- 1Leibniz Centre for Agricultural Landscape Research (ZALF), Müncheberg, Germany
- 2Faculty of Life Sciences, Thaer-Institute of Agricultural and Horticultural Science, Humboldt University of Berlin, Berlin, Germany
This review focused on the inventory of current digital technologies available on the agricultural market in Germany. A total of 189 digital technologies were found as of December 2023. Digital technologies in agriculture rarely contain few components. They consist of various other technologies that have many common interfaces. Therefore, a classification on two levels was done: technologies categorized according to their type (software-based and hardware-based technologies) and mode of operation (farm management information systems/ decision support systems, digital technologies for guidance and steering, digital information platforms, citizen science applications and platforms, sensors, field robots and unmanned aerial vehicles). Furthermore, the expected potentials of these digital tools for the promotion of nature conservation and ecosystem service provisioning in Germany were framed. The review also discusses barriers that can impact nature conservation and ecosystem service provisioning. Germany, as one of the world’s leading nations in the production and use of modern technologies, had set ambitious goals regarding digitalisation as a solution for nature conservation and ecosystem service provisioning problems, which have not yet been fulfilled. The potentials for nature conservation and ecosystem service provisioning are still strongly supressed by non-sustainable barriers, e.g., high acquisition costs, practical maturity, mode of operation and infrastructure. Current policies and societal preferences are not yet contributing enough to steer the use of digital technologies in a direction of nature conservation and providing ecosystem services. Furthermore, the main participants in the digitalisation discussion are researchers, whereby the smallest group of participants are farmers. For a sustainable digital transformation of agriculture, including restoration and protection targets of nature, and ecosystems, more wide-ranging, and diversifying changes supported by digitalisation are needed along agricultural and ecological concepts leading to long-term resilience of agricultural systems.
1 Introduction
Digitalization within agriculture is already being used to optimize procedures and processes (Hennes et al., 2022). Digital technologies offer new opportunities that can facilitate coordination among different stakeholders (Kliem et al., 2023; WEF, 2020). Due to rapid digitalization progress, technological innovations can significantly increase resource use efficiency and reduce for instance greenhouse gas (GHG) emissions (Basso and Antle, 2020; Finger et al., 2019). However, nature conservation and ecosystem service provisioning is currently not a primary goal of digitalization in agriculture, which is mainly used as a yield-increasing and effort-reducing tool, hence for the production and economic optimization (Kliem et al., 2023; Techen and Helming, 2017). However, the productivity increase in agriculture is often accompanied by significant environmental impacts.
Characterized by fertile soils and favorable climate conditions – moderate temperatures and sufficient precipitation – Germany is a prime location for conventional and organic agriculture in Europe. For hundreds of years, agricultural land use has shaped the landscape in Germany and has created a unique cultivated landscape with distinct ecosystems (ZKL, 2021). The complexity of nature conservation in agriculture in Germany is currently the focus of public discussion and different scientific disciplines. Since 1970, land-use change in agriculture has had the largest negative impact on the environment, in particular biodiversity (IPBES, 2019). The diversity of floristic and faunistic species in Germany continues to decline. Of the 97 mammalian taxa assessed in Germany, 30 are listed as endangered, including well-known species such as the brown hare (Lepus europaeus) (Meinig et al., 2020). A considerable proportion of a wide range of insect species in Germany are affected by long- and short-term population declines (Deutsche Akademie der Naturforscher Leopoldina et al., 2020; Ries et al., 2019). The population situation of every third bird species in Germany has declined noticeably since the end of the 1990s (BfN, 2015).
Furthermore, ecosystems have been so severely damaged that their ability to provide beneficial services for humans and society has drastically decreased (Millenium Ecosystem Assessment, 2005). An EU-wide analysis of agroecological indicators showed no substantial changes made in agriculture in the past 10 years to reduce the use of chemicals and intensification (Maes et al., 2020).
However, changes in land use, pesticide application, fertilizer use, and crop rotation can contribute to the conservation of nature ecosystem services in agricultural landscapes. The reduction of synthetic pesticides, in particular those containing hazardous compounds, can make a decisive contribution to the protection of, e.g., soil and species richness biodiversity on arable fields (European Environmental Agency, 2015; Kumar et al., 2021; Uwizeyimana et al., 2017). The diversification of crop varieties and species as well as the cultivation of mixed, cover crops and flowering fields also contribute to agrobiodiversity conservation (Elhakeem et al., 2019; Fiorini et al., 2022; Gayer et al., 2021).
Although legal frames as well as strategies and action plans for biodiversity protection exist, their implementation has been incomplete and insufficient (European Commission, 2015). Land use options offered by digitalization in agriculture promoting nature conservation have been set as a goal in Germany (Deutscher Bundestag, 2019). Digitalization of agricultural management gives rise to new, different challenges and risks, such as rebound effects assessing the energy efficiency of digital tools (Golde, 2016; Madlener and Alcott, 2011; Weller von Ahlefeld, 2019).
Germany is one of the world’s leading nations in the production and use of modern technologies. With help of digitalization, the competitiveness of German agriculture can be advanced (Bundesministerium für Ernährung und Landwirtschaft, 2022). Therefore, this study aims to present (1) an extensive list of current digital technologies available on the agricultural market and for nature conservation purposes in Germany, (2) a simplified categorization of digital technologies in agriculture, that reflect their mode of operation, and (3) frame the expected impacts of digital technologies as potentials, barriers and risks for the promotion of nature conservation and ecosystem service provisioning (NCES) in Germany. The 7 categories are assigned to their utilization in agriculture. The review of technologies from all 7 categories covers biotic as well as abiotic impacts. The potentials review following aspects: mitigation of greenhouse gas (GHG) emissions, improvement of nitrogen (N) use efficiency, reduction of pesticide pollution, diversification of crop species and crop rotations, calculating and mapping for NCES purposes, improvement of soil management, climate change management, innovative cropping systems as well as communication and knowledge-sharing. The review of barriers and risks is based on economic, administrative, and legal barriers as well as limitations, maturity level of digital technologies in practice, risks for non-NCES targets, further intensification, cyber security and trade-offs. All aspects are assessed including critical insights on digitalization toward NCES in agriculture.
2 Methodology, conceptual background, and definitions
This manuscript is a sub-study of the scientific project under the technological report Geppert et al. (2024a). The project included a review of digital technologies, an expert discussion as well as literature assessment with authors’ insights on the current use of digital technologies for NCES and a questionnaire of farmers. The NCES indicators used in this review were compiled in 2022 to 2023 and presented in 2023 in a German technical report (Kliem et al., 2023). In the following NCES study the authors also drew own critical conclusions about possible impacts to shape sustainable agriculture. The integration of ecosystem services aimed at increasing public awareness about NCES as means to strengthen NCES providers. Farmer’s perceptions assessed by a questionnaire and an expert discussion toward NCES from this project were investigated in Geppert et al. (2024b) within the same project, but are not handled in this manuscript.
2.1 Identification of digital technologies, potentials, barriers, and risks in agriculture
The basis of the digital technology categories and most relevant potentials, barriers, and risks for NCES were presented in the technical report of Kliem et al. (2023) as a preliminary project. Therefore, that basis of categories and indicators was used and further developed and compiled for this study. The literature search consisted of four rounds and an expert discussion.
The first selection of digital technologies (“Identification,” Figure 1) of the academic research was done by means of appropriate article abstracts that deal with the review topic between November 2022 and December 2023. Technologies that solely aim at the economic optimization of agricultural production and administration were not considered. Digital technologies used for indoor livestock management and farming were excluded (e.g., milking, cleaning, and feeding robots). A few virtual fencing sensor technologies were incorporated into the study because of their relevance to NCES. Technologies which are available in German language but only on the market for farmers in Austria and Switzerland, were sorted out. From a total of 993 peer-reviewed articles on agricultural-related digitalization and internet search, an extensive list of technologies with a total of 189 entries was compiled (Figure 1; see Supplementary materials).
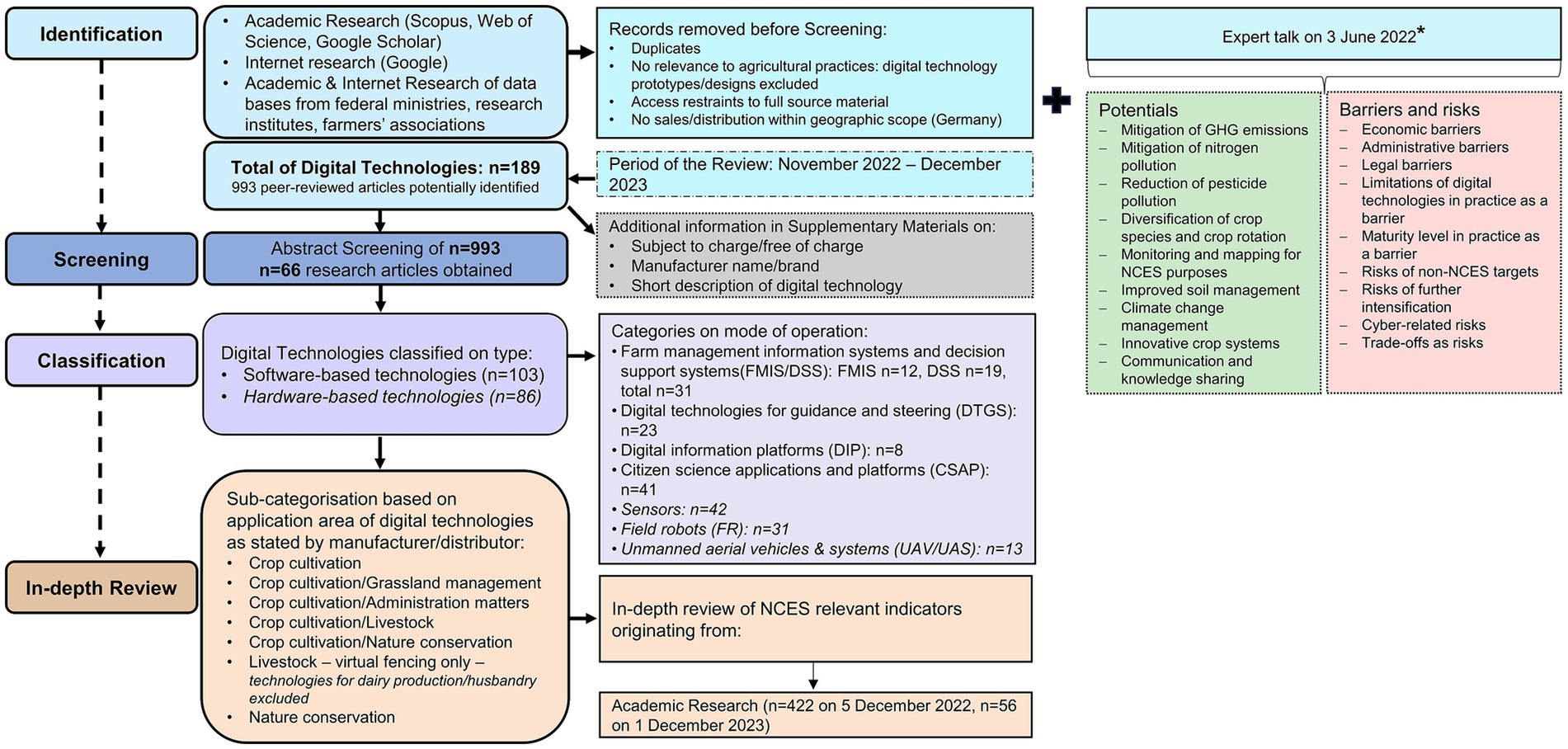
Figure 1. Literature review and internet search process for the identification of currently commercially available digital technologies in Germany.
Considering that many of the commercially available digital products have been investigated in research on a limited scale or not at all, an internet search via Google was used to complement the list of technologies (“Identificaition, Screening,” Figure 1). In the next step, the data portals of ministries, authorities, agricultural research institutes, and associations of the individual federal states in Germany were browsed. Individual search queries were performed for each technology group in both English and German. Additionally, in Supplementary materials we presented the most important information on each technology, manufacturer, availability, and application areas.
At the time the review was conducted, there was a boom of announcements and abstracts from technology manufacturers about new products, research projects, and case studies on digital technologies that could support NCES. However, in most cases no further information on NCES indicators was disclosed or the research projects were in the early stages and no results were available. Therefore, we did not compile an empirical impact or a comparative analysis of scenarios from real-world NCES oriented digital technology use in regions within Germany.
Projected potentials in this manuscript refer to potentials not yet practiced on a large scale, which, however, were outlined, during the study, as great opportunities for NCES-related measures in agriculture in general.
The NCES indicators were additionally compiled after an expert talk conducted in June 2022 (“In-depth Review,” Figure 1), whereby experts from agriculture, research, policy making, industry and non-profit organizations were asked to describe current NCES issues regarding digital technologies in Germany. This expert talk was used as a screening of the situation on NCES potentials, barriers, and risks through digitalization and used for the further search. The talk was done online with a total of 23 experts (eight researchers, four farmers, four policy makers, four representatives from civil society and three technology developers from industry). A table with the results of this expert talk is available under Supplementary materials.
Afterwards, the projected potentials, barriers, and risks were reviewed with additional keywords “Sustainability” and “Nature Conservation.” Overall, the results of relevant potentials, barriers, and risks were compiled in NCES indicators as listed under Figure 1. We also included additional information from the screening process of websites of technology manufacturers, which cannot be classified with keywords. We used relevant information and examples of technology manufacturers in the assessment of potentials, barriers, and risks.
2.2 Digital technologies’ classification
For this study, two classifications related to the original mode of operation of the technologies listed, were elaborated in a first step: software- and hardware-based technologies (Figure 1). The categories were expanded to a total of 7 in a second step after the literature review: (1) Farm management information systems and decision support systems (FMIS/DSS); (2) Digital technologies for guidance and steering (DTGS); (3) Digital information platforms (DIP); (4) Citizen science applications and platforms (CSAP); (5) Sensors; (6) Field robots (FR) and (7) Unmanned aerial vehicles and systems (UAV/UAS) (Figure 1). Figure 2 schematically illustrates the hierarchy of the digital technologies’ categories in this study toward machine learning (ML), artificial intelligence (AI) and Big Data (BD). In the middle are ML and AI, which are the cornerstones for the extensive functions of software-based technologies. ML and AI are independent concepts, whereby the ML configurations have hardly any points of contact with the everyday life of farmers – it is a task for computer and mathematics experts, who rely on the data generated by farmers (De Jong and De Boer, 2009). Farmers provide the input for the ML algorithms, but they do not design these algorithms themselves. BD is a term for the processing of very large and heterogeneous data volumes at high speed. Therefore, it is combining both ML and AI as predictive analytics and as an important component of business intelligence (Bhat and Huang, 2021).
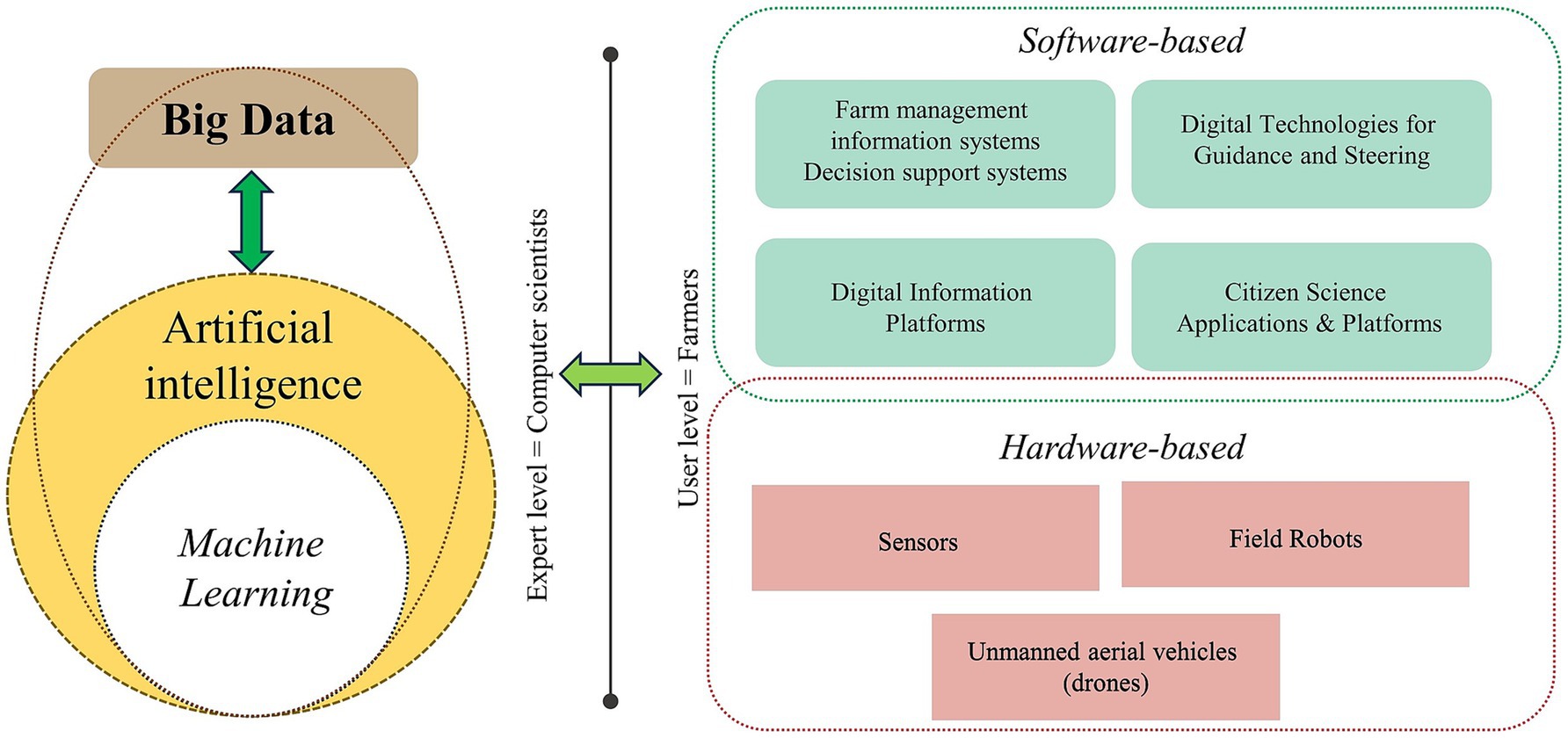
Figure 2. Relationships between Big Data (BD), artificial intelligence (AI), and machine learning (ML) in the context of software- und hardware-based digital technologies.
2.2.1 Software-based digital technologies
We sorted the software-based technologies into 4 sub-categories (Table 1). The main difference between farm management information systems (FMIS) and decision support systems (DSS) is the group of people targeted by the technologies within a farm or company. FMIS optimize the farm as a whole and not just parts of it – this is achieved by offering everything on one platform – including crop and livestock management, machinery management, payroll and administrative work and reports, as well spatial and temporal management (Henningsen et al., 2022; Streimelweger et al., 2020). DSS support decision-makers by identifying information for operational and strategic tasks. Therefore, DSS are used in the production process. We explicitly used the original definition of FMIS and categorized software, which is referred to as an “electronic field diary” or a “digital field index,” as DSS (Geppert et al., 2024a). The list of Digital technologies for guidance and steering (DTGS) does not go in detail about the hardware of steering systems (e.g., touchscreen monitors, tablets, steering wheel motors, antennas, steering angle sensors or cable harnesses). In some cases, the offered DTGS technology is combined with hardware. Digital information platforms (DIP) serve as intermediaries between different stakeholders, for example, as a simple supply–demand relationship. DIP can provide data for various applications that can be applied and developed at a higher software level, such as FMIS/DSS. Data from Citizen science applications and platforms (CSAP) can be used to develop and enhance ML algorithms. CSAP platforms and apps support innovations and promote new learning as an important source of data for science (Koffler et al., 2021).
2.2.2 Hardware-based digital technologies
We sorted the hardware-based technologies into 3 sub-categories (Table 2). Sensors are often used in combination with robotics (Bellon Maurel and Huyghe, 2017; Tansey et al., 2009). Passive sensors reflect sunlight and cannot emit any radiation of their own. Their measurements take place in the visible and infrared range of the electromagnetic spectrum (Erdle et al., 2011). Active sensors can emit radiation and receive it at the same time. They transmit radiation in the microwave range (Erdle et al., 2011). A field robot (FR) in agriculture also refer to semi-autonomous and fully autonomous machines that work with the help of AI-algorithms. FR are associated with improved efficiency for specific tasks as well as for overall performance (Ghobadpour et al., 2022). The size of Unmanned aerial vehicles (UAV) (also known as unmanned aerial systems (UAS) and drones) can vary a lot (Kardasz and Doskocz, 2016). The basic element of a drone is a frame, which should be very light. The number of arms and the motors of a drone can be divided into different categories, e.g., bicopters = two engines, octocopters = eight engines (Kardasz and Doskocz, 2016).
3 Results and discussion
3.1 Digital technologies commercially available on the market in Germany
3.1.1 Software-based technologies
Figure 3 shows the digital technologies by sub-category that were identified in the review process. Extensive lists with additional information are available in the Supplementary materials.
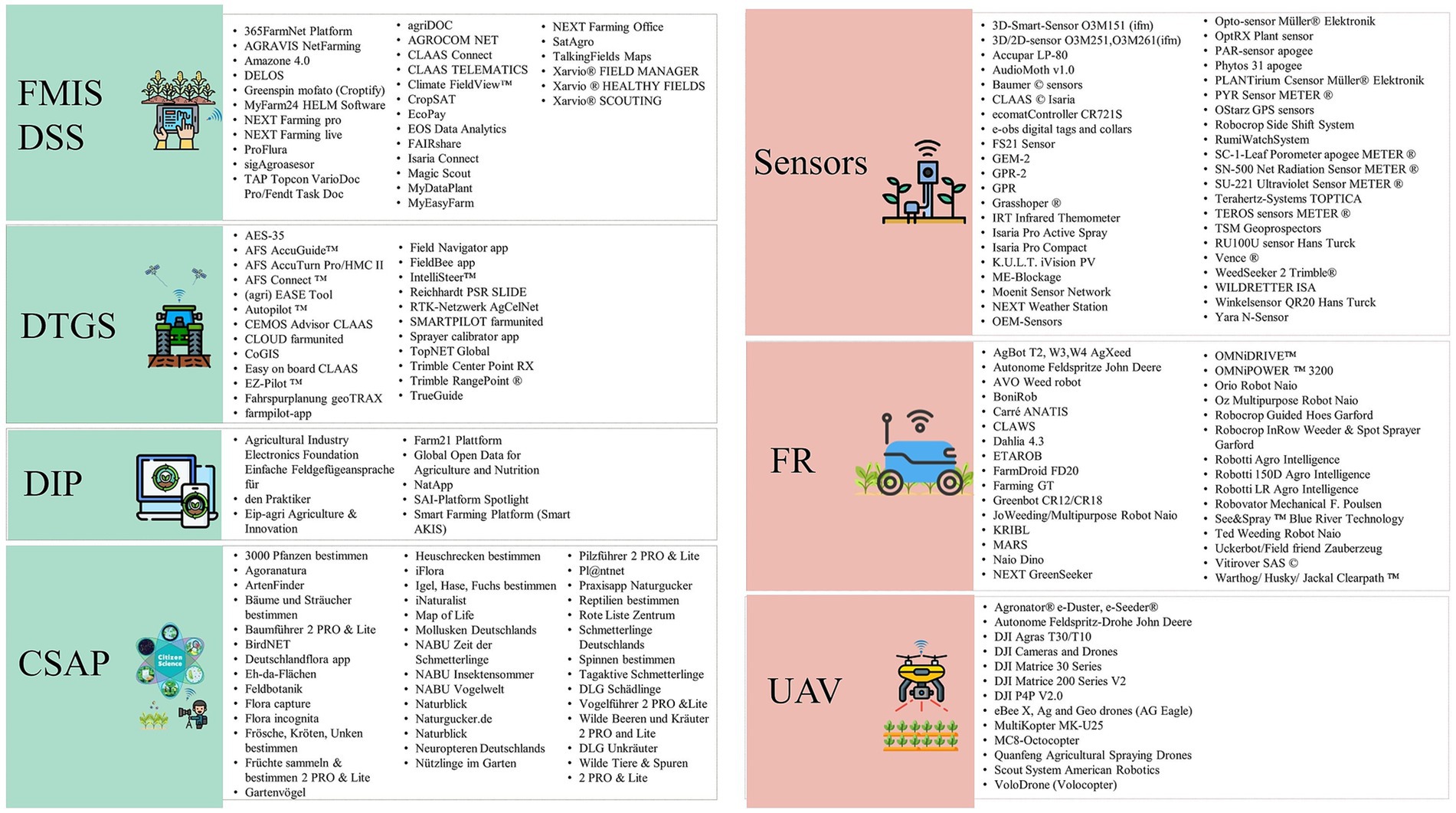
Figure 3. Digital technologies commercially available for farmers in Germany by mode of operation. The total of digital technologies is n = 189. FMIS, Farm management information systems; DSS, decision support systems; DTGS, digital technologies for guidance and steering; DIP, digital information platforms; CSAP, citizen science applications and platforms; FR, field robots; UAV, unmanned aerial vehicle.
By giving a few explicit examples, we highlighted the technologies’ characteristics and identification criteria. The review showed that FMIS and DSS providers develop both mobile and web applications (Figure 3). DSS developed in cooperation with science (CropSAT and EcoPay) and DSS for simpler tasks (Magic Scout) are free for farmers but offer only limited functions. FMIS and DSS cannot be developed in a general and uniform way for small- to large-scale farms because of the great variety of farm needs and goals (LfULG, 2020).
Trimble® CenterPoint RTX is a DTGS for precise point positioning with high level-accuracy (2.5 cm = 95%) delivered via satellite or cellular/IP (Rodriguez-Solano et al., 2019). Applications such as FieldBee or FieldNavigator work with software that adds routes to existing map material. DTGS are still being constantly expanded and their user-friendliness is further developed (Lundström and Lindblom, 2018).
SMART AKIS is a DIP for farmers, which collects existing knowledge and application examples of practices that are about to be established (www.smart-akis.com). Furthermore, it converts academic and practical knowledge into easily understandable information for farmers, e.g., recommendations, or brief instructions. The material is permanently available online and shared on the platform eip-agri, too. SMART AKIS is for the entire EU. However, it is not regularly updated. During our literature review, we found that many of the technologies presented as “available on the market” no longer existed. We could not derive digital technologies from the SMART AKIS platform for our technology list for any of the categories.
Most CSAP databases have a spatial reference from geo-tagged photographs or location information from a smartphone. CSAP data collection by citizens may take place in hard-to-reach locations, which is an advantage compared with traditional data sources. CSAP comprises denser and more frequent observations as well as a diversity of subject areas (Fritz et al., 2019). CSAP supports research regarding the influence of agricultural production, land use, and agricultural change on biodiversity (Frigerio et al., 2021). Most well-known CSAP projects in Germany are supervised by experts. The large interactive CSAP platforms Deutschlandflora and The German Red List Center are directly funded and managed by the Federal Agency for Nature Conservation. NABU (Naturschutzbund Deutschland e.V.) has various platforms and apps that can collect data. The well-known Naturgucker app organizes observation competition events to attract as many participants as possible.
3.1.2 Hardware-based technologies
The most famous sensors in agriculture are aimed at optimizing crop yields, for example by using the reflectance (e.g., Yara N-Sensor and Isaria) of crops to provide information on the chlorophyll content for fertilizer reduction (Bogue, 2017; Reckleben, 2014). Yield potential maps with multi-year images of areas during the vegetation period are necessary as background information for the correct calibration of the sensors. The virtual fencing collars Vence® guide, track, and monitor livestock.1 There is still insufficient research on virtual fencing regarding the welfare of livestock (Waterhouse, 2023).
Although field robots are currently being researched and tested in field conditions, the dynamic development in the past 10 years and especially currently suggests that in the next 10 years, field robots will probably be seen working in fields more often. The FR FarmDroid (FD20) operates with four photovoltaic modules that generate the electricity to move and work. FD20 performs sowing and weeding in different crops such as sugar beets, onion, spinach, kale, flowers, and rapeseed. Bonirob is a multipurpose FR with different application modules (Goettinger et al., 2014; Schwich et al., 2018). BoniRob can achieve a control rate of 97% in the intra-row area (Langsenkamp et al., 2014). The K.U.L.T Robovator from KRESS is a vision-based robot for mechanical weeding. It uses hoeing blades that move in and out of the crop row as a crop plant passes, to remove weeds (Lati et al., 2016). Similarly, the Robocrop InRow Weeder from Garford relies on video-image-analysis (machine vision) to determine the positions of individual crop plants in order to then remove the weeds mechanically from between and within the crop rows (Fontanelli et al., 2015; Hemming et al., 2018; Muscalu et al., 2019). The French company Naïo Technologies has developed the small electric OZ weeding robot mainly for asparagus producers, small-scale farms, and greenhouses equipped with comb harrow, brush, and a trailer (Epée Missé et al., 2020; Robert et al., 2020).
The use of drones in agriculture is currently increasing and is mostly associated with data collection. Probably the best-known use of drones in agriculture, directly related to NCES, is the rescue of fawns. In Germany, various animal welfare associations and hunters are already working on a voluntary basis every year in active cooperation with farmers to organize and actively use private drones with thermal cameras to reduce the mortality of fawns during mowing operations, saving up to 100.000 animals a year (Artmann, 2021; Gehrke, 2021; Pohle, 2021; Van Bevern, 2021; WDR, 2021).
3.1.3 Employment of digital technologies
The results of the research showed that, in total, the share of software-based digital technologies is higher compared with the total of hardware-based technologies. A closer look at the shares of the total numbers of the sub-categories showed that Sensors with 22.2% and CSAP with 21.7% had the highest share (Figure 4A). FMIS/DSS and FR had with 16.4% equal shares, while UAV/UAS and DIP shared the last two places. As mobile tools, sensors are used in the reduction of fertilizers and pesticides as well as in the determination of forage quality traits (Ali et al., 2017; Duckett et al., 2018). In 2020, less than 7% of the German farms were using sensors (Gabriel and Gandorfer, 2020), in 2023, the figure increased slightly to 9%, whereby 69% of the surveyed farmers stated they do not plan to use sensors (Geppert et al., 2024a). CSAP are often accessible free of charge and usually involve unpaid volunteers in the data collection process (Koffler et al., 2021), which is why their proportion is high. The application of FMIS is partly cost-intensive and often complex, which is why their use is still limited (Munz et al., 2020).
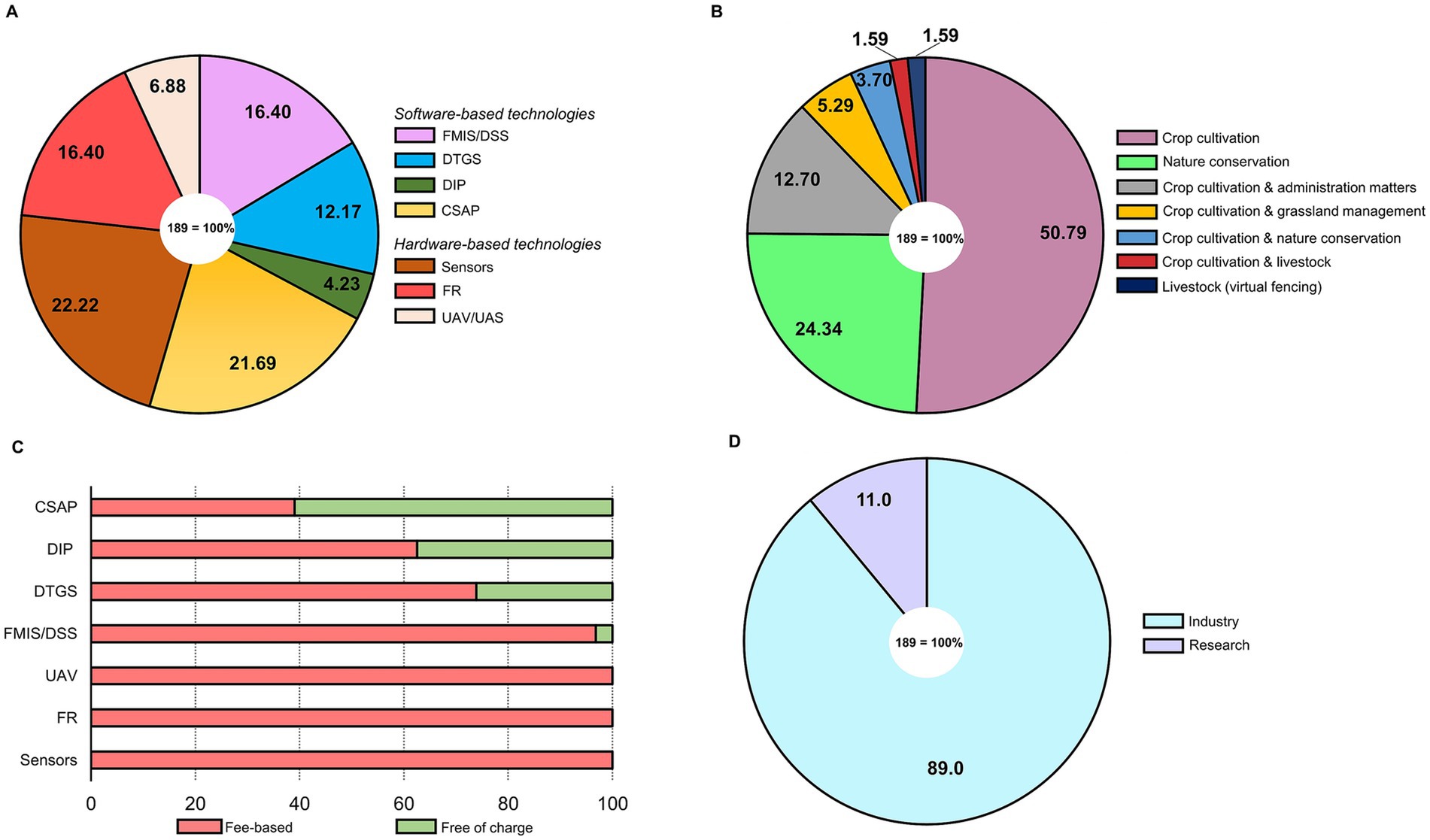
Figure 4. Share [%] of digital technologies by (A) categories (mode of operation). (B) sub-categories (application area). (C) fee-based and free of charge digital technologies by category. (D) digital technologies from industry and from cooperations of research and industry. The total of digital technologies n = 189 equals 100%.
With the application area of the digital technologies, 50.8% of the technologies were only used for crop cultivation, the other 24.9% of the technologies had combined features and 22.3% focused on nature conservation (Figure 4B). At 12.7%, the proportion of crop cultivation and nature conservation was the highest compared with the other listed combinations. Although crop cultivation and nature conservation had the highest proportions, the combination of both was less represented at 3.7%. CSAP are the only technologies entirely directed at nature conservation in agricultural landscapes Citizens can participate in data collection projects in agriculture and promote NCES. All other technologies primarily aim at crop cultivation. Technology providers react with their products to the requests of farmers, which currently prioritize crop cultivation facilitation than NCES. Detailed lists of the technologies are available as Supplementary material.
Figure 4C shows that most of the technologies are fee-based. While there were some free usage options in the software-based classification, the use of hardware is 100% chargeable. The majority of CSAP is available free of charge at just over 60%. In the case of DIP, state-subsidized platforms, such as eip-agri Agriculture and Innovation and Smart AKIS, are free of charge. However, most DIPs are offered by technology manufacturers as a fee-based service (Figure 4C). A modest share of DTGS and FMIS are available free of charge, but these applications are designed for simplified tasks, e.g., Sprayer calibrator or FieldBee app.
The search also showed that 89% of digital technologies were developed by industry (Figure 4D). These include sensors, FR, UAV, FMIS/DSS and DTGS. Industry is also developing DIP for farmers. Solely 11% of the technologies were developed in research in cooperation with industry. Large CSAP in Germany are scientifically supervised and used for further research.
3.2 Assessment of digital technologies: projected potentials
The potentials are presented in two levels in Table 3. In the first section of Table 3, the projected potentials are listed as the top category. In the sub-category, the digital technologies that could be assigned to the potentials are listed by mode of operation.
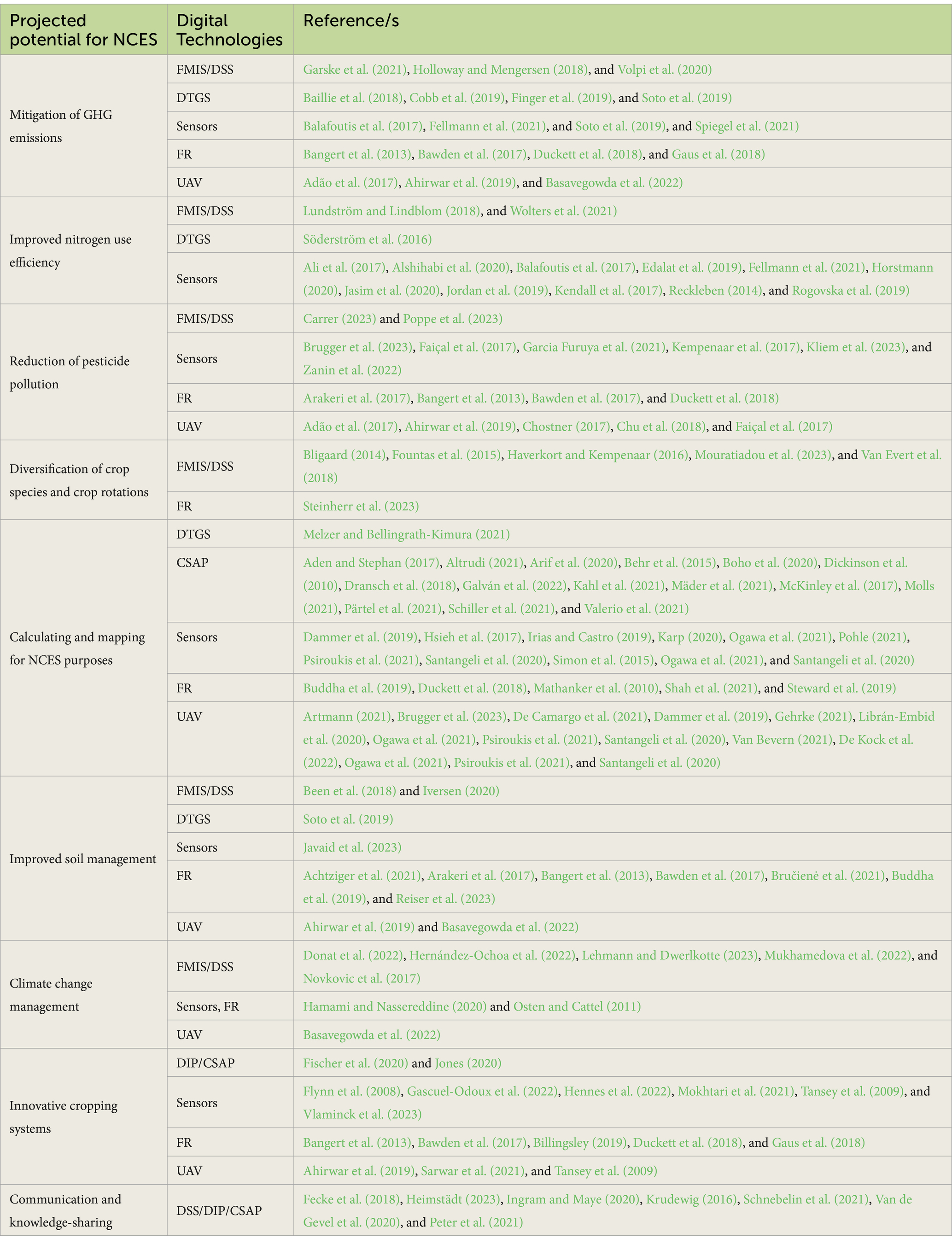
Table 3. Overview of projected potentials for NCES implementation of digital technologies by category.
The mitigation of GHG emissions from crop cultivation can be supported by digitalization (Soto et al., 2019). DSS can predict and proceed information on nitrous oxide and carbon dioxide emissions from different cropping systems and help farmers with crop management (Volpi et al., 2020). DTGS and sensors can have a positive impact on NCES through a reduction of fuel consumption (Cillis et al., 2018), which can lead to 0.3 to 1.5% less carbon dioxide of the total GHG emissions of the EU in agriculture (Soto et al., 2019). Germany is ranked second in the EU for successfully reducing GHG emissions through the use of digital technologies (Soto et al., 2019) and was ranked with high carbon dioxide mitigation potentials at relatively low costs (Fellmann et al., 2021).
Sensors offer site-adapted fertilizer application through chlorophyll measurements for improved nitrogen (N) use efficiency (Ali et al., 2020; Edalat et al., 2019; Noack, 2018; Rogovska et al., 2019; Spiegel et al., 2021). If crops are fertilized according to their nutrient requirements, less surplus N is released into the environment and causes less negative impacts, such as GHG emissions (especially during soil cultivation after harvest) (Haas et al., 2022; Simionescu et al., 2019). Furthermore, nitrous oxide emissions and nitrate leaching can be reduced through time-adjusted fertilizer application by means of sensor-based data (Alshihabi et al., 2020; Fellmann et al., 2021).
Site-adapted or reduced application of pesticides also represent a NCES approach. Sensors, FR, and drones work at the plant level: they use an own camera or newer models a data source (e.g., satellite imagery and AI) that can differentiate crop plants from weeds or healthy from pest-infested crop plants. Sensors also detect fertilizer requirements and water stress in plants to support site-adapted management for healthy plant stands. Site-adapted pesticide application relies mainly on DTGS. Reduced pesticide application shows favorable NCES-related effects (Kuhn et al., 2022; Ludwig-Ohm et al., 2023). DSS can recommend the optimal herbicide rate and time of application (Van Evert et al., 2017). Sensor-assisted applications can reduce the amount of herbicide by 20 to 40% (Kempenaar et al., 2017; Kliem et al., 2023).
Planning a crop rotation is a time-consuming process in which many different aspects must be considered: previous crops, the plant-available mineral content of N in the soil, the soil characteristics of the site, weather conditions, pests, and disease, among others. As planning must take place separately for each field, digital technologies can facilitate the process by collecting and partially analyzing the necessary data to decide which crops to plant to diversify crop species and crop rotations. FMIS/DSS help farmers to choose the optimal site-specific crop rotation in potato cultivation based on data and laboratory analysis of soil sampling (Haverkort and Kempenaar, 2016; van Evert et al., 2018).
UAVs offer high-definition image-processing in combination with object and pattern recognition, which can be enhanced, restored and analyzed for calculating and mapping of NCES (Da Silva and Mendonça, 2005; Patrício and Rieder, 2018). Drones’ most important usage is in weed detection, detection of nutrient and water stress, mapping and management (Boursianis et al., 2022; Tsouros et al., 2019). Other applications include predicting of crop development, yield and plant health (Brugger et al., 2023; Tsouros et al., 2019). Multispectral images from sensors can also be useful for calculating and assessing agroecosystem services (Gascuel-Odoux et al., 2022). Although weeds are reluctantly tolerated among crops, they should be considered a direct measure for biodiversity conservation (Steinmann, 2020). In this sense, a direct contribution to NCES is also possible with the help of field robots: they can distinguish between crop plants and other plants when a certain degree of ML is reached (Mathanker et al., 2010; Steward et al., 2019). If a sufficient database associated with NCES becomes available, FR will be able to contribute to direct biodiversity conservation by targeting endemic and protected plants and sparing weeds for insect feeding during mechanical weed control. UAVs can be successfully used in wildlife ecology, especially to observe bird species and their nests (Ogawa et al., 2021; Santangeli et al., 2020), to detect mammals (De Kock et al., 2022; Psiroukis et al., 2021) and indicator plants of, e.g., high nature value (Basavegowda et al., 2022). By combining FMIS with other hardware-based technologies, farm management and system planning can be used to contribute to nature conservation (Mouratiadou et al., 2023).
FMIS/DSS, DTGS, FR and sensors can help farmers to improve soil management. Measures for soil protection and preparation are closely linked to the mitigation of GHG emissions (Fellmann et al., 2021). DTGS and sensors can help farmers to adapt and implement precise tillage management (Gabriel and Gandorfer, 2022; Kliem et al., 2023). Sensors and FMIS/DSS process large datasets that include numerous variables, such as soil temperature, soil humidity, weather, and crop plants, and can structure the results as recommendations to reduce soil defects (Javaid et al., 2023). Mechanical lightweight FR weed control can significantly increase soil bulk density in the topsoil layer compared with conventional heavy agricultural machinery (Bručienė et al., 2022).
The major climate challenges farms are facing in Germany are increasing winter precipitation along with a higher risk of erosion and nutrient leaching, an increase in dry periods during the main growth stage, and heavy rainfall events that lead to soil erosion and flooding’s (BMEL, 2022b). Climate change management includes a change in crop rotation, e.g., with the help of crop managing through FMIS/DSS (Mukhamedova et al., 2022; Novkovic et al., 2017) and landscape diversification (Donat et al., 2022; Hernández-Ochoa et al., 2022). Adaptation actions such as improved soil organic carbon management can also have mitigation co-benefits (Lehmann and Dwerlkotte, 2023). Any adaptation measures that increase the resilience of NCES to climate change – for example, reduced fragmentation or extending natural habitats – can allow species to persist (IPCC, 2023).
Digital technologies cannot stop climate change alone, but they can help farmers implement innovative cropping systems. The digital platform AgoraNatura2 aims at enabling anyone who manages land and wants to implement a nature conservation project to finance it via crowdfunding or through partnerships with companies. Private investors and companies can specifically promote biodiversity and nature services by purchasing nature conservation certificates. The price of a single certificate (as a donation for a certain project) is between 3 and 20 euros. The donations will be used for the development of, e.g., an herb- and species-rich grassland and for the selective introduction of important plant species in areas with open patches of soil until 2027 (Geppert et al., 2024a). The Uckerbot field robot has been developed in cooperation between industry and research. It is a system that promotes ecological sugar beet cultivation under unfavorable soil conditions while simultaneously supporting weed biodiversity. The weed diversity on the field is examined from the beginning of the field robot development as an adaption measure to poor soil conditions (Steinherr et al., 2023). Furthermore, FR can work 24 h a day, 7 days a week, allowing farmers to adopt diverse small-scale agroecological-friendly approaches (Daum, 2021). CSAP and DIP are particularly relevant for NCES, as biodiversity indicators and the diversity of flora and fauna must be first recorded and assessed before NCES can begin and lead to profound decision-making (Fischer et al., 2020; Jones, 2020).
The communication and knowledge-sharing among different stakeholders are important for the development of new digital tools and the collection and analysis of data for NCES (BMEL, 2022a). New learning opportunities emerge in the context of agricultural knowledge and innovation systems (Ingram and Maye, 2020), where communication and interaction between different actors is a crucial component to push innovation processes (Knierim et al., 2015; Van de Gevel et al., 2020). These actors can be individual farmers, whole farms, and extension services for farmers. Knowledge sharing is necessary in the same environment (e.g., in same the geographical region) as well as at the national and EU levels. A survey from Germany showed that 89% of the participating farmers use smartphone in their daily life and work, with 79% of them agreeing that mobile and digital communication will make it easier to check farm workflows (Fecke et al., 2018). Farmers want to show society that they are improving their skills and are willing to profit from all new digital technologies and information in order to improve their production and sustainability (Schnebelin et al., 2021). With the help of DIP experts can supply farmers, e.g., with knowledge on crop management options excluding or mitigating the use of pesticides (Heimstädt, 2023).
3.3 Assessment of digital technologies: projected barriers and risks
In this section, in contrast to the potentials (Table 3), the barriers and risks are shown in Table 4 in three stages. The barriers are listed in the first section of the table in orange, with the first level representing the top category, which has been projected into three to four sub-categories. In this case, the categories listed could mostly be assigned to all technologies. The projected risks are in red in Table 4.
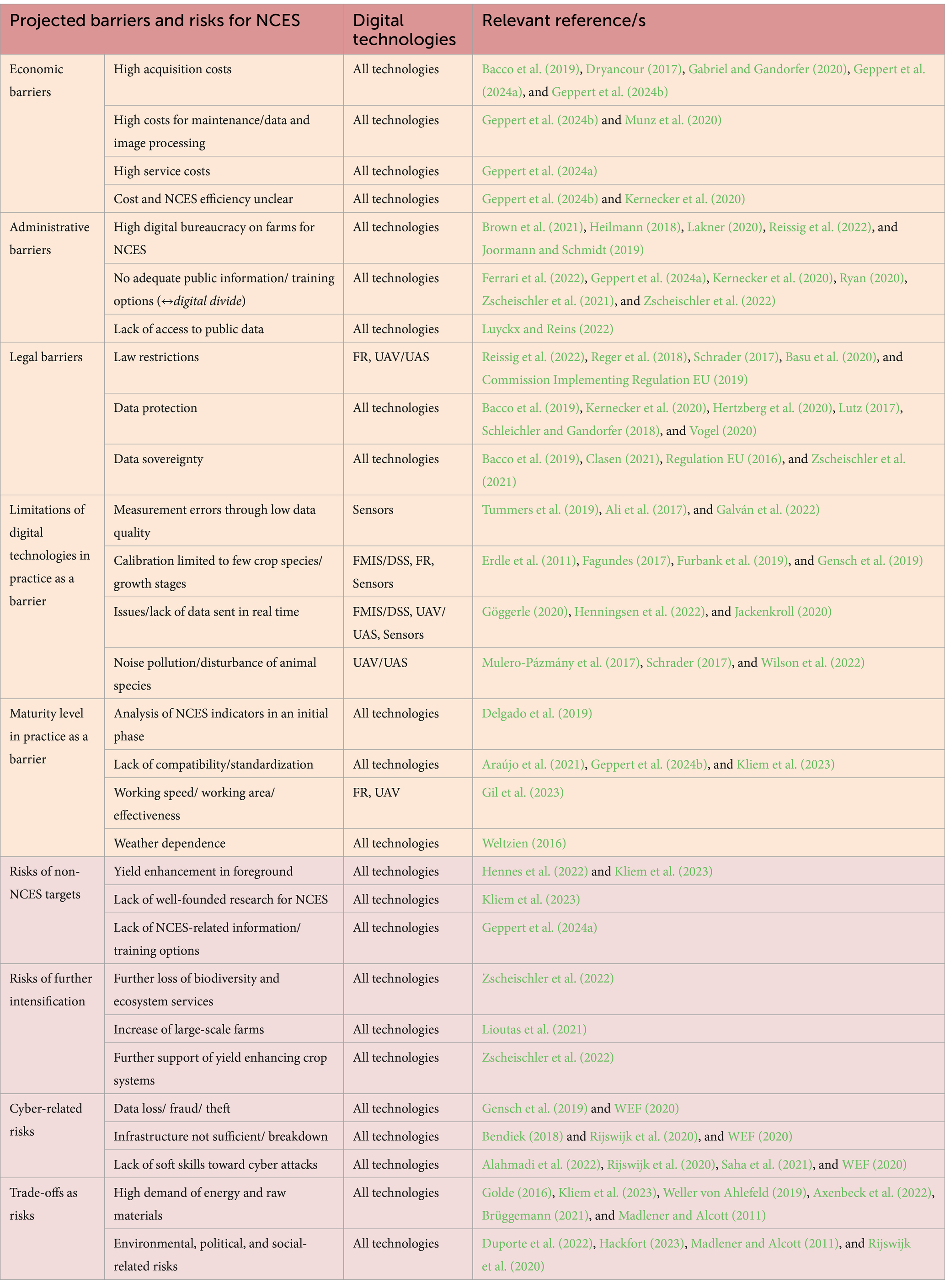
Table 4. Overview of projected risks and barriers for NCES implementation of digital technologies by category.
The average income of farms with arable area under 100 ha is rather low, whereby almost 69% of all farms in Germany manage less than 50 ha (Destatis, 2021). High costs for acquisition, maintenance, and service are the most impeding factor preventing the use of all digital technologies (Table 3; Gabriel and Gandorfer, 2020) and especially for NCES purposes, because farmers cannot afford to invest in expensive technologies, such as FR or UAV/UAS (Geppert et al., 2024a). Since many technology manufacturers do not provide prices online, the authors of the study wrote to four companies that offer drone services for agriculture in Germany. The names and locations of the companies are kept anonymous. A drone survey with RGB, multispectral and thermal camera for a one-day flight for a maximum of 300 ha costs between 6,000 and 9,000 euros, depending on the company’s offer. The evaluation of the raw data is an additional service, which can vary between 1,500 and 3,000 euros. In this case, a farmer will not have a direct income source for providing NCES, as the NCES monitoring will not increase the price of the agricultural products. For this reason, satellite images from current and previous years are mostly used. Furthermore, policy makers are not ready to finance acquisition costs for FR and contractors do not offer FR hire yet. A single FR acquisition starts between 50,000 and 70,000 euros, as found in the review process.
The administrative workload on farms for NCES through digitalization is an increasing obstacle in Germany (Table 3; Gabriel and Gandorfer, 2020). This burden realistically results in little time for the implementation of NCES, especially when the practical implementation is associated with additional administrative workload (Brown et al., 2021) and there is a threat of strict sanctions in case of administrative gaps (Joormann and Schmidt, 2019). Digital administration platforms do not necessarily facilitate farms as the regulations remain very complicated, the sanctions strict, and the controls time-consuming (Reissig et al., 2022). Based on our review the requirements for applying for NCES subsidies from CAP are equally as complicated in the digital format. Furthermore, payments for specific NCES are currently quite low (Batáry et al., 2015; Regulation EU, 2013).
Autonomous technologies, such as FR, are facing legal barriers as they are still in a grey area according to EU law (Basu et al., 2020). In Germany, the European Machinery Directive (European Parliament, 2006) applies to manufacturing and marketing: autonomous robots must be operated by a person. According to Basu et al. (2020), the applicable laws for autonomous field robots are not so clear and the introduction of the term field robot (such as an agribot) is necessary, as the working conditions in the field are not equivalent to other areas of operation, such as road traffic. In December 2022, the European Commission announced that a new proposal for a Regulation of the European Parliament and of the Council on machinery products had been filed (European Commission, 2022). From the current point of view, the liability regime is challenged by technical legal arguments.
EU-wide regulations for the operation of UAVs have been in force since the beginning of 2021, following a decision by the European Commission on 24 May 2019 (Commission Implementing Regulation EU, 2019). For the operation of drones ≥250 g, proof of competence and registration of the drone is mandatory (Commission Implementing Regulation EU, 2019). Based on our request to the responsible authority, a processing time of 6 weeks is estimated for the approval of one drone flight for monitoring purposes over an agricultural area.
The data management of digital technologies is characterized by a difficult legal situation and controversial discussions about data protection (Lutz, 2017; Vogel, 2020). The problem is mainly the General Data Protection Regulation (Regulation EU, 2016), currently in force within the EU, which does not guarantee data sovereignty for non-personal data. As the application of digital technologies involves the collection and storage of very sensitive data (e.g., location, engine operating hours, and fuel consumption), there is still skepticism among farmers regarding data protection (Schleichler and Gandorfer, 2018). There is also a danger in handling digital data, which is a valuable commodity for data traders (Clasen, 2021). Large-scale data collections and analyses are often not directly accessible to farms, but rather to global and financially strong actors (Zscheischler et al., 2021). Farmers do not want their data to be stored on unknown/foreign servers that are not a subject to the EU law, as pointed out during our second expert talk. Systems that can be used on local computers are considered to be trustworthy for farmers (Gabriel and Gandorfer, 2020). However, with increasing use of AI the storage of data is moved to servers. It is therefore important for policy makers to intervene and endorse state-subsidized technologies or demand more transparency from large companies. The DIP named OdiL (abbreviation in German for “open software platform for service innovations in an agricultural value network”), which is currently in development, aims to enforce data ownership to each farm during data exchange with different stakeholders to win the trust of each user (Hertzberg et al., 2020). The Dutch data platform Akkerweb similarly promises farmers that they can decide for themselves exactly which farm data they want to pass on for further use (Van Evert et al., 2018). Another aspect is the fear of data collection, as mentioned by farmers in our second expert talk – farmers are afraid that if they allow NCES indicators to be monitored by authorities and a rare or severely endangered plant or animal species is recorded, their farmland will be blocked. For this, farmers do not receive any subsidies in Germany.
There are various limitations of digital technologies in practice in arable farming. The data collection and recording of biodiversity CSAP are in a very initial phase (Ball-Damerow et al., 2019). While sensors generate a great amount of data, only a very small fraction of these data can be used in agricultural DSS or software-based technologies. The main reasons are compatibility problems when processing data from different sensors, excessive time and cost expenditures, and a lack of multicausal DSS (Kehl et al., 2021). Public agricultural data are collected for administrative purposes only and there is currently no intention to collect and/or use it for decision-making regarding NCES (Luyckx and Reins, 2022). Furthermore, it is not yet clear whether large datasets will be able to capture and assess the complexity of agricultural systems, NCES as a whole (Delgado et al., 2019).
Further challenges for practice arise from data quality. The GreenSeeker optical sensor for instance, is for large-scale measurements, as smaller measurement units can lead to measurement errors (Ali et al., 2020). Limitations also emerge from the calibration of sensors, which are primarily intended to detect the genetic diversity of solely a few common crops, such as wheat (Khadka et al., 2021; Pratap, 2019). Currently, the genetic diversity of other crops and/or weeds in the stand remains unaddressed for cost reasons. Furthermore, plant disease are often caused by more than one pathogen and can show different symptoms, including different colors and patterns, which is a challenge for sensors, since they rely on image material (N. Zhang et al., 2020). UAVs have some disadvantages for species’ protection: they can cause noise pollution and disturb bird species (Schrader, 2017). The comprehensive use of drones is set back by the necessity of complex and expensive image processing and analysis software for application in practice. In addition, real-time recordings and analyses with UAVs are still not possible. The delayed provision of the results of drone pictures limits their implementation in agriculture (Kehl et al., 2021).
The maturity level of FR in practice is an impeding factor for NCES use (Geppert et al., 2024a). The current working speed of FR varies between 1 and 4 km/h (Gil et al., 2023). Furthermore, the effectiveness of weeding can vary greatly between the FR, depending on the site, soil type, weed infestation, weed composition and weeding time (Ahmad et al., 2014; Bručienė et al., 2021; Fountas et al., 2015). Especially on loamy soils, FR must have enough power and weight to successfully plough and further cultivate the soil such as farm tractors. Up to now, FR are still representing niche products (Kehl et al., 2021).
Digitalization could push the further intensification to cultivate agricultural residual areas that are important for NCES as ecological stepping stone biotopes, insofar as they are not under protection or ecological priority areas within the framework of the CAP. This phenomenon could lead to the loss of valuable areas. At the same time, there are concerns that an increase in the number of large-scale farms alongside digital technologies would have a negative impact on NCES. There is fundamental skepticism regarding the use of algorithms that are being developed primarily for yield enhancement (Reichel et al., 2021). If NCES is not considered from the beginning by policy makers and farmers, it will be more difficult to add new drivers into such a complex system at a later time (Geppert et al., 2024a; Kliem et al., 2023). The precise and intensive use of all land areas can lead to a reduction in habitat niches in the marginal areas (Reichel et al., 2021). Currently, site-specific functions in FMIS are less represented (Melzer et al., 2023). During the expert discussion in this study, experts saw a higher risk for intensification on conventional farms through digitalization, if financial support of NCES is not sufficient. For organic farms the risk of NCES losses through digitalization were described as low. Organic farming, as an agricultural concept, concentrates on the promotion of NCES mainly through agro-ecological practices and not through digitalization.
A great challenge is also the interoperability among digital technologies from different vendors. In general, there is a lack of compatibility among data formats and available systems. There are issues with data from sensors and sensor networks when a transfer in a DSS or FMIS application is needed, which is why a variety of isolated solutions are offered (Kehl et al., 2021). Due to non-uniform federal systems, it is for instance not possible to use public geospatial data on a national scale in Germany, which leads to data fragmentation in digital technologies. The Shapefile (.shp) from ESRI is meanwhile a common data format for software-based technologies in agriculture for the storage of geometric locations. However, its size is limited to 2 GB and it does not support topologic information. ISO-XML commonly used as a communication protocol between agricultural machine terminals (MICS, e.g., sensors, robots) and agricultural software (e.g., FMIS/DSS). There are different ISO-XML versions available, so it is not always possible to use together hardware and software from different vendors. With large volumes of data, it is practically impossible for farmers to find time to look at many databases and check data sets or formats.
Digital technologies can lead to trade-offs. An increased use of digital tools can lead to a higher demand of energy and certain raw materials. The mining and installation of raw material can cause massive damage to the environment and wastes are costly and difficult to dispose (Hoiß, 2023). Furthermore, it is not known with certainty whether data storage is relying on renewable energy sources (Van der Velden, 2018). Moreover, data centers produce high amounts of heat loss, which negatively contributes to global warming. Likewise, the production and use of digital and smart technologies demands a lot of energy (Gensch et al., 2019).
3.4 Challenges in agriculture slowing down the use of digital technologies for NCES, critical insights and future research
Germany is one of the world’s leading nations in the production and use of modern technologies. With the help of digitalization, the competitiveness of German agriculture can be advanced (BMEL, 2022a). However, the ambitious goals set regarding digitalization as a solution for NCES problems have not yet been fulfilled, and future success depends heavily on agriculture as well as social acceptance and economic and political conditions.
The main participants in the digitalization discussion currently are researchers and the smallest cohort of participants are farmers (Martens and Zscheischler, 2022). In our project, we also found that farmers were rather unwilling to participate in talks on NCES promotion through digital technologies, while researchers and industry already expressed ideas for the direction of digitization. The opinions of the experts from the competence areas of research and farms differ greatly regarding digitalization and NCES. Researchers tend to see a broader spectrum of obstacles and preconditions for NCES measures through digital technologies before, during and after the technologies reach the field. Farmers were interested in practical and financial preconditions for NCES measures with digital technologies during and after applications on field. For the future development and use of digital technologies, as well as many research gaps, it is important for farmers to address the economic aspects that currently characterize everyday life on a farm in public discussions (Kliem et al., 2023). According to a survey of 500 farmers in Germany (2022), some of the biggest obstacles for farmers are (1) the implementation of sustainability measures (67%) and (2) digitalization is an economic challenge for the farmers’ business (51%). Furthermore, surveyed participants from rural areas in Central Europe were asked to describe socio-cultural impacts and found that there are concerns about an exclusion of those subjects who cannot keep up with digitalization (Ferrari et al., 2022).
Alongside farmers, society must also be included in the design of NCES supported by digitalization (Kliem et al., 2023). Beyond that, however, the public perception of digitalization in agriculture has hardly occurred. Assuming that farmers were to actively implement NCES measures using digital technologies, it is not known whether consumers would increasingly access products from innovative systems. The question of how to measure and monetize NCES indicators remains unanswered. This would require a case study that compiles a cost breakdown from the acquisition of a new technology to the commercialization of an agricultural product and examines the monetary value of all NCES implemented measures though digital technologies for consumers, e.g., through choice experiments when buying the agricultural product. However, the problem with NCES value remains a broader discussion and the facilitation of NCES measures through digitalization in agriculture can offer solely a partial solution.
In a study it was concluded that the companies dictating the agricultural digital technology market in Germany, as well as in the rest of the world, and are allied with powerful national and international policy actors (Hackfort, 2023). The author added that digitalization pushes low-tech approaches into the background and argued for a structural transformation on policy and funding (Hackfort, 2023) Whole systems could be marginalized by claiming primacy of “sustainable” digitalization in agriculture, while in practice more time will be needed to achieve any declared sustainability goal. Currently, there are two development paths for the further transformation of the agricultural sector: digitalization of existing management methods and administrative processes, where existing large-scale farm machines and technologies are supplemented with digital and automated applications supported by industry; and cultivation of fundamentally new small-scale technologies (e.g., FR and UAV) that deviate from previously used agricultural machinery and cultivation methods supported by researchers (Gaus et al., 2017; Kliem et al., 2023). Both paths have one thing in common for the future - digital technologies must be cost-, yield- and NCES-effective.
Digital technologies can enable an ecological utopia for sustainability or trigger an ecological dystopia and support unsustainable intensification of agriculture (Daum, 2021). Therefore, the industry alone should not set the guiding goals, nor should policy makers only look at food security. Responsible innovation in agriculture is possible only when a wide range of stakeholders is included in the entire design and development process of a technology (2021). As we found, the current digital transformation is strongly focused on global food security, sustainable intensification, and climate-smart agriculture measures. However, digitalization in organic farming, permaculture, or regenerative agriculture is not of interest due to a lower yield potential (Geppert et al., 2024a). Currently, research does not provide sufficient information on the potentials and risks of how the use of digital technologies affects NCES in Germany. This research gap is an obstacle to the implementation of more NCES-enhancing measures in agriculture. On the other hand, the difficulty analyzing the rapidly developing digitalization based on publications could be behind the development of digital technologies in practice. Either way, without a well-founded knowledge base from research, it is difficult to formulate regulatory measures or policy recommendations (Geppert et al., 2024a). There is a great deal of potential in the use of digital technologies. However, the potentials need to be recognized, developed, and combined for practical NCES so that NCES can be widely implemented in agriculture in the next 10 years (Geppert et al., 2024a; Lange et al., 2023). For NCES transformation of agriculture, however, more far-reaching changes are needed along agricultural and ecological concepts that promote the diversification and long-term resilience of agricultural systems (Geppert et al., 2024a).
Future research needs to determine, based on practical investigations, whether the trade-offs in the production of hardware-based digital technologies undercut or exceed NCES potentials in their use in Germany. Furthermore, aspects of beneficial insect promotion and monitoring in the field using digital technologies are currently neglected, but need active research to promote NCES. It would also make economic sense for commercially available technologies in Germany to be analyzed more closely in practice on their NCES potentials and risks before more and more new technologies are launched.
4 Conclusion
Digitalization in agriculture is advancing rapidly, and the market as well as the motivations for the use of technologies are changing dynamically. Therefore, it is important to set certain goals for NCES and to identify potentials as well as the barriers and risks that currently prevent digital technologies in agriculture in the realization NCES measures. The technology market in Germany offers many digital technologies. However, farmers struggle with many challenges – digitalization for NCES has not yet arrived convincingly in practice – the barriers and risks outweigh the potentials and cause skepticism. The projected potentials are either barely established and used in agricultural practices, but are expected to be useful in the future. Digital support for precise management processes while saving resources as well as site-specific regional management and implementation of NCES measures have high potential in Germany. Moreover, there is a high potential to increase and mitigate NCES harming emissions. Key aspects impeding the potentials are caused by significantly high effort for administrational matters, high acquisition costs, and the lack of adequate training programs for NCES measures within digitalization.
The ambitious goals set on digitalization as a solution for ecological problems have not been fulfilled until now and the success depends heavily not only on agriculture but also on social acceptance, economic and political conditions, as well as on conquering barriers.
Author contributions
TK: Conceptualization, Funding acquisition, Methodology, Visualization, Writing – original draft, Writing – review & editing. FG: Conceptualization, Funding acquisition, Methodology, Validation, Writing – review & editing. NL: Methodology, Visualization, Writing – review & editing. SB-K: Conceptualization, Funding acquisition, Supervision, Writing – review & editing.
Funding
The author(s) declare that financial support was received for the research and/or publication of this article. This study was funded by Expertenkommission Forschung und Innovation (EFI). The findings, interpretations and conclusions expressed in this review paper do not necessarily reflect the view of the Expertenkommission Forschung und Innovation (EFI) or its members.
Conflict of interest
The authors declare that the research was conducted in the absence of any commercial or financial relationships that could be construed as a potential conflict of interest.
Publisher’s note
All claims expressed in this article are solely those of the authors and do not necessarily represent those of their affiliated organizations, or those of the publisher, the editors and the reviewers. Any product that may be evaluated in this article, or claim that may be made by its manufacturer, is not guaranteed or endorsed by the publisher.
Supplementary material
The Supplementary material for this article can be found online at: https://www.frontiersin.org/articles/10.3389/fsufs.2025.1464020/full#supplementary-material
Footnotes
References
Achtziger, R., Richert, E., Köstner, B., and Nigmann, U. (2021). Biodiversität in Weinbergen: -Biodiversität und Ökosystemleistungen. Bundesministerium für Umwelt, Naturschutz und Nukleare Sicherheit (BMU).
Adão, T., Hruška, J., Pádua, L., Bessa, J., Peres, E., Morais, R., et al. (2017). Hyperspectral imaging: A review on UAV-based sensors, data processing and applications for agriculture and forestry. Remote Sens. 9:1110. doi: 10.3390/rs9111110
Aden, C., and Stephan, K. (2017). Web-based citizen involvement in research into pathways and hotspots of marine litter in the southern North Sea. GI Forum 1, 60–77. doi: 10.1553/giscience2017_02_s60
Ahirwar, S., Swarnkar, R., Bhukya, S., and Namwade, G. (2019). Application of drone in agriculture. Int. J. Curr. Microbiol. Appl. Sci. 8, 2500–2505. doi: 10.20546/ijcmas.2019.801.264
Ahmad, M. T., Tang, L., and Steward, B. L. (2014). “Automated mechanical weeding” in SpringerLink Bücher. Automation: The future of weed control in cropping systems. eds. F. J. Pierce and S. L. Young (Netherlands: Springer), 125–137.
Alahmadi, A. N., Rehman, S. U., Alhazmi, H. S., Glynn, D. G., Shoaib, H., and Solé, P. (2022). Cyber-security threats and Side-Channel attacks for digital agriculture. Sensors (Basel, Switzerland) 22:3520. doi: 10.3390/s22093520
Ali, M. M., Al-Ani, A., Eamus, D., and Tan, D. K. Y. (2017). Leaf nitrogen determination using non-destructive techniques–A review. J. Plant Nutr. 40, 928–953. doi: 10.1080/01904167.2016.1143954
Ali, A. M., Ibrahim, S. M., and Bijay-Singh, B. (2020). Wheat grain yield and nitrogen uptake prediction using atLeaf and GreenSeeker portable optical sensors at jointing growth stage. Inf. Process. Agric. 7, 375–383. doi: 10.1016/j.inpa.2019.09.008
Alshihabi, O., Piikki, K., and Söderström, M. (2020). “Cropsat – A decision support system for practical use of satellite images in precision agriculture” in Springer eBook collection: Vol. 684. Advances in smart technologies applications and case studies: Selected papers from the first international conference on smart information and communication technologies, SmartICT 2019, September 26–28, 2019, Saidia, Morocco. eds. A. El Moussati, K. Kpalma, M. G. Belkasmi, M. Saber, and S. Guégan. 1st ed (Berlin: Springer International Publishing), 415–421.
Altrudi, S. (2021). Connecting to nature through tech? The case of the iNaturalist app. Convergence 27, 124–141. doi: 10.1177/1354856520933064
Arakeri, M. P., Vijaya, K. B. P., Barsaiya, S., and Sairam, H. V. (2017). Computer vision based robotic weed control system for precision agriculture. In: 2017 international conference on advances in computing, communications and informatics (ICACCI), pp. 1201–1205.
Araújo, S. O., Peres, R. S., Barata, J., Lidon, F., and Ramalho, J. C. (2021). Characterising the agriculture 4.0 landscape—emerging trends, challenges and opportunities. Agronomy 11:667. doi: 10.3390/agronomy11040667
Arif, M., Hedley, R., and Bayne, E. (2020). Testing the accuracy of a birdNET, Automatic bird song Classifier. Available online at: https://era.library.ualberta.ca/items/42690fd9-7e50-4534-8361-cefbf7f40b49.
Artmann, W. (2021). Drohne hilft bei Rehkitz-Rettung: Immer wieder kommt es vor, dass Kitze den Mähtod erleiden. Technologie kann helfen. Die Kooperation aller Beteiligten auch. Mittelbayerische. Available online at: https://www.mittelbayerische.de/region/schwandorf-nachrichten/drohne-hilft-bei-rehkitz-rettung-21416-art2009234.html.
Axenbeck, J., Berner, A., and Kneib, T. (2022). What drives the relationship between digitalization and industrial energy demand? Exploring firm-level heterogeneity: Disucssion paper no. 22-059 11/2022. Available online at: https://ftp.zew.de/pub/zew-docs/dp/dp22059.pdf.
Bacco, M., Barsocchi, P., Ferro, E., Gotta, A., and Ruggeri, M. (2019). The digitisation of agriculture: a survey of research activities on smart farming. Array 3:100009. doi: 10.1016/j.array.2019.100009
Baillie, C., Lobsey, C. R., Antille, D. L., McCarthy, C. L., and Thomasson, J. A. (2018). A review of the state of the art in agricultural automation. Part III: Agricultural machinery navigation systems, no. 1.
Balafoutis, A., Beck, B., Fountas, S., Vangeyte, J., Wal, T., Soto, I., et al. (2017). Precision agriculture technologies positively contributing to GHG emissions mitigation, farm productivity and economics. Sustainability 9:1339. doi: 10.3390/su9081339
Ball-Damerow, J. E., Brenskelle, L., Barve, N., Soltis, P. S., Sierwald, P., Bieler, R., et al. (2019). Research applications of primary biodiversity databases in the digital age. PLoS One 14:e0215794. doi: 10.1371/journal.pone.0215794
Bangert, W., Kielhorn, A., Rahe, F., Albert, A., Biber, P., Grzonka, S., et al. (2013). Field-robot-based agriculture:“RemoteFarming. 1” and “BoniRob-apps”. Available at: http://www.slawomir.de/publications/bangert13vdi_ageng/bangert13vdi_ageng.pdf.
Basavegowda, D. H., Mosebach, P., Schleip, I., and Weltzien, C. (2022). “Indicator plant species detection in grassland using EfficientDet object detector” in GI-Edition. Proceedings: Volume P-317. Informatik in der Land-, Forst- und Ernährungswirtschaft: Fokus: Künstliche Intelligenz in der Agrar- und Ernährungswirtschaft: Referate der 42. Gil-Jahrestagung 21.- 22. Februar 2022, Agroscope, Tänikon, Ettenhausen, Schweiz. eds. M. Gandorfer, C. Hoffmann, N. El Benni, M. Cockburn, T. Anken, and H. Floto (Bonn, Germany: Gesellschaft für Informatik), 57–62.
Basso, B., and Antle, J. (2020). Digital agriculture to design sustainable agricultural systems. Nat. Sustain. 3, 254–256. doi: 10.1038/s41893-020-0510-0
Basu, S., Omotubora, A., Beeson, M., and Fox, C. (2020). Legal framework for small autonomous agricultural robots. AI & Soc. 35, 113–134. doi: 10.1007/s00146-018-0846-4
Batáry, P., Dicks, L. V., Kleijn, D., and Sutherland, W. J. (2015). The role of Agri-environment schemes in conservation and environmental management. Conserv. Biol. 29, 1006–1016. doi: 10.1111/cobi.12536
Bawden, O., Kulk, J., Russell, R., McCool, C., English, A., Dayoub, F., et al. (2017). Robot for weed species plant-specific management. J. Field Robot. 34, 1179–1199. doi: 10.1002/rob.21727
Been, T. H., Kempenaar, C., Van Evert, F. K., Hoving, A., Michielsen, J., Booij, J. A., et al. (2018). Akkerweb, a new platform for use of spatial and temporal data in precision farming. Book of abstracts of the European conference on agricultural engineering AgEng2018, 31. Available online at: https://research.wur.nl/en/publications/akkerweb-a-new-platform-for-use-of-spatial-and-temporal-data-in-p.
Behr, O., Brinkmann, R., Korner-Nievergelt, F., Nagy, M., Niermann, I., Reich, M., et al. (2015). Reduktion des Kollisionsrisikos von Fledermäusen an Onshore-Windenergieanlagen RENEBAT II: Schriftenreihe Institut für Umweltplanung.
Bekey, G. A. (1998). On autonomous robots. Knowl. Eng. Rev. 13, 143–146. doi: 10.1017/S0269888998002033
Bellon Maurel, V., and Huyghe, C. (2017). Putting agricultural equipment and digital technologies at the cutting edge of agroecology. OCL 24:D307. doi: 10.1051/ocl/2017028
Bendiek, S. (2018). “Die richtigen Rahmenbedingungen für eine erfolgreiche digitale Transformation” in Digitalisierung im Spannungsfeld von Politik, Wirtschaft, Wissenschaft und Recht. eds. C. Bär, T. Grädler, and R. Mayr (Berlin: Springer Gabler), 21–29.
BfN. (2015). Artenschutz-Report 2015: Tiere und Pflanzen in Deutschland. Available online at: https://www.bfn.de/sites/default/files/2021-04/Artenschutzreport_Download.pdf.
Bhat, S. A., and Huang, N.-F. (2021). Big data and AI revolution in precision agriculture: survey and challenges. IEEE Access 9, 110209–110222. doi: 10.1109/ACCESS.2021.3102227
Billingsley, J. (2019). Burleigh Dodds series in agricultural science: Vol. 44. Robotics and automation for improving agriculture. Cambridge: Burleigh Dodds Science Publishing.
Bligaard, J. (2014). Mark online, a full scale GIS-based Danish farm management information system. Int. J. Food Syst. Dyn. 5, 190–195. doi: 10.22004/ag.econ.198969
BMEL. (2022a). Digitalisierung in der Landwirtschaft: Chancen nutzen - Risiken minimieren. Available online at: https://www.bmel.de/SharedDocs/Downloads/DE/Broschueren/digitalpolitik-landwirtschaft.pdf?__blob=publicationFile&v=20.
BMEL. (2022b). Landwirtschaft, Klimaschutz und Klimaresilienz. Available online at: https://www.bmel.de/DE/themen/landwirtschaft/klimaschutz/landwirtschaft-und-klimaschutz.html.
Bogue, R. (2017). Sensors key to advances in precision agriculture. Sens. Rev. 37, 1–6. doi: 10.1108/SR-10-2016-0215
Boho, D., Rzanny, M., Wäldchen, J., Nitsche, F., Deggelmann, A., Wittich, H. C., et al. (2020). Flora capture: A citizen science application for collecting structured plant observations. BMC Bioinformatics 21:576. doi: 10.1186/s12859-020-03920-9
Boursianis, A. D., Papadopoulou, M. S., Diamantoulakis, P., Liopa-Tsakalidi, A., Barouchas, P., Salahas, G., et al. (2022). Internet of things (IoT) and agricultural unmanned aerial vehicles (UAVs) in smart farming: A comprehensive review. Internet Things 18:100187. doi: 10.1016/j.iot.2020.100187
Brown, C., Kovács, E., Herzon, I., Villamayor-Tomas, S., Albizua, A., Galanaki, A., et al. (2021). Simplistic understandings of farmer motivations could undermine the environmental potential of the common agricultural policy. Land Use Policy 101:105136. doi: 10.1016/j.landusepol.2020.105136
Bručienė, I., Aleliūnas, D., Šarauskis, E., and Romaneckas, K. (2021). Influence of mechanical and intelligent robotic weed control methods on energy efficiency and environment in organic sugar beet production. Agriculture 11:449. doi: 10.3390/agriculture11050449
Bručienė, I., Buragienė, S., and Šarauskis, E. (2022). Weeding effectiveness and changes in soil physical properties using inter-row hoeing and a robot. Agronomy 12:1514. doi: 10.3390/agronomy12071514
Brüggemann, A. (2021). The trade-offs between digitalisation and climate action: why digitalisation must be sustainable: KfW research/ focus on Economics, No. 341. Available online at: https://www.kfw.de/PDF/Download-Center/Konzernthemen/Research/PDF-Dokumente-Fokus-Volkswirtschaft/Fokus-englische-Dateien/Fokus-2021-EN/Focus-No.-341-August-2021-Digitalisation-and-Climate.pdf.
Brugger, A., Yamati, F. I., Barreto, A., Paulus, S., Schramowsk, P., Kersting, K., et al. (2023). Hyperspectral imaging in the UV range allows for differentiation of sugar beet diseases based on changes in secondary plant metabolites. Phytopathology 113, 44–54. doi: 10.1094/PHYTO-03-22-0086-R
Buddha, K., Nelson, H. J., Zermas, D., and Papanikolopoulos, N. (2019). Weed detection and classification in high altitude aerial images for robot-based precision agriculture. In: 2019 27th Mediterranean conference on control and automation (MED), pp. 280–285.
Bundesministerium für Ernährung und Landwirtschaft. (2022). Digitalisierung in der Landwirtschaft: Chancen nutzen - Risiken minimieren. Available online at: https://www.bmel.de/SharedDocs/Downloads/DE/Broschueren/digitalpolitik-landwirtschaft.pdf?__blob=publicationFile&v=20.
Carrer, M. J. (2023). “Farm management information systems (FMIS)” in Encyclopedia of digital agricultural technologies. ed. Q. Zhang. 1st ed (Berlin: Springer International Publishing), 503–509.
Cheng, C., Fu, J., Su, H., and Ren, L. (2023). Recent advancements in agriculture robots: benefits and challenges. Mach. Des. 11:48. doi: 10.3390/machines11010048
Chu, H., Zhang, D., Shao, Y., Chang, Z., Guo, Y., and Zhang, N. (2018). Using HOG descriptors and UAV for crop Pest monitoring. In: Proceedings 2018 Chinese automation congress (CAC): Nov. 30-Dec. 02, 2018, Xi’an, China, pp. 1516–1519. IEEE.
Cillis, D., Maestrini, B., Pezzuolo, A., Marinello, F., and Sartori, L. (2018). Modeling soil organic carbon and carbon dioxide emissions in different tillage systems supported by precision agriculture technologies under current climatic conditions. Soil Tillage Res. 183, 51–59. doi: 10.1016/j.still.2018.06.001
Clarke, R. (2014). Understanding the drone epidemic. Comput. Law Secur. Rev. 30, 230–246. doi: 10.1016/j.clsr.2014.03.002
Clasen, M. (2021). “Über den Wert von Daten in der Landwirtschaft” in GI-Edition. Proceedings: volume P-309, Informatik in der Land-, Forst- und Ernährungswirtschaft: Fokus: Informations- und Kommunikationstechnologie in kritischen Zeiten: Referate der 41. GIL-Jahrestagung, 08.-09. März 2021, Leibniz-Institut für Agrartechnik und Bioökonomie e.V., Potsdam. eds. M. Gandorfer, A. Meyer-Aurich, H. Bernhardt, C. Hoffmann, C. Weltzien, and S. D. Bellingrath-Kimura (Germany: Gesellschaft für Informatik), 61–66.
Cobb, P. J., Earley-Spadoni, T., and Dames, P. (2019). Centimeter-level recording for all: field experimentation with new, affordable geolocation technology. Adv. Archaeol. Pract. 7, 353–365. doi: 10.1017/aap.2019.21
Commission Implementing Regulation EU (2019). 2019/947 of 24 may 2019 on the rules and procedures for the operation of unmanned aircraft, official journal of the European Union L152/45. Available online at: https://eur-lex.europa.eu/legal-content/EN/TXT/HTML/?uri=CELEX:32019R0947.
Da Silva, E. A., and Mendonça, G. V. (2005). Digital Image Processing. In: W. K. Chen The Electrical Engineering Handbook. New York: Academic Press, pp. 891–910.
Dammer, K. H., Intreß, J., Schirrmann, M., and Garz, A. (2019). Wachstumsverhalten der Beifußblättrigen Ambrosie (Ambrosia artemisiifolia L.) auf landwirtschaftlichen Nutzflächen Brandenburgs – Schlussfolgerungen für die Bildverarbeitung kameragestützter Monitoring-Strategien. Gesunde Pflanzen 71, 227–235. doi: 10.1007/s10343-019-00488-0
Daum, T. (2021). Farm robots: ecological utopia or dystopia? Trends Ecol. Evol. 36, 774–777. doi: 10.1016/j.tree.2021.06.002
De Camargo, T., Schirrmann, M., Landwehr, N., Dammer, K.-H., and Pflanz, M. (2021). Optimized deep learning model as a basis for fast UAV mapping of weed species in winter wheat crops. Remote Sens. 13:1704. doi: 10.3390/rs13091704
De Jong, E. D., and De Boer, B. G. (2009). “Dynamical systems, individual-based modeling, and self-organization” in Artificial intelligence: encyclopedia of life support systems. ed. J. N. Kok (Oxford, UK: Eolss Publishers), 64–88.
De Kock, M. E., Pohůnek, V., and Hejcmanová, P. (2022). Semi-automated detection of ungulates using UAV imagery and reflective spectrometry. J. Environ. Manag. 320:115807. doi: 10.1016/j.jenvman.2022.115807
Delgado, J. A., Short, N. M., Roberts, D. P., and Vandenberg, B. (2019). Big data analysis for sustainable agriculture on a geospatial cloud framework. Front. Sustain. Food Syst. 3:Article 54. doi: 10.3389/fsufs.2019.00054
Destatis. (2021). Strukturwandel in der Landwirtschaft hält an: Umstellung auf den Ökolandbau hält an: Anteil der Ökofläche steigt auf 9,6%. Available online at: https://www.destatis.de/DE/Presse/Pressemitteilungen/2021/01/PD21_028_412.html#:~:text=Die%20durchschnittliche%20Gr%C3%B6%C3%9Fe%20der%20Betriebe,Betrieb%20durchschnittlich%2056%20Hektar20bewirtschaftete.
Deutsche Akademie der Naturforscher Leopoldina. (2020). Deutsche Akademie der Technikwissenschaften, and Union der Deutschen Akademien der Wissenschaften. Biodiversität und Management von Agrarlandschaften: umfassendes Handeln ist jetzt wichtig.
Deutscher Bundestag. (2019). Aktionsprogramm Insektenschutz der Bundesregierung - Gemeinsam wirksam gegen das Insektensterben: Unterrichtung durch die Bundesregierung. Drucksache 19/13031. Available online at: https://dserver.bundestag.de/btd/19/130/1913031.pdf.
Dickinson, J. L., Zuckerberg, B., and Bonter, D. N. (2010). Citizen science as an ecological research tool: challenges and benefits. Annu. Rev. Ecol. Evol. Syst. 41, 149–172. doi: 10.1146/annurev-ecolsys-102209-144636
Donat, M., Geistert, J., Grahmann, K., Bloch, R., and Bellingrath-Kimura, S. D. (2022). Patch cropping- a new methodological approach to determine new field arrangements that increase the multifunctionality of agricultural landscapes. Comput. Electron. Agric. 197:106894. doi: 10.1016/j.compag.2022.106894
Dransch, D., Kyba, C. C., Schröter, K., Yang, B., Schorlemmer, D., Barz, B., et al. (2018). Citizen Science–gemeinsam Wissen schaffen. 500 kB/System Erde; 8/System Erde; 8.
Dryancour, G. (2017). Smart agriculture for all farms: what needs to be done to help small farms access precision agriculture? How can the CAP help? Available online at: https://www.cema-agri.org/images/publications/position-papers/CEMA-smart-agriculture-for-all-farms_December-2017_.pdf.
Duckett, T., Pearson, S., Blackmore, S., Grieve, B., Chen, W.-H., Cielniak, G., et al. (2018). Agricultural robotics: the future of robotic agriculture. arXiv 2024:762. doi: 10.48550/arXiv.1806.06762
Duporte, A., Carmasa, G., Tazo, A. N., Nieto, E., and Salter, R. (2022). Environmental impacts of digitalisation: what to bear in mind: policy unit. Available online at: https://www.aeidl.eu/wp-content/uploads/2022/10/AEIDL-PolicyUnit-Environmental-impacts-of-digitalisation-AD-v4.pdf.
Edalat, M., Naderi, R., and Egan, T. P. (2019). Corn nitrogen management using NDVI and SPAD sensor-based data under conventional vs. reduced tillage systems. J. Plant Nutr. 42, 2310–2322. doi: 10.1080/01904167.2019.1648686
Elhakeem, A., Van der Werf, W., Ajal, J., Lucà, D., Claus, S., Vico, R. A., et al. (2019). Cover crop mixtures result in a positive net biodiversity effect irrespective of seeding configuration. Agric. Ecosyst. Environ. 285:106627. doi: 10.1016/j.agee.2019.106627
Epée Missé, P. T., Werner, A., and Almond, P. (2020). Developing automated and autonomous weed control methods on vegetable crops in New Zealand. SSRN Electron. J. 14:319. doi: 10.2139/ssrn.3745319
Erdle, K., Mistele, B., and Schmidhalter, U. (2011). Comparison of active and passive spectral sensors in discriminating biomass parameters and nitrogen status in wheat cultivars. Field Crop Res. 124, 74–84. doi: 10.1016/j.fcr.2011.06.007
European Commission. (2015). Report from the commission to the European Parliament and the council: The mid-term review of the EU biodiversity strategy to 2020. COM(2015) 478 final. European Commission. Available online at: https://eur-lex.europa.eu/legal-content/EN/TXT/PDF/?uri=CELEX:52015DC0478&from=EN.
European Commission. (2022). Commission welcomes political agreement on new rules to ensure the safety of machinery and robots. Available online at: https://ec.europa.eu/commission/presscorner/detail/en/ip_22_7741.
European Environmental Agency. (2015). The European environment: State and outlook 2015: Synthesis report.
European Parliament. (2006). Directive 2006/42/EC of the European Parliament and of the council of 17 may 2006 on machinery, and amending directive 95/16/EC (recast), The Official Journal of the European Union, L 157/24. Available online at: https://eur-lex.europa.eu/legal-content/EN/TXT/HTML/?uri=CELEX:32006L0042.
Fagundes, H. P. (2017). Development of cost effective IMU calibration procedure. Available online at: https://repositorio.ufsc.br/handle/123456789/197634
Faiçal, B. S., Freitas, H., Gomes, P. H., Mano, L. Y., Pessin, G., de Carvalho, A. C., et al. (2017). An adaptive approach for UAV-based pesticide spraying in dynamic environments. Comput. Electron. Agric. 138, 210–223. doi: 10.1016/j.compag.2017.04.011
FAO. (2019). The 10 elements of agroecology: Guiding the transition to sustainable food and agricultural systems. Available online at: https://www.fao.org/3/i9037en/i9037en.pdf
Fecke, W., Michels, M., Von Hobe, C.-F., and Mußhoff, O. (2018). Wie kommunizieren Landwirte in Zeiten der Digitalisierung? Ber. Landwirtsch. Z. Agrarpolitik Landwirtsch. 96:194. doi: 10.12767/buel.v96i2.194
Fellmann, T., Domínguez, I. P., Witzke, P., Weiss, F., Hristov, J., Barreiro-Hurle, J., et al. (2021). Greenhouse gas mitigation technologies in agriculture: regional circumstances and interactions determine cost-effectiveness. J. Clean. Prod. 317:128406. doi: 10.1016/j.jclepro.2021.128406
Ferrari, A., Bacco, M., Gaber, K., Jedlitschka, A., Hess, S., Kaipainen, J., et al. (2022). Drivers, barriers and impacts of digitalisation in rural areas from the viewpoint of experts. Inf. Softw. Technol. 145:106816. doi: 10.1016/j.infsof.2021.106816
Finger, R., Swinton, S. M., El Benni, N., and Walter, A. (2019). Precision farming at the Nexus of agricultural production and the environment. Ann. Rev. Resour. Econ. 11, 313–335. doi: 10.1146/annurev-resource-100518-093929
Fiorini, A., Remelli, S., Boselli, R., Mantovi, P., Ardenti, F., Trevisan, M., et al. (2022). Driving crop yield, soil organic C pools, and soil biodiversity with selected winter cover crops under no-till. Soil Tillage Res. 217:105283. doi: 10.1016/j.still.2021.105283
Fischer, M. C., Pärli, R., Gugerli, F., Holderegger, R., Lieberherr, E., and Widmer, A. (2020). Machbarkeitsstudie zur Untersuchung des Zustands und der Veränderung der genetischen Vielfalt. Vernetzung, Inzucht und Anpassungsfähigkeit.
Flynn, E. S., Dougherty, C. T., and Wendroth, O. (2008). Assessment of pasture biomass with the normalized difference vegetation index from active ground-based sensors. Agron. J. 100, 114–121. doi: 10.2134/agronj2006.0363
Fontanelli, M., Martelloni, L., Raffaelli, M., Frasconi, C., Ginanni, M., and Peruzzi, A. (2015). Weed Management in autumn fresh market spinach: A nonchemical alternative. HortTechnology 25, 177–184. doi: 10.21273/HORTTECH.25.2.177
Fountas, S., Carli, G., Sørensen, C. G., Tsiropoulos, Z., Cavalaris, C., Vatsanidou, A., et al. (2015). Farm management information systems: current situation and future perspectives. Comput. Electron. Agric. 115, 40–50. doi: 10.1016/j.compag.2015.05.011
Fountas, S., Mylonas, N., Malounas, I., Rodias, E., Hellmann Santos, C., and Pekkeriet, E. (2020). Agricultural robotics for field operations. Sensors (Basel, Switzerland) 20:2672. doi: 10.3390/s20092672
Frigerio, D., Richter, A., Per, E., Pruse, B., and Vohland, K. (2021). “Citizen science in the natural sciences” in Springer eBook collection. The science of citizen science. eds. K. Vohland, A. Land-Zandstra, L. Ceccaroni, R. Lemmens, J. Perelló, and M. Ponti (Berlin: Springer), 79–96.
Fritz, S., See, L., Carlson, T., Haklay, M., Oliver, J. L., Fraisl, D., et al. (2019). Citizen science and the United Nations sustainable development goals. Nat. Sustain. 2, 922–930. doi: 10.1038/s41893-019-0390-3
Furbank, R. T., Sirault, X. R. R., and Stone, E. (2019). “Plant phenome to genome: a big data challenge” in Sustaining global food security: The nexus of science and policy. ed. R. S. Zeigler (Clayton, Australia: CSIRO PUBLISHING).
Gabriel, A., and Gandorfer, M. (2020). Landwirte-Befragung 2020 Digitale Landwirtschaft Bayern. Bayerische Landesanstalt für LandwirtschaftInstitut für Landtechnik und Tierhaltun.
Gabriel, A., and Gandorfer, M. (2022). Landwirte-Befragung 2022: Digitale Landwirtschaft Bayern. Ergebnisband, No. 805. Available online at: https://www.lfl.bayern.de/mam/cms07/ilt/dateien/ilt6_dft_ergebnisband_by_2022_805.pdf.
Galván, S., Barrientos, R., and Varela, S. (2022). No bird database is perfect: citizen science and professional datasets contain different and complementary biodiversity information. Ardeola 69:6. doi: 10.13157/arla.69.1.2022.ra6
Garcia Furuya, D. E., Ma, L., Faita Pinheiro, M. M., Georges Gomes, F. D., Gonçalvez, W. N., Marcato, J., et al. (2021). Prediction of insect-herbivory-damage and insect-type attack in maize plants using hyperspectral data. Int. J. Appl. Earth Obs. Geoinf. 105:102608. doi: 10.1016/j.jag.2021.102608
Garske, B., Bau, A., and Ekardt, F. (2021). Digitalization and AI in European agriculture: A strategy for achieving climate and biodiversity targets? Sustain. For. 13:4652. doi: 10.3390/su13094652
Gascuel-Odoux, C., Lescourret, F., Dedieu, B., Detang-Dessendre, C., Faverdin, P., Hazard, L., et al. (2022). A research agenda for scaling up agroecology in European countries. Agron. Sustain. Dev. 42:53. doi: 10.1007/s13593-022-00786-4
Gaus, C., Minßen, T. F., Urso, L. M., De Witte, T., and Wegener, J. (2017). Mit autonomen Landmaschinen zu neuen Pflanzenbausystemen: New plant production systems with autonomous agricultural machinery. Available online at: https://orgprints.org/id/eprint/32438/1/32437_14NA004_011_012_thuenen_institut_de_Witte_Landmaschinen_Pflanzenbau.pdf.
Gaus, C. C., Minßen, T. F., Urso, L. M, De Witte, T., and Wegener, J. (2018). New plant production systems with autonomous agricultural machinery. Available online at: https://orgprints.org/id/eprint/32438/1/32437_14NA004_011_012_thuenen_institut_de_Witte_Landmaschinen_Pflanzenbau.pdf.
Gayer, C., Berger, J., Dieterich, M., Gallé, R., Reidl, K., Witty, R., et al. (2021). Flowering fields, organic farming and edge habitats promote diversity of plants and arthropods on arable land. J. Appl. Ecol. 58, 1155–1166. doi: 10.1111/1365-2664.13851
Gehrke, K. (2021). Drohne rettet bei Dargun Rehkitze vor Mähmaschinen. Available online at: https://www.nordkurier.de/mecklenburgische-schweiz/drohne-rettet-bei-dargun-rehkitze-vor-maehmaschinen-0343770206.html.
Gensch, C.-O., Gailhofer, P., Gsell, M., Heimann, C., Kampffmeyer, N., and Krohn, J. (2019). Digitalisierung und Nachhaltigkeit: Politische Gestaltung zwischen Möglichkeiten, falschen Versprechungen und Risiken: Zusammenfassendes Rahmenpapier. Available online at: https://www.zukunftserbe.de/fileadmin/user_upload/PDF/Rahmenpapier_Digitalisierung_20190503_final.pdf.
Geppert, F., Krachunova, T., and Bellingrath-Kimura, S. (2024a). Digital and smart technologies in agriculture in Germany: identification of key recommendations for sustainability actions: Studien zum deutschen Innovationsssystem. Available online at: https://www.e-fi.de/publikationen/studien.
Geppert, F., Krachunova, T., Mouratiadou, I., von der Nuell, J., and Bellingrath-Kimura, S. D. (2024b). Digital and smart technologies to enhance biodiversity in agricultural landscapes: an analysis of stakeholders’ perceptions of opportunities and challenges for broader adoption. Environ. Sustain. Indic. 23:100444. doi: 10.1016/j.indic.2024.100444
Ghobadpour, A., Monsalve, G., Cardenas, A., and Mousazadeh, H. (2022). Off-road electric vehicles and autonomous robots in agricultural sector: trends, challenges, and opportunities. Vehicles 4, 843–864. doi: 10.3390/vehicles4030047
Gil, G., Casagrande, D. E., Cortés, L. P., and Verschae, R. (2023). Why the low adoption of robotics in the farms? Challenges for the establishment of commercial agricultural robots. Smart Agric. Technol. 3:100069. doi: 10.1016/j.atech.2022.100069
Goettinger, M., Scholz, C., Ruckelshausen, A., Strothmann, W., Hinck, S., and Grzonka, S. (2014). GNSS-based navigation for the multipurpose field robot platform BoniRob to measure soil properties. Available online at: http://humanaturo.org/publications/goettinger14vdi/goettinger14vdi.pdf.
Göggerle, T. (2020). Was bedeutet eigentlich ISO-XML? Available online at: https://www.agrarheute.com/technik/traktoren/iso-xml-Datei-erklaert-563240.
Golde, M. (2016). Rebound-Effekte: Empirische Ergebnisse und Handlungsstrategien: Hintergrund Juni 2016. Available online at: https://www.umweltbundesamt.de/sites/default/files/medien/376/publikationen/rebound-effekte_empirische_ergebnisse_und_handlungsstrategien_hintergrundpapier.pdf.
Haas, E., Carozzi, M., Massad, R. S., Butterbach-Bahl, K., and Scheer, C. (2022). Long term impact of residue management on soil organic carbon stocks and nitrous oxide emissions from European croplands. Sci. Total Environ. 836:154932. doi: 10.1016/j.scitotenv.2022.154932
Hackfort, S. (2023). Unlocking sustainability? The power of corporate lock-ins and how they shape digital agriculture in Germany. J. Rural. Stud. 101:103065. doi: 10.1016/j.jrurstud.2023.103065
Hamami, L., and Nassereddine, B. (2020). Application of wireless sensor networks in the field of irrigation: A review. Comput. Electron. Agric. 179:105782. doi: 10.1016/j.compag.2020.105782
Haverkort, A. J., and Kempenaar, C. (2016). Recent advances in biotechnology and information technology in the potato industry. In: The Dundee conference, Dundee, Scotland. Available online at: https://library.wur.nl/WebQuery/wurpubs/fulltext/410605.
Heilmann, H. (2018). Gedanken zur Ausgestaltung von AUKM aus Sicht der Landwirtschaft. Institut für Pflanzenproduktion und Betriebswirtschaft. Available online at: https://www.lung.mvregierung.de/dateien/lls_vortrag_18_11_21_heilmann.pdf.
Heimstädt, C. (2023). The exploratory assetization of a crop protection app. Environ. Sci. Pol. 140, 242–249. doi: 10.1016/j.envsci.2022.12.014
Hemming, J., de Jong, H., Struik, L., van Meer, J., and Henten, E. J. V. (2018). Field performance evaluation method for a machine vision based intra row hoeing machine. Proceedings of the European conference on agricultural engineering AgEng2018. Symposium conducted at the meeting of Wageningen University and Research. Available online at: https://edepot.wur.nl/509139.
Hennes, L., Speck, M., and Liedtke, C. (2022). Digitalisation for A sustainable food system: study within the project “shaping the digital transformation”. Available online at: https://epub.wupperinst.org/frontdoor/deliver/index/docId/8119/file/8119_Sustainable_Food_System.pdf.
Henningsen, J., Herlitzius, T., Jeswein, T., Martini, D., Neuschwander, P., Rauch, B., et al. (2022). Machbarkeitsstudie für “Betriebliches Datenmanagement und Farm-Management-Information-System (FMIS)” in sächsischen Landwirtschaftsbetrieben. Available online at: https://slub.qucosa.de/landing-page/?tx_dlf[id]=https3A%2F%2Fslub.qucosa.de%2Fapi%2Fqucosa%253A77615%2Fmets.
Hernández-Ochoa, I. M., Gaiser, T., Kersebaum, K.-C., Webber, H., Seidel, S. J., Grahmann, K., et al. (2022). Model-based design of crop diversification through new field arrangements in spatially heterogeneous landscapes. A review. Agron. Sustain. Dev. 42:805. doi: 10.1007/s13593-022-00805-4
Hertzberg, J., Heijne, D., Kisliuk, B., Scheuren, S., and Stiene, S. (2020). “Geschützte Transparenz. Über technische und andere Voraussetzungen für die Digitalisierung der Landwirtschaft” in Robotik und Recht: Band 20. Digitalisierung, Automatisierung, KI und Recht: Festgabe zum 10-jährigen Bestehen der Forschungsstelle RobotRecht. eds. S. Beck, C. Kusche, and B. Valerius (Baden-Baden: Nomos), 59–80.
Hoiß, C. (2023). Zur Stofflichkeit digitaler Medien. MedienPädagogik 52, 47–63. doi: 10.21240/mpaed/52/2023.02.03.X
Holloway, J., and Mengersen, K. (2018). Statistical machine learning methods and remote sensing for sustainable development goals: A review. Remote Sens. 10:1365. doi: 10.3390/rs10091365
Horstmann, J. (2020). Digitalisierung und Vernetzung - Landwirtschaft im Wandel. In L. Frerichs Jahrbuch Agrartechnik 2019. Braunschweig: Institut für mobile Maschinen und Nutzfahrzeuge, pp. 1–8.
Hsieh, Y.-T., Chen, C.-T., and Chen, J.-C. (2017). Applying object-based image analysis and knowledge-based classification to ADS-40 digital aerial photographs to facilitate complex forest land cover classification. J. Appl. Remote. Sens. 11:015001. doi: 10.1117/1.JRS.11.015001
Ingram, J., and Maye, D. (2020). What are the implications of digitalisation for agricultural knowledge? Front. Sustain. Food Syst. 4:66. doi: 10.3389/fsufs.2020.00066
IPBES (2019). Global assessment report on biodiversity and ecosystem services of the intergovernmental science-policy platform on biodiversity and ecosystem services. Germany: IPBES.
IPCC. (2023). Synthesis report of the IPCC sixth assessment report (AR6): Longer report. Available online at: https://www.ipcc.ch/report/ar6/syr/downloads/report/IPCC_AR6_SYR_LongerReport.pdf.
Irias, A. J., and Castro, R. C. (2019). Algorithm of weed detection in crops by computational vision. 2019 International Conference on Electronics, Communications and Computers (CONIELECOMP), pp. 124–128.
Iversen, A. (2020). Marketing strategies of the German farm management information systems startups that reached critical mass. GI-Edition. Proceedings: Vol. 299, GI Edition Proceedings Band 299 Informatik in der Land-, Forst- und Ernährungswirtschaft: 40. GIL-Jahrestagung 17.-18. Februar 2020 Campus Weihenstephan, Freising, pp. 83–88.
Jackenkroll, M. (2020). Development of a spatial data infrastructure for precision agriculture applications: dissertation. Available online at: https://opus.uni-hohenheim.de/volltexte/2022/1983/pdf/Diss_Markus_Jackenkroll.pdf.
Jasim, A., Zaeen, A., Sharma, L. K., Bali, S. K., Wang, C., Buzza, A., et al. (2020). Predicting phosphorus and potato yield using active and passive sensors. Agriculture 10:564. doi: 10.3390/agriculture10110564
Javaid, M., Haleem, A., Khan, I. H., and Suman, R. (2023). Understanding the potential applications of artificial intelligence in agriculture sector. Adv. Agrochem. 2, 15–30. doi: 10.1016/j.aac.2022.10.001
Jones, H. G. (2020). What plant is that? Tests of automated image recognition apps for plant identification on plants from the British flora. AoB Plants 12:plaa052. doi: 10.1093/aobpla/plaa052
Joormann, I., and Schmidt, T. (2019). Landwirtebefragung und Expertendiskussionen zur Akzeptanz von Biodiversitätsmaßnahmen. 59th Annual Conference, Braunschweig, Germany, September 25-27, 2019 292337, German Association of Agricultural Economists (GEWISOLA).
Jordan, B. S., Branch, W. D., Coffin, A. W., Smith, C. M., and Culbreath, A. K. (2019). Comparison of Trimble GreenSeeker and crop circle (model ACS-210) reflectance meters for assessment of severity of late leaf spot. Peanut Sci. 46, 110–117. doi: 10.3146/PS18-19.1
Kahl, S., Wood, C. M., Eibl, M., and Klinck, H. (2021). BirdNET: A deep learning solution for avian diversity monitoring. Eco. Inform. 61:101236. doi: 10.1016/j.ecoinf.2021.101236
Kardasz, P., and Doskocz, J. (2016). Drones and possibilities of their using. J. Civil Environ. Eng. 6:233. doi: 10.4172/2165-784X.1000233
Karp, D. (2020). Detecting small and cryptic animals by combining thermography and a wildlife detection dog. Sci. Rep. 10:5220. doi: 10.1038/s41598-020-61594-y
Kehl, C., Meyer, R., and Steiger, S. (2021). Digitalisierung der Landwirtschaft: technologischer Stand und Perspektiven. Teil I des Endberichts zum TA-Projekt.
Kempenaar, C., Been, T., Booij, J., van Evert, F., Michielsen, J.-M., and Kocks, C. (2017). Advances in variable rate technology application in potato in the Netherlands. Potato Res. 60, 295–305. doi: 10.1007/s11540-018-9357-4
Kendall, S. L., Storer, K., and Berry, P. M. (2017). Measuring canopy size and nitrogen content in oilseed rape for variable plant growth regulator and nitrogen fertiliser application. Adv. Anim. Biosci. 8, 299–302. doi: 10.1017/S2040470017000875
Kernecker, M., Knierim, A., Wurbs, A., Kraus, T., and Borges, F. (2020). Experience versus expectation: farmers’ perceptions of smart farming technologies for cropping systems across Europe. Precis. Agric. 21, 34–50. doi: 10.1007/s11119-019-09651-z
Khadka, K., Burt, A. J., Earl, H. J., Raizada, M. N., and Navabi, A. (2021). Does leaf waxiness confound the use of NDVI in the assessment of chlorophyll when evaluating genetic diversity panels of wheat? Agronomy 11:486. doi: 10.3390/agronomy11030486
Kliem, L., Krachunova, T., Lange, S., Wagner, J., and Bellingrath-Kimura, S. (2023). Chancen und Risiken der Digitalisierung in der Landwirtschaft aus Sicht des Umwelt- und Naturschutzes. Bundesamt für Naturschutz.
Knierim, A., Boenning, K., Caggiano, M., Cristóvão, A., Dirimanova, V., Koehnen, T., et al. (2015). The AKIS concept and its relevance in selected EU member states. Outlook Agric. 44, 29–36. doi: 10.5367/oa.2015.0194
Koffler, S., Barbiéri, C., Ghilardi-Lopes, N. P., Leocadio, J. N., Albertini, B., Francoy, T. M., et al. (2021). A buzz for sustainability and conservation: the growing potential of citizen science studies on bees. Sustain. For. 13:959. doi: 10.3390/su13020959
Krudewig, K.-H. (2016). Vernetzung landwirtschaftlicher Prozesse-Know How nutzbar machen, Dresden. Available online at: https://core.ac.uk/download/pdf/236374536.pdf.
Kuhn, T., Möhring, N., Töpel, A., Jakob, F., Britz, W., Bröring, S., et al. (2022). Using a bio-economic farm model to evaluate the economic potential and pesticide load reduction of the greenRelease technology. Agric. Syst. 201:103454. doi: 10.1016/j.agsy.2022.103454
Kuhwald, M., Saggau, P., and Augustin, K. (2020). “Konflikte um Flächennutzung und Bodenfunktionen in Agrarlandschaften” in RaumFragen: Stadt – Region – Landschaft. Landschaft als Prozess. eds. R. Duttmann, O. Kühne, and F. Weber (Berlin: Springer VS), 657–688.
Kumar, M., Yadav, A. N., Saxena, R., Paul, D., and Tomar, R. S. (2021). Biodiversity of pesticides degrading microbial communities and their environmental impact. Biocatal. Agric. Biotechnol. 31:101883. doi: 10.1016/j.bcab.2020.101883
Lakner, S. (2020). Was kann die Gemeinsame Agrarpolitik der EU (CAP) zum Biodiversitätsschutz beitragen? Preprint: Tagungsbeitrag Zu Den Loccumer Protokollen; “Biodiversität Und Die Reform Der Gemeinsamen Agrarpolitik”.
Lange, S., Santarius, T., Dencik, L., Diez, T., Ferreboeuf, H., Hankey, S., et al. (2023). Digital reset, redirecting technologies for the deep sustainability transformation. Germany: Oekom Verlag.
Langsenkamp, F., Sellmann, F., Kohlbrecher, M., Kielhorn, A., Strothmann, W., Michaels, A., et al. (2014). Tube stamp for mechanical intra-row individual plant weed control. Available online at: https://www.hs-osnabrueck.de/fileadmin/HSOS/Homepages/COALA/Veroeffentlichungen/2014-CIGR_2014_Tube_Stamp_for_mechanical_intra-row_individual_Plant_Weed_Control.pdf.
Lati, R. N., Siemens, M. C., Rachuy, J. S., and Fennimore, S. A. (2016). Intrarow weed removal in broccoli and transplanted lettuce with an intelligent cultivator. Weed Technol. 30, 655–663. doi: 10.1614/WT-D-15-00179.1
LfULG. (2020). Positionspaper zum workshop - farm management information system (FMIS). Available online at: https://www.landwirtschaft.sachsen.de/download/Positionspapier_betriebliches_Datenmanagement_und_FMIS.pdf.
Li, B., Wu, S., Zhou, S., Wang, T., and Chen, H. (2017). Quantifying and mapping threats to soil biodiversity in Nanjing, China. Eur. J. Soil Biol. 82, 72–80. doi: 10.1016/j.ejsobi.2017.08.007
Librán-Embid, F., Klaus, F., Tscharntke, T., and Grass, I. (2020). Unmanned aerial vehicles for biodiversity-friendly agricultural landscapes - A systematic review. Sci. Total Environ. 732:139204. doi: 10.1016/j.scitotenv.2020.139204
Lioutas, E. D., Charatsari, C., and De Rosa, M. (2021). Digitalization of agriculture: A way to solve the food problem or a trolley dilemma? Technol. Soc. 67:101744. doi: 10.1016/j.techsoc.2021.101744
Ludwig-Ohm, S., Hildner, P., Isaak, M., Dirksmeyer, W., and Schattenberg, J. (2023). The contribution of horticulture 4.0 innovations to more sustainable horticulture. Proc. Comput. Sci. 217, 465–477. doi: 10.1016/j.procs.2022.12.242
Lundström, C., and Lindblom, J. (2018). Considering farmers’ situated knowledge of using agricultural decision support systems (AgriDSS) to Foster farming practices: the case of CropSAT. Agric. Syst. 159, 9–20. doi: 10.1016/j.agsy.2017.10.004
Lutz, K. J. (2017). “Digitalisierung der Landwirtschaft: Revolution mit evolutionärem Charakter” in Management-Reihe Corporate Social Responsibility. CSR und Digitalisierung: Der digitale Wandel als Chance und Herausforderung für Wirtschaft und Gesellschaft. eds. A. Hildebrandt and W. Landhäußer (Berlin: Springer Gabler), 429–442.
Luyckx, M., and Reins, L. (2022). The future of farming: the (non)-sense of big data predictive tools for sustainable EU agriculture. Sustain. For. 14:12968. doi: 10.3390/su142012968
Mäder, P., Boho, D., Rzanny, M., Seeland, M., Wittich, H. C., Deggelmann, A., et al. (2021). The Flora incognita app – interactive plant species identification. Methods Ecol. Evol. 12, 1335–1342. doi: 10.1111/2041-210X.13611
Madlener, R., and Alcott, B. (2011). Herausforderungen für eine technisch-ökonomische Entkoppelung von Naturverbrauch und Wirtschaftswachstum. Unter besonderer Berücksichtigung der Systematisierung von Rebound-Effekten und Problemverschiebungen: Enquette-Kommission Wachstum, Wohlstand, Lebensqualität. Available online at: http://webarchiv.bundestag.de/archive/2013/0510/bundestag/gremien/enquete/wachstum/gutachten/m17-26-13.pdf.
Maes, J., Teller, A., Erhard, M., Conde, S., Vallecillo Rodriguez, S., Barredo Cano, J. I., et al. (2020). Mapping and assessment of ecosystems and their services - an EU wide ecosystem assessment in support of the EU biodiversity strategy.
Martens, K., and Zscheischler, J. (2022). The digital transformation of the agricultural value chain: discourses on opportunities, challenges and controversial perspectives on governance approaches. Sustain. For. 14:3905. doi: 10.3390/su14073905
Mathanker, S. K., Weckler, P. R., Taylor, R. K., and Fan, G. (2010). Adaboost and support vector machine classifiers for automatic weed control: Canola and wheat. ASABE Annual International Meeting, No. 1008834.
McKinley, D. C., Miller-Rushing, A. J., Ballard, H. L., Bonney, R., Brown, H., Cook-Patton, S. C., et al. (2017). Citizen science can improve conservation science, natural resource management, and environmental protection. Biol. Conserv. 208, 15–28. doi: 10.1016/j.biocon.2016.05.015
Meinig, H., Boye, P., Dähne, M., Hutterer, R., and Lang, J. (2020). “Rote Liste und Gesamtartenliste der Säugetiere (Mammalia) Deutschlands” in Naturschutz und Biologische Vielfalt: Heft 170,2 (Bundesamt für: Naturschutz).
Melzer, M., and Bellingrath-Kimura, S. (2021). GIS-gestützte Modellierung von Ökosystemleistungen – Status quo, Potenziale und Trade-offs. Modellierung von Ökosystemleistungen, pp. 115807–115468.
Melzer, M., Bellingrath-Kimura, S., and Gandorfer, M. (2023). Commercial farm management information systems - A demand-oriented analysis of functions in practical use. Smart Agric. Technol. 4:100203. doi: 10.1016/j.atech.2023.100203
Millenium Ecosystem Assessment. (2005). Ecosystems and human well-being: synthesis. Available online at: https://www.millenniumassessment.org/documents/document.356.aspx.pdf.
Mokhtari, A., Ahmadi, A., Daccache, A., and Drechsler, K. (2021). Actual evapotranspiration from UAV images: A multi-sensor data fusion approach. Remote Sens. 13:2315. doi: 10.3390/rs13122315
Molls, C. (2021). The Obs-services and their potentials for biodiversity data assessments with a test of the current reliability of photo-identification of Coleoptera in the field. Tijdschr. Entomol. 164, 143–153. doi: 10.1163/22119434-bja10018
Mouratiadou, I., Lemke, N., Chen, C., Wartenberg, A., Bloch, R., Donat, M., et al. (2023). The digital agricultural knowledge and information system (DAKIS): employing digitalisation to encourage diversified and multifunctional agricultural systems. Environ. Sci. Ecotechnol. 16:100274. doi: 10.1016/j.ese.2023.100274
Mukhamedova, K. R., Cherepkova, N. P., Korotkov, A. V., Dagasheva, Z. B., and Tvaronavičienė, M. (2022). Digitalisation of agricultural production for precision farming: A case study. Sustain. For. 14:14802. doi: 10.3390/su142214802
Mulero-Pázmány, M., Jenni-Eiermann, S., Strebel, N., Sattler, T., Negro, J. J., and Tablado, Z. (2017). Unmanned aircraft systems as a new source of disturbance for wildlife: A systematic review. PLoS One 12:e0178448. doi: 10.1371/journal.pone.0178448
Munz, J., Gindele, N., and Doluschitz, R. (2020). Exploring the characteristics and utilisation of farm management information systems (FMIS) in Germany. Comput. Electron. Agric. 170:105246. doi: 10.1016/j.compag.2020.105246
Muscalu, A., Tudora, C., Sorică, C., and Burnichi, F. (2019). Weed control methods for organic vegetable crops. In I. Kiss (chair), Acta Technica Corviniensis - bulletin of engineering: tome XII [2019]. Fascicule 2 [April-June]. Available online at: https://acta.fih.upt.ro/pdf/2019-2/ACTA-2019-2-04.pdf.
Noack, P. O. (2018). Einsatz von Multi- und Hyperspektralsensoren in der Landwirtschaft. In: Wissenschaftlich-Technische Jahrestagung der DGPF und PFGK18 Tagung, München. Available online at: https://www.dgpf.de/src/tagung/jt2018/proceedings/proceedings/papers/16_PFGK18_Noack.pdf.
Novkovic, N., Husemann, C., Zoranovic, T., and Mutavdzic, B. (2017). Farm management Infromation system: case study. Proceedings of the 8th international conference on information and communication technologies in agriculture, food and environment (HAICTA 2017), Chania, Crete Island, Greece. Available online at: https://ceur-ws.org/Vol-2030/HAICTA_2017_paper53.pdf.
Ogawa, K., Lin, Y., Takeda, H., Hashimoto, K., Konno, Y., and Mori, K. (2021). “Automated counting wild birds on UAV image using deep learning” in Igarss 2021–2021 IEEE international geoscience and remote sensing symposium: Proceedings: 12–16 July, 2021, virtual symposium, Brussels, Belgium. eds. D. Miralles, C. Persello, and K. Beenen (Brussels, Belgium: IEEE), 5259–5262.
Osten, V., and Cattel, A. (2011). WeedSeeker technology can be used effectively in wide-row crops in dryland broadacre farming systems. Available online at: https://researchoutput.csu.edu.au/files/9704340/29497_weston_23rd%20apwss%20conference%20proceedings%20vol%201.pdf#page=411
Pärtel, J., Pärtel, M., and Wäldchen, J. (2021). Plant image identification application demonstrates high accuracy in northern Europe. AoB Plants 13:plab050. doi: 10.1093/aobpla/plab050
Patrício, D. I., and Rieder, R. (2018). Computer vision and artificial intelligence in precision agriculture for grain crops: A systematic review. Comput. Electron. Agric. 153, 69–81. doi: 10.1016/j.compag.2018.08.001
Peter, M., Diekötter, T., Kremer, K., and Höffler, T. (2021). Citizen science project characteristics: connection to participants’ gains in knowledge and skills. PLoS One 16:e0253692. doi: 10.1371/journal.pone.0253692
Pohle, M. (2021). So werden Rehkitze in Redefin vor der Mähmaschine bewahrt. SVZ. Available online at: https://www.svz.de/lokales/hagenower-kreisblatt/Neue-Drohne-spuert-zuverlaessig-jedes-Rehkitz-im-Feld-auf-id32449777.html.
Poppe, K., Vrolijk, H., and Bosloper, I. (2023). Integration of farm financial accounting and farm management information Systems for Better Sustainability Reporting. Electronics 12:1485. doi: 10.3390/electronics12061485
Pratap, T. (2019). Performance of wheat (Triticum aestivum L.) under need based nitrogen management strategies and tillage options using active crop canopy sensor. Available online at: https://krishikosh.egranth.ac.in/handle/1/5810156805.
Psiroukis, V., Malounas, I., Mylonas, N., Grivakis, K.-E., Fountas, S., and Hadjigeorgiou, I. (2021). Monitoring of free-range rabbits using aerial thermal imaging. Smart Agric. Technol. 1:100002. doi: 10.1016/j.atech.2021.100002
Radicioni, F., Stoppini, A., Brigante, R., Brozzi, A., and Tosi, G. (2020). “Gnss network RTK for automatic guidance in agriculture: testing and performance evaluation” in Springer eBook collection: Vol. 12253, computational science and its applications – ICCSA 2020: 20th international conference, Cagliari, Italy, July 1–4, 2020, proceedings, part V. eds. O. Gervasi, B. Murgante, S. Misra, C. Garau, I. Blečić, and D. Taniar. 1st ed (Berlin: Springer International Publishing), 19–35.
Reckleben, Y. (2014). Sensoren für die Stickstoffdüngung - Erfahrungen in 12 Jahren praktischem Einsatz. J. Kulturpflanzen 66:2. doi: 10.5073/JFK.2014.02.02
Reger, M., Bauerdick, J., and Bernhardt, H. (2018). Drohnen in der Landwirtschaft: Aktuelle und zukünftige Rechtslage in Deutschland, der EU, den USA und Japan. Seiten 73:3183. doi: 10.15150/lt.2018.3183
Regulation EU. (2013). Regulation (EU) No 1305/2013 of the European Parliament and of the Council of 17 December 2013 on support for rural development by the European Agricultural Fund for Rural Development (EARFD) and repealing Council Regulation (EC) No 1698/2005, Official journal of the European Union L 347/487. Available online at: https://eur-lex.europa.eu/LexUriServ/LexUriServ.do?uri=OJ:L:2013:347:0487:0548:en:PDF.
Regulation EU. (2016). 2016/679 of the European Parliament and of the council of 27 April 2016 on the protection of natural persons with regard to the processing of personal data and on the free movement of such data, and repealing directive 95/46/EC (general data protection regulation), official journal of the European Union L119/1 Available online at: https://eur-lex.europa.eu/legal-content/EN/TXT/HTML/?uri=CELEX:32016R0679.
Reichel, C., Pascher, P., Scholz, R. W., Berger, G., Brunch, R., Strobel-Unbehaun, T., et al. (2021). “4.1 Agrarökologische Auswirkungen” in Supplementarische Informationen zum DiDaT Weißbuch: Verantwortungsvoller Umgang mit Daten - Orientierungen eines transdisziplinären Prozesses. eds. R. W. Scholz, E. Albrecht, D. Marx, M. Mißler-Behr, O. Renn, and V. Van Zyl-Bulitta (Baden-Baden: Nomos), 156–163.
Reiser, D., Sharipov, G. M., Hubel, G., Nannen, V., and Griepentrog, H. W. (2023). Development and experimental validation of an agricultural robotic platform with high traction and low compaction. Agriculture 13:1510. doi: 10.3390/agriculture13081510
Reissig, L., Stoinescu, A., and Mack, G. (2022). Why farmers perceive the use of e-government services as an administrative burden: A conceptual framework on influencing factors. J. Rural. Stud. 89, 387–396. doi: 10.1016/j.jrurstud.2022.01.002
Ries, M., Reinhardt, T., Nigmann, U., and Balzer, S. (2019). Analyse der bundesweiten Roten Listen zum Rückgang der Insekten in Deutschland. Manuskripteinreichung 94, 236–244. doi: 10.17433/6.2019.50153697.236-244
Rijswijk, K., Bulten, E., Klerkx, L., Dessein, J., Debruyne, L., Brunori, G., et al. (2020). Digital transformation of agriculture. Forestry and Rural Areas: Developing a futureproof Socio-Cyber-Physical System.
Robert, C., Sotiropoulos, T., Waeselynck, H., Guiochet, J., and Vernhes, S. (2020). The virtual lands of Oz: testing an agribot in simulation. Empir. Softw. Eng. 25, 2025–2054. doi: 10.1007/s10664-020-09800-3
Rodriguez-Solano, C., Brandl, M., Chen, X., Herwig, M., Kipka, A., Kreikenbohm, P., et al. (2019). Integrity real-time performance of the Trimble RTX correction service. In: Proceedings of the 32nd international technical meeting of the satellite division of the Institute of Navigation (ION GNSS+ 2019), Miami, Florida.
Rogovska, N., Laird, D. A., Chiou, C.-P., and Bond, L. J. (2019). Development of field mobile soil nitrate sensor technology to facilitate precision fertilizer management. Precis. Agric. 20, 40–55. doi: 10.1007/s11119-018-9579-0
Ryan, M. (2020). Agricultural big data analytics and the ethics of power. J. Agric. Environ. Ethics 33, 49–69. doi: 10.1007/s10806-019-09812-0
Saha, H. N., Roy, R., Chakraborty, M., and Sarkar, C. (2021). “IoT-enabled agricultural system application, challenges and security issues” in Agricultural Informatics: Automation Using the IoT and Machine Learning. eds. A. Choudhury, A. Biswas, M. Prateek, and A. Chakrabarti (Hoboken, NJ: Wiley), 223–247.
Saiz-Rubio, V., and Rovira-Más, F. (2020). From smart farming towards agriculture 5.0: A review on crop data management. Agronomy 10:207. doi: 10.3390/agronomy10020207
Santangeli, A., Chen, Y., Kluen, E., Chirumamilla, R., Tiainen, J., and Loehr, J. (2020). Integrating drone-borne thermal imaging with artificial intelligence to locate bird nests on agricultural land. Sci. Rep. 10:10993. doi: 10.1038/s41598-020-67898-3
Sarwar, F., Griffin, A., Rehman, S. U., and Pasang, T. (2021). Detecting sheep in UAV images. Comput. Electron. Agric. 187:106219. doi: 10.1016/j.compag.2021.106219
Schiller, C., Schmidtlein, S., Boonman, C., Moreno-Martínez, A., and Kattenborn, T. (2021). Deep learning and citizen science enable automated plant trait predictions from photographs. Sci. Rep. 11:16395. doi: 10.1038/s41598-021-95616-0
Schleichler, S., and Gandorfer, M. (2018). “Digitalisierung in der Landwirtschaft: Eine Analyse der Akzeptanzhemmnisse” in Referate der GIL-Jahrestagung: Vol. 38, Informatik in der Land-, Forst- und Ernährungswirtschaft: Fokus: Digitale Marktplätze und Plattformen: Referate der 38. Gil-Jahrestagung 26.-27. Februar 2018, Kiel, Germany. eds. A. Ruckelshausen, A. Meyer-Aurich, K. Borchard, C. Hofacker, J. P. Loy, and R. Schwerdtfeger (Germany: Köllen Druck and Verlag GmbH), 203–206.
Schnebelin, É., Labarthe, P., and Touzard, J.-M. (2021). How digitalisation interacts with ecologisation? Perspectives from actors of the French agricultural innovation system. J. Rural. Stud. 86, 599–610. doi: 10.1016/j.jrurstud.2021.07.023
Schrader, C. (2017). Drohnen und Naturschutz(recht). Nat. Recht 39, 378–385. doi: 10.1007/s10357-017-3189-x
Schwich, S., Stasewitsch, I., Fricke, M., and Schattenberg, J. (2018). “Übersicht zur Feld-Robotik in der Landtechnik” in Jahrbuch Agrartechnik 2018: Digitalisierung und Automatisierung Assistenzsysteme. ed. L. Frerichs (Germany: Institut für mobile Maschinen und Nutzfahrzeuge), 36–46.
Sedera, D., Lokuge, S., Grover, V., and Sarker, S. (2016). Innovating with enterprise systems and digital platforms: A contingent resource-based theory view. Inf. Manag. 53, 366–379. doi: 10.1016/j.im.2016.01.001
Shah, T. M., Nasika, D. P. B., and Otterpohl, R. (2021). Plant and weed identifier robot as an Agroecological tool using artificial neural networks for image identification. Agriculture 11:222. doi: 10.3390/agriculture11030222
Simionescu, M., Bilan, Y., Gędek, S., and Streimikiene, D. (2019). The effects of greenhouse gas emissions on cereal production in the European Union. Sustain. For. 11:3433. doi: 10.3390/su11123433
Simon, R., Hochradel, K., Mages, J., Nagy, M., Naucke, A., Niermann, I., et al. (2015). “Methoden akustischer Erfassung der Fledermausaktivität an Windenergieanlagen” in Reduktion des Kollisionsrisikos von Fledermäusen an Onshore-Windenergieanlagen (RENEBAT II): Schriftenreihe Institut für Umweltplanung. eds. O. Behr, R. Brinkmann, F. Korner-Nievergelt, M. Nagy, I. Niermann, and M. Reich, 40–79.
Söderström, M., Stadig, H., Martinsson, J., Pikki, K., and Stenberg, M. (2016). Cropsat – A public satellite-based decision support system for variable-rate nitrogen fertilization in Scandinavia.
Soto, I., Barnes, A., Balafoutis, A., Beck, B., Sanchez, B., Vangeyte, J., et al. (2019). The contribution of precision agriculture technologies to farm productivity and the mitigation of greenhouse gas emissions in the EU: Jrc technical reports. JRC technical reports. Publications Office of the European Union.
Spiegel, H., Sandén, T., Essl, L., and Vuolo, F. (2021). “Toward improved nitrogen fertilization with precision farming based on sensor and satellite technologies” in Women in engineering and science. Women in precision agriculture: technological breakthroughs, challenges and aspirations for a prosperous and sustainable future. ed. T. K. Hamrita. 1st ed (Berlin: Springer International Publishing), 69–83.
Steinherr, L., Krachunova, T., Birkmann, A., Acosta Ortiz, D. I., Bloch, R., and Bellingrath-Kimura, S. D. (2023). “Unkrautregulierung im ökologischen Zuckerrübenanbau in der Uckermark - Erprobung und Bewertung des autonomen Feldroboter Uckerbot” in Digital tools, big data, modelling and sensing methods for sustainable and climate smart crop and grassland systems. eds. H. P. K. Neugschwandtner and L. Francke-Weltmann (Berlin: Verlag Liddy Halm), 131–132.
Steinmann, H. H. (2020). Unkrautmanagement im Zeichen von Biodiversität, Glyphosatkrise und knappen Ressourcen – Versuch einer Zwischenbilanz. Seiten 22:2. doi: 10.5073/jka.2020.464.002
Steward, B., Gai, J., and Tang, L. (2019). “The use of agricultural robots in weed management and control” in Burleigh Dodds series in agricultural science: Vol. 44. Robotics and automation for improving agriculture. ed. J. Billingsley (Berlin: Burleigh Dodds Science Publishing), 161–186.
Streimelweger, R., Handl, L., and Hauer, L. (2020). Bericht zum Forschungsprojekt GIS-ELA: GIS-ELA_FMIS_Übersicht_2020. Available online at: https://gis-ela.josephinum.at/documents/BERICHT_LW_4.0_FMIS_%C3%9Cbersicht_2019.pdf
Tansey, K., Chambers, I., Anstee, A., Denniss, A., and Lamb, A. (2009). Object-oriented classification of very high resolution airborne imagery for the extraction of hedgerows and field margin cover in agricultural areas. Appl. Geogr. 29, 145–157. doi: 10.1016/j.apgeog.2008.08.004
Techen, A.-K., and Helming, K. (2017). Pressures on soil functions from soil management in Germany. A foresight review. Agron. Sustain. Dev. 37:473. doi: 10.1007/s13593-017-0473-3
Tsouros, D. C., Bibi, S., and Sarigiannidis, P. G. (2019). A review on UAV-based applications for precision agriculture. Information 10:349. doi: 10.3390/info10110349
Tummers, J., Kassahun, A., and Tekinerdogan, B. (2019). Obstacles and features of farm management information systems: A systematic literature review. Comput. Electron. Agric. 157, 189–204. doi: 10.1016/j.compag.2018.12.044
Uwizeyimana, H., Wang, M., Chen, W., and Khan, K. (2017). The eco-toxic effects of pesticide and heavy metal mixtures towards earthworms in soil. Environ. Toxicol. Pharmacol. 55, 20–29. doi: 10.1016/j.etap.2017.08.001
Valerio, F., Basile, M., and Balestrieri, R. (2021). The identification of wildlife-vehicle collision hotspots: citizen science reveals spatial and temporal patterns. Ecol. Process. 10:271. doi: 10.1186/s13717-020-00271-4
Van Bevern, C. (2021). Mit Drohnen gegen Mähtod: Hilfe aus Lengerich für Hopstener Rehkitz-Retter. Neue Osnabrücker Zeitung. Available online at: https://www.noz.de/lokales/lengerich/artikel/2328432/hopstener-naturschuetzer-retten-rehkitze-mit-drohnen-vor-maehtod.
Van de Gevel, J., van Etten, J., and Deterding, S. (2020). Citizen science breathes new life into participatory agricultural research. A review. Agron. Sustain. Dev. 40:636. doi: 10.1007/s13593-020-00636-1
Van der Velden, M. (2018). Digitalisation and the UN sustainable development goals: what role for design. ID&A Interact. Des. Arch. 37, 160–174. doi: 10.55612/s-5002-037-008
Van Evert, F. K., Been, T., Booij, A. J., Kempenaar, C., Kesser, J. G., and Molendijk, P. L. (2018). Akkerweb: a platform for precision farming data, science, and practice. 14th international conference on precision agriculture, Montreal, Quebec, Canada.
Van Evert, F. K., Fountas, S., Jakovetic, D., Crnojevic, V., Travlos, I., and Kempenaar, C. (2017). Big data for weed control and crop protection. Weed Res. 57, 218–233. doi: 10.1111/wre.12255
Villa-Henriksen, A., Edwards, G. T., Pesonen, L. A., Green, O., and Sørensen, C. A. G. (2020). Internet of things in arable farming: implementation, applications, challenges and potential. Biosyst. Eng. 191, 60–84. doi: 10.1016/j.biosystemseng.2019.12.013
Vlaminck, M., Diels, L., Philips, W., Maes, W., Heim, R., de Wit, B., et al. (2023). A multisensor UAV payload and processing pipeline for generating multispectral point clouds. Remote Sens. 15:1524. doi: 10.3390/rs15061524
Vogel, P. (2020). Datenhoheit in der Landwirtschaft 4.0. In: GI-Edition. Proceedings: Vol. 299, GI Edition Proceedings Band 299 Informatik in der Land-, Forst- und Ernährungswirtschaft: 40. GIL-Jahrestagung 17–18. Februar 2020 Campus Weihenstephan, Freising. Köllen, pp. 331–336.
Vohland, K., Land-Zandstra, A., Ceccaroni, L., Lemmens, R., Perelló, J., Ponti, M., et al. (2021). Springer eBook collection. The science of citizen science. Berlin: Springer.
Volpi, I., Bosco, S., Guidotti, D., Mammini, M., Neri, S., Virgili, G., et al. (2020). “Improving GHG flux monitoring in agricultural soil through the AGRESTIC prototype: A focus on the assessment of data quality” in 2020 IEEE international workshop on metrology for agriculture and forestry: Metroagrifor: Virtual conference: Proceedings. eds. A. Berardinelli, D. Brunelli, P. Daponte, and D. Petri (Piscataway, NJ: IEEE), 139–143.
Waterhouse, T. (2023). Virtual fencing systems: balancing production and welfare outcomes. Livestock 28, 227–234. doi: 10.12968/live.2023.28.5.227
WDR. (2021). Neue Drohnen retten Rehkitze vor Mäh-Tod. Available online at: https://www1.wdr.de/nachrichten/landespolitik/umwelt-tierschutz-100.html.
WEF. (2020). The Global Risks Report 2020. Available online at: https://www3.weforum.org/docs/WEF_Global_Risk_Report_2020.pdf.
Weller von Ahlefeld, P. J. (2019). Rebound Effekte in der Präzisionslandwirtschaft - Ein Kommentar. Berich. Landwirtsch 675:452. doi: 10.12767/buel.v97i3.247.g452
Weltzien, C. (2016). Digital agriculture - or why agriculture 4.0 still offers only modest returns. Landtechnik 71, 66–68. doi: 10.15150/lt.2015.3123
Wilson, A. M., Boyle, K. S., Gilmore, J. L., Kiefer, C. J., and Walker, M. F. (2022). Species-specific responses of bird song output in the presence of drones. Drones 6:1. doi: 10.3390/drones6010001
Wolters, S., Söderström, M., Piikki, K., Reese, H., and Stenberg, M. (2021). Upscaling proximal sensor N-uptake predictions in winter wheat (Triticum aestivum L.) with Sentinel-2 satellite data for use in a decision support system. Precis. Agric. 22, 1263–1283. doi: 10.1007/s11119-020-09783-7
Wood, T., Ramakrishnan, K. K., Hwang, J., Liu, G., and Zhang, W. (2015). Toward a software-based network: integrating software defined networking and network function virtualization. IEEE Netw. 29, 36–41. doi: 10.1109/MNET.2015.7113223
Zanin, A. R. A., Neves, D. C., Teodoro, L. P. R., Da Silva Júnior, C. A., Da Silva, S. P., Teodoro, P. E., et al. (2022). Reduction of pesticide application via real-time precision spraying. Sci. Rep. 12:5638. doi: 10.1038/s41598-022-09607-w
Zhang, N., Yang, G., Pan, Y., Yang, X., Chen, L., and Zhao, C. (2020). A review of advanced technologies and development for hyperspectral-based plant disease detection in the past three decades. Remote Sens. 12:3188. doi: 10.3390/rs12193188
ZKL. (2021). Zukunft Landwirtschaft. Eine gesamtgesellschaftliche Aufgabe: Empfehlungen der Zukunftskommission Landwirtschaft. Available at: https://www.bundesregierung.de/resource/blob/975226/1939908/2c63a7d6ce38e8c92aa5f73aff1cd87a/2021-07-06-zukunftskommission-landwirtschaft-data.pdf?download=1.
Zscheischler, J., Brunsch, R., Griepentrog, H. W., Tölle-Nolting, C., Rogga, S., Berger, G., et al. (2021). “Kapitel 4 Landwirtschaft, Digitalisierung und digitale Daten” in DiDaT Weißbuch: Verantwortungsvoller Umgang mit digitalen Daten - Orientierungen eines transdisziplinären Prozesses. eds. R. W. Scholz, M. Beckedahl, S. N. O. Albrecht, and D. Marx (Baden-Baden: Nomos), 145–168.
Keywords: biodiversity protection, crop management, sensors, smart technologies, sustainable agriculture smart technologies, sustainable agriculture
Citation: Krachunova T, Geppert F, Lemke N and Bellingrath-Kimura SD (2025) Digital technologies commercially available in Germany in the context of nature conservation and ecosystem service provisioning in agriculture. Front. Sustain. Food Syst. 9:1464020. doi: 10.3389/fsufs.2025.1464020
Edited by:
Rishi Raj, Indian Agricultural Research Institute (ICAR), IndiaReviewed by:
Luciano Gebler, Brazilian Agricultural Research Corporation (EMBRAPA), BrazilCarlo Grignani, University of Turin, Italy
Copyright © 2025 Krachunova, Geppert, Lemke and Bellingrath-Kimura. This is an open-access article distributed under the terms of the Creative Commons Attribution License (CC BY). The use, distribution or reproduction in other forums is permitted, provided the original author(s) and the copyright owner(s) are credited and that the original publication in this journal is cited, in accordance with accepted academic practice. No use, distribution or reproduction is permitted which does not comply with these terms.
*Correspondence: Tsvetelina Krachunova, VHN2ZXRlbGluYS5LcmFjaHVub3ZhQHphbGYuZGU=