence from an experimental auction in Kenya. Food Policy 85, 64–71. doi: 10.1016/j.foodpol.2019.03.005
Cotty, T. L., Maître D’Hôtel, T. E., Soubeyran, R., and Subervie, J. (2019). Inventory credit as a commitment device to save food until the hunger season. Am. J. Agric. Econ. 101, 1115–1139. doi: 10.1093/ajae/aaz009
Deaton, A., and Laroque, G. (1992). On the behaviour of commodity prices. Rev. Econ. Stud. 59, 1–23. doi: 10.2307/2297923
FAO, IFAD, IMF, OECD, UNCTAD, WFP, the World Bank, the WTO, IFPRI and the UN HLTF. (2011): Price volatility in food and agricultural markets: policy responses. Policy Report for the G-20.
Gilbert, C. L., Christiaensen, L., and Kaminski, J. (2017). Food price seasonality in Africa: measurement and extent. Food Policy 67, 119–132. doi: 10.1016/j.foodpol.2016.09.016
Kahneman, D., and Tversky, A. (1979). Prospect theory: an analysis of decision under risk. Econometrica 47, 263–291. doi: 10.2307/1914185
Kolko, J. (2012). Broadband and local growth. J. Urban Econ. 71, 100–113. doi: 10.1016/j.jue.2011.07.004
Liebenehm, S., Schumacher, I., and Strobl, E. (2024). Rainfall shocks and risk aversion: evidence from Southeast Asia. Am. J. Agric. Econ. 106, 145–176. doi: 10.1111/ajae.12403
Peng, P., and Xu, Z. G. (2022). Price expectations, risk aversion, and choice of sales methods for large-scale farmers under incomplete market conditions. Agribusiness 38, 1012–1031. doi: 10.1002/agr.21757
Qiu, H., Su, L., Zhang, Y., and Tang, J. (2020). Risk preference, risk perception and Farmers’Adoption of conservation tillage. Chin. Rural Econ. 7, 59–79.
Reddy, A. A. (2021). Assuring farmers income in the context of new farm Laws: issues and the way forward. In 2021 conference, august 17-31, 2021, virtual (no. 315243). International Association of Agricultural Economists.
Sandmo, A. (1971). On the theory of the competitive labor-managed firm under Price uncertainty. Am. Econ. Rev. 61, 65–73.
Sharma, S. K., and Shajahan, A. A. (2024). WTO and a permanent solution for food security: striving for a hunger-free world. Food Secur. 16, 321–337. doi: 10.1007/s12571-023-01426-9
Springmann, M., and Freund, F. (2022). Options for reforming agricultural subsidies from health, climate, and economic perspectives. Nat. Commun. 13:82. doi: 10.1038/s41467-021-27645-2
Stephens, E. C., and Barrett, C. B. (2011). Incomplete credit markets and commodity marketing behavior. J. Agric. Econ. 62, 1–24. doi: 10.1111/j.1477-9552.2010.00274.x
Stigler, G. J., and Becker, G. S. (1977). De Gustibus Non Est Disputandum. Am. Econ. Rev. 67, 76–90.
Stiglitz, J. E. (1969). Behavior towards risk with many commodities. Econometrica 37, 660–667. doi: 10.2307/1910441
Tian, T., Zhao, X., and Cao, B. (2024). Risk perception, risk preference and farmers’ indifference in food sales. Appl. Econ. Lett., 1–8. doi: 10.1080/13504851.2024.2332526
Tripathi, A. K. (2024). Price support policy and market price dynamics: the case of Indian wheat. Agric. Econ. 55, 412–427. doi: 10.1111/agec.12825
Winterfeldt, D. V., and Edwards, W. (1986). Decision Analysis and Behavioral Research. Am. Econ. Rev. Cambridge: Cambridge University Press.
Xu, J., Chu, Y., and Feng, L. (2018). Analysis of differences in food selling behavior and influencing factors of farm households of different scales. Rural Econ. 11, 102–109.
Keywords: food price, risk aversion, food sale channels, certainty effect, farmers’ food sales behaviour
Citation: Tian T, Su YY and Chen P (2025) Small profits mean peace: food price, risk aversion, and farmers’ choices of sale channels. Front. Sustain. Food Syst. 9:1501600. doi: 10.3389/fsufs.2025.1501600
Received: 25 September 2024; Accepted: 06 January 2025;
Published: 22 January 2025.
Edited by:
Maria Alzira Pimenta Dinis, Fernando Pessoa University, PortugalReviewed by:
Peter Moffatt, University of East Anglia, United KingdomCopyright © 2025 Tian, Su and Chen. This is an open-access article distributed under the terms of the Creative Commons Attribution License (CC BY). The use, distribution or reproduction in other forums is permitted, provided the original author(s) and the copyright owner(s) are credited and that the original publication in this journal is cited, in accordance with accepted academic practice. No use, distribution or reproduction is permitted which does not comply with these terms.
*Correspondence: Tan Tian, dHRyeXVAMTI2LmNvbQ==
Disclaimer: All claims expressed in this article are solely those of the authors and do not necessarily represent those of their affiliated organizations, or those of the publisher, the editors and the reviewers. Any product that may be evaluated in this article or claim that may be made by its manufacturer is not guaranteed or endorsed by the publisher.
Research integrity at Frontiers
Learn more about the work of our research integrity team to safeguard the quality of each article we publish.