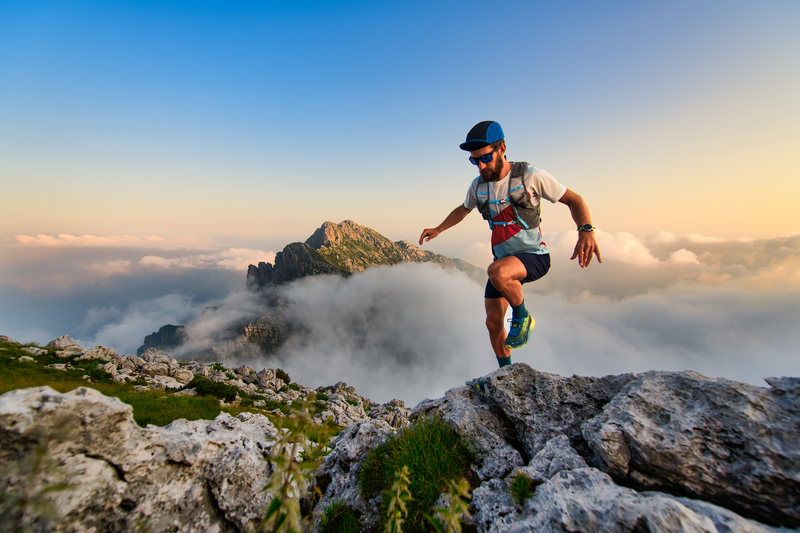
95% of researchers rate our articles as excellent or good
Learn more about the work of our research integrity team to safeguard the quality of each article we publish.
Find out more
ORIGINAL RESEARCH article
Front. Sustain. Food Syst. , 24 March 2025
Sec. Agricultural and Food Economics
Volume 9 - 2025 | https://doi.org/10.3389/fsufs.2025.1488994
Introduction: The rising concerns about food security and the increasing demands for meat-based diet in China have highlighted the imbalance between the supply and demand of its feed grains. Scholars view the imports of soybeans as an unsustainable way of ensuring feed grain security. Therefore, this study investigates the optimization of soybean and pig integration zones in China.
Methods: The study has adopted a multi algorithm approach based on Random Forest Model, LSTM model, KMeans clustering, and PCA to highlight the factors influencing the integration of feed grain plantation and meat security.
Results: The results of the PCA report that consumption, production levels, land availability, and pig quantity play an instrumental role in defining the integration of soybeans and pig farming. The results indicate that LSTM offers accurate predictions regarding the integration zones.
Conclusion: The study concludes that areas with high consumption of meat and large production volumes offer an opportunity to integrate soybean cultivation and pig farming in China.
Food security holds immense significance in a nation’s economic growth and social stability. Similarly, scholars view food security as crucial to maintaining the well-being of people in China (Lee et al., 2024). Today, China is responsible for feeding approximately 20% of the world’s population but has less than 10% of the global arable land (Dong et al., 2024). Over the years, the dietary preferences of the Chinese have shifted from plant-based cuisine to meat-based diets. Consequently, there has been a growing demand for animal-based diets as shown in Figure 1.
Figure 1. Meat consumption in China (pork dominates meat consumption in China). Source: Dong et al. (2024).
Meat consumption in China has shown a steady rise since the 1990s (Grimmelt et al., 2023). Therefore, meat security has emerged as a major concern in China (Yang and Cui, 2023). In 2021, Chinese citizens consumed approximately 100 million tons of meat, which made up 27% of the global meat consumption. Moreover, pork dominated meat-based consumption as the Chinese consumed 57 million tons of pork in 2021 alone. This accounted for 60% of their total meat consumption (Grimmelt et al., 2023). The increasing meat consumption has caused a rise in the demand for grains used as animal feed (Niu et al., 2021). Consequently, China is currently grappling with the issue of balancing the supply of grains for human needs and animal feed (Yang et al., 2021). Moreover, feed production in China increased by 10.3% to 319.8 million in 2023. This led to an increased demand for soybeans. In 2022–23, soybean production was recorded at 19.4 million tons and is expected to rise to 19.7 million tons in 2023–24. Total consumption is estimated to cross 118.2 million tons in 2024. However, domestic production has not been able to meet the increasing demand for soybean feed. Therefore, China’s imports of soybeans have also increased as the country imported 100.86 million tons of soybeans in 2022–23 (Reidy, 2023). China’s grain and soybeans imports are projected to grow till 2030 as shown in Figure 2. Consequently, a critical challenge of striking a balance between feed grain security and meat security has emerged in China (Yang and Cui, 2023; Wu et al., 2020).
Figure 2. China’s grain and soybean imports (China’s grain and soybeans imports are projected to grow alongside the growing meat imports). Source: Gale (2021).
Soybean is a major part of pig feed (Sporchia et al., 2021). It is due to its high protein content (up to 50%) along with amino acids and low water content. However, scholars have shown concerns due to the unsustainability associated with soybean imports and the risks of trade disruptions (Gomez Izquierdo et al., 2020).
Systems Theory together with Resource-Based View (RBV) form the theoretical basis for studying soybean production and pig farming integration practices in China. The agricultural supply chain operates as an entire system under Systems Theory since feed grain availability alongside livestock production along with environmental elements affect each other through interconnected components. Food security outcomes for the soybean-pig integration areas require balanced operation of interdependent feeding grain production (soybeans) alongside meat security through pig farming. Sustained meat production performance relies on functional interactions between all components of supply chain operations because even marginal soybean import changes or land availability limitations will negatively affect sustainability. As per the Resource-Based View (RBV) industrial competitive advantages materialize through proper resource management and utilization. Agricultural land alongside soybean crops and pig farm infrastructure deserve strategic planning because it enables sustainability while reducing imports of soybeans. Machine learning techniques including Random Forest (RF) and Long Short-Term Memory (LSTM) as well as clustering algorithms assist this study to locate resource-efficient integration zones through data-driven identification of regions possessing big soybean yields and valuable pig farming conditions. Traditional agricultural planning systems lack awareness of soybean production-pig farming spatial correlations yet PCA analysis with clustering along with predictive models (RF and LSTM) establish data-based methods to determine the best integration areas. The dynamics of different agricultural factors explained through Systems Theory validate the link between RBV and strategic resource distribution for improving sustainability along with food security. This theoretical framework demonstrates that managers must navigate between sustainably securing feed grains and animal products through technological enhancements that enhance farming performance within discussions about food sustainability and efficient agriculture applications of machine learning.
Relying solely on imports would not ensure feed grain security and meat security in China (Yang and Cui, 2023). Localization of feed production is regarded as a more sustainable way of ensuring the availability of feed. Moreover, reliance on the feed produced near animal farms would offer several economic, environmental, and social advantages. Maximum efficiency in pig farming depends on the integration of pig facilities with nearby soybean fields located in top agricultural regions. Within the selection clusters it is vital to evaluate how crops mix because this assessment protects soil conditions while enhancing land resource efficiency and securing sustainable long-term growth. This includes reduced logistics expenditure and strengthened viability of animal farms (Gomez Izquierdo et al., 2020). Though several studies have investigated food security in China from several perspectives by using quantitative and qualitative methods, few have focused on the balance between feed security and meat security (Niu et al., 2021; Liu Z. et al., 2021). There is a noticeable gap in research that specifically addresses the critical balance between feed security and meat security. Despite this pressing challenge, there is a scarcity of research that directly addresses the optimization of resource allocation for pig feed within the context of China. This study uniquely contributes to the field by focusing on this balance, utilizing an innovative multi-algorithm approach that includes Random Forest (RF), LSTM, and clustering techniques to optimize soybean-pig integration zones. Therefore, the first objective of this study is to investigate factors influencing the balance between feed grain and meat security. The methodological novelty of this study lies in its reliance on a multi-algorithm approach, involving RF, LSTM networks, and clustering analysis. Previous studies on food security and feed efficiency have predominantly employed traditional econometric models (e.g., linear and logistic regression), simulation-based techniques, and qualitative case studies (Rauw et al., 2023). While these approaches have provided valuable insights, they often fail to fully capture complex, nonlinear relationships and spatial dependencies within the data. In contrast, the integration of machine learning techniques in this study allows for improved pattern recognition and predictive modeling. Furthermore, the study utilizes Principal Component Analysis (PCA) for dimensionality reduction and visualization of the contribution of various agricultural and demographic features to the overall variability in the selected dataset. Unlike conventional regression-based studies that rely on predefined assumptions, PCA enables a data-driven exploration of key factors influencing feed grain and meat security. This approach not only streamlines the datasets but also provides unique insights into the complex interactions between these features, enhancing the understanding of sustainability challenges in soybean-pig integration zones.
Existing studies on animal feed efficiency and sustainability lack a geographical focus on China, despite its significant role in global meat production (Gomez Izquierdo et al., 2020). Addressing this research gap, the second objective of this study is to generate data-driven strategic recommendations for policymakers and stakeholders based on the insights gained from the analysis. This is aimed at achieving a sustainable balance between feed grain availability and meat production in China’s soybean-pig integration zones by leveraging machine learning-based optimization techniques. This study has immense relevance and significance in the context of increasing global demand for meat and feed grain (Rauw et al., 2023). In the context of China, this study has the potential to extend the literature concerning the strengthening of self-sufficiency in feed grain (Li et al., 2024). By focusing on region-specific resource allocation, this study also adds to the understanding of the significance of the geographical location of feed grain production to strengthen the self-sufficiency of a region. Moreover, the insights derived from this research offer innovative, evidence-based strategies for improving feed efficiency and reducing reliance on imports. This indicates the unique contribution of the study to the literature focused on China’s agricultural policy and food security.
Pig diets are typically composed of soybeans and wheat (Sporchia et al., 2021) with around 70–75% of the soybean production of the world which is being utilised in animal feed (Brack et al., 2016). According to Wilke et al. (2023), in 2021/2022, Brazil was considered the leading producer followed by Argentina and the United States. However, the cultivation of soybeans in Brazil contributes to deforestation (Rajão et al., 2020), which leads to a significant greenhouse gas emission. It is further exacerbated by the transportation of soybeans to Europe. Soybean meal is considered to have the highest greenhouse gas emissions among the sources of protein. It is primarily due to the land-use changes in those regions where agriculture has intensified in recent years (Wilke et al., 2023). The diet of pigs typically involves a balanced mix of grains, proteins, vitamins and minerals so that proper growth, productivity and health can be ensured. Research by Parrini et al. (2023) highlights that Soybean is one of the most expensive and limiting ingredients of food in the formulation of diet. However, in pig farming it represents the key source of protein. Soybean is the primary imported protein food in Europe largely due to the limited available cultivated area within the region. The increasingly prevailing demand for soybean is driving global land use changes and has been directly associated with deforestation in South America. However, it in turn increases the carbon footprint of feed (van Zanten et al., 2018). Despite of the detrimental influence of soybean cultivation on tropical forests ecosystems (Świątkiewicz et al., 2021) and the growing recognition of unsustainability of relying heavily on imported feed proteins for livestock (Tallentire et al., 2018), Soybean has become the preferred choice among the protein feed due to its availability and quality. Post-extraction soybean meal is particularly vital for monogastric animals such as pigs and poultry which serves as their key source of protein (Wilkinson and Lee, 2018; Sońta et al., 2022). Poultry and swine account for approximately 42% of the total feed production which contributes to the supply of 20% of animal proteins, while ruminants use about 19% of the feed to produce the same 20% of the animal proteins. In the organic pig sector, the challenge of meeting protein demand is even more pronounced (Schwediauer et al., 2018). Soybean is an essential and common component in the diet of pigs (Parrini et al., 2023) which provides a high-quality source of protein (Deng et al., 2023) and is important for muscle development.
Monogastric animals are mainly broilers which lay pigs and hens and are kept in industrial housing systems. Their feed consists of more than 25% of grains. In this regard, removing these human edible ingredients from the diets of pigs and poultry can contribute substantially to the human food supply globally while at the same time reducing the environmental impact of entire food systems (Boumans et al., 2022). Modern production practices represent challenges to the health and welfare of pigs which has justified the use of antibiotic health promoters. However, the influence of AGP on weight gain and feed conversion is variable. Several studies (Cardinal et al., 2021) have found no significant difference in the performance of animals fed an AGP diet when there are no underlying health issues. Conversely, some researchers have also studied that AGP can have a positive influence on the weight gain of pigs and feed conversion (Cardinal et al., 2021). In the past few decades, the exponential growth in intercontinental soybean trade has paralleled a significant increase in the production of pigs (Wang et al., 2018). Soybean meal is the key component in livestock feed because of its high protein content which typically ranges from 40 to 50% along with its optimal profile of amino acid. Furthermore, it has a relatively lower water content, consistent nutrition composition and anti-nutritional factors that can be easily eliminated and mitigated (Gomez Izquierdo et al., 2020). However, the global reliance on soybean meal as a primary source of protein is also a significant concern with varying points of emphasis depending on the stakeholder. In this regard, the non-governmental organisations (NGOs) are particularly concerned regarding the expansion of soybean cultivation into natural ecosystems in the countries including Brazil and Argentina. It is resulting in deforestation, loss of biodiversity, degradation of soil and the displacement of small farmers and indigenous communities (Roman et al., 2016; Rauw et al., 2020). On the other hand, the European parliament is primarily concerned with its heavy reliance on costly imports of soybean which accounts for approximately 70% of agricultural protein products and over 95% of soybean grains and meal (Roman et al., 2016; Gomez Izquierdo et al., 2020). Due to the perceived unsustainability of this heavy dependence on the imports of soybean meals and the associated risks of trade disruptions, the European Parliament passed a resolution in 2011 aimed at encouraging on-farm animal feed production utilizing mixed crops such as beans and cereals (Rauw et al., 2020; Gomez Izquierdo et al., 2020).
The quantity and quality of food resources directly influence the pig production output. Feeding pigs’ diet based on local protein crops with suboptimal nutritional quality can also result in a decline in the efficiency of production. This is significant as it can negatively influence the profitability thereby affecting the economic sustainability of livestock farms (Galioto et al., 2017). Consequently, it is crucial to assess the extent to which pigs can maintain the efficiency of production when fed locally produced protein crops (SUSPIG, 2020). However, the research by Gomez Izquierdo et al. (2020) also declared that there is an interest in replacing the soybean meal with locally produced ingredients in the feeds of livestock. Due to the cumulative small differences in feed efficiency among the four diets, their study mentioned that pigs in the V0 group attained the highest body weight (BW), while pigs in the V20 group had the lowest BW despite the similar intake of feed. However, the economic implications of including Narbon vetch in the diet depend on the additional feed required and the associated costs, as well as the expenses associated with the extra days on the far required for the pigs to reach a specific slaughter weight.
Gomez Izquierdo et al. (2020) argue that due to the reliance of the European Union on Soybean meal imports for animal feed, there is growing interest in the exploration of competitiveness of locally produced protein crop alternatives. The local production of feed crops is usually deemed more sustainable than relying on imported feed. This is because it can reduce the usage of energy associated with transport, support on-farm crop rotation so that the risk of crop diseases can be lowered, and enhance the economic viability of local farms. However, the economic sustainability of using local feed crops can be compromised if their sub-optimal nutritional quality results in decreased animal production efficiency and lower farm profitability. Narbon vetch is considered a potential alternative protein crop due to its reliance on adverse climate and social conditions, resistance to common pests, favorable nutritional profile and suitability for the Mediterranean climate. Despite these advantages, the presence of the “anti-nutritional factor y-glutamyl-S-ethenyl-cysteine” limits its application in animal nutrition. The results of their study showed that there is a notable decrease in daily intake of feed and consequently lower daily body weight gain up to four weeks after introducing this novel feed (Gomez Izquierdo et al., 2020). However, pigs adapted to the new feeds and daily efficiency of feeds measured as body weight gained per unit of feed intake was only reduced significantly during the initial period of transition particularly at the highest inclusion level of 20% Narbon Vetch.
According to the “China Animal Husbandry and Veterinary Yearbook,” pig farms with more than 50 slaughtered pigs are classified as scale farms. In contrast, smallholder pig producers in China typically have low animal pig output (Yang and Cui, 2023). Currently, almost 90% of all the farm pigs in China are raised on small-scale farms. These traditional farming practices often overlook the significance of suitable geographical locations for farm construction. It results in pigs being raised in close quarters with inefficient utilization of space and generally a poor infrastructure (Jiang et al., 2022). Such farms are more susceptible to animal-borne diseases which complicate the tracking and control of outbreaks (Dixon et al., 2020). Furthermore, traditional backyard farming (with fewer than 50 pigs) consumes significant natural resources (Chen et al., 2022). However, large-scale pig farming is viewed as a promising approach to boost the productivity and income of farmers (Yu et al., 2020; Tian et al., 2021). These days many large-scale farms integrate grain production within their agricultural operations which usually integrates livestock, planting, and renewable energy production (Yang and Cui, 2023). Placing high-productivity zones at the forefront will provide the best opportunity to maximize feed efficiency when integrating pig farms with soybean production. The evaluation of crop suitability within clusters plays a vital role for sustainability purposes and controlling soil degradation when maximizing resource utilization (see Table 1).
The flowchart of this study is shown in Figure 3.
The data for this study was collected from (Yang and Cui, 2023) using different sources to verify extensive coverage for evaluating Soybean-Pig Integration Zones in China. The data about farming practices, pig quantity, human consumption per capita, production volume, and agriculture land collected from China Animal Husbandry and Veterinary Yearbook, China National Bureau of Statistics, FAOSTAT (Food and Agricultural Organization of the United Nations) and Ministry of agriculture and rural affairs of the people’s republic of China-database. The reference to the dataset is provided in the Appendix section.
Missing values were removed using the function dropna() in the pandas’ library, in the result many categorical and numeric variables related to soybean production and pig farming will be comprised in the dataset, see Equation 1.
Where is a dataset, are the records, is an indicator function, and indicates the new dataset.
To standardize model training features, continuous variables has been standardized for standardize model training features using Equation 2.
Where are the value of original feature, are minimum feature values and are the maximum feature values.
For the prediction of porks’ production in million tons with the use of multiple variables of predictor such as quantity of pig in x 1,000 heads, Land of agriculture in sq. km, and more. LabelEncoder was used to encoded categorized variables within numerical values using Equation 3.
The feature selection process was employed utilizing a random forest (RF) for the enhancement of model efficiency and accuracy. RF is an ensemble technique that was utilized for every feature’s importance to ranking (Han and Kim, 2021). Many decision trees were constructed by the RF method during the training and output the class mode for classification or regression mean prediction. The score of feature importance was calculated and findings for prediction were utilized to organize the influential features. The feature importance formulation using RF can be calculated using Equation 4.
Where is the feature s importance , are total trees, and is the feature s importance in tree .
The principal component analysis (PCA) method was used for dimensionality reduction for converting a larger variable set to a smaller set but most information still exists in the larger set (Wang et al., 2024). In this study, the scaling features with the use of “StandardScaler,” and PCA were applied, and two principal elements were saved, which captured the most important variance within the set. The initial two elements as new features are then appended with a dataset which was called PCA1 and PCA2. The following Equation 5 can be used for PCA.
Where is the principal component, and are a matrix of scaled data and PCA matrix.
A feature engineering process was performed after the reduction in selected features. Interaction terms and temporal features like rolling statistics and lag features were generated. The feature engineering set of features can be calculated using Equation 6.
It was applied initially on selected features and features engineering to estimate the performance of the model. In this study, our model was trained using 80–20 data splitting, in which 80% of the set was used for training the model and 20% for testing the model. With the use of MSE (mean squared error), the performance of the model was estimated, see Equation 7.
Where p is the total observation, and are the actual and predicted values, respectively.
A process of hyperparameter tuning was established utilizing the GridSearchCV for the random forest optimization parameters. The parameters were explored n_estimators for the tree number, min_samples_split for node’s splitting samples, max_depth, and min_samples_leaf for the sample needed for leaf node. The selection of optimal parameters was based on the smallest MSE attained using cross-validation.
An LSTM technique was also applied in this study. LSTM consists of 3 sigmoid gates as well as 1 tanh layer. Gates are introduced to limit the passage of information across the cell. It determines which information’s part is required for the next unit and which information’s part is discarded. Generally, output lies between 0–1, 0 means reject all, and 1 accept all (Liu C.-H. et al., 2021). In this study with the use of features sequence the lstm model is trained, along with the setting of time steps is 10. The architecture of the model consists of two layers each with 50 units, and a dense layer adopted with output one. With the use of the MSE loss function and Adam optimizer the model LSTM was trained, see Equation 8.
Here represent sequences of input.
This approach for regions group through same features recognition. This algorithm divides the data within k groups or clusters (Coates and Ng, 2012), reducing the variance in each group. K-means clustering served as the analysis method to evaluate pig farming with soybean production integration prospects throughout China. The research evaluated four essential variables including available land areas and distributed pig numbers and existing soybean cultivation rates as well as animal feed requirements. The chosen factors directly affect the possibility of realizing the integration between pig farms and soybean production. The research evaluated these factors to discover geographic areas for optimal resource management which would enhance integration and establish sustainable farming practices.
The Silhouette Score indicated the best cluster number when set at k = 4 through its evaluation of cluster similarities. The best partition among clusters occurred when the analysis employed k = 4 clusters. The selected cluster number produced the best separation between different regions according to their agricultural characteristics.
Analysis revealed that the first cluster contains regions having both high soybean output alongside minimal pig livestock existence. The land resources in these regions provide a large potential for developing the pig farming industry because sufficient space is available for increasing production. The second data cluster demonstrates perfect conditions for establishing integrated farming when pig farms match the soybean cultivation levels. The existing conditions in these regions create perfect conditions for producing both pigs and soybeans so their territories represent top choices for resource utilization to achieve integrated production goals.
Areas belonging to Cluster 3 demonstrate high numbers of pigs yet low soybean crop cultivation levels. The main difficulty in these areas exists because there are not enough soybeans that pigs need for their feed as pig farming depends on soybean feed supplies. Improving feed grain availability together with procedures to enhance soybean cultivation needs to be implemented as a solution. The cluster 4 demonstrates that areas with limited soybean farming and low pig population density are considered unfit for integration purposes. The deficits of resources and poor infrastructure in these zones endanger future agricultural integration projects between pigs and soybeans.
Clustering results were examined on the basis of Silhouette scores to estimate the optimal clusters’ number and its correlation with soybean pig integration. The formula for KMeans can be considered as Equation 9.
To evaluate the clustering quality Silhouette score metric was found using Equation 10.
This study provides a detailed analysis of how to incorporate the two elements for feed grain and Meat security. This section summarizes the results of Random Forest, LSTM networks, and clustering analysis and presents figures and tables to explain the results in detail.
From the aspect of feature importance analysis, the Random Forest model, which is widely known for its outstanding ability to resist the elimination of feature importance, has given a comprehensive view of how different features affect the optimization of soybean-pig integration spaces. The feature importance scores are provided in the following Table 2.
Their importance score indicates that “Human Consumption per Capita” is the most important factor with a score of 34. 87%. This implies that areas of high human endowment are strategic in defining areas of the right blend between the two. Its prominence signifies that soybeans and pigs can be integrated into these areas to mirror consumption hence boosting feed and meat security. The second highest-ranked criterion is the “Production Volume” with an average score of 28. 89. This underlines its important position in the model, stressing thereby that intensified production rates are essential for satisfying the needs of integration zones. This policy was scored 22 when considered under the ‘Agricultural Land’ policy. Availability of land for cultivation, cost of seeds and fertilizers, plant diseases and pests, weeder, training of farmers, adequacy of water supply, market demand for produce, and many more, contribute 09% to the optimization. However, the “Pig Quantity” is responsible for 13% of the total variance. Humble, but still significant, the blog claimed that sugar influenced only 31%. Low values of mean decrease in accuracy of “Farming Practices” (0. 83%) and “Location” (0. 00%) indicate that these variables have insignificant influence on the choice of variables that the model makes.
The scores of the feature importance are also presented in a bar chart as shown in Figure 4. As it is evident from the given chart, both the ‘Human Consumption per Capita’ and the ‘Production Volume’ contribute immensely towards the integration zones and so there is a huge requirement to put more attention on these two parameters only which needs optimization (Breiman, 2001; Zhang et al., 2024).
For analysis of the dimensionality of the data, the method of Principal Component Analysis was used. From the above discussion, it is clear that PCA has the ability to simplify the data by pointing out the factors that explain most of the variance. The PCA components are shown in the following Table 3.
Thus ‘Human Consumption’ together with ‘Production Volume’ and ‘Agricultural Land’ make up the Primary Component better referred to as Principal Component 1 (PC1). The fact that the above-mentioned features have negative loadings means that high human consumption, large volume of production, and the area under agriculture are in the same direction of the component. In the second principal component on the other hand, we see that there are clear loadings of the features “Pig Quantity” and “Location” which means these have done a contributory function in the second principal component in a parallel fashion to other factors (Greenacre et al., 2022; Abdi and Williams, 2010).
The PCA biplot, displayed in Figure 5, represents the loadings of features on the first two principal components. The plot also enables one to grasp how these features supply differentiation to the PC 1 & 2, pointing to the spatial configuration of such integration zones based on certain factors.
In the case of Random Forest model performance, the values arising from the surveys are stated below and in Table 4.
A forecast with Mean Squared Error (MSE) equal to 1 at the beginning. 6,469 refers to the model when it has not been adjusted for hyperparameters. This value assesses how well the model approximates the data at the start. Having done hyperparameter tuning the MSE rose to 3. 2,619, hence, the values may require fine-tuning to come up with the best model of the regression. The chosen parameters are the highest depth of 10, 100 estimators, minimum split and minimum of 2, and the least possible number of leaf nodes. These parameters are the best choice for enhancing the work of the model or the given algorithm (Liaw and Wiener, 2002; Friedman, 2001).
These are depicted in Figure 6, which shows the MSE values before and after hyperparameter tuning. The plot demonstrates a change in performance with different parameter settings, which means that though the models have initial acceptable performance, further optimization could improve the prediction.
The performance of the LSTM model was assessed concerning its training loss over epochs as indicated in the following Table 5.
The analysis of the LSTM model prescribed considerable enhancement in aspects of the training loss, ranging from 0. The total cross-sectional sample is 5,926 at epoch 1 to 0. 0198 by epoch 50. This reduction of loss shows good training and learning over the epochs of the model and LSTM in particular proves it can model sequential data well (Greff et al., 2016).
As shown in Figure 7, the performance of the LSTM model is shown in terms of loss reduction over 50 epochs, in order to present the learning process of the model. From the graph, it is apparent that losses are gradually falling which proves the enhancement of the model’s performance together with its capacity to capture the temporal characteristics of the data.
The LSTM model predictions summarized in Table 6 include the regular unscaled and the scaled by 100 values for comparison while the inverse transform function is also applied to these scaled predictions.
The scaled predictions that were obtained in this study range from 0. 748 to 0. 782, are the normalized values which is an output of the LSTM model. The predictions, when transformed inversely, vary between 39 and 71 for the customers. 922 to 41. 266, are the figures from the model reproduced in the original scale. This is seen from their actual predicted outcomes on the integration zones as will be illustrated below (Zhang et al., 2018).
Figure 8 shows the scaled and the inverse transform obtained to present how the output of the LSTM model looks when the normalized values are translated back to such predictions. This comparison is useful in comparing the model in as much as it predicts the optimality of integration zones accurately.
Then, using a KMeans clustering approach on the respective scaled data, it was possible to identify those zones that would require the integration of soybeans and pigs. Table 7 presents the Silhouette Score as well as the scaled as well as the descaled cluster centroids.
Using KMeans clustering, more areas can be divided according to important agricultural characteristics and assist in determining patterns suited to resource distribution. It also serves to increase food security by recognizing areas of concern, for instance, feed grain, and application to increase feed meat ratios sustainably.
By using the KMeans clustering analysis, the author of the paper defines the areas within China with the similar features concerning feed grain and meat production. In so doing, the analysis opens avenues into optimizing resource distribution, as well as improving food security, and achieving some balance in meat supply in these clustered regions. The specific division of the 12 countries into clusters for further analysis, and the explanations for these clusters, can be seen in Figure 9.
Understanding these findings from the present study that uses a multi-algorithm approach, the following conclusions can be drawn concerning the soybean-pig integration zones: the independent variable importance in the Random Forest model: it offers information about features and their importance where the major ones include Human Consumption per Capita and Production Volume. It also displays how features together contribute to the optimization of integration strategy, which is why PCA shows the principal components that are responsible for that. It’s seen that the LSTM model is a good learning and prediction model that reduces over epochs and has accurate predictions in the original scale. Altogether these assessments provide all-inclusive insight into the variables affecting detailed optimal integration zones, thus promoting feed grain and meat security in China.
The Random Forest model uncovered essential information about substantial features that should be prioritized during the optimization process of soybean-pig integration areas. Human Consumption per Capita (34.87%) together with Production Volume (28.89%) emerged as the primary indicators for determining zone integration outcomes. Results from the analysis show that zones with peak pork consumption together with large production numbers should be prioritized for structural integration. Designated zones need large-scale farming infrastructure because both Agricultural Land Availability and Pig Quantity proved significant based on the analysis results (22.09 and 13.31% respectively). Before hyperparameter optimization the model’s Mean Squared Error (MSE) was 1.6469 then it rose slightly to 3.2619, indicating the model had to sacrifice performance for additional complexity.
The tested LSTM neural network system successfully identified temporal patterns that existed in soybean-pig integration data datasets. Training loss measurements showed an ongoing reduction from epoch 1 initial value of 0.5926 to epoch 50 value of 0.0198 during the learning process. The predictions from the model produced scale value ranges from 0.748 to 0.782 which correspond to actual outcomes from 39.922 to 41.266 million tons. As a consequence the LSTM demonstrates strong performance in processing sequential dependencies thus becoming effective for agricultural time-series forecasting applications.
A Principal Component Analysis (PCA) reduced the dimensions of data to maintain the most significant variance between them. The majority of variables in the dataset were explained through the initial two principal components (PC1 and PC2). PC1 received its main influencing variables from Human Consumption along with Production Volume while Agricultural Land contributed as a third variable which shows production and consumption areas match in demand rates. The PC2 analysis measured Pig Quantity and Location as vital variables for optimally distributing livestock farms. The PCA biplot effectively displayed the correct spatial distribution of integration zones in addition to KMeans clustering results.
This study aimed to investigate the integration of feed grain security and meat security in China. The results of the “Random Forest Model” regarding the feature importance score indicate that “Human Consumption per Capita” with a score of 34.87% occupies the most significant position for defining the integration of feed grain and pig farms. These findings imply that areas of high meat consumption are promising zones for integrating feed grain and pig farms. Subsequently, the “Production Volume” with a score of 4.28 emerges as the most significant factor for blending feed grain production and pig farms. This indicates that there should be a high production volume to ensure efficient integration of both zones. The research of Cordeiro et al. (2022) supports the key role of feed availability for promoting sustainable meat supply in areas heavily focused on livestock production. According to Grimmelt et al. (2023) the steady Chinese meat consumption increase since the 1990s reinforces the necessity of developing feed-to-meat security strategies. In 2023, China produced approximately 96.41 million metric tons of meat, indicating an increase of almost 3.4% over 2022 (Textor, 2024). This shows that the production volume of meat in China has the potential to satisfy the needs of both zones. These findings also imply that meat security and feed grain sufficiency are particularly important in areas with high levels of consumption and production. Moreover, “Agricultural Land” with an average score of 22.09 also emerged as a significant feature for an efficient integration of both zones. These findings indicate that the availability of land area is crucial to maintaining sufficiency in feed grain cultivation and meat production. The research results confirm (Jiang et al., 2020) who established the fundamental role of land resources in obtaining sufficient food outputs. These findings reinforce (Yang and Cui, 2023) argument that localized feed production is essential for reducing import dependence. Researchers have warned of ecological damage from big-scale soybean imports to Chinese territory thus supporting the demand for domestic soybean self-sufficiency under China’s agricultural policy system (Rajão et al., 2020). Additionally, the role of Pig Quantity in the integration process is supported by previous literature. The significant role of Pig Quantity with an average score of 13.31 indicates that the farms with large numbers of pigs would suffice the purpose of zone integration.
In addition, the results of the PCA group the regions with high human consumption, large production volumes, and land availability together. This analysis has immense significance as it suggests areas, which may promise an efficient integration of soybean and pigs. The research results align with (van Zanten et al., 2018), who highlighted the environmental impact of soybean demand on global land-use changes (Dixon et al., 2020) studied how disease risks affect small-scale pig farms which motivated the need for structured integration to enhance productivity and food security. In addition, the results indicate the potential of the LSTM model to provide accurate predictions. These findings are significant considering the dominant position of pork in the meat market in China. Moreover, pig farms are responsible for the largest proportion of soybean consumption due to herbivorous livestock (Yang and Cui, 2023). Since domestic feed grain production falls short of meeting demand, integrating feed grain and pig farms offers a sustainable solution (Li et al., 2024). In addition, considering the unsustainability of soybean imports, the integration of zones would be instrumental in ensuring local cultivation of feed grains. The results encourage a large-scale production of both soybeans and pork production. This aligns with the vision of the Chinese Government to promote agriculture as highlighted in the “14th Five-Year Plan” (Houkai, 2022). This plan encourages the optimization of agricultural practices by allotting functional zones for products like soybeans. This crop-livestock integration system would serve as an effective strategy to strengthen feed grain security and meat security in China. This would help China achieve a balance between both zones. However, it is important to consider various factors, including consumption patterns and production volume while integrating the production of soybeans and pigs. Thus, targeting areas with high consumption and large production volumes of pork would help sustain a balance between the two zones.
This study extends the understanding of crop-livestock balance by highlighting the need for optimizing feed grains cultivation and meat security (Paul et al., 2022). These findings have significant implications for sustainability literature as they highlight the unsustainability of soybeans imports. Focusing on the local production of soybeans makes the findings of this study immensely relevant in the context of sustainable agriculture. Moreover, the focus of the study on a country like China where pork consumption is at its all-time high, makes it relevant to the contemporary context. Another important implication of this study is its focus on the potential of the LSTM model to provide accurate predictions. Moreover, the study has made use of PCA to categorize production zones based on their similar characteristics.
The practical implications of this study focus on how a crop-livestock integration can answer the concerns related to food security in China. This implication is supported by the existing literature (Sekaran et al., 2021; Battheu-Noirfalise et al., 2024). The study suggests that policymakers need to integrate these two zones where the consumption and production of pork are at high levels. Therefore, large-scale integration of soybeans and pig farms is suggested here. In addition, this integration has the potential to strengthen the agricultural resilience of the region (Devkota et al., 2022). These findings are relevant in other geographical regions where meat consumption is high. The “Ministry of Agriculture and Rural Affairs” can play a crucial role in proposing a development plan focused on encouraging crop-livestock integration in high-yield regions. However, this plan should also consider the environmental impact of soybean production into consideration.
Despite the comprehensive analysis provided by the study; several limitations exist in the study which highlights avenues for future research. Firstly, this study relies on specific datasets for training the models. It includes Random Forest LSTM (He et al., 2019) which has limited the generalizability of the findings to other regions or different scales of integration. Furthermore, the performance of the model in this study is highly dependent on the quality and completeness of the data, which may introduce biases if certain variables are under-represented or missing. The study also primarily focuses on the technical aspects of the integration without deeply considering the socio-economic or environmental influence. It can influence the feasibility and sustainability of the proposed zones of integration. For future research, it is recommended to incorporate a broader range of variables, including environmental and economic factors so that the robustness of the models can be enhanced. Furthermore, the application of frameworks to different geographic regions or scales could help in the validation and refinement of the model further. Integration of advanced AI techniques such as the Internet of Things (IoT) or remote sensing as suggested by Fuentes-Peñailillo et al. (2024) can also be helpful with the input of stakeholders. It can also provide a more holistic approach to the optimization of soybean pig-integration zones. In this way, it can be ensured that the solutions are practical and sustainable in real-world applications. The following table verifies the study’s objectives against the research conclusions to confirm that the study methods accomplished their intended goals.
Optimizing a balance between feed grain security and meat security would strengthen China’s overall food security. The localized production of soybeans can enhance sustainable agriculture. Based on a multi-algorithm approach, this study has drawn the following conclusions: (1) Ensuring a sustainable level of feed grain and meat production in regions with high consumption is crucial. (2) Large production volumes contribute to self-sufficiency in soybean production and help balance the demand and supply of feed grains. (3) The availability of extensive land resources enables the efficient integration of feed grain plantation and pig farming. The reliance of pork production on feed grains highlights the insufficiency of the domestic soybean supply, emphasizing the need to rebuild the link between crop cultivation and livestock farming. The findings suggest that integrating soybeans and pig farms in areas with high consumption, large production volumes, and sufficient land resources would help optimize the balance between feed grain cultivation and meat security in China.
The original contributions presented in the study are included in the article/Supplementary material, further inquiries can be directed to the corresponding authors.
ZZ: Conceptualization, Writing – review & editing. YF: Data curation, Writing – original draft. HW: Supervision, Writing – review & editing. BA: Methodology, Writing – review & editing. FJ: Conceptualization, Writing – original draft.
The author(s) declare that no financial support was received for the research and/or publication of this article.
The authors declare that the research was conducted in the absence of any commercial or financial relationships that could be construed as a potential conflict of interest.
All claims expressed in this article are solely those of the authors and do not necessarily represent those of their affiliated organizations, or those of the publisher, the editors and the reviewers. Any product that may be evaluated in this article, or claim that may be made by its manufacturer, is not guaranteed or endorsed by the publisher.
The Supplementary material for this article can be found online at: https://www.frontiersin.org/articles/10.3389/fsufs.2025.1488994/full#supplementary-material
Abdi, H., and Williams, L. J. (2010). Principal component analysis. Wiley Interdis. Rev. 2, 433–459. doi: 10.1002/wics.101
Battheu-Noirfalise, C., Mertens, A., Soyeurt, H., Stilmant, D., Froidmont, E., and Beckers, Y. (2024). Influence of crop-livestock integration on direct and indirect contributions of beef systems to food security. Agric. Syst. 220:104067. doi: 10.1016/j.agsy.2024.104067
Boumans, I. J., Schop, M., Bracke, M. B., de Boer, I. J., Gerrits, W. J., and Bokkers, E. A. (2022). Feeding food losses and waste to pigs and poultry: implications for feed quality and production. J. Clean. Prod. 378:134623. doi: 10.1016/j.jclepro.2022.134623
Brack, D., Glover, A., and Wellesley, L. (2016). “Agricultural commodity supply chains” in Global Trade Analysis Project (GTAP) West Lafayette, IN: Purdue University, (Indiana, USA: Chatham House).
Cardinal, K. M., Andretta, I., Silva, M. K. D., Stefanello, T. B., Schroeder, B., and Ribeiro, A. M. L. (2021). Estimation of productive losses caused by withdrawal of antibiotic growth promoter from pig diets–Meta-analysis. Sci. Agric. 78:e20200266. doi: 10.1590/1678-992x-2020-0266
Chen, L., Guttieres, D., Koenigsberg, A., Barone, P. W., Sinskey, A. J., and Springs, S. L. (2022). Large-scale cultured meat production: trends, challenges and promising biomanufacturing technologies. Biomaterials 280:121274. doi: 10.1016/j.biomaterials.2021.121274
Coates, A., and Ng, A. Y. (2012). “Learning feature representations with k-means” in Neural networks: tricks of the trade. Second ed (Cham, Switzerland: Springer Berlin Heidelberg), 561–580.
Cordeiro, M., Mengistu, G., Pogue, S., Legesse, G., Gunte, K., Taylor, A., et al. (2022). Assessing feed security for beef production within livestock-intensive regions. Agric. Syst. 196:103348. doi: 10.1016/j.agsy.2021.103348
Deng, Z., Duarte, M. E., Kim, S. Y., Hwang, Y., and Kim, S. W. (2023). Comparative effects of soy protein concentrate, enzyme-treated soybean meal, and fermented soybean meal replacing animal protein supplements in feeds on growth performance and intestinal health of nursery pigs. J. Anim. Sci. Biotechnol. 14:89. doi: 10.1186/s40104-023-00888-3
Devkota, M., Frija, A., Dhehibi, B., Rudiger, U., Alary, V., Mhamed, H. C., et al. (2022). “Better crop-livestock integration for enhanced agricultural system resilience and food security in the changing climate: case study from low-rainfall areas of North Africa” in Food security and climate-smart food systems: Building resilience for the global south (Springer International Publishing), 263–287.
Dixon, L. K., Stahl, K., Jori, F., Vial, L., and Pfeiffer, D. U. (2020). African swine fever epidemiology and control. Ann. Rev. Animal Biosci. 8, 221–246. doi: 10.1146/annurev-animal-021419-083741
Dong, K., Prytherch, M., McElwee, L., Kim, P., Blanchette, J., and Hass, R. (2024). China’s food security: key challenges and emerging policy responses. Available online at: https://www.csis.org/analysis/chinas-food-security-key-challenges-and-emerging-policy-responses.
Friedman, J. H. (2001). Greedy function approximation: a gradient boosting machine. Ann. Stat. 29, 1189–1232. doi: 10.1214/aos/1013203451
Fuentes-Peñailillo, F., Gutter, K., Vega, R., and Silva, G. C. (2024). Transformative Technologies in Digital Agriculture: leveraging internet of things, remote sensing, and artificial intelligence for smart crop management. J. Sens. Actuator Netw. 13:39. doi: 10.3390/jsan13040039
Gale, F. (2021). China projected to be a leading meat importer. Available online at: https://www.usda.gov/sites/default/files/documents/S7_Gale-China-livestock-import-projections.pdf
Galioto, F., Paffarini, C., Chiorri, M., Torquati, B., and Cecchini, L. (2017). Economic, environmental, and animal welfare performance on livestock farms: conceptual model and application to some case studies in Italy. Sustain. For. 9:1615. doi: 10.3390/su9091615
Gomez Izquierdo, E., Gomez-Raya, L., de Mercado de la Pena, E., Ciruelos, J. J., and Rauw, W. M. (2020). Feed efficiency can be sustained in pigs fed with locally produced Narbon vetch (Vicia narbonensis L.). Sustainability 12:3993. doi: 10.3390/su12103993
Greenacre, M., Groenen, P. J., Hastie, T., d’Enza, A. I., Markos, A., and Tuzhilina, E. (2022). Principal component analysis. Nat. Rev. Methods Prim. 2:100. doi: 10.1038/s43586-022-00184-w
Greff, K., Srivastava, R. K., Koutník, J., Steunebrink, B. R., and Schmidhuber, J. (2016). LSTM: a search space odyssey. IEEE Trans. Neur. Netw. Learn. Syst. 28, 2222–2232. doi: 10.1109/TNNLS.2016.2582924
Grimmelt, A., Hong, S., Paula, R.U.D., Zhang, C., and Zhou, J. (2023). For love of meat: five trends in China that meat executives must grasp. Available online at: https://www.mckinsey.com/industries/consumer-packaged-goods/our-insights/for-love-of-meat-five-trends-in-china-that-meat-executives-must-grasp#/
Han, S., and Kim, H. (2021). Optimal feature set size in random forest regression. Appl. Sci. 11:3428. doi: 10.3390/app11083428
He, T., Xie, C., Liu, Q., Guan, S., and Liu, G. (2019). Evaluation and comparison of random forest and A-LSTM networks for large-scale winter wheat identification. Remote Sens. 11:1665. doi: 10.3390/rs11141665
Houkai, W. (2022). "China's rural development in the 14th five-year plan period." China Econ. 17:2–11. Available online at: http://www.chinaeconomist.com/pdf/2022/2022-1/Wei%20Houkai.pdf.
Jiang, L., Guo, S., Wang, G., Kan, S., and Jiang, H. (2020). Changes in agricultural land requirements for food provision in China 2003–2011: a comparison between urban and rural residents. Sci. Total Environ. 725:138293. doi: 10.1016/j.scitotenv.2020.138293
Jiang, S., Zhou, J., and Qiu, S. (2022). Digital agriculture and urbanization: mechanism and empirical research. Technol. Forecast. Soc. Chang. 180:121724. doi: 10.1016/j.techfore.2022.121724
Lee, C.-C., Zeng, M., and Luo, K. (2024). How does climate change affect food security? Evidence from China. Environ. Impact Assess. Rev. 104:107324. doi: 10.1016/j.eiar.2023.107324
Li, Y., Shi, Y., Deng, X., Sun, Z., and Accatino, F. (2024). Increasing food and feed self-sufficiency and avoiding manure N surplus in eastern regions of China through a spatial crop-livestock optimisation model. Agric. Syst. 217:103911. doi: 10.1016/j.agsy.2024.103911
Liaw, A., and Wiener, M. (2002). "Classification and regression by random Forest." R News, 2:18–22. Available online at: https://journal.r-project.org/articles/RN-2002-022/RN-2002-022.pdf
Liu, C.-H., Gu, J.-C., and Yang, M.-T. (2021). A simplified LSTM neural networks for one day-ahead solar power forecasting. IEEE Access 9, 17174–17195. doi: 10.1109/ACCESS.2021.3053638
Liu, Z., Ying, H., Chen, M., Bai, J., Xue, Y., Yin, Y., et al. (2021). Optimization of China’s maize and soy production can ensure feed sufficiency at lower nitrogen and carbon footprints. Nat. Food 2, 426–433. doi: 10.1038/s43016-021-00300-1
Niu, Y., Xie, G., Xiao, Y., Liu, J., Wang, Y., Luo, Q., et al. (2021). Spatiotemporal patterns and determinants of grain self-sufficiency in China. Food Secur. 10:747. doi: 10.3390/foods10040747
Parrini, S., Aquilani, C., Pugliese, C., Bozzi, R., and Sirtori, F. (2023). Soybean replacement by alternative protein sources in pig nutrition and its effect on meat quality. Animals 13:494. doi: 10.3390/ani13030494
Paul, B. K., Epper, C. A., Tschopp, D., Long, C. T. M., Tungani, V., Burra, D., et al. (2022). Crop-livestock integration provides opportunities to mitigate environmental trade-offs in transitioning smallholder agricultural systems of the greater Mekong subregion. Agric. Syst. 195:103285. doi: 10.1016/j.agsy.2021.103285
Rajão, R., Soares-Filho, B., Nunes, F., Börner, J., Machado, L., Assis, D., et al. (2020). The rotten apples of Brazil's agribusiness. Science 369, 246–248. doi: 10.1126/science.aba6646
Rauw, W. M., Gómez Izquierdo, E., Torres, O., García Gil, M., de Miguel Beascoechea, E., Rey Benayas, J. M., et al. (2023). Future farming: protein production for livestock feed in the EU. Sustain. Earth Rev. 6:3. doi: 10.1186/s42055-023-00052-9
Rauw, W. M., Rydhmer, L., Kyriazakis, I., Øverland, M., Gilbert, H., Dekkers, J. C., et al. (2020). Prospects for sustainability of pig production in relation to climate change and novel feed resources. J. Sci. Food Agric. 100, 3575–3586. doi: 10.1002/jsfa.10338
Reidy, J. (2023). China soybean imports top 100 million tonnes. Available online at: https://www.world-grain.com/articles/19251-china-soybean-imports-top-100-million-tonnes.
Roman, G. V., Epure, L. I., Toader, M., and Lombardi, A.-R. (2016). Grain legumes-main source of vegetal proteins for European consumption. AgroLife Sci. J. 5, 178–183. Available at: https://agrolifejournal.usamv.ro/index.php/agrolife/article/view/121.
Schwediauer, P., Hagmüller, W., and Zollitsch, W. (2018). Germination of faba beans (Vicia faba L.) for organic weaning piglets. Org. Agric. 8, 249–258. doi: 10.1007/s13165-017-0195-5
Sekaran, U., Lai, L., Ussiri, D. A., Kumar, S., and Clay, S. (2021). Role of integrated crop-livestock systems in improving agriculture production and addressing food security–a review. J. Agric. Food Res. 5:100190. doi: 10.1016/j.jafr.2021.100190
Sońta, M., Łukasiewicz-Mierzejewska, M., Puppel, K., Rekiel, A., Więcek, J., and Batorska, M. (2022). Influence of raw pea () or blue lupin seeds () on the level of selected bioactive substances in pork meat. Ann. Anim. Sci. 22, 701–709. doi: 10.2478/aoas-2021-0052
Sporchia, F., Kebreab, E., and Caro, D. (2021). Assessing the multiple resource use associated with pig feed consumption in the European Union. Sci. Total Environ. 759:144306. doi: 10.1016/j.scitotenv.2020.144306
SUSPIG. (2020). "Sustainability of pig production through improved feed efficiency." Available online at: https://era-susan.eu/content/suspig.
Świątkiewicz, M., Olszewska, A., Grela, E. R., and Tyra, M. (2021). The effect of replacement of soybean meal with corn dried distillers grains with solubles (cDDGS) and differentiation of dietary fat sources on pig meat quality and fatty acid profile. Animals 11:1277. doi: 10.3390/ani11051277
Tallentire, C., Mackenzie, S., and Kyriazakis, I. (2018). Can novel ingredients replace soybeans and reduce the environmental burdens of European livestock systems in the future? J. Clean. Prod. 187, 338–347. doi: 10.1016/j.jclepro.2018.03.212
Textor, C. (2024). "Meat production volume in China 2013-2023." Statista. Available online at: https://www.statista.com/statistics/275668/meat-production-in-china/.
Tian, G., Duan, J., and Yang, L. (2021). Spatio-temporal pattern and driving mechanisms of cropland circulation in China. Land Use Policy 100:105118. doi: 10.1016/j.landusepol.2020.105118
van Zanten, H. H., Bikker, P., Meerburg, B. G., and de Boer, I. J. (2018). Attributional versus consequential life cycle assessment and feed optimization: alternative protein sources in pig diets. Int. J. Life Cycle Assess. 23, 1–11. doi: 10.1007/s11367-017-1299-6
Wang, J., Liu, Q., Hou, Y., Qin, W., Lesschen, J. P., Zhang, F., et al. (2018). International trade of animal feed: its relationships with livestock density and N and P balances at country level. Nutr. Cycl. Agroecosyst. 110, 197–211. doi: 10.1007/s10705-017-9885-3
Wang, Z., Zhang, G., Xing, X., Xu, X., and Sun, T. (2024). Comparison of dimensionality reduction techniques for multi-variable spatiotemporal flow fields. Ocean Eng. 291:116421. doi: 10.1016/j.oceaneng.2023.116421
Wilke, V., Gickel, J., and Visscher, C. (2023). Monitoring of performance-based environmental impacts of substituting soybean meal with rapeseed meal in the Rye-based diet of weaned pigs. Sustain. For. 15:2210. doi: 10.3390/su15032210
Wilkinson, J., and Lee, M. (2018). Use of human-edible animal feeds by ruminant livestock. Animal 12, 1735–1743. doi: 10.1017/S175173111700218X
Wu, F., Geng, Y., Zhang, Y., Ji, C., Chen, Y., Sun, L., et al. (2020). Assessing sustainability of soybean supply in China: evidence from provincial production and trade data. J. Clean. Prod. 244:119006. doi: 10.1016/j.jclepro.2019.119006
Yang, S., and Cui, X. (2023). Large-scale production: a possible way to the balance between feed grain security and meat security in China. J. Agric. Food Res. 14:100745. doi: 10.1016/j.jafr.2023.100745
Yang, C., Jiang, X., Du, H., Li, Q., Zhang, Z., Qiu, M., et al. (2021). A review: achievements and new obstacles in China’s food security revealed by grain and animal meat production. IOP Conf. Series 705:012025. doi: 10.1088/1755-1315/705/1/012025
Yu, C., Zou, J., Fang, X., Wang, J., Cao, Y., and Li, G. (2020). Effect of land tenure fragmentation on the decision-making and scale of agricultural land transfer in China. Land Use Policy 99:104996. doi: 10.1016/j.landusepol.2020.104996
Zhang, J., Wu, Q., Liu, C., Xiong, S., Yang, H., Chang, S., et al. (2024). "Enhanced crop LAI estimation via random Forest regression: Bayesian optimization and feature selection approach." Available at: https://www.researchsquare.com/
Zhang, X., Zhang, Q., Zhang, G., Nie, Z., Gui, Z., and Que, H. (2018). A novel hybrid data-driven model for daily land surface temperature forecasting using long short-term memory neural network based on ensemble empirical mode decomposition. Int. J. Environ. Res. Public Health 15:1032. doi: 10.3390/ijerph15051032
Details about data collection are illustrated in the Table A1.
Keywords: soybeans, pig farming, feed grains, meat security, China
Citation: Zhao Z, Fu Y, Wang H, Ahmad B and Jin F (2025) Optimizing soybean-pig integration zones in China: a multi-algorithm approach using random forest, LSTM, and clustering for feed grain and meat security balance. Front. Sustain. Food Syst. 9:1488994. doi: 10.3389/fsufs.2025.1488994
Received: 31 August 2024; Accepted: 06 March 2025;
Published: 24 March 2025.
Edited by:
Baohua Wang, Nantong University, ChinaReviewed by:
Justice Gameli Djokoto, Dominion University College, GhanaCopyright © 2025 Zhao, Fu, Wang, Ahmad and Jin. This is an open-access article distributed under the terms of the Creative Commons Attribution License (CC BY). The use, distribution or reproduction in other forums is permitted, provided the original author(s) and the copyright owner(s) are credited and that the original publication in this journal is cited, in accordance with accepted academic practice. No use, distribution or reproduction is permitted which does not comply with these terms.
*Correspondence: Yuanjing Fu, ZnV5dWFuamluZzE3QGdtYWlsLmNvbQ==; Haiyan Wang, d2FuZ2hhaXlhbkBqaXN1LmVkdS5jbg==
Disclaimer: All claims expressed in this article are solely those of the authors and do not necessarily represent those of their affiliated organizations, or those of the publisher, the editors and the reviewers. Any product that may be evaluated in this article or claim that may be made by its manufacturer is not guaranteed or endorsed by the publisher.
Research integrity at Frontiers
Learn more about the work of our research integrity team to safeguard the quality of each article we publish.