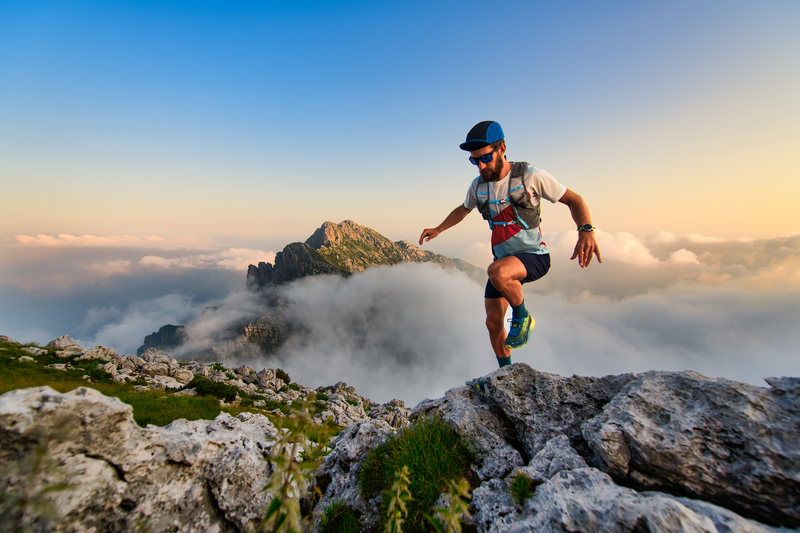
95% of researchers rate our articles as excellent or good
Learn more about the work of our research integrity team to safeguard the quality of each article we publish.
Find out more
ORIGINAL RESEARCH article
Front. Sustain. Food Syst. , 11 March 2025
Sec. Agricultural and Food Economics
Volume 9 - 2025 | https://doi.org/10.3389/fsufs.2025.1496295
This study examines the impact of information and communication technology (ICT) adoption on food security in emerging seven countries: namely China, India, Indonesia, Russia, Mexico, Brazil, and Turkey from 1995 to 2019. To obtain robust findings, we deploy several econometric approaches, including the Westerlund ECM co-integration test, PMG method, and D-H causality test. This research paper presents significant findings through the utilization of panel co-integration tests as the primary estimation techniques. The findings from the PMG method reveal that increases in usage of Mobile phone and Internet access significantly boost long-term food security by 0.092 and 0.036%, respectively. In addition, the findings from the Dumitrescy–Hurlin causal relationship test show a bidirectional causal relationship between Mobile phone use and food security but a unidirectional causality from Internet use to food security. Furthermore, the impact of ICT on food security is more prominent in the long run than in the short run. The current study’s conclusions have significant policy implications for the E7 countries. For example, the E7 countries should invest in ICT to gain future benefits. Authorities in the countries should develop and implement policies that encourage Mobile phone and Internet use in rural areas. Such a promotion will strengthen the farming system while also increasing sustainable food production.
The advent of information and communications technology (ICT) had a profound impact on various industries worldwide, including agriculture, which was not immune to its far-reaching effects (Chandio et al., 2024). The rapid growth of the world population has led to an increased demand for food, hence necessitating the urgent need to improve agricultural output (Chandio et al., 2023a; Farooq et al., 2023; Khan et al., 2022). This concern is particularly relevant to the Emerging 7 (E7) economies, namely, “China, India, Indonesia, Russia, Mexico, Brazil, and Turkey,” which are marked to become major markets by 2050 (PwC, 2017). Nations distinguished by rapid economic advancement and burgeoning influence on the global platform hold a pivotal position in the realm of global food provisioning. Consequently, grasping the implications of ICT on food productivity within such economies bears significant ramifications of significant magnitude.
Agriculture stands as the foundation of the E7 markets, employing sizable influence over their Gross Domestic Product (GDP) and employment backdrop. Particularly, in India, farming sector hires more than 50% of rural labor force and contributes about 17% to the overall GDP (Baig et al., 2023). Similarly, in China, despite the quick developments of industrialization, agriculture remains a vital sector, sustaining employment for nearly 25% of the population (Chandio et al., 2023b). Likewise, farming sector has a fundamental role in the profitable outlook of the remaining E7 countries. In this context, food productivity appears as an utmost indicator of agricultural sector vitality. The expansion of food productivity has emerged as an imperative priority within these economies, aimed at ensuring food security, alleviating poverty, and targeting sustainable development.
In the current times, the attention has progressively transformed toward the role of ICT in the farming sector. Encircling a diverse range of technologies, covering from traditional media for instance radio and television to cutting-edge systems like geographic information systems, remote sensing, and mobile applications, ICT shows the potential to revolutionize the agricultural industry. By yielding timely and precise information, enhancing market accessibility, and optimizing resource utilization efficiency, ICT emerges as promising in reshaping agricultural practices. However, despite its growing importance, there exists a lack of comprehensive studies revealing the applications and influences of ICT within the agricultural sector of E7 markets.
The transformative influence of ICT on augmenting agricultural productivity and food provisioning has acquired substantial scholarly attention in recent times. The integration of ICT into farming practices has been associated with intensified efficiency and productivity, adopting advancements in the landscape of food security (FS) (Aker, 2011; Bimber, 2003). Technologies such as Mobile phones, Internet, and satellite imagery have led a revolution in agricultural practices, empowering farmers with access to precise information regarding weather patterns and optimal farming techniques (Aker and Mbiti, 2010).
Despite the hopeful prospects offered by ICT, the agricultural domain within the E7 economies meets a numerous of challenges that hinder the realization of its full potential benefits. These challenges involve deficiencies in infrastructure, limited digital literacy among farmers, and inadequate policy frameworks. In addition, the impact of ICT on food productivity is not uniform and is subject to disparities influenced by factors such as the type of technology deployed, the contextual nuances of its utilization, and the distinct characteristics of each economy’s agricultural sector. Against this backdrop, empirical investigation requires a thorough exploration of these complexities, aiming to provide insights into how ICT can effectively augment food productivity within the E7 economies.
Preceding studies into the influence of ICT on agriculture have returned varied outcomes. While certain studies have uncovered advantageous effects, including enhanced access to market intelligence, increased adoption of optimal agricultural practices, and high productivity (Armenta-Medina et al., 2020; Toriyama, 2020; Zhang et al., 2016), others have shed light on the challenges and constraints associated with ICT integration in agriculture. These challenges embrace issues of accessibility, affordability, and usability (Ma and Zheng, 2022; Rahman et al., 2023). However, extant studies have focused on the adoption of ICT, rather than exploring into its direct impact on food productivity. In the context of the E7 countries, research remains relatively limited. Although a few studies probed into the role of ICT in agriculture in individual E7 countries, such as (Zhang et al., 2021; Zheng et al., 2021) for China and (Balkrishna et al., 2020) for India, a comprehensive analysis covering all the E7 countries is noticeably lacking. Consequently, a comprehensive research in this area, focusing on the impact of ICT on food yield, is required.
Mobile phone users, Internet users, and cereal output in the emerging markets are depicted in Figures 1–3. Figure 1 shows that the number of mobile users grew during the period in the seven emerging economies; however, most of the nations demonstrated a stabilization or a slight decrease in mobile phones between 2015 and 2019. This result may be attributed to market saturation in a country that has mobile phones in large numbers. Similarly, Figures 2, 3 show that, except in Turkey and Indonesia, a gradual increase in the number of internet users and cereal output can be seen in the E7 countries. The graphs indicate that the sharp rise in the number of internet and mobile phones signaled a dramatic shift in the socioeconomic landscape of emerging nations. An analysis of this technological wave, in conjunction with cereal production, can reveal deep insights into the connection between technology and food supply. The selection of the emerging economies, covering diverse dynamics such as economic development, population growth, and environmental conditions, can offer a unique chance to understand the complex nature of FS issues.
The implications of this study extend to policy and practice. By furnishing evidence for the influence of ICT on food productivity in the E7 economies, this research can provide crucial insights that can inform policy decisions on the interplay between ICT and agriculture. Policymakers can leverage the insights to design and implement strategies that can promote the effective utilization of ICT in the agricultural sector and thus augment food productivity and fortify FS and sustainable development. This study adds a few new things to the related literature. Firstly, this research examines the impact of Mobile phone use on FS by incorporating agricultural factors as control variables, specifically, harvested area, fertilizer consumption, agricultural employment, and cereal yield. Secondly, this research also scrutinizes the influence of the Internet access on FS in the E7 economies. Thirdly, this study uses serval econometric techniques, including the second-generation panel unit root tests-CADF, panel co-integration method-Westerlund ECM, PMG method, and D-H causality test for panel data estimation. Lastly, this study provides actionable recommendations to policymakers and practitioners, illuminating how they can harness ICT to augment food productivity.
The sections of the study in coming part are designed as follows: Section 2 offers a detailed literature review, encapsulating the evolution of ICT in agriculture and prior research on its impact on agricultural productivity; Section 3 elucidates the methodology adopted for this study, encompassing the data sources and analytical techniques employed; and Section 4 presents the empirical outcomes, followed by discussions about it. The study goes further in Section 5, where the main findings are sum up, the consequences for policy and practice are illustrated, and future research avenues are discussed in detailed.
A great deal of theoretical and empirical research on how ICT may improve FS can be seen in recent literature. FS means that there is always enough food available for everyone to eat in quantities that are both sufficient and healthy (Manikas et al., 2023). The word ‘ICT’ is used to refer to a wide range of technologies that make it easier to create, save, transmit, and process information and communicate (Ahmad et al., 2022; Gonzalez et al., 2022). ICT can potentially improve FS by empowering farmers, consumers, traders, and policymakers to access timely and accurate information, improve market efficiency and transparency, optimize resource allocation and utilization, and foster innovation and learning (Anser et al., 2021; Ayim et al., 2022). However, the impact of ICT on FS is not uniform and depends on several factors, such as the type of technology used, the context of its application, the characteristics of the users, and the institutional and policy environment (Biswal and Jenamani, 2018). Therefore, a comprehensive and systematic framework is needed to capture the multidimensional and multifactorial nature of ICT impact on FS.
In the recent years, the role of ICT in farming sector has been a subject of intensive inquiry. The evolution of ICT in agriculture, as elucidated by Maarsingh et al. (2023), demonstrated a trajectory from rudimentary tools for record keeping and weather forecasting in the early 20th century to sophisticated systems for precision farming and remote sensing in the 21st century. The authors contended that the adoption of ICT substantially amplified agricultural output and food production, albeit not without challenges, such as the digital divide and imperative for tailored training and education for farmers. For example, Paul et al. (2023) scrutinized the differential effects of ICT, energy intensity, urbanization, and inequality on agricultural output in a sample of 20 Asia-Pacific nations spanning the period from 1990 to 2020. Existing research endeavors have consistently evidenced that fluctuations, whether favorable or adverse, stemming from ICT, energy intensity, and the process of urbanization, exert an extended asymmetric influence on the efficacy of agricultural activities. The findings indicated that the implementation of ICT and efficient energy management in the agricultural sector can greatly enhance agricultural production.
An empirical research accompanied by Qiubo et al. (2020) on farming sector investigated the effects of ICT on total factor productivity (TFP) from 2004 to 2016. The findings of the study showed that ICT has the likelihood to boost agricultural TFP by improving agricultural technical efficiency. Very recently, an empirical study conducted by Domguia and Asongu (2022) inspected the interconnection between ICT and agricultural productivity in 18 countries in Sub-Saharan Africa. Their research spanned the period from 1990 to 2014, and they found evidence suggesting that the presence of ICT, including internet access and mobile and fixed-line telephone usage, had a positive and beneficial effect on agricultural production. The study conducted by Chandio et al. (2022) scrutinized the influences of ICT on cereal productivity in seven Asian markets from 2000 to 2018. The study’s observed outcomes suggest that the utilization of ICT has the potential to enlarge cereal productivity. The investigation described above collectively indicate that the use of ICT into agricultural practices has the potential to significantly enhance food yield.
Mittal (2012) emphasized the potential of modern ICT in the context of developing countries, specifically in relation to agricultural development within smallholder agriculture of India. The research highlighted the significance of ICT in providing farmers with essential resources such as market information, weather forecasts, and agricultural guidance. This, in turn, can contribute to improved productivity and higher revenue levels. The impact of ICT on agricultural productivity has generally been proven to be auspicious, as discerned by Spielman et al. (2021). The authors’ review of extant studies on the subject demonstrated that the magnitude of influence varies, contingent on the type of ICT deployed and the context in which it was operationalized. The review also underscored the imperative for in-depth inquiry to reveal the mechanisms through which ICT can influence productivity and identify the most efficacious modes for implementing and employing ICT in diverse agricultural settings. The transition from the Green Revolution to the Digital Revolution in agriculture was profoundly impacted by ICT. Abdulai (2022) argued that ICT plays an indispensable role in this transition by enabling efficient and sustainable farming practices. The author furnished numerous case studies illustrating how ICT has been harnessed to augment food production, encompassing the utilization of remote sensing for crop monitoring and digital platforms for farm management.
When we look at the studies on the impact of ICT on food production in developing economies, we see that there are more narrow studies. Emerging economies often grapple with distinct challenges in the adoption and implementation of ICT in agriculture, including limited infrastructure, restricted technology access, and farmers’ dearth of technical expertise. Such challenges can curtail the efficacy of ICT in increasing food production and agricultural productivity. Nonetheless, evidence shows that, when effectively implemented, ICT can exert a positive influence on food production in emerging economies. For instance, mobile phones have been deployed to furnish farmers with access to market information, weather forecasts, and agricultural advice, resulting in heightened productivity and increased income (Aker, 2011). Similarly, digital platforms have facilitated connectivity between farmers and buyers/suppliers, thereby reducing transaction costs and ameliorating market access (Nakasone, 2013). However, a deep probe is warranted to comprehend the specific mechanisms through which ICT can augment food production in emerging economies and discern the barriers to ICT adoption and implementation in such contexts. The increasing importance of the concept of FS, especially in the face of major challenges such as climate change and population growth, makes such a study even more important. Hence, based on the above background of the empirical review of relevant literature, this research posits the following hypotheses:
Hypothesis 1: Mobile phone use significantly boosts food security in E7 economies.
Hypothesis 2: Internet use significantly enhances food security in E7 economies.
Despite the growing body of research on the use of ICT in farming sector, studies that particularly look at how ICT affects food productivity in the E7 economies are notably lacking. The majority of research focuses on either a single nation or one technology, failing to take into account the complexity and diversity of the E7 economies. Additionally, there is a lack of a thorough and comprehensive framework that can take into consideration the different aspects and factors that influence how ICT affects food production. By undertaking a cross-country comparative examination of the effect of ICT on food productivity in the E7 nations using a multidimensional and multifactorial methodology, this study seeks to close this gap. This research also addresses the following research questions: Does the usage of Mobile phone boost food security in the E7 economies? How does Internet access influence food security in the E7 economies? Do production factors enhance food security in the E7 economies? Figure 4 shows the determinants of food production.
This research investigates the influence of ICT on FS in the E7 nations, including “China, India, Indonesia, Russia, Mexico, Brazil, and Turkey,” throughout the time span from 1995 to 2019 (see Figure 5). Table 1 shows the abbreviations and data sources of the examined variables. This study uses the food production index as the dependent variable and Mobile phone use and Internet use as the fundamental independent variables. In addition, this study uses harvested area, fertilizer consumption, employment in agriculture, and cereal yield as the control variables. Data on all variables used in the analyzes were compiled from the World Bank database.
The main purpose of this study is to reveal the FS situation of the E7 countries and analyze how a modern and sustainable agricultural economy can be achieved in the countries in light of the selected indicators. In this study, the relationship between some selected indicators and FS was tried to be examined using two different models. The models created for the context are as follows in Equations 1, 2:
Model 1:
Model 2:
where FS, MOB, INT, HA, FER, EMP, and CY denote food security, Mobile phone use, Internet use, harvested area, fertilizer usage, agricultural employment, and cereal yield, respectively. Figure 6 depicts the flowchart of panel data estimations for E7 economies.
Whether or not the horizontal CSD between the series is considered can significantly affect the results (Breusch and Pagan, 1980; Pesaran, 2004). Therefore, before the analysis, the existence of horizontal CSD in the series and co-integration equation should be tested. This factor should be considered when choosing the unit root and co-integration tests; otherwise, the analysis may yield erroneous results. The existence of horizontal CSD can be tested with alternative methods, such as the Breusch and Pagan (1980) Lagrang multiplier (LM) test; Pesaran (2004) scaled LM_test, and Pesaran CSD test (Pesaran et al., 2008). The mathematical expression of the CSD test is as follows in Equation 3:
Once the horizontal common stochastic trends have been assessed, it is imperative to examine the stationarity of the variables. The subsequent empirical analysis entails examining the stationarity property of the variables. The stationarity of the variables is tested in this work using “cross-sectional Im–Pesaran–Shin (CIPS) and cross-sectional augmented Dickey–Fuller (CADF)” panel unit root tests, as suggested by Pesaran (2007). The mathematical representation of the CADF panel stationarity test is given by the following equation:
When one more lag value (t − 1) is added to this equation, Equation 4 will be formed, as follows in Equation 5:
After this step, the CIPS unit root test can be expressed, as follows in Equation 6:
where indicates the panel CADF; thus, it can be replaced in a new function as in Equation 7:
As suggested by Westerlund (2007), the probability values obtained from the “bootstrap” distribution can account for the horizontal CSD, which are shown in the table. Once the examination of the stationarity of the series has been done, the selection of the appropriate cointegration test is determined based on the acquired information. The sort of panel cointegration test to be undertaken is contingent upon the degree of stationarity exhibited by the variables, as assumed in the analysis. In order to derive the panel test statistics for the Westerlund cointegration test, the estimation of the following model is undertaken in Equation 8:
To test whether a co-integration relationship exists we estimated Equations 9, 10 as follows:
The hypothesis are as follows:
• H0: = 0, no co-integration exists for all the cross-sections.
• H1: < 0, co-integration exists for some cross-sections.
In case Ho rejected, it indicates that a co-integration relationship exists between the variables for at least one horizontal section.
In the next stage, the error correction coefficient in the entire panel and its standard error are calculated in Equations 11, 12 as follows.
The hypothesis are as follows:
• H0: = 0, no co-integration exists for all the horizontal sections.
• H1: = < 0, co-integration exists for all the horizontal sections.
Rejecting the null hypothesis means that there is a cointegration between all variables.
In this work, the PMG estimate approach described by Pesaran et al. (1999) was employed to analyze the interactions among the variables under consideration. This approach demonstrates enhanced efficiency and consistency in both short run (SR) & long run (LR) estimation. The technique discussed in the preceding study is commonly utilized for estimating panel datasets. According to Pesaran and Smith (1995), the PMG estimator is known for its ability to generate dependable and effective parameter estimates. Notably, this approach eliminates the need for pre-testing the order of integration, namely whether the variables are stationary (I (0)) or possess a unit root (I (1)). Therefore, this study employed the aforementioned methodology to investigate the enduring and immediate effects of ICT on FS in the E7 nations. Equation 13 presents the long-term autoregressive distributed lag (ARDL) model in the specified sequence:
The time frame and cross-sectional unit are denoted by t and i, while indicates the exogenous variables LnMOB/LnINT, which represent the Mobile phone/Internet use, LnHA (agricultural harvested area), LnFER (fertilizer consumption), LnEMP (employment in agriculture), and LnCY (cereal yield), respectively. Moreover, p and q represent the lag orders and stands for the error term. The SR estimation of the variables, following Equation 14 is formulated as:
Table 2 shows the descriptive statistics of the examined variables. The mean value of FS is 82.945 with a standard deviation of 16.391, while the mean value of MOB and INT is 59.144 and 23.419. It shows that the adaptation of Mobile phone is higher in E7 economies, however the infrastructure of Internet access needs improvement. The descriptive statistics of other variables show that the mean values of HA, FER, EMP, CY are 412, 155.091, 28.011, and 3,447.396. Moreover, Figures 7, 8 reveal that Mobile phone usage and Internet usage are significantly correlated with FS.
Checking the CSD of the data is imperative. Thus, in this investigation, various CSD tests are employed, including the “Breusch–Pagan LM, Pesaran scaled LM, Pesaran CD, and bias-corrected scaled LM tests.” The CSD test outcomes are detailed in Table 3. The calculated results validate the presence of CSD in the dataset, and at the 1% significance level, all variables exhibit statistical significance. Therefore, the outcomes validate the existence of CSD among FS, Mobile phone use and Internet use, harvested area, fertilizer consumption, employment in agriculture, and cereal yield.
Table 4 presents the outcomes of the Levin–Lin–Chu (LLC) and IPS panel unit root tests. In the LLC test, only LnFS, LnFER, and LnCY are stationary at I (1), and LnMOB, LnINT, LnHA, and LnEMP are stationary at I (0) and I (1). The IPS test obtains the same results as the LLC test. The dependent variable LnFS is I (1) in the LLC and IPS tests; thus, it is a necessary condition for the PMG model. The outcomes of both tests are also verified by using the CADF unit root test (see Table 5).
Once the panel data stationarity has been confirmed, it is necessary to examine the long-term co-integration between the series. The Westerlund ECM panel cointegration tests are performed in relation to this matter. The findings for Models 1–2 are presented in Table 6, providing evidence that supports the existence of co-integration. This research also employs the Kao panel cointegration test to check the robustness of the findings of the Westerlund ECM test. The outcomes are reported in Table 7, confirming that there is a long-term connection between the variables.
In this study, the PMG estimate method, as introduced by Pesaran et al. (1999), is employed to examine the effects of explanatory variables on FS in the selected E7 economies, both in the LR and SR. The empirical results of the PMG estimation for (Model 1), both in the SR and LR, are displayed in Table 8. Figure 9 shows the main findings of long-run estimate for Model 1. The results of the PMG estimate reveal that Mobile phone use improves FS in both LR and SR. The coefficients of Mobile phone use are positive concerning FS. Therefore, a 1% increases in the usage of Mobile phone in farming would lead to improves FS by 0.09 and 0.01% in E7 countries. The association between Mobile phone usage and FS suggests that the utilization of ICTs significantly promotes sustainable food production and contemporary agriculture. Furthermore, the adoption of ICT is often regarded as a crucial means to enhance farmers’ income and improve their overall wellbeing (Zhang et al., 2021; Zheng et al., 2021; Zou and Mishra, 2022). Our empirical results are in line with Chandio et al. (2022), Onyeneke et al. (2023), and Paul et al. (2023). Cai et al. (2022) shown that the adoption of Mobile & Internet in China has a substantial impact on the extent of technology adoption. Furthermore, the degree to which technology is adopted is also influenced by factors such as educational attainment, training, the proportion of income derived from litchi farming, utilization of guidebooks, and membership in agricultural cooperatives. The findings from disaggregated studies provide more evidence to support the notion that the adoption of Mobile & Internet has a beneficial influence on the adoption of technologies that require significant capital and labor resources. A study in the context of Ghana, Issahaku et al. (2018) revealed that the usage of Mobile phone considerably improves agricultural output. The Mobile phone, in a more precise manner, enhances the output of user-farmers by a minimum of 261.20 kg/ha during each production season.
The significance and favorable impact of agricultural land on FS are evident in both the SR and LR. The findings indicate that the availability of agricultural land has a positive impact on FS. An increase of 1% in agricultural land has been found to have a positive impact on FS, resulting in a respective rise of 0.30 and 0.21%. The findings suggest that the cultivation of agricultural land has a significant role in enhancing FS in E7 nations. This finding is similar to the finding of Anh et al. (2023), Farooq et al. (2023), Gul et al. (2022a), Gul et al. (2022b), Nouman et al. (2022).
The utilization of fertilizer has a good long-term effect on food production, but conversely, it has a detrimental SR impact. The coefficient of fertilizer usage in the long run, which is 0.20, suggests that a 1% increase in fertilizer usage leads to a corresponding 0.20% increase in food production. This finding aligns with the findings reported by Zhang et al. (2022a). The proper use of fertilizer is essential for optimizing crop yield, as it serves as a crucial ingredient in promoting plant growth and development. Fertilizer is a crucial component in the cultivation and development of plants (Zhang et al., 2022b).
Cereal production is a fundamental for promoting the FS. It is observed that cereal production has positive and significant impact on FS. The coefficients of cereal production both in the long and SR, which are 0.18 and 0.30, indicate that a 1% increases in food production would lead to promote FS by 0.18 and 0.30%. In the industry of agricultural systems, the adoption of modern agricultural technology, enhanced cultivation techniques, financial accessibility, environmentally conscious investments in agriculture, and technological advancements collectively contribute to the enhancement of food production and the assurance of FS (Affoh et al., 2022; Chandio et al., 2022).
Table 9 presents the robustness of the long run association between FS and its linked variables. This research employs the FMOLS and DOLS methods to further explore the long run estimations of the connection between variables, and the robustness results of both approaches are consistent with the findings obtained from the ARDL procedure. The robustness results reveal that all variables are statistically significant in the FMOLS regression except HA. Mobile phone use and other production factors positively and significantly boot food security in E7 economies. The results obtained from FMOLS and DOLS are robust and reliable.
The empirical outcomes of the PMG estimation for (Model 2) are presented in Table 10. The estimated coefficients of LnINT, LnHA, LnFER, and LnCY are statistically significant at 1% significance level; expect LnEMP in the long term, respectively. Figure 10 shows the major long-run findings for Model 2.
The long-term findings verify that Internet use is highly likely to boost FS in E7 countries. These findings also indicate that a 1% upsurge in Internet use would increase FS by 0.03%. However, in the SR Internet use adversely influence FS. This outcomes supports earlier study of Kaila and Tarp (2019), who found that there is a positive correlation between Internet connectivity and a 6.8% increase in the overall volume of agricultural productivity. Additionally, it was discovered that this outcome is demonstrated by a heightened level of fertilizer utilization efficiency. The research results also demonstrate a higher degree of statistical significance among households in younger age groups. The emergence of the Internet has had a disproportionately positive impact on the underdeveloped provinces in the northern region of Vietnam. In a recent study conducted by Chandio et al. (2023b), who found that Internet usage has a considerable positive impact on FS in China. The utilization of the Internet promotes the engagement of farmers in the procurement of inputs, as well as the buying and selling in the market, thereby reducing the traveling expenses (Cai et al., 2022; Zheng et al., 2021). The usage of the Internet is a very effective technological advancement and innovative instrument that farmers can easily adopt in order to derive additional advantages from its implementation (Rahman et al., 2023; Zhang et al., 2023). In addition, the Internet use may also facilitate the acquisition of novel agricultural knowledge and foster efficient information exchange among farmers, hence enhancing agricultural productivity and augmenting income levels (Kaila and Tarp, 2019; Onyeneke et al., 2023; Oyelami et al., 2022).
In the LR and SR, the association between agricultural harvested area and FS is statistically significant and positive at 1 and 5% significance levels, meaning that agricultural harvested area significantly promotes FS. Specifically, a 1% increase in agricultural harvested area would lead to improve FS by 0.32 and 0.20%. The results infer that agricultural land is a crucial factor in improving FS in E7 countries. This finding is similar to the findings of Affoh et al. (2022) and Pickson et al. (2022).
In the long-term, the association between fertilizer usage and FS is statistically significant and positive at the 1% significance level, showing that fertilizer use significantly increases FS. To be more specific, a 1% increase in fertilizer use would result in a 0.34% increase in FS. This finding supports Baig et al. (2023). Since fertilizer is the actual production and plant development component. Hence, proper fertilizer application is primarily essential to improve crop yields.
In addition, at the 1 and 5% significance levels, the estimated LR and SR coefficients of employment in agriculture and cereal production are positive and statistically significant. According to these findings, a 1% increase in agricultural and cereal production employment would raise FS by 0.10, 0.13, 0.70, and 0.26%, respectively. Agricultural labor and food production can be considered as playing an essential role in ensuring FS in E7 countries. Such findings are also visible in the earlier literature (Affoh et al., 2022; Akhtar and Masud, 2022; Anh et al., 2023; Bhardwaj et al., 2022; Ozdemir, 2022).
The current study re-estimates the long run regression results of the PMG method by using the FMOLS and DOLS regression methods. The results of the robustness tests are shown in Table 11. We can see that the regression results of the FMOLS method are positive and significant except EMP. This means that Internet use and other variables can improve food security in E7 countries.
Although this study reveals the LR and SR dynamics and co-integration between Mobile phone use, Internet use, harvested area, fertilizer consumption, employment in agriculture, cereal yield, and FS, it does not verify the causality movement. To elucidate the directionality of causality between the variables, this study employs the Dumitrescu–Hurlin (DH) panel causality test. Table 12 delineates the diverse pathways of the causal nexus between the variables. The findings demonstrate a bidirectional link between Mobile phone use and FS, which is consistent with the outcomes obtained by previous studies. Furthermore, bidirectional causality is observed between harvested area, fertilizer consumption, employment in agriculture, cereal yield, and FS. The results confirm that Mobile phone use, agricultural cultivated land, fertilizer use, agricultural employment, and cereal production play a key role in promoting FS and achieving the SDGs in the E7 economies.
The present study explores the influence of ICT on FS in the E7 countries from 1991 to 2019. In addition, this study develops two models to answer the research questions. The first model examines the relationship between FS, as the dependent variable, and Mobile phone use, as the main proxy for ICT, in the LR and SR. The second model examines the relationship between FS and Internet use, as the proxy for ICT, in the LR and SR. In both models, agricultural variables are added as the controls, namely, harvested area, fertilizer usage, agricultural employment, and cereal yield. The data of all the variables are obtained from the World Bank. This study conducts CSD and stationary tests and the PMG estimation proposed by Pesaran et al. (1999) as the primary estimation methods.
The analysis results provide some interesting findings. Through the first model, this study demonstrates that Mobile phone use can improve FS in the LR and SR. Specifically, a 1% increase in Mobile phone usage in farming will improve FS by 0.09% in the SR and by 0.01% in the long run in the sample countries. The relationship between Mobile phone usage and FS suggests that the utilization of ICT can significantly promote sustainable food production and contemporary agriculture. In addition, some of the control variables are statistically significant. That is, in the first model, harvested area and fertilizer consumption are positively and statistically significantly affecting FS in the long run in the sample countries. Meanwhile, in the SR, harvested area and cereal yield are positively and statistically significantly affecting FS in the sample countries.
In the second model, Internet use will likely promote FS in the E7 countries more in the long run than in the SR. Specifically, a 1% increase in Internet usage will enhance FS by 0.03% in the long run. However, in the SR, Internet use will negatively influence FS. Moreover, some of the control variables are statistically significant. This study also re-estimated the long run association between the variables by using the FMOLS and DOLS and verified the same findings of the PMG method. In addition, the DH causal relationship test demonstrates a bidirectional causal relationship between Mobile phone use and FS but a unidirectional causal relationship from Internet use to FS.
The results show that ICT can benefit FS more in the long run than in the SR. ICT will have a positive impact on FS in the long run; thus, the E7 countries should invest in ICT to reap its benefits in the future. Specifically, authorities in the countries should formulate and implement policies that can promote Mobile phone and Internet use. Such a promotion will accelerate infrastructure development to support ICT, such as strong networks, fast bandwidth speed, and affordable Mobile phones. From the business perspective, authorities should encourage firms, especially those in the food sector, to utilize Mobile phones and the Internet in distributing their final goods or selling their food, which can reduce transportation costs, final prices, and overall consumer surplus. Furthermore, the government should support the development of a web marketplace and encourage firms to utilize the platform.
In addition to Mobile phone use and Internet use, policies to increase harvested areas and cereal yields are important. To achieve such goals, fertilizer use should be increased to increase harvested areas and cereal yields. Such efforts are expected to accelerate the positive impact of Mobile phone use and Internet use on FS in the long run. Furthermore, labor policies supporting agricultural employment are crucial to strengthen the impact of Internet use on FS. Thus, authorities must maintain price stability in the agricultural sector to stabilize agricultural employees’ income and maintain employment in the sector. The results of the causality test reveal that agricultural employment and FS have a bidirectional causal relationship; thus, public policies strengthening the agricultural sector must be prioritized.
This study has some limitations. First, this study uses only two ICT variables, namely, Mobile phone use and Internet use, as proxies for ICT in the sample countries, which may be considered as limited. Other variables can be used to measure ICT accurately, such as the number of households or individuals with a computer, the volume of online traffic, or the proportion of ICT costs in the total average income. However, owing to various constraints, this study used only the two variables. Second, though the sample period is 1991–2019, future studies can extend the study period up to 2023 based on the data availability. Lastly, this study incorporates agricultural factors as control variables, including agricultural cultivated land, fertilizer use, and agricultural employment, however other critical factors influencing food security, such as climate change, capita/machinery, water application, political stability, and economic policies can be considered in further investigation in Asian developing countries. Future research should address the aforementioned issues and limitations.
This study lays a solid foundation for future research to understand the impact of ICT on FS. However, future studies can improve this study to a certain extent. First, future studies can expand this research by elaborating on the proxies for either FS or ICT. From the perspective of ICT, technology-related proxies can be developed by incorporating variables such as the number of households or individuals with a computer, the volume of online traffic, the proportion of ICT costs in the total average income, and so on. Furthermore, future studies can aggregate multiple ICT-related variables into a single index using principal component analysis (PCA), then incorporate the PCA index into the model. Similarly, other FS metrics can be employed, such as the proportion of malnourished adults or children in the total population, or poverty-related variables, as the controls, in the model. Second, future studies can compare panel versus individual regressions to measure the impact gap between individual countries and group countries. Although the sample countries are categorized into a single group, some of the countries may be more developed than the others (e.g., China vs. Mexico). Panel versus individual regressions can also be compared to ensure that policy recommendations for one country are not identical to those for another country. Last, future studies should conduct robustness tests by generating models with alternative key independent variables or by incorporating external shocks, such as the 1997 Asian crisis or 2008 global financial crisis.
The raw data supporting the conclusions of this article will be made available by the authors without undue reservation.
AC: Conceptualization, Formal analysis, Project administration, Supervision, Validation, Writing – original draft, Writing – review & editing. MA: Funding acquisition, Validation, Visualization, Writing – original draft, Writing – review & editing. AA: Data curation, Formal analysis, Investigation, Methodology, Validation, Writing – original draft, Writing – review & editing. IH: Investigation, Methodology, Software, Validation, Visualization, Writing – original draft, Writing – review & editing. ND: Data curation, Formal analysis, Investigation, Methodology, Software, Writing – original draft, Writing – review & editing.
The author(s) declare that financial support was received for the research, authorship, and/or publication of this article. The authors of this study extend their appreciation to Researchers Supporting Project number (RSPD2025R932), King Saud University, Riyadh, Saudi Arabia.
The authors declare that the research was conducted in the absence of any commercial or financial relationships that could be construed as a potential conflict of interest.
All claims expressed in this article are solely those of the authors and do not necessarily represent those of their affiliated organizations, or those of the publisher, the editors and the reviewers. Any product that may be evaluated in this article, or claim that may be made by its manufacturer, is not guaranteed or endorsed by the publisher.
Abdulai, A.-R. (2022). A new green revolution (GR) or neoliberal entrenchment in agri-food systems? Exploring narratives around digital agriculture (da), food systems, and development in sub-Sahara Africa. J. Dev. Stud. 58, 1588–1604. doi: 10.1080/00220388.2022.2032673
Affoh, R., Zheng, H., Dangui, K., and Dissani, B. M. (2022). The impact of climate variability and change on food security in sub-Saharan Africa: perspective from panel data analysis. Sustain. For. 14:759. doi: 10.3390/su14020759
Ahmad, S., Khan, D., and Haq, I. U. (2022). Assessing the role of information and communication technology in reducing the gap between rich and poor: the case of South Asia. Int. J. Soc. Econ. 49, 1663–1679. doi: 10.1108/IJSE-10-2021-0638
Aker, J. C. (2011). Dial “a” for agriculture: a review of information and communication technologies for agricultural extension in developing countries. Agric. Econ. 42, 631–647. doi: 10.1111/j.1574-0862.2011.00545.x
Aker, J. C., and Mbiti, I. M. (2010). Mobile phones and economic development in Africa. J. Econ. Perspect. 24, 207–232. doi: 10.1257/jep.24.3.207
Akhtar, R., and Masud, M. M. (2022). Dynamic linkages between climatic variables and agriculture production in Malaysia: a generalized method of moments approach. Environ. Sci. Pollut. Res. 29, 41557–41566. doi: 10.1007/s11356-021-18210-x
Anh, D. L. T., Anh, N. T., and Chandio, A. A. (2023). Climate change and its impacts on Vietnam agriculture: a macroeconomic perspective. Eco. Inform. 74:101960. doi: 10.1016/j.ecoinf.2022.101960
Anser, M. K., Osabohien, R., Olonade, O., Karakara, A. A., Olalekan, I. B., Ashraf, J., et al. (2021). Impact of ICT adoption and governance interaction on food security in West Africa. Sustain. For. 13:5570. doi: 10.3390/su13105570
Armenta-Medina, D., Ramirez-delReal, T. A., Villanueva-Vásquez, D., and Mejia-Aguirre, C. (2020). Trends on advanced information and communication technologies for improving agricultural productivities: a bibliometric analysis. Agronomy 10:1989. doi: 10.3390/agronomy10121989
Ayim, C., Kassahun, A., Addison, C., and Tekinerdogan, B. (2022). Adoption of ICT innovations in the agriculture sector in Africa: a review of the literature. Agric. Food Secur. 11, 1–16. doi: 10.1186/s40066-022-00364-7
Baig, I. A., Mohammad, S., Akram, V., Chandio, A. A., and Gupta, Y. (2023). Examining the impacts of climatological factors and technological advancement on wheat production: a road framework for sustainable grain production in India. Environ. Dev. Sustain. 26, 12193–12217. doi: 10.1007/s10668-023-03746-4
Balkrishna, A., Mittal, R., Kumar, A., Singh, D., and Arya, V. (2020). Analysis of policy interventions in agriculture and ICT based mechanistic approach towards sustainability: an Indian perspective. Asian J. Agric. Rural Dev. 10, 194–213. doi: 10.18488/journal.1005/2020.10.1/1005.1.194.213
Bhardwaj, M., Kumar, P., Kumar, S., Dagar, V., and Kumar, A. (2022). A district-level analysis for measuring the effects of climate change on production of agricultural crops, ie, wheat and paddy: evidence from India. Environ. Sci. Pollut. Res. 29, 31861–31885. doi: 10.1007/s11356-021-17994-2
Bimber, B. (2003). Information and American democracy: technology in the evolution of political power : Cambridge University Press.
Biswal, A. K., and Jenamani, M. (2018). Leveraging ICT for food security: an analysis in the context of PDS in India, Social transformation–digital way: 52nd annual convention of the Computer Society of India, CSI 2017, Kolkata, India, January 19–21, 2018, revised selected papers 52. Springer, pp. 376–390.
Breusch, T. S., and Pagan, A. R. J. T. r. o. e. s. (1980). The Lagrange multiplier test and its applications to model specification in econometrics. 47, 239–253.
Cai, Y., Qi, W., and Yi, F. (2022). Mobile internet adoption and technology adoption extensity: evidence from litchi growers in southern China. China Agric. Econ. Rev. 14, 106–121. doi: 10.1108/CAER-11-2020-0260
Chandio, A. A., Bashir, U., Akram, W., Usman, M., Ahmad, M., and Jiang, Y. (2023a). What role do international remittance inflows play in boosting agricultural productivity? Empirical analysis of emerging Asian economies. Int. J. Emerg. Mark. [ahead-of-print]. doi: 10.1108/IJOEM-06-2022-1019
Chandio, A. A., Gokmenoglu, K. K., Khan, I., Ahmad, F., and Jiang, Y. (2023b). Does internet technology usage improve food production? Recent evidence from major rice-producing provinces of China. Comput. Electron. Agric. 211:108053. doi: 10.1016/j.compag.2023.108053
Chandio, A. A., Ozdemir, D., Gokmenoglu, K. K., Usman, M., and Jiang, Y. (2024). Digital agriculture for sustainable development in China: the promise of computerization. Technol. Soc. 76:102479. doi: 10.1016/j.techsoc.2024.102479
Chandio, A. A., Sethi, N., Dash, D. P., and Usman, M. (2022). Towards sustainable food production: what role ICT and technological development can play for cereal production in Asian–7 countries? Comput. Electron. Agric. 202:107368. doi: 10.1016/j.compag.2022.107368
Domguia, E. N., and Asongu, S. (2022). ICT and agriculture in sub-Saharan Africa: effects and transmission channels. AGDI Working Paper.
Farooq, U., Gang, F., Guan, Z., Rauf, A., Chandio, A. A., and Ahsan, F. (2023). Exploring the long-run relationship between financial inclusion and agricultural growth: evidence from Pakistan. Int. J. Emerg. Mark. 18, 1677–1696. doi: 10.1108/IJOEM-06-2019-0434
Gonzalez, R., Gasco, J., and Llopis, J. (2022). Information and communication technologies in food services and restaurants: a systematic review. Int. J. Contemp. Hosp. Manag. 34, 1423–1447. doi: 10.1108/IJCHM-05-2021-0624
Gul, A., Chandio, A. A., Siyal, S. A., Rehman, A., and Xiumin, W. (2022a). How climate change is impacting the major yield crops of Pakistan? An exploration from long-and short-run estimation. Environ. Sci. Pollut. Res. 29, 26660–26674. doi: 10.1007/s11356-021-17579-z
Gul, A., Xiumin, W., and Chandio, A. A. (2022b). Tracking the effect of climatic and non-climatic elements on rice production in Pakistan using the ARDL approach. Environ Sci Pollut Res 29, 31886–31900. doi: 10.1007/s11356-022-18541-3
Issahaku, H., Abu, B. M., and Nkegbe, P. K. (2018). Does the use of mobile phones by smallholder maize farmers affect productivity in Ghana? J. Afr. Bus. 19, 302–322. doi: 10.1080/15228916.2017.1416215
Kaila, H., and Tarp, F. (2019). Can the internet improve agricultural production? Evidence from Viet Nam. Agric. Econ. 50, 675–691. doi: 10.1111/agec.12517
Khan, N., Ray, R. L., Zhang, S., Osabuohien, E., and Ihtisham, M. (2022). Influence of mobile phone and internet technology on income of rural farmers: evidence from Khyber Pakhtunkhwa Province, Pakistan. Technol. Soc. 68:101866. doi: 10.1016/j.techsoc.2022.101866
Ma, W., and Zheng, H. (2022). Heterogeneous impacts of information technology adoption on pesticide and fertiliser expenditures: evidence from wheat farmers in China. Aust. J. Agric. Resour. Econ. 66, 72–92. doi: 10.1111/1467-8489.12446
Maarsingh, B., Grobbelaar, S. S., Uriona-Maldonado, M., and Herselman, M. (2023). Exploring functional dynamics of innovation for inclusive development: event history analysis of an ICT4D project. Innov. Dev. 13, 243–273. doi: 10.1080/2157930X.2021.1956712
Manikas, I., Ali, B. M., and Sundarakani, B. (2023). A systematic literature review of indicators measuring food security. Agric. Food Secur. 12:10. doi: 10.1186/s40066-023-00415-7
Mittal, S. (2012). Modern ICT for agricultural development and risk management in smallholder agriculture in India : CIMMYT.
Nakasone, E. (2013). The role of price information in agricultural markets: experimental evidence from rural Peru.
Nouman, M., Khan, D., Ul Haq, I., Naz, N., Zahra, B. T. E., and Ullah, A. (2022). Assessing the implication of green revolution for food security in Pakistan: a multivariate cointegration decomposition analysis. J. Public Aff. 22:e2758. doi: 10.1002/pa.2758
Onyeneke, R. U., Ankrah, D. A., Atta-Ankomah, R., Agyarko, F. F., Onyeneke, C. J., and Nejad, J. G. (2023). Information and communication technologies and agricultural production: new evidence from Africa. Appl. Sci. 13:3918. doi: 10.3390/app13063918
Oyelami, L. O., Sofoluwe, N. A., and Ajeigbe, O. M. (2022). ICT and agricultural sector performance: empirical evidence from sub-Saharan Africa. Future Bus. J. 8:18. doi: 10.1186/s43093-022-00130-y
Ozdemir, D. (2022). The impact of climate change on agricultural productivity in Asian countries: a heterogeneous panel data approach. Environ. Sci. Pollut. Res. 29, 8205–8217. doi: 10.1007/s11356-021-16291-2
Paul, A., Sahoo, D., and Jena, A. (2023). Asymmetric impacts of ICT and energy intensity on agricultural productivity: evidence from Asia-Pacific region. Rev. Dev. Econ. 27, 1537–1561. doi: 10.1111/rode.12993
Pesaran, M. H., Shin, Y., and Smith, R. P. (1999). Pooled mean group estimation of dynamic heterogeneous panels. J. Am. Stat. Assoc. 94, 621–634. doi: 10.1080/01621459.1999.10474156
Pesaran, M. H., and Smith, R. (1995). Estimating long-run relationships from dynamic heterogeneous panels. J. Econ. 68, 79–113. doi: 10.1016/0304-4076(94)01644-F
Pesaran, M. H. (2007). A simple panel unit root test in the presence of cross‐section dependence. Journal of applied econometrics. 22, 265–312.
Pesaran, M. H. (2004). General diagnostic tests for cross section dependence in panels. Cambridge Working Papers. Economics. 1240:1.
Pesaran, M. H., Ullah, A., and Yamagata, T. (2008). A bias‐adjusted LM test of error cross‐section independence. The econometrics journal 11, 105–127. doi: 10.1111/j.1368-423X.2007.00227.x
Pickson, R. B., Gui, P., and Chen, A. (2022). Empirical analysis of rice and maize production under climate change in China. Environ Sci Pollut Res. 29, 70242–70261. doi: 10.1007/s11356-022-20722-z
PwC. (2017). The world in 2050: the long view: how will the global economic order change by 2050? Available online at: https://www.pwc.com/gx/en/research-insights/economy/the-world-in-2050.html (Accessed July 27, 2023).
Qiubo, Z., Junfei, B., Chao, P., and Chen, Z. (2020). Do ICTs boost agricultural productivity? China Economist 15, 9–26.
Rahman, M. S., Toiba, H., Nugroho, T. W., Hartono, R., Shaleh, M. I., Sugiono, S., et al. (2023). Does internet use make farmers happier? Evidence from Indonesia. Cogent Soc. Sci. 9:2243716. doi: 10.1080/23311886.2023.2243716
Spielman, D., Lecoutere, E., Makhija, S., and Van Campenhout, B. (2021). Information and communications technology (ICT) and agricultural extension in developing countries. Ann. Rev. Resour. Econ. 13, 177–201. doi: 10.1146/annurev-resource-101520-080657
Toriyama, K. (2020). Development of precision agriculture and ICT application thereof to manage spatial variability of crop growth. Soil Sci. Plant Nutr. 66, 811–819. doi: 10.1080/00380768.2020.1791675
Westerlund, J. (2007). Testing for error correction in panel data. Oxford Bulletin of Economics and statistics. 69, 709–748.
Zhang, A., Chandio, A. A., Yang, T., Ding, Z., and Liu, Y. (2023). Examining how internet use and non-farm employment affect rural households’ income gap? Evidence from China. Front. Sustain. Food Syst. 7:1173158. doi: 10.3389/fsufs.2023.1173158
Zhang, H., Chandio, A. A., Yang, F., Tang, Y., Ankrah Twumasi, M., and Sargani, G. R. (2022b). Modeling the impact of climatological factors and technological revolution on soybean yield: evidence from 13-major provinces of China. Int. J. Environ. Res. Public Health 19:5708. doi: 10.3390/ijerph19095708
Zhang, F., Sarkar, A., and Wang, H. (2021). Does internet and information technology help farmers to maximize profit: a cross-sectional study of apple farmers in Shandong, China. Land 10:390. doi: 10.3390/land10040390
Zhang, H., Tang, Y., Chandio, A. A., Sargani, G. R., and Ankrah Twumasi, M. (2022a). Measuring the effects of climate change on wheat production: evidence from northern China. Int. J. Environ. Res. Public Health 19:12341. doi: 10.3390/ijerph191912341
Zhang, Y., Wang, L., and Duan, Y. (2016). Agricultural information dissemination using ICTs: a review and analysis of information dissemination models in China. Inf. Proces. Agric. 3, 17–29. doi: 10.1016/j.inpa.2015.11.002
Zheng, H., Ma, W., Wang, F., and Li, G. (2021). Does internet use improve technical efficiency of banana production in China? Evidence from a selectivity-corrected analysis. Food Policy 102:102044. doi: 10.1016/j.foodpol.2021.102044
Keywords: internet technology, Mobile phone, food production, food security, emerging economies
Citation: Chandio AA, Alnafissa M, Awan A, Haouas I and Doganalp N (2025) Developing pathways for modern and sustainable agriculture in emerging economies: investigating the impact of information and communication technology adoption on food security. Front. Sustain. Food Syst. 9:1496295. doi: 10.3389/fsufs.2025.1496295
Received: 14 September 2024; Accepted: 18 February 2025;
Published: 11 March 2025.
Edited by:
Eleni Zafeiriou, Democritus University of Thrace, GreeceReviewed by:
Francesco Bozzo, University of Bari Aldo Moro, ItalyCopyright © 2025 Chandio, Alnafissa, Awan, Haouas and Doganalp. This is an open-access article distributed under the terms of the Creative Commons Attribution License (CC BY). The use, distribution or reproduction in other forums is permitted, provided the original author(s) and the copyright owner(s) are credited and that the original publication in this journal is cited, in accordance with accepted academic practice. No use, distribution or reproduction is permitted which does not comply with these terms.
*Correspondence: Abbas Ali Chandio, YWxpY2hhbmRpb0BnenUuZWR1LmNu
Disclaimer: All claims expressed in this article are solely those of the authors and do not necessarily represent those of their affiliated organizations, or those of the publisher, the editors and the reviewers. Any product that may be evaluated in this article or claim that may be made by its manufacturer is not guaranteed or endorsed by the publisher.
Research integrity at Frontiers
Learn more about the work of our research integrity team to safeguard the quality of each article we publish.