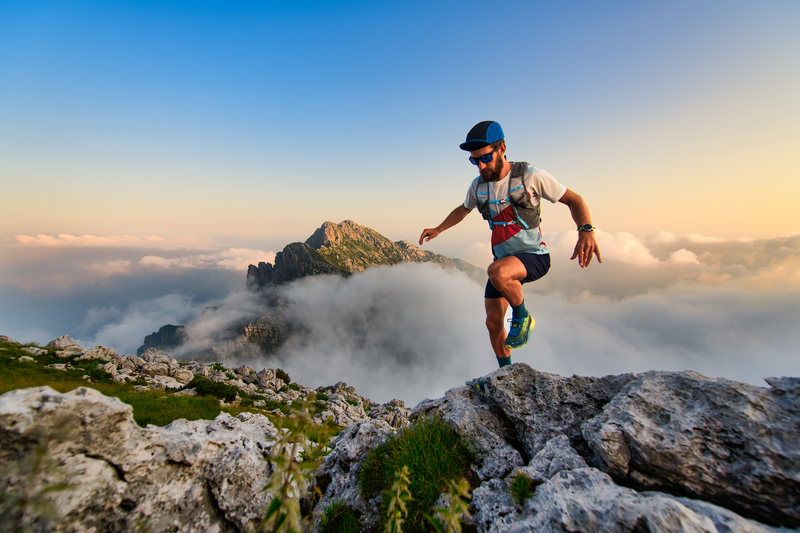
94% of researchers rate our articles as excellent or good
Learn more about the work of our research integrity team to safeguard the quality of each article we publish.
Find out more
CONCEPTUAL ANALYSIS article
Front. Sustain. Food Syst. , 27 January 2025
Sec. Agricultural and Food Economics
Volume 9 - 2025 | https://doi.org/10.3389/fsufs.2025.1491973
The dairy farming industry is an important contributor to both South Africa’s economy and food security. However, dairy in South Africa, like elsewhere in the world, has come under scrutiny because of animal welfare concerns and contributions to greenhouse gas (GHG) emissions. To address GHG mitigation, we have constructed a farm-level system dynamics model to assess both the emissions, and capture and storage of carbon (C) on farms, to determine whether farms are net emitters of C (i.e., sources) or sequestrators (i.e., sinks). We have considered nutrient flows, the type and volume of feed, the effluent management system, various parameters relating to the herd dynamics, and the overall effect on the farm economy. The resulting online Dairy Environment Sustainability Tool (DESTiny) can help dairy farmers adopt sustainable practices, as well as improve competitiveness and financial sustainability while reducing the farm’s emissions profile, thereby building value-chain and consumer trust. DESTiny can be considered a science-informed evidence-based tool for estimating, monitoring and understanding nutrient and C flows in dairy production systems. It is also a web-based tool (see https://assetresearch.org.za/destiny-tool/) with a user-interface which allows remote users, researchers, practitioners, farmers and technicians ease of access while integrating the system dynamics models with on-farm realities.
The dairy industry plays an important role in the economy of any country, and South Africa is no exception. It also contributes significantly towards food security (Meissner et al., 2023). When evaluating the financial and environmental sustainability of the agricultural industry, several complex factors must be taken into account, including the cost of inputs, market fluctuations that can affect revenue streams, soil health and its impact on long-term productivity, and the adoption of sustainability practices. Given the broad spectrum of these intricate, interrelated issues, both on- and off-farm, a comprehensive tool that estimates, monitors and clarifies the financial, environmental and production-related aspects of dairy farming would be invaluable in helping stakeholders make informed decisions that promote sustainability across the industry. This concept relates to the integrated farming systems described by Shanmugam et al. (2024) which combine crop and livestock production to enhance resource efficiency and sustainability by utilising manure as fertilizer and crop residues as animal feed. At farm-level, such a tool should include nutrient and organic carbon flows, feed and fodder utilisation, the cost, efficiency and profitability of the operation, as well as waste and effluent generation. Information obtained from the tool will allow appropriate on-farm management and guide constructive policy formulation.
Utilising this tool could assist the producer and, where applicable, other industry stakeholders to estimate a dairy farm’s net on-farm carbon footprint, as part of the value-chain, when considering both emissions and sinks. Calculating the net difference between the sources and sinks, as opposed to only calculating the emissions, holds significant implications for environmental sustainability and economic viability within the industry.
Dairy farmers face significant challenges in understanding and managing their farms’ carbon footprints, which directly impacts their ability to implement sustainable practices and enhance their competitiveness in an increasingly environmentally-conscious market. Without a comprehensive tool to provide insights into carbon emissions, farmers may struggle to mitigate environmental risks effectively, leading to potential regulatory penalties and loss of consumer trust. This situation highlights the urgent need for a tool, which can empower dairy farmers to assess their environmental impact while simultaneously improving profitability. By harmonising sustainability with financial performance, this tool should foster value-chain integrity and strengthen consumer confidence, ultimately bolstering the long-term resilience of the dairy industry (Blignaut et al., 2022; Reinecke et al., 2024).
Therefore, the aim of this paper is to present the development of the Dairy Environment Sustainability Tool (DESTiny), an innovative online platform designed for farm-level application, accessible at: https://assetresearch.org.za/destiny-tool/. This tool is underpinned by a specialised system dynamics model that addresses the intricate dynamics of dairy production prior to the farm gate, facilitating enhanced sustainability practices within the dairy industry. The development of such tools is critical as they can help address pressing issues such as climate change impacts on milk production (Milk, 2024) and support rural development through improved economic opportunities (DAFF, 2015; WWF South Africa, 2023).
In the process of milk production, carbon follows a dynamic flux with some of it being stored and some continuously circulating within the system without being emitted into the atmosphere. Various carbon pathways are possible within the dairy production environment. Lean and Moate (2021) refer to cows as biogenic carbon sources. This is since cows, all ruminants and other terrestrial animals for that matter, are only involved in natural above-ground and soil-based carbon cycles. Biogenic carbon is stored in, sequestered by, and released through organic material. This process mainly results through processes such as photosynthesis. Also, when plants decompose the organic carbon can be captured by soil. Animals, and notably within the context of this study, dairy cows, are not part of the fossil carbon cycle that involves the release of carbon from sequestrated carbon in fossil fuels, and which impacts atmospheric CO2 concentrations (Meissner et al., 2023).
Rumination, a digestive process in ruminant animals, facilitates the breakdown of complex plant nutrients and results in the emission of methane (CH4), a potent greenhouse gas (GHG). While CH4 is naturally mitigated by methanotrophic microorganisms in the soil, it is also oxidised in the atmosphere, converting to CO2 through hydroxyl oxidation. This conversion plays a crucial role in enhancing plant productivity via photosynthesis, thereby contributing to the carbon sequestration cycle (IPCC, 2013). The biogenic carbon cycle is characterised by its relatively short duration; CH4 typically takes approximately 60 days to reach the stratosphere. Once there, however, it can persist for about 13 years before being oxidised. This lifespan is significantly shorter than that of CO2 emitted from fossil fuel combustion, which has long-term climatic implications (Lynch et al., 2020). The dynamic interplay between CH4 emissions and carbon sequestration underscores the complexity of carbon movement within animal–plant systems, particularly in dairy production. In addition to CH4 emissions, nitrous oxide (N2O)—primarily resulting from synthetic fertilisers and animal manure—must also be considered within the context of dairy farming systems (IPCC, 2019). A critical question arises: when accounting for the differing global warming potentials of CH4, N2O, and CO2, do on-farm GHG emissions exceed CO2 sequestration? Or does sequestration play a significant role in mitigating overall emissions? Addressing this question is essential for developing effective strategies to manage GHG emissions in agricultural systems. Consequently, it is possible through changes in management to affect the net position and change a dairy operation from a net emitter to a net sink. Salvador et al. (2017) showed that soil carbon sequestration on pasture-based systems can be a substantial sink to mitigate GHG emissions. The DESTiny model, therefore, has been developed to calculate the on-farm difference between the sources and sinks of GHG.
An overview of the sources and sinks of carbon captured by the DESTiny model are highlighted in Table 1. It is important to note that these sources and sinks are subject to various on-farm management decisions and protocols that can impact on the level of each. These sources can either increase or decrease net GHG, resulting in farms that are either carbon-emitting or carbon-capturing. The primary factors contributing to emissions can have a mitigating effect on overall GHG output, contingent upon their management and application, thereby facilitating a reduction in total emissions.
The results of the model are disaggregated in such a manner that they provide a systems-view of the entire farm as a production unit while considering all the major factors needed to accurately calculate emissions and carbon storage. By doing so, producers, consumers and the industry stakeholders can have a thorough understanding of how carbon flows through the respective farms, calculate the net GHG emissions, and measure the economic stability of the operations.
Dairy production systems are multi-faceted involving various factors such as the geographical location, animal performance indicators, feeding system, management, market, turnover and profitability. The sustainability of these systems is influenced by factors such as climate, fodder production and the cost thereof, milk yield and the efficiency of milk production, and the integrity of natural resources. In this context, we clarify that while emissions from fuel consumption, electricity use for irrigation pumps, and tillage operations are included in our tool’s calculations, however, embedded emissions from machinery and buildings are not currently accounted for. To analyse and optimise these factors, a system dynamics model approach has been deployed. We used the Vensim DSS software (Vensim, 2024)1 to develop the model and the Forio simulation interface for the web-bed version.2
Vensim allows for analysing the interactions between the diverse variables involved in dairy farming systems to capture the net carbon flow through the system. Vensim, and system dynamics in general, has been successfully used in various similar analyses, such as the study by Blignaut et al. (2022) that highlights the importance of considering the complex interactions between various factors in agricultural systems, and the potential for system dynamics modelling to provide insights into the behaviour of these systems over time. System dynamics modelling for dairy herd management have been used before for a heat-detection study to enhance fixed-term artificial insemination (Davila et al., 2022). A model for developing sustainable land reformation based on laying hens, highlighted the influence of factors such as carrying capacity, egg prices and farmer income (Yuzaria et al., 2022). Lastly, a study on milk production in an industrial ranch used system dynamics to identify and optimise variables, including production cycle rate and medical costs (Shahsavari-Pour et al., 2023). These studies collectively demonstrate the potential of system dynamics in addressing complex challenges and improving sustainability in livestock production.
Figure 1 is a high-level illustration of the DESTiny model where each of the sub-models is indicated by a different colour.
Figure 1. Causal loop diagram showing the flow of sources and sinks within a dairy farm system. Red sub-model: belowground; Green sub-model: aboveground; Purple sub-model: animal model; Blue sub-model: within-product; Orange sub-model: external sources.
The red sub-model (see Supplementary material) pertains to the belowground component and involves main soil management components such as soil type, the nutrient availability and soil health. Some of the input data require soil analysis, such as soil organic carbon. The different soil types and their associated carbon content also require input from soil analysis. Total carbon in soil can then be calculated using these inputs and the different soil bulk densities, depending on soil type. The different tillage practices used in DESTiny are shown in Table 2 and include categories for no-tillage, reduced tillage, conventional tillage and intensive tillage, which is based on their impact on GHG emissions (Liu et al., 2023). Emission factors for the different tillage practices were adopted from Liu et al. (2023) and NRCS (2023), which provided insights into the use of different emission factors for dairy pasture production systems. They highlighted the significance of emission factors and emission intensity in understanding GHG emissions across various farming systems.
Choice of fertiliser type with its specific nitrogen content within the different fertilisers, as well as the amount added to the soil are accounted for in this section, although synthetic fertiliser bought forms part of the external sources and therefore the manufacturing is included as part of the orange arrows. The equation of Smit et al. (2020) was used to calculate N2O emissions and its associated emission factors from intensive pasture-based dairy systems in South Africa (Equation 1).
Where,
N = nitrogen.
N2O = nitrous oxide.
Fertiliser applications connect this sub-model to the pasture sub-model since the requirements for plant growth depend on the type of pasture and pasture yield, which can either indicate compromised plant growth or excess nitrogen in the soil that might add to nitrogen leaching, depending on the management system. Where urine and manure are integrated into the soil they have been connected to the animal sub-model as part of the nutrient availability from animal origin for plant growth. Other external factors related to this model are discussed in the orange sub-model.
The green highlighted sub-model (see Supplementary Figure S2) deals with the aboveground component, which interconnects with the animal and belowground sub-models. This sub-model provides nutrients to the animals while taking nutrients from the soil. Additionally, it contributes to the soil nutrients through plant litter. The pasture sub-model is designed to assess the quality of the available forages. It breaks down the feed production area into irrigated and dryland pasture, as well as crop and fodder production. The sub-model calculates different yields for each pasture type at the farm level, utilising existing data to determine quality and nutritional values. It does this by using the pasture production, yield and nutritional composition to calculate the weighted average for each type of planted forage with its nutritional content, which are model-derived. It further evaluates the total nutrient fractions in the pasture and their digestibility, incorporating these into farm-based weighted averages. Additionally, the offered feed and how it is allocated from a farm management perspective, are then included in the animal sub-model as part of the total diet.
Planting legumes in grass pastures can play a significant role in lowering emissions (Galloway et al., 2024). This is because it increases nutrient variety available for animal consumption and has a positive impact on nitrogen fixation in the soil (Teixeira et al., 2019). The carbon and nitrogen flux within the pasture/aboveground sub-model involves the uptake of atmospheric carbon dioxide (CO2) by plants during photosynthesis. A portion of the captured carbon is stored in the biomass of grasses and other vegetation. The carbon storage in predominantly grass pasture was calculated using the IPCC (2006) default values of 30% of the biomass. For legume or forage herb pastures, the default IPCC value was 40%. Moreover, the calculations also include the rotations per year on the pasture, which are critical for understanding the dynamics of carbon and nitrogen cycling. Rotational grazing systems, where pastures are managed through periodic rest and grazing, can enhance soil health and improve nutrient cycling. This practice not only contributes to increased biomass production but also optimises carbon sequestration by allowing legumes to thrive, thereby enhancing their nitrogen-fixing capabilities. Research indicates that such rotational systems can lead to more efficient use of resources, resulting in lower greenhouse gas emissions per unit of livestock product (Augustine et al., 2023). Thus, integrating rotations into pasture management strategies is essential for maximising both productivity and environmental sustainability.
However, respiration and decomposition of plant litter play crucial roles in the cycling of carbon and nitrogen. During respiration, plants and microorganisms consume stored carbohydrates, releasing CO2 back into the atmosphere. This respiratory loss of carbon is a natural process that contributes to the carbon balance. Additionally, as plant litter decomposes, it undergoes microbial decomposition leading to the release of carbon as CO2 and affecting nitrogen dynamics in the system. Both photosynthesis and respiration are calculated in the model, incorporating default values of 50 and 30% for respiration and decomposition, respectively. These outcomes are then multiplied by the molecular weights of CO2 and N2O, and N2O is then multiplied by its global warming potential (GWP). By optimising the nutrient uptake and ensuring adequate photosynthesis, the yield of the pasture can be increased, subsequently reducing the net GHG emissions.
The purple sub-model (see Supplementary material S3) refers to the animal sub-model and differentiates between emissions from fermentation and manure management for lactating and dry cows, heifers and calves. To provide a comprehensive understanding, it considers the animal’s metabolic processes, herd information, milk production and physiological status, all of which contribute to the underlying processes within the animal carbon pool. A crucial feature of this sub-model is dry matter intake (DMI), which plays a significant role in demonstrating the interrelatedness of the variables. The DMI is determined by the animal’s nutrient requirements, which are influenced by its physiological status and available nutrient fractions from the feed. The estimation of DMI for dairy cows is a critical element in optimising feeding strategies and enhancing milk production efficiency. In this regard, Allen et al. (2019) proposed an approach to predict and manage DMI based on neutral detergent fibre (NDF) and NDF digestibility equations. Equation 2 outlines Allen et al.’s (2019) methodology, with particular emphasis on the characteristics of the feed, including NDF content and digestibility, to ensure that dairy cows receive adequate nutrition for optimal milk production and overall health. This approach is instrumental in ensuring that dairy cows receive optimal nutrition, which is essential for maximising milk production and maintaining their health and wellbeing.
Where,
DMI = dry matter intake (kgd−1).
NDF = neutral detergent fibre (% of DM).
fNDF = forage NDF (% of DM).
ADF = acid detergent fibre (% of DM).
fNDFD = forage NDF digestibility (%).
MY = milk yield (kgd−1).
Milk yield and NDF in the feed are related to the productivity of cows, while for heifers and calves, productivity is influenced by animal factors (Niu et al., 2018). The amount and quality of nutrient intake, as well as the physiological status of the animal, determine milk production and composition. The weighted average for all nutrient fractions, such as NDF, ADF, NDF digestibility and crude protein (CP) for all feeds are accounted for including feed from pastures, silage and all purchased feeds. Nutrient fractions from farm-produced feeds are obtained from the pasture sub-model, hence the different feed qualities will impact emission results. Any nutrients that are not used for milk production, growth or animal requirements including maintenance, reproduction and pregnancy, will be excreted in the form of manure and urine (Appuhamy et al., 2016) as shown in Equation 3 or result in CH4 from fermentation (Dong et al., 2022) as shown in Equation 4.
Where,
DMI = dry matter intake (kgd−1).
HC = NDF-ADF.
NDF = neutral detergent fibre (% of DM)
ADF = acid detergent fibre, % of DM.
CP = crude protein (% of DM).
Where,
CH4 = methane (MJ day−1).
DMI = dry matter intake (kgd−1).
NDF = neutral detergent fibre (% of DM).
To calculate the CH4 from enteric fermentation for heifers, the following Equation 5 in the IPCC (2019) was used:
Where,
CH4 = methane (MJ day-1)
GE = gross energy (MJ kg-1)
Ym = CH4 yielding potential (% of GE intake)
The term Ym, or CH4 yielding potential, indicates the maximum CH4 produced from a substrate as a percentage of gross energy (GE) intake. According to the IPCC (2006) guidelines, particularly Tables 10.12 and 10.13. Ym values are based on empirical data regarding the digestibility and fermentability of various feedstuffs, which can differ significantly. Ym values are crucial for estimating enteric CH4 emissions from ruminants, as they reflect the efficiency of feed conversion into CH4.
One mitigation strategy is through feeding feed additives, such as 3-nitrooxypropanol (3-NOP) and nitrate. In their life cycle analysis study, Uddin et al. (2022) confirmed that 3-NOP was more effective in decreasing CH4 emissions, at 31% average reduction, as opposed to the reduction potential of nitrate at 12%. In another study, Van Wyngaard et al. (2018) found that nitrate effectively lowered CH4 emissions, but DMI from concentrate feed and total milk production were compromised. Hence, in the DESTiny model the reduction in enteric CH4 due to 3-NOP supplementation was calculated according to the equations described by Uddin et al. (2022), as illustrated in Equations 6, 7:
Where,
CH4 = methane reduction (% of total CH4)
3-NOP = 3-nitrooxypropanol (g day−1)
NDF = neutral detergent fibre (% DM)
With more research and development of calculations for specific feed additives, their effects on enteric fermentations can be added into this section. However, caution with these inclusions should be considered, especially weighing up the possible trade-offs with higher feed intake, effects on milk production or when feed efficiencies can be compromised. By subtracting the total effect of these additives from the enteric emissions, a net fermentation CH4 can be generated within this sub-model. Similarly, except for different formulae used, the net enteric emissions for heifers and calves are calculated and by adding all of these, and a net CH4 emission from enteric fermentation can be generated.
A supporting strategy to mitigate fermentation emissions can be to add these feed additives to increase feed efficiency (Feng and Kebreab, 2020), thereby further resulting in lower fermentation emissions. This is also included in the DESTiny tool. Examples of reduction by adding nitrate to the concentrates are shown in Equations 8, 9.
Where,
CH4 = methane (% of total CH4)
DMI = dry matter intake (kgd−1)
External feed sources form part of this section due to their importance in animal emissions as well as being part of the fulfilment in nutrient requirements. However, they are also accounted for as part of the external sources in the orange sub-model.
Urine nitrogen excretions will increase with higher protein, and the impact of manure will depend on the manure handling system (IPCC, 2019). To estimate the amount of volatile solids in manure, we used the DMI and specific emission factors for different manure management systems. This allowed us to calculate the CH4 emissions from the manure (Equations 10–13). Similarly, nitrogen emissions from the manure and urine were calculated based on nitrogen intake (NI), which results in volatilisation and leaching (Johnson et al., 2016).
Where,
N = Nitrogen
NI = Nitrogen intake (g d-J)
The applicable fractions of each animal group per manure system are to be completed from farm information. These emissions will be different for each system and will indirectly either contribute to total emissions or lower emissions from manure. Emissions from manure handling can be reduced with biodigesters or incorporating manure into the soil (IPCC, 2019).
The blue section in Figure 1 represents carbon in the final product. The virtual carbon and nitrogen in the milk are derived from the milk solids and related to the milk yield, which is shown as emission reduction in green circles in Figure 1. The carbon and nitrogen are multiplied by the relevant molecular masses and then converted into CO2eq. To calculate the number of carbon atoms in milk, the molecular formula of the major components of milk was considered. Milk is composed of various molecules, including proteins, fats, carbohydrates and other minor components. The exact composition can vary, but the molecular formulas of some of the major components and how to calculate the carbon atoms in each were included in the model. These components were lactose, the casein fraction in the milk protein, and triglycerides in the milk fat. To calculate the total number of carbon atoms in milk, the proportion of each component present in the milk were accounted for according to their atomic weight and density, hence accounting for the carbon atoms, and added together accordingly.
The orange arrows (Figure 1) serve as the input on the farm associated with direct carbon emissions and correspond to specific emission factors. Emissions from pasture production include emissions from seeds, pesticides, herbicides, machine operation, fertiliser manufacturing and irrigation (Wang et al., 2015; EMEP/EEA Database, 2019; Siegl et al., 2023). The computation of emission factors for herbicides, pesticides and pasture chemicals holds immense significance in comprehending their environmental impact. This entails a meticulous assessment of energy intensities and active ingredients, which are fundamental determinants of the energy requirements for their production. The model takes into account the energy per unit of active ingredient, inclusive of formulation, packaging and transport energy, and as such estimate the aggregate energy input associated with the production of these chemicals (EMEP/EEA Database, 2019; and emission factor described by Wang et al., 2015). The derivation of emission factors that reflect the environmental footprint of herbicides, pesticides and pasture chemicals in terms of GHG emissions necessitates an all-encompassing analysis of the energy intensities and active ingredients of these chemicals. The different emission factors used in the DESTiny model are summarised in Table 3.
It is important to take into account the various factors that contribute to emissions when evaluating a system or process. Among these factors, direct emissions can arise from the utilisation of electricity and fuel, as well as from the use of external feed sources such as concentrates and roughages (Jiménez et al., 2021). By including each of these, it becomes possible to gain a more comprehensive understanding of the overall level of emissions associated with a dairy operation and adapt to more sustainable practices. These default emission factors included in the DESTiny are illustrated in Table 4.
The concept of the DESTiny tool demonstrates important potential as a comprehensive model for assessing the carbon footprint of dairy farms, with its focus on the biogenic carbon cycle and its ability to incorporate formulae from new research, such as the effect of different feedstuffs (Reinecke et al., 2024). However, further data is required to compare its performance to alternative tools and ensure its accuracy and reliability before widespread adoption (Rotz et al., 2010; Pirlo et al., 2014). The growing interest in sustainability presents an opportunity for the tool, but it will need to overcome competition and potential resistance to new technologies (Oyinbo and Hansson, 2024).
The DESTiny tool can also leverage insights from life cycle assessments (LCA) that have been conducted on various dairy farming systems, which highlight the complexities of carbon emissions and the importance of considering both direct and indirect sources (Carvalho et al., 2021; Mazzetto et al., 2022). By integrating these methodologies, DESTiny can provide a more nuanced understanding of how different management practices impact carbon footprints at both the farm level and system-wide interactions (WWF South Africa, 2023). This comprehensive approach will not only enhance the tool’s credibility but also facilitate its acceptance among stakeholders in the dairy industry.
Table 5 provides a summary of the quantitative evaluation of the DESTiny model, highlighting its strengths, weaknesses and opportunities. This evaluation underscores the tool’s potential to support farmers in their efforts to optimise their outcomes while minimising environmental impact.
Farmers face significant challenges in optimising their outcomes, including the complexity of the farming system and the uncertainty of decision outcomes. To overcome these challenges, farmers can leverage scenario analysis, a robust approach that enables them to explore trade-offs, test hypotheses and identify effective strategies across multiple scenarios. By doing so, farmers can make more informed and effective decisions, mitigate risks and maximise desired outcomes in complex systems. Scenario analysis empowers farmers to optimise their actions by gaining insights into the system’s dynamics and making informed decisions.
By changing the input parameters of the tool, one can perform an analysis of various runs and their outcomes. For instance, when the number of livestock units on a farm increases, the overall requirements also increase. This phenomenon can occur due to a higher fertility rate which results in more calves and, in turn, creates a positive feedback loop. In addition, the livestock units can increase through the acquisition of new animals or by replacing deceased animals with new ones over time. Once the desired maximum animal numbers are reached, surplus animals can be sold to balance the herd size. However, it is crucial to consider mortalities as they have a negative impact on the number of livestock units.
The DESTiny model is developed to present results in terms of C emissions, C captured in the system and Net GHG, in CO2eq. The emission results provide a detailed analysis of the primary sources of emissions on the farm, along with their respective contributions towards the total emissions. The model can also simulate the system’s behaviour over time, taking into account feedback loops, delays and non-linear relationships between variables.
By studying the results, one can identify the larger sources of emissions and determine the best approach to reduce them efficiently. This analysis will help in prioritising the implementation of emission control measures, thereby leading to a more sustainable and environmentally-friendly farming operation. In addition, the outcomes of capturing and sequestering C in the system have been computed and presented on the results page for a more comprehensive analysis. Furthermore, the model includes detailed information on the performance of individual animals, reporting emissions and productivity metrics per kilogram of fat and protein corrected milk as well as per hectare of land. It allows farmers to simulate various scenarios and analyse their impact on decision-making.
Consequently, the net GHG emissions are presented as the balance from both the emissions of CH4 from cow digestion and manure management, as well as the capture of carbon through pasture growth, soil sequestration and other C sinks. Achieving a negative net GHG balance indicates that the system sequesters more GHGs than it emits, thereby contributing to a reduction in atmospheric GHG concentrations and promoting environmental sustainability. This outcome is a direct consequence of both the emissions and the capturing of C within the system.
By leveraging the tool’s capabilities, farmers can gain a deeper understanding of the ecological impact of their farming systems and optimise sustainability efforts. This integrated approach enables farmers to ensure that their decisions are both environmentally responsible and economically feasible. For instance, the implementation of mixed pastures can initially incur higher costs compared to monoculture grass species. However, the increased yield and reduced nitrogen fertiliser requirements can lead to significant long-term financial benefits. This aligns with the notion that future studies should focus on developing frameworks to assess the interactions between various farm elements, which will further enhance our understanding of their collective impact on sustainability (FAO, 2019).
Sustainable intensification has been proposed as a strategy to achieve better environmental responsibility and be economically feasible. Additional strategies involve carbon sequestration in soil and pasture-based systems, as well as alternative management practices like manure separation and switching to renewable energy sources to reduce emissions. Measuring the carbon capturing and storage capacity within different dairy production systems is critical to drive sustainable on-farm dairy practices. Calculating the total inflow and outflow of carbon and nitrogen across the farm and its subsystems is crucial, as diverse management strategies can affect various indicators, leading to some variables acting as a source or sink.
Another effect can be understanding that the dynamics of nitrogen on a dairy farm is crucial for optimising environmental and economic outcomes. It assesses carbon and nitrogen flux within the pasture/aboveground sub-model by integrating photosynthesis, respiration and decomposition values. For example, it provides detailed metrics on N excretion rates, N content in urine and manure per cow per day, and N use efficiency for milk production. This information enables farmers to make informed decisions to improve N management. Furthermore, the critical role of different management practices between farms will impact whether a farm functions as a carbon sink or source.
A new tool has been developed to help dairy farmers estimate, monitor and understand the nutrient and carbon flows involved in milk production. Furthermore, the concept of this tool can promote sustainable practices, enhance competitiveness and minimise potential environmental risks. The impact of a dairy farm on the environment greatly depends on the management practices employed. In order to tackle the challenges of climate change, it is important to implement specific management strategies that promote carbon sequestration. By integrating these practices into the DESTiny tool, farmers can optimise their operations to minimise greenhouse gas emissions while maximising carbon sequestration, ultimately contributing to a more sustainable and environmentally friendly dairy industry.
The DESTiny tool is a comprehensive solution designed to help dairy farmers make informed decisions regarding their farm management practices. It provides valuable insights that empower farmers to reduce their environmental impact, improve their economic performance, and ensure their long-term sustainability. Through its data-driven approach, DESTiny enables farmers to optimise their operations by giving them a complete overview of their farming practices, thereby allowing them to make informed decisions about resource utilisation, which can significantly enhance their output and profitability. Its ability to provide insights into the environmental impact of farming practices makes DESTiny an indispensable resource for farmers seeking to adopt sustainable practices. Overall, DESTiny is a critical tool for dairy farmers aiming to boost productivity, minimise their environmental impact, and ensure their long-term sustainability.
RR: Conceptualization, Investigation, Writing – original draft. JB: Conceptualization, Funding acquisition, Supervision, Validation, Visualization, Writing – review & editing. HM: Funding acquisition, Project administration, Writing – review & editing. PS: Conceptualization, Supervision, Validation, Visualization, Writing – review & editing.
The author(s) declare that financial support was received for the research, authorship, and/or publication of this article. The research team gratefully acknowledges research funding received from MilkSA (project number 0331-2022), as implemented by ASSET Research.
The research team gratefully acknowledges the research funding received from MilkSA. The administrative support of L vd Elst is also acknowledged.
The authors declare that the research was conducted in the absence of any commercial or financial relationships that could be construed as a potential conflict of interest.
All claims expressed in this article are solely those of the authors and do not necessarily represent those of their affiliated organizations, or those of the publisher, the editors and the reviewers. Any product that may be evaluated in this article, or claim that may be made by its manufacturer, is not guaranteed or endorsed by the publisher.
The Supplementary material for this article can be found online at: https://www.frontiersin.org/articles/10.3389/fsufs.2025.1491973/full#supplementary-material
Allen, M. S., Sousa, D. O., and VandeHaar, M. J. (2019). Equation to predict feed intake response by lactating cows to factors related to the filling effect of rations. J. Dairy Sci. 102, 7961–7969. doi: 10.3168/jds.2018-16166
Appuhamy, J. A. D. R. N., France, J., and Kebreab, E. (2016). Models for predicting enteric methane emissions from dairy cows in North America, Europe, and Australia and New Zealand. Glob. Chang. Biol. 22, 3039–3056. doi: 10.1111/gcb.13339
Augustine, D. J., Kearney, S. P., Raynor, E. J., Porensky, L. M., and Derner, J. D. (2023). Adaptive multi-paddock grazing management alters foraging behavior and spatial grazing distribution of free-ranging cattle. Agric. Ecosyst. Environ. 339:108164. doi: 10.1016/j.agee.2023.108164
Blignaut, J. N., Meissner, H., Smith, H., and Du Toit, L. (2022). An integrative bio-physical approach to determine the greenhouse gas emissions and carbon sinks of a cow and her offspring in a beef cattle operation: a system dynamics approach. Agric. Syst. 195:103286. doi: 10.1016/j.agsy.2021.103286
Carvalho, L. S., Willers, C. D., Soares, B. B., Nogueira, A. R., Neto, A., and Rodrigues, L. B. (2021). Environmental life cycle assessment of cow milk in a conventional semi-intensive Brazilian production system. Environ. Sci. Pollution Res. Int. 29:21259. doi: 10.1007/s11356-021-17317-5
Davila, D. J. H., Ceballos, Y. F., and Sanchez Torres, G. (2022). A system dynamics approach for dairy herd modeling considering fixed-term artificial insemination scenario. Inv. Innov. Ingenierías. 10, 93–105. doi: 10.17081/invinno.10.2.5283
DAFF (2015). Dairy market value chain profile 2021. Gauteng: Department of Agriculture, Forestry and Fisheries, South Africa.
Dong, L., Peng, J. I. A., Li, B., Wang, B., Yang, C., Liu, Z., et al. (2022). Quantification and prediction of enteric methane emissions from Chinese lactating Holstein dairy cows fed diets with different dietary neutral detergent fiber/non-fibrous carbohydrate (NDF/NFC) ratios. J. Int. Agri. 21, 797–811. doi: 10.1016/S2095-3119(21)63825-X
EMEP/EEA. (2020). EMEP/EEA air pollutant emission inventory guidebook 2020: technical guidance to prepare national emission inventories. European Environment Agency. Available at: https://www.eea.europa.eu/publications/emep-eea-guidebook-2020 (Accessed April 14, 2024).
EMEP/EEA Database. (2019). EMEP/EEA air pollutant emission inventory guidebook 2019. Technical guidance to prepare national emission inventories. Available at: https://www.eea.europa.eu/publications/emep-eea-guidebook-2019 (Accessed April 14, 2024).
Eskom (2022). Department of Energy or the National Energy Regulator of South Africa (NERSA). Available at: https://www.energy.gov.za/files/media/explained/2022-South-African-Energy-Sector-Report.pdf (accessed July 22, 2024).
Food and Agriculture Organization of the United Nations. FAO. (2018). The state of food and agriculture 2018: Migration, agriculture and rural development. Rome. https://doi.org/10.4060/CA1584E
FAO (2019). The state of food and agriculture. Moving forward on food loss and waste reduction. Rome: FAO.
Feng, X., and Kebreab, E. (2020). Net reductions in greenhouse gas emissions from feed additive use in California dairy cattle. PLoS One 15:e0234289. doi: 10.1371/journal.pone.0234289
Galloway, J. N., Aber, J. D., Erisman, J. W., Seitzinger, S. P., Howarth, R. W., Cowling, E. B., et al. (2024). Planting legumes in grass pastures can play a significant role in lowering emissions. Glob. Chang. Biol. 26, 6959–6973. doi: 10.1111/gcb.15345
IPCC (2013). Climate change 2013: the physical science basis. Contribution of working group I to the fifth assessment report of the intergovernmental panel on climate change. Cambridge: Cambridge University Press.
IPCC. (2019). “Chapter 10: emissions from livestock and manure management,” in 2019 Refinement to the 2006 IPCC Guidelines for National Greenhouse Gas Inventories, Volume 4: Agriculture, Forestry and Other Land Use. Kanagawa: IPCC Task Force.
Jiménez, L. E., Hernández, A. X., Benaouda, M., Avalos, J. O., Corona, L., Castillo-Gallegos, E., et al. (2021). Concentrate supplementation on milk yield, methane and CO2 production in crossbred dairy cows grazing in tropical climate regions. J. Anim. Behav. Biometeorol. 9:2118. doi: 10.31893/jabb.21018
Johnson, A., Reed, K., and Kebreab, E. (2016). Short communication: evaluation of nitrogen excretion equations from cattle. J. Dairy Sci. 99, 7669–7678. doi: 10.3168/jds.2015-10730
Kornelius, G., Forbes, P., Fischer, T., and Govender, M. (2022). Tier 2 greenhouse gas emission factors for south African liquid and gaseous fuels. Clean Air J. 32:15226. doi: 10.17159/caj/2022/32/2.15226
Lean, I. J., and Moate, P. J. (2021). Cattle, climate and complexity: food security, quality and sustainability of the Australian cattle industries. Aust. Vet. J. 99, 293–308. doi: 10.1111/avj.13072
Liu, F., Chen, Z., Cai, G., Peng, X., and Wang, X. (2023). CO2 emissions from soils under different tillage practices and their correlation with weather conditions. Plan. Theory 13:3084. doi: 10.3390/plants13123084
Lynch, J., Cain, M., Pierrehumbert, R., and Allen, M. (2020). Demonstrating GWP: a means of reporting warming-equivalent emissions that captures the contrasting impacts of short- and long-lived climate pollutants. Environm. Res. Letters. 15:044023. doi: 10.1088/1748-9326/ab6d7e
Mazzetto, A. M., Falconer, S., and Ledgard, S. (2022). Mapping the carbon footprint of milk production from cattle: a systematic review. J. Dairy Sci. 105, 9713–9725. doi: 10.3168/jds.2022-22117
Meissner, H. H., Blignaut, J. N., Smith, H. J., and Du Toit, C. J. L. (2023). The broad-based eco-economic impact of beef and dairy production: a global review. SA J. Anim. Sci. 53, 250–275. doi: 10.4314/sajas.v53i2.11
Milk, S. A. (2024). Sustainability in the South African Dairy Industry: A Status and Progress Report. Available at: https://milksa.co.za/sites/default/files/MILK%20SA%20Sustainability%20in%20the%20SA%20dairy%20Industry%20A%20Status%20and%20Progress%20Report.pdf (Accessed August 17, 2024).
Niu, M., Kebreab, E., Hristov, A. N., Oh, J., Arndt, C., Bannink, A., et al. (2018). Prediction of enteric methane production, yield, and intensity in dairy cattle using an intercontinental database. Glob. Change Biol. 24, 3368–3389. doi: 10.1111/gcb.14094
NRCS (2023). Tillage intensity classification for greenhouse gas (GHG) emission estimations. Ithaca, NY: Agronomy Fact Sheet Series, Field Crops Extension, College of Agriculture and Life Sciences, Cornell University.
Oyinbo, O., and Hansson, H. (2024). Understanding dairy farmers’ trade-offs between environmental, social and economic sustainability attributes in feeding systems: The role of farmers’ identities. J. Agric. Econ. 75, 869–888. doi: 10.1111/1477-9552.12588
Pirlo, G., De Rosa, G., and Di Francia, A. (2014). Factors affecting life cycle assessment of milk produced on six Mediterranean buffalo farms. J. Dairy Sci. 97, 6583–6593. doi: 10.3168/jds.2014-8007
Reinecke, R., Blignaut, J. N., Meissner, H. H., and Swanepoel, P. A. (2024). Advancing carbon sequestration and nutrient management in the south African dairy industry for sustainable growth. Front. Sust. Food Syst. 8:1397305. doi: 10.3389/fsufs.2024.1397305
Rotz, C. A., Montes, F., and Chianese, D. S. (2010). The carbon footprint of dairy production systems through partial life cycle assessment. J. Dairy Sci. 93, 1266–1282.
Salvador, S., Corazzin, M., Romanzin, A., and Bovolenta, S. (2017). Greenhouse gas balance of mountain dairy farms as affected by grassland carbon sequestration. J. Environ. Manag. 196, 644–650. doi: 10.1016/j.jenvman.2017.03.052
Shahsavari-Pour, N., Rahimi-Ashjerdi, S., Heydari, A., and Fekih, A. (2023). A system dynamics approach to optimize Milk production in an industrial ranch [article]. Appl. Sci. 13:1662. doi: 10.3390/app13031662
Shanmugam, P. M., Sangeetha, S. P., Prabu, P. C., Varshini, S. V., Renukadevi, S. V., Ravisankar, A., et al. (2024). Crop–livestock-integrated farming system: a strategy to achieve synergy between agricultural production, nutritional security, and environmental sustainability. Front. Sustainable Food Systems. 8:1338299. doi: 10.3389/fsufs.2024.1338299
Siegl, S., Hagenbucher, S., Niggli, U., and Riedel, J. (2023). Addressing dairy industry’s scope 3 greenhouse gas emissions by efficiently managing farm carbon footprints. Environm. Chal. 11:100719. doi: 10.1016/j.envc.2023.100719
Smit, H. P. J., Reinsch, T., Swanepoel, P. A., Kluß, C., and Taube, F. (2020). Grazing under irrigation affects N2O-emissions substantially in South Africa. Atmosphere 11:925. doi: 10.3390/atmos11090925
Teixeira, R. F. M., Barão, L., Morais, T. G., and Domingos, T. (2019). “BalSim”: a carbon, nitrogen and greenhouse gas mass balance model for pastures. Sustainability 11:53. doi: 10.3390/su1101005
Uddin, M. E., Tricarico, J. M., and Kebreab, E. (2022). Supplementary materials-impact of nitrate and 3-nitrooxypropanol on the carbon footprint of milk from cattle produced in confined-feeding systems across regions in the United States: a life cycle analysis. Mendelay Data V1, 1–15. doi: 10.17632/55s6mczd9r.1
Van Wyngaard, J. D. V., Meeske, R., and Erasmus, L. J. (2018). Effect of dietary nitrate on enteric methane emissions, production performance and rumen fermentation of dairy cows grazing kikuyu-dominant pasture during summer. Anim. Feed Sci. Technol. 244, 76–87. doi: 10.1016/J.ANIFEEDSCI.2018.08.005
Vensim, D. S. S. (2024). Ventana systems. Available at: https://vensim.com/ (Accessed January 8, 2024).
Wang, J. M., Ellermann, T., Wåhlin, P., Jensen, S. S., Fang, D., and Massling, A. (2015). Particle number, particle mass and NOx emission factors at a highway and an urban street in Copenhagen. Atmos. Chem. Phys. 10, 2745–2764. doi: 10.5194/acp-10-2745-2010
Keywords: sustainable dairy, system dynamics, DESTiny, carbon sinks, carbon emissions, nutrient flows, South Africa
Citation: Reinecke R, Blignaut JN, Meissner HH and Swanepoel PA (2025) DESTiny, an online farm-wide tool to estimate the net carbon emissions of a pasture-based dairy farms in South Africa. Front. Sustain. Food Syst. 9:1491973. doi: 10.3389/fsufs.2025.1491973
Received: 05 September 2024; Accepted: 13 January 2025;
Published: 27 January 2025.
Edited by:
Christian Bux, University of Foggia, ItalyReviewed by:
Paul Wilson, University of Nottingham, United KingdomCopyright © 2025 Reinecke, Blignaut, Meissner and Swanepoel. This is an open-access article distributed under the terms of the Creative Commons Attribution License (CC BY). The use, distribution or reproduction in other forums is permitted, provided the original author(s) and the copyright owner(s) are credited and that the original publication in this journal is cited, in accordance with accepted academic practice. No use, distribution or reproduction is permitted which does not comply with these terms.
*Correspondence: Riana Reinecke, cmlhbmFAZmFybXZpc2lvbi5jby56YQ==
Disclaimer: All claims expressed in this article are solely those of the authors and do not necessarily represent those of their affiliated organizations, or those of the publisher, the editors and the reviewers. Any product that may be evaluated in this article or claim that may be made by its manufacturer is not guaranteed or endorsed by the publisher.
Research integrity at Frontiers
Learn more about the work of our research integrity team to safeguard the quality of each article we publish.