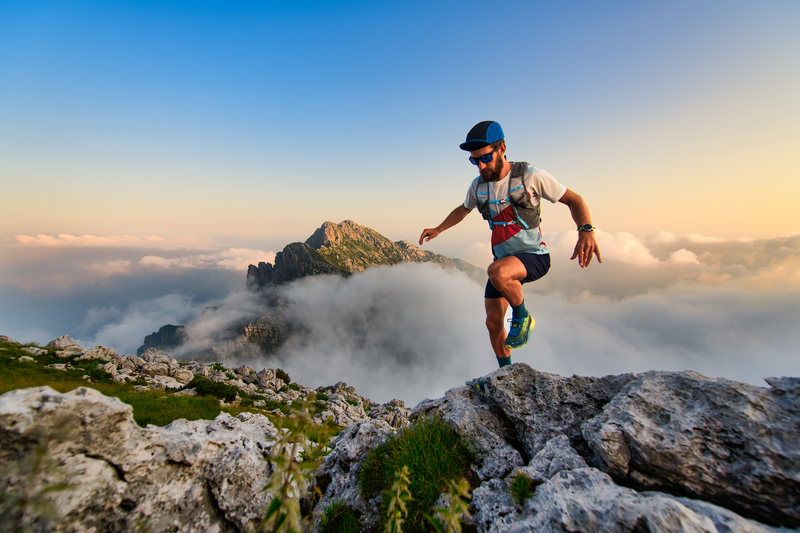
94% of researchers rate our articles as excellent or good
Learn more about the work of our research integrity team to safeguard the quality of each article we publish.
Find out more
ORIGINAL RESEARCH article
Front. Sustain. Food Syst.
Sec. Agricultural and Food Economics
Volume 9 - 2025 | doi: 10.3389/fsufs.2025.1494247
The final, formatted version of the article will be published soon.
You have multiple emails registered with Frontiers:
Please enter your email address:
If you already have an account, please login
You don't have a Frontiers account ? You can register here
This study explores the mechanism by which the digital economy can promote the integrated development of urban and rural areas by optimizing the allocation of factors, with the aim of revealing the positive role of the development of the digital economy in narrowing the gap between urban and rural areas. Based on panel data from 30 provinces in China from 2011 to 2022, this paper constructs measurement indicators for the level of development of the digital economy and the level of integrated development of urban and rural areas, and analyzes the spatial evolution characteristics of the two. Using a two-way fixed-effects model, a mediating effect model and a spatial Durbin model, this study systematically examines the impact of the digital economy on urban-rural integration, with a particular focus on the mediating role of factor allocation.The study finds that the digital economy has a dual effect in promoting urban-rural integration: on the one hand, it directly promotes the process of urban-rural integration; on the other hand, it indirectly promotes urban-rural integration by optimizing the allocation of factors such as labor, capital, land, technology and information. However, this promoting effect has a significant threshold effect and spatial heterogeneity.Based on these findings, this study proposes that the mobility of urban and rural resources should be enhanced by accelerating the development of the digital economy and optimizing the allocation of factors, thereby accelerating the process of urban-rural integration. These conclusions provide a valuable reference for policymakers around the world seeking to achieve coordinated urban-rural development and sustainable development.
Keywords: digital economy, Resource Allocation, Urban-rural integration, mediating effects, Spatial spillovers
Received: 10 Sep 2024; Accepted: 14 Feb 2025.
Copyright: © 2025 Lu, Zhuang, Li and Kong. This is an open-access article distributed under the terms of the Creative Commons Attribution License (CC BY). The use, distribution or reproduction in other forums is permitted, provided the original author(s) or licensor are credited and that the original publication in this journal is cited, in accordance with accepted academic practice. No use, distribution or reproduction is permitted which does not comply with these terms.
* Correspondence:
YuChen Lu, Beijing Normal University, Beijing, China
Jiakun Zhuang, Institute of Economic System and Management, Academy of Macroeconomic Research, Beijing, China
Lu Li, Beijing Normal University, Beijing, China
Mei Kong, China Institute Of Education And Social Development, Beijing Normal University, Beijing, China
Disclaimer: All claims expressed in this article are solely those of the authors and do not necessarily represent those of their affiliated organizations, or those of the publisher, the editors and the reviewers. Any product that may be evaluated in this article or claim that may be made by its manufacturer is not guaranteed or endorsed by the publisher.
Research integrity at Frontiers
Learn more about the work of our research integrity team to safeguard the quality of each article we publish.