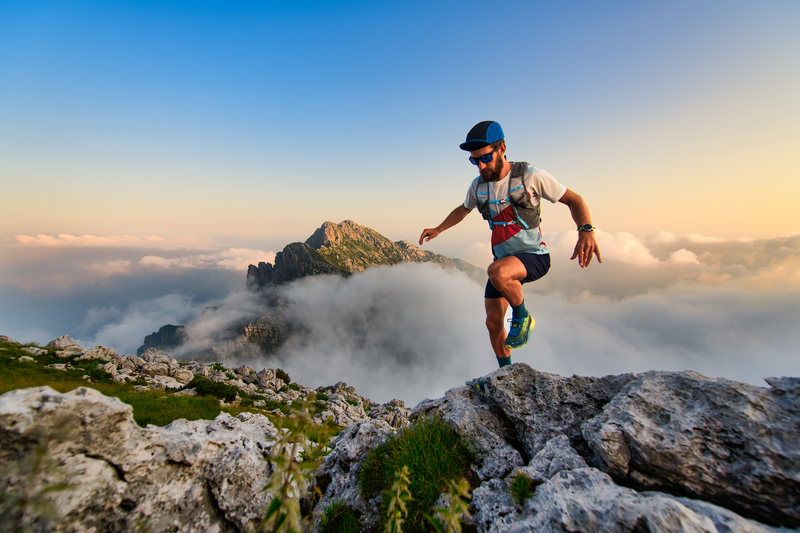
95% of researchers rate our articles as excellent or good
Learn more about the work of our research integrity team to safeguard the quality of each article we publish.
Find out more
ORIGINAL RESEARCH article
Front. Sustain. Food Syst. , 26 March 2025
Sec. Agricultural and Food Economics
Volume 9 - 2025 | https://doi.org/10.3389/fsufs.2025.1432501
This article is part of the Research Topic Economic visions to mitigate Climate Change View all 4 articles
China faces multiple pressures on productivity enhancement, ecological protection and livelihood improvement, and how to reconcile these objectives is an important consideration in the choice of strategies for agricultural green development. In this study, we try to propose a comprehensive approach to consider multiple objectives of agricultural green development from a system dynamics perspective, which aims to identify the synergies and trade-offs among objectives under different scenarios in the National Pilot Zones for Green Development of Agriculture in China, and Xuzhou City and Nanping City are selected as two different target areas. The research demonstrates that system dynamics modeling can effectively facilitate strategic choices for decision-makers. There are upward trends in the system in terms of the agricultural improvement index, gross food production, and farmers’ income in the two target pilot zones, which can achieve overall synergy before 2030, although a trade-off relationship between food production and environmental protection. Furthermore, the agricultural green transformation index based on different scenarios could guide strategic choices. Xuzhou in economically prosperous zones has a leading level of index for the green transition scenario (reaching 83.76 in 2030, surpassing the other two scenarios), and shows greater potential to prioritize green transition for high-quality development. Meanwhile, the business-as-usual scenario for economically less-developed city of Nanping has the highest index of 97.61 in 2030, indicating the need for supportive policies to maintain the agricultural transition process. In brief, strategic decisions for green transformation in China should be based on the structural characteristics and development goals of the agricultural system, with an emphasis on both short-term progress and long-term trends.
Agriculture is a fundamental sector of the national economy, ensuring food security and serving as a cornerstone of social stability (Wang W. et al., 2022). As we know, intensive agricultural practices have resulted in resource depletion, environmental degradation, and climate change, globally constraining the achievement of SDGs (Dardonville et al., 2020; Deng and Zeng, 2022; Tao et al., 2023). For instance, greenhouse gas emissions and agricultural land pollution have actually threatened agricultural sustainable development in many countries (Mbow et al., 2019; Zhang R. et al., 2022; IPCC, 2023). By 2050, feeding nearly 10 billion people worldwide will be a challenge, relying on sustainable solutions to address resource scarcity and ecological degradation (FAO, 2018). Furthermore, with the rising cost of food production and the widening gap between urban and rural incomes, the issue of farmers’ livelihoods has undermined the sustainability of agricultural systems (FAO, IFAD, UNICEF, WFP and WHO, 2023; Yu et al., 2020; Zhang et al., 2023). Regarding these multiple challenges, there is an urgent need for a resource-efficient, environmentally friendly and highly profitable pattern of agricultural development (Hansen et al., 2020; Guo et al., 2021).
As a major agricultural country, China’s ability to feed 22% of the world’s population with just 9% of the arable land has largely depended on intensive chemical inputs (Ghose, 2014). Meanwhile, the widespread use of chemical fertilizers and pesticides has resulted in severe pollution and environmental degradation. In 2021, agricultural sources accounted for 66.2% of the country’s chemical oxygen demand emissions (Ministry of Ecology and Environment of the People’s Republic of China, 2023), and soil organic carbon levels remain significantly lower than those in developed countries (Ding et al., 2023). Transforming agricultural production is critical for China’s sustainable development and the pursuit of harmonious human-nature coexistence. Agricultural green development has emerged as a critical strategy to balance productivity enhancement, environmental protection, and livelihood improvement (Musvoto et al., 2018; Chen and Li, 2023). Consequently, China has made great efforts toward green transformation, establishing the first batch of the National Pilot Zones for Green Development of Agriculture in 2017. These efforts focus on exploring mechanisms for the green transformation of agriculture, considering different ecological types by integrating factors such as resource endowments, regional characteristics, and local development foundations, with the objective of providing patterns for the sustainable development in agricultural sector.
Agriculture operates as a complex natural-economic-social system, and how to coordinate the three core objectives of agricultural green development (productivity, ecosystem health, and livelihoods) is determined by the interconnections and dynamics of components within the agricultural system (Fader et al., 2018; Barbier, 2020; Jagustović et al., 2021). Existing studies primarily focus on static relationships of single objectives (Xu et al., 2024), or adopted linear models to simplify interactions among system components, lacking exploration of the dynamic feedback and long-term evolutionary trends of the system (Egerer et al., 2021; Zhang et al., 2023). Due to heterogeneity in resource endowment characteristics, levels of economic development, and the functional positioning of agriculture across different regions, the outcomes of objective interactions are different (Tripathi et al., 2022). Thus, it might be important to pay considerable attention to the trends of agricultural green transformation practices in different regions.
To bridge this research gap, integrated simulation methods are essential for analyzing the dynamic evolution of agricultural systems under policy interventions (Elsawah et al., 2017). This study employs a system dynamics approach to quantitatively assess agricultural green development effectiveness in different pilot zones, with the following research objectives: (1) analyze trade-offs and synergies among ecological, production and livelihood subsystems; (2) identify differences in the long-term evolutionary trajectories of agricultural systems under different scenarios; and (3) guide strategic decision-making based on trend projections. To address these issues, we developed a system dynamics model to simulate changes of agricultural systems in the National Pilot Zones for Green Development of Agriculture, aiming to facilitate strategic choices for the green transformation of agricultural systems and provide a foundation for guiding green transformation pathways by identifying the synergies and trade-offs in coordinating ecological protection, productivity enhancement, and livelihood improvement under different scenarios.
The coordination of multiple objectives in agricultural systems has become a critical research topic in recent years. Agricultural green development is a key strategy for achieving this coordination by promoting resource efficiency and optimizing allocation to balance multiple development objectives (Liu et al., 2020; Zhang H. et al., 2022; Lankoski and Lankoski, 2023; Sarfraz et al., 2022). Current research hotspots on agricultural green development primarily emphasizes system evaluation and examines the static relationships between individual objectives, such as production efficiency, environmental protection, and industrial chain construction (Xu et al., 2024). For instance, some studies employ Data Envelopment Analysis to quantify agro-ecological efficiency (Yu et al., 2020) or utilize life cycle assessment to identify key influencing factors (Wang W. et al., 2023). However, these studies often ignore the impacts on other dimensions, while linear models fail to capture the complex interactions and dynamic feedback within agricultural systems (Egerer et al., 2021; Zhang et al., 2023), leading to the lack of information available for informed governmental decision-making and policy adjustments. The challenge of identifying agricultural strategies that reconcile multiple objectives within a dynamic evolutionary framework remains unresolved (Barbier, 2020; Ali et al., 2022), highlighting the need for a systemic approach to explore the intricate interactions among ecological, economic, and social dimensions.
Model simulation plays a vital role in understanding the evolution of complex systems (Walters et al., 2016). System dynamics is a computer simulation methodology that employs systems theory, cybernetics, and information feedback theory to address non-linear and complex system problems (Ostrom, 2009; Arshad et al., 2015). This modeling approach simulates and predicts the dynamic evolution of a system by capturing relationships between elements and abstracting the real world (Nazir et al., 2018). Consequently, system dynamics has been widely applied in sustainability research, including carbon emission reduction in industrial sectors (Liu et al., 2023; Liu et al., 2024), agricultural sustainability (Egerer et al., 2021), climate-smart villages (Jagustović et al., 2021), and agro-ecosystem resilience (Hosseinzadeh et al., 2022). Based on the premise that the structure of a system determines its function, this method endeavors to measure the intricate interplay by establishing causal relationships and feedback loops connecting various components of the system (Elsawah et al., 2017). Computer technologies enable us to effectively implement the simulation, modeling, and analysis of system dynamics and behaviors (Datola et al., 2022). Notably, this methodology offers compelling advantages in tackling challenges characterized by lengthy timeframes and intricate relationships (Li et al., 2023). In addition, system dynamics models support synergy and trade-off analyzes in scenarios with multiple attributes, allowing for the assessment and simulation of long-term outcomes resulting from different policy interventions (Nazir et al., 2018; Weiskopf et al., 2020; Wang W. et al., 2022). Therefore, this study integrates ecological, economic, and social subsystems into a SD model, providing forward-looking insights to guide the agricultural green transformation.
Two National Pilot Zones for Green Development of Agriculture, Xuzhou City in Jiangsu Province and Nanping City in Fujian Province, are selected as target study areas (Supplementary material 1).
Xuzhou City is situated on the north–south divide in China, within the agricultural region of the middle and lower reaches of the Yangtze River. The city encompasses a total area of 11,765 square kilometers, including 576,673 hectares of arable land. Xuzhou is a representative agricultural area and a national base for staple food production. Its comprehensive mechanization rate of grain crops and contribution rate of agricultural technology progress exceed the national average by nearly 10%. With notable advantages in the application of green technologies and equipment, Xuzhou plays a vital role in securing agricultural product supply. Furthermore, its economic development level is at the forefront of prefecture-level cities in China, characterized by robust economic momentum, rapid growth of rural industries, and strong local government support. These factors create a solid foundation for the region’s agricultural green transformation. As Xuzhou pursues the ecological enhancement of agricultural productivity, its transformation trajectory will serve as a valuable model for other grain-producing regions with a robust agricultural foundation in China.
Nanping City is in the north of Fujian Province, covering a land area of 26,280 square kilometers and holding 237,960 hectares of arable land. The city attains the leading grain production in the province and serves as a crucial supply assurance zone for tropical cash crops in China. Nanping acts as a prominent green barrier in the Minjiang River Basin, exhibiting one of the most sound ecological environments within the same latitude on earth. However, Nanping is an economically underdeveloped region with limited agricultural technology and industrial growth, where large-scale food production has yet to materialize. Although the local government places great emphasis on resource allocation and policy protection for agriculture and rural areas, the primary challenge remains the prioritization of environmental protection and the conversion of ecological resources into green productivity. Thus, Nanping’s agricultural transformation offers a crucial model for promoting sustainable agricultural development within the ecological functional zones of southern China.
Xuzhou and Nanping are both designated optimized development zones in China’s Sustainable Agricultural Development Plan and have been selected as the first batch of the National Pilot Zones for Green Development of Agriculture. As representative regions, these two cities show distinct resource endowments and development objectives, yet both grapple with the complex trade-offs inherent in agricultural transformation. This highlights the key issues in advancing agricultural green development across different regions in China. Consequently, the green transformation pathways of these two study areas offer valuable insights for shaping China’s strategic choices and policy formulation in agriculture.
As agricultural green transformation is a complex long-term progress, we developed a model of system dynamics to investigate the practices of two representative National Pilot Zones for Green Development of Agriculture in China to explore strategic pathways for sustainable development in the future (Figure 1). We simulated regional development trends to analyze the trade-offs and synergies among the three core objectives of green agriculture in terms of improving environmental quality, securing agricultural productivity and enhancing farmers’ well-being. Furthermore, we explored appropriate development strategies for various agricultural zones based on projections of future trajectories under different interventions.
The process of developing the simulation model in this study is divided into five steps: (1) clarification of system boundaries and functions, and identification of key system factors and processes; (2) qualitative modeling of causal feedback processes in the system and establishing causal loops to depict the logical structure of the system; (3) development of stock-and-flow diagrams to establish quantitative relationships among system elements; (4) checking and validation of conceptualized model, and (5) simulation of various system dynamic results for scenario options.
China has consistently advanced the ecological civilization initiatives since 2007, and has driven a significant green transformation since 2012, including agricultural sector with the objective of basically achieving sustainable development by 2030 in agriculture. By using data from the decade prior to 2021, this study simulates and forecasts the agricultural green transformation processes in the regions of Xuzhou and Nanping. The period from 2012 to 2021 is the historical baseline, while 2022 to 2030 is projected as the forecast horizon. The agricultural green development system is a complex, multi-dimensional framework that integrates ecological, economic, and social aspects. To investigate the multi-objective synergies and trade-offs of China’s agricultural green transformation, key variables in the model must be constructed in an authoritative, systematic, and measurable manner. Therefore, we employed the assessment system for National Pilot Zones of Agricultural Green Development, China Agricultural Green Development Report, and the findings of existing literature (Wang S. et al., 2022; Zhang et al., 2023) to develop a holistic model comprising ecological quality, economic efficiency and social livelihood subsystem. Key variables in the system (Table 1) were selected from three perspectives to monitor the dynamic progress toward achieving the triple objectives of agricultural green development, and they are also present in the stock and flow diagram.
The first development objective of improving environmental quality and resource utilization focuses on regional measures for input reduction and resource intensification, which is evaluated by the usage intensity of chemical fertilizer, pesticide, and agricultural film in the ecological quality subsystem, as well as changes in the agricultural improvement index and effective irrigation area over time. The second objective pertains to securing agricultural productivity and food supply during the agricultural green transformation, which is assessed by monitoring indicators such as gross grain production, grain yield per hectare, grain sown area and gross output value of agriculture in the National Pilot Zones for Green Development of Agriculture. Finally, the third objective of enhancing the welfare of farmers is primarily demonstrated by net income from agricultural business and per capita disposable income of rural residents in the social livelihood subsystem.
It is a necessary prerequisite to establish relationship of causal feedback for system dynamics simulation (Lin et al., 2020). As shown in Figure 2, the arrows indicate the causality, and plus/minus signs at the arrowhead show the polarity of the relationships between different variables. The basic causal loop in the model shows that gross grain production increases with the grain yield per hectare, simultaneously having a positive effect on the gross output value of agriculture. In addition, labor inputs and agricultural finance expenditures also influence the level of regional gross agricultural output value. The economic growth in agricultural sector will lead to higher farmers’ business income, resulting in an enhancement of their disposable income. Reducing the use of pesticides and other inputs can improve the agricultural improvement index, which in turn will benefit grain productivity and promote a sustainable transformation of agriculture.
Qualitative modeling processes facilitate the understanding of systems by explaining mechanisms of phenomena (Forbus, 1984). The causal loop diagram emphasizes the system’s feedback structure, while the stock-flow diagram reflects the actual structure behind the system (Lin et al., 2020). There are different types of variables in the stock-flow diagram such as stock variables, rate variables, auxiliary variables, and constants. The stock variables (e.g., population) describe the accumulation of historical behavior and reflect the current state of the system, while the flow variable is the change rate of the stock variable (e.g., population growth rate). Auxiliary variables are mediators that connect different variables, thereby increasing the credibility of the model (Jagustović et al., 2021; Li et al., 2023). In order to illustrate the interactions among objectives within the system boundary, three sub-systems were constructed in this study and merged into an overall model for simulation (Figure 3). There is a significant interdependence between the three sub-models of ecological quality, economic efficiency, and social livelihood. The model generally describes the basic process of agricultural green development in pilot zone, focuses on analyzing the interacting mechanism of components within the system and predicts future trajectories under multiple scenarios.
Figure 3. Stock and flow diagram of the National Pilot Zones for Green Development of Agriculture in China.
The model in this study is developed and parameterized using data from diverse sources, including provincial and municipal Statistical Yearbook, Statistical Communiqué of the People’s Republic of China National Economic and Social Development, China Rural Statistical Yearbook, Bulletin of National Census for Water, Communique of the National Agricultural Census, and statistics from the municipal Bureaus of Agriculture and Rural Affairs and Bureaus of Water Resources.
The temporal evolution is determined by input variables and system structure, therefore quantitative simulation is based on a qualitative model (Coyle, 2000; Jagustović et al., 2021). The equations and parameters in the system mathematically represent the causal relationships between directly linked variables in the conceptual model of stocks and flows. Initial values for state variables are assigned based on historical data. The relationships between auxiliary variables are primarily determined using linear fitting, regression analysis, and lookup functions. Additionally, logical functions are employed in this study to address the issue of matching characteristic equations across different stages. Further information about mathematical expressions and model equations for all variables of SDM are provided in Supplementary material 2.
The testing and validation of the structural and behavioral validity are critical for ensuring confidence in simulation results (Forrester and Senge, 1980; Sterman, 2000; Ten Broeke and Tobi, 2021). The structure test evaluates the model’s validity by comparing it to the relationships of the real system. A boundary test is conducted to verify whether the model includes all the essential variables, processes, and relationships necessary to explain the system’s behaviors. This assessment was based on expert interviews and field investigations, and model checking was performed with the Vensim software, ensuring the integrity of the structure. The behavior validation test is used to determine if the model can accurately reproduce the behavior of the real system. Considering the research requirements, 2–3 variables from each subsystem were selected to fit the simulated curves and historical data. The results (Supplementary material 1) show that the data error between the simulated and real values is within 10%. This demonstrates that the system model is able to verify the behavior of the green development system in agriculture and can be employed for further system simulation and scenario analysis.
In order to analyze the dynamic changing trends in the National Pilot Zones for Green Development of Agriculture in China under different conditions, the historical period of 2012–2021 and the forecast period of 2022–2030 are chosen to verify the agricultural green transformation in Xuzhou and Nanping. According to the objectives outlined in the “14th Five-Year Plan” National Agricultural Green Development Plan, the Plan for Municipal Agriculture and Rural Modernization, the findings from the China Agricultural Green Development Report, and the current situation of agricultural development, we select adjustable variables in each subsystem to create a combined indicator. Accordingly, three development scenarios are proposed (Table 2): a business-as-usual scenario, an economic priority scenario, and a transition to green development scenario. By regulating variables such as the change rates of chemical fertilizer and pesticide use, effective irrigation, machinery power, and the proportion of agricultural financial expenditures, our simulation analysis aims to explore high-quality pathways to the green transition of agriculture in Xuzhou and Nanping.
1. A business-as-usual scenario (BAU). This scenario assumes that the development of ecological, social, and economic subsystems keeps going on as it is going, using the 2021 data as the baseline.
2. An economic priority scenario (EP). This scenario highlights the growth of the agricultural economy, which tends to allocate more resources to agricultural production and emphasizes the economic benefits derived from agricultural sector. Therefore, variables in the economic subsystem, such as the GDP growth rate and the change rate of fertilizer and pesticide usage, are set to their maximum values during the forecast period.
3. A transition to green development scenario (TGD). This scenario prioritizes resource conservation and environmental protection, emphasizing the coordinated development of subsystems to achieve economic, social, and ecological benefits, aiming for the high-quality development of agriculture. Accordingly, some regulating variables are set to the mean values observed during the forecast period in the simulation system.
The entropy weight method (EWM) is a comprehensive evaluation method that establishes objective weights through the differentiation of the measured value (Zhu et al., 2020; Kumar et al., 2020). In this study, we use EWM to construct the agricultural green transformation index to intuitively measure the developing status of the National Pilot Zones for Green Development of Agriculture under different scenarios. Hence, we introduce time variable into the EWM for comparison and calculate weights based on four key indicators: agricultural improvement index, gross grain production, gross output value of agriculture, per capita disposable income of rural residents. The agricultural green transformation index can help us to make strategic choices for green development in the target zones. This specific method is provided in Supplementary material 1.
The following sections present the trends in the three key subsystems of agricultural green development in the two pilot zones. The agricultural improvement index measures the environmental impact of synthetic input use, while agricultural output indicators reflect food supply capacity. Changes in farmers’ incomes indicate the performance of pilot zones in maintaining rural stability. The agricultural green transformation index calculated based on these key variables facilitates us to compare future trajectories of different scenarios for strategic decision-making. The simulation results show that cities with strong economic, technological, and agricultural foundations, like Xuzhou, have the potential to intensify green practices. In contrast, economically less-developed, ecologically focused cities, such as Nanping, will need to maintain current measures based on multi-objective trade-offs.
The utilization of chemical fertilizers, pesticides, agricultural plastic film, and irrigation water plays a crucial role in environmental protection and resource utilization. The impact of green agricultural measures in Xuzhou is remarkable, resulting from the continuous expansion of the effective irrigated area and a steady decline in the utilization of agricultural film. Moreover, the intensity of chemical fertilizers and pesticides shows a continuous downward trend, with estimations suggesting a 24.8% and 32.2% reduction in 2021 compared to 2012 (Figures 4a,c). These positive changes significantly contribute to the increase in Xuzhou’s agricultural improvement index. In contrast, the adoption of agricultural green transformation policies in Nanping was relatively weak, leading to a rapid increase in the intensity of chemical fertilizers, pesticides, and agricultural films, reaching its peak in 2016. As China has been implementing the “Zero Growth Action on Fertilizer and Pesticide Use” since 2015, Nanping is experiencing a substantial decrease in agricultural inputs as well. Consequently, its agricultural improvement index gradually rebounded after reaching its lowest point in 2016 (Figures 4b,d).
Figure 4. Improvements in environmental quality and resource use 2012–2030: (a,b) show intensity of fertilizers, pesticides and agricultural plastic film use in Xuzhou and Nanping; (c,d) show agricultural improvement index and effective irrigation area in Xuzhou and Nanping.
Improving agricultural productivity is primarily assessed through changes in grain yield per hectare, grain sown area, and gross grain production. The model result shows an increasing trend of the grain yield per hectare in Xuzhou, reaching 6,535 kg/ha in 2021. With the rising total power of agricultural machinery and the expanding effective irrigated area, it is projected to increase continuously and reach 6680.6 kg/ha by 2030. Figure 5 indicates that the trends of gross grain production are essentially the same as grain sown area. The northward shift of the agricultural production center in Jiangsu Province has enhanced the role of Xuzhou City in food supply. There has been a notable increase in the grain sown area after 2015, which is expected to stabilize at around 765,000 hectares in the near future. Gross grain production is influenced by both the grain yield per hectare and grain sown area. Despite fluctuations in some years, Xuzhou’s gross grain production surpassed 5 million tons in 2019 and is expected to obtain higher productivity levels under stable production conditions in the future. The model shows a steady upward trend in grain yield per hectare in Nanping as well, increasing by 9% in 2021 compared to 2012. Nevertheless, there is a decline in the local grain sown area leading to a reduction in gross grain production until 2018, after that, the gross grain production continued to increase due to a rise in grain yield per hectare. In addition, the gross output value of agriculture in both pilot zones show an upward trend, which is negatively correlated with labor input but positively affected by government financial expenditure on agriculture sector (Figures 5b,d).
Figure 5. Assurance of agricultural productivity and food supply 2012–2030: (a,b) show grain production per hectare, grain sown area, and gross grain production in Xuzhou and Nanping; (c,d) show gross output value of agriculture, financial expenditure on agriculture and labor input in Xuzhou and Nanping.
Farmers achieved income growth and optimized their income structure over time, thus improving social wellbeing. The simulation results (Figure 6) demonstrate that the increase in farmers’ income in Xuzhou is stable, and the per capita disposable income of rural residents grows from only 10,762 CNY in 2012 to nearly 22,000 CNY in 2021, doubling farmers’ income in the last decade. Farmers’ net income from agricultural rises steadily due to advances in agricultural production and industry development. Meanwhile, its share of disposable income is decreasing, and the structure of farmers’ income becomes more diversified in Xuzhou. Similar results are found in Nanping. Although farmers’ net income from agriculture fluctuated slightly in the early years due to the scale of agricultural production, the per capita disposable income of rural residents still achieved a significant increase, rising from only 8,893 CNY in 2012 to over 20,000 CNY in 2021. After 2022, the growth rate of farmers’ income will significantly exceed that of business income, and the structure of farmers’ income would probably be continuously optimized.
Figure 6. Enhancement of farmers’ social well-being 2012–2030: (a,b) show per capita disposable income of rural residents and net income from agricultural business in Xuzhou and Nanping.
The quantitative analysis above is used to examine the interaction of multi-dimension variables related to goals of agricultural green development, and the subsequent section illustrates projected trajectories of future agricultural transformation in the two pilot zones. Combinations of variables from different scenarios are introduced into the model to simulate the development dynamics of the agricultural system under various pathways. This progress is evaluated through four key variables: the agricultural improvement index, gross grain production, gross output value of agriculture, and per capita disposable income of rural residents. The results of integrating the different subsystems illustrate that the agricultural system in Xuzhou keeps an ongoing improvement process across all scenarios (Figure 7). The EP scenario stands out in enhancing the long-term trend of food production, with an annual growth rate of 3.63% over the forecast period. On the other hand, the TGD scenario demonstrates synergistic improvements across all objectives of green agricultural development. By 2030, the agricultural improvement index is projected to reach 72.21, with an annual growth rate of 11.78% throughout the forecast period. Furthermore, the gross agricultural output value is expected to reach 112 billion CNY. Notably, this scenario also yields a significant increase in per capita disposable income for farmers, projected to reach 48,283 CNY in 2030. This amount is 2.2 times higher than the level of 2021, aligning with the objective outlined in the 10-Year Farmer Income Doubling Plan established by Xuzhou. In contrast, the BAU scenario, characterized by the continuation of the status quo, falls behind the other two development pathways in terms of yield improvement and income enhancement.
Figure 7. Trends of Xuzhou’s agricultural system development under different scenarios: (a) shows agricultural improvement index, (b) reflects per capita disposable income of rural residents, (c) shows the change in gross grain production, and (d) reflects gross output value of agriculture.
The simulation results for Nanping show significant disparities among different development pathways (Figure 8). The EP scenario, which relies on inputs such as chemical fertilizers and pesticides, demonstrates a continuous decline in the agricultural improvement index after 2021. This scenario fails to achieve advantages in terms of gross grain production and gross output value of agriculture, lagging behind both the BAU and TGD scenario. Due to the strict implementation of fertilizer and pesticide reduction measures, the transition to green development scenario is at the forefront of efforts to enhance environmental quality, with the agricultural improvement index expected to exceed 60 in 2030. In addition, it is more effective in securing food supplies in the short term (until 2025) than the others, but this advantage gradually diminishes thereafter. According to the evolutionary trends of the agricultural system, the BAU scenario exhibits better performance in terms of multi-objective synergy. The agricultural improvement index shows a positive trend, with an annual growth rate of 11.1% throughout the forecast period. Additionally, both gross grain production and gross output value of agriculture experience faster growth rates, expected to reach 1.437 million tons and 29.8 billion CNY, respectively, by 2030. Furthermore, the impact on the income of rural residents is noteworthy. It is anticipated that farmers’ disposable income will surpass 30,000 CNY by 2025, with an annual growth rate of 9.8% throughout the forecast period, which exceeds the target value of the local modern agricultural development plan. This development scenario will steadily propel the synergy of multi-objectives for green agricultural transformation.
Figure 8. Trends of Nanping’s agricultural system development under different scenarios: (a) shows agricultural improvement index, (b) reflects per capita disposable income of rural residents, (c) shows the change in gross grain production, and (d) reflects gross output value of agriculture.
Variables of different dimensions within the system, along with the trends of the agricultural green transformation index contribute to a more direct comparison of development trajectories under different scenarios, which provide essential support for strategic choices of green transformation in both Xuzhou and Nanping. In terms of the trend of multidimensional objectives, Xuzhou’s ecological, production and living subsystems improve synergistically, with similar trajectories for the BAU and TGD scenarios (Figure 9a). Considering the comprehensive assessment indicators, Xuzhou’s agricultural sector is experiencing a steady increase in its green transformation index (Figure 9c). The TGD scenario is projected to maintain a leading position and reach 83.76 by 2030 (higher than 80.02 of the EP scenario and 63.54 of the BAU scenario), making it more suitable for the long-term enhancement of the agricultural system. As to Nanping, there is a shift from trade-offs to synergies among the multiple development objectives, and the BAU scenario shows the most significant improvement (Figure 9b). Nanping’s agricultural green transformation index initially declined from 2012 to 2021, followed by fluctuating growth. When comparing trends under different scenarios, the TGD scenario demonstrates a greater effect until 2025. At a later stage, the BAU scenario overtakes with an increasing disparity. By 2030, the three scenarios reach 97.61 (BAU), 39.76 (EP), and 79.15 (TGD) respectively. Therefore, the BAU path is more suitable for Nanping from a long-term perspective.
Figure 9. The trends of multi-objective variables and agricultural green transformation index in Xuzhou and Nanping (2012–2030): (a,b) show the development trajectories of the multidimensional objectives of ecology, production and livelihoods under different scenarios in Xuzhou and Nanping, and (c,d) reflect the trends of the agricultural green transformation index in Xuzhou and Nanping under different scenarios.
The analysis of multi-objective related variables in the model allows us to explore synergies and trade-offs in the green transformation of agriculture, aiming for a balance between ecology, production, and livelihoods. Additionally, variations in resource endowment, economic development level and agricultural function across the pilot zones lead to different outcomes when complex systems are subject to policy interventions. The scenario-based simulation results thus reveal the evolutionary trends of agricultural systems, helping diverse agricultural regions select appropriate strategic paths for green transformation.
Overall, the target pilot zones make a significant progress in synergy of three core objectives in terms of improving the ecological environment, ensuring sustainable productivity, and enhancing farmers’ well-being. National policies have resulted in a significant reduction in the use of chemical fertilizers and pesticides, and the promotion of plastic film recycling technologies in the past decade. The use of agricultural inputs gradually shifts to a more efficient way, which makes an important contribution to environmental sub-objectives. Although the simulation results reveal a trade-off between food production and environmental protection in the system, the overall synergies dominate the trends until 2030. The rapid rise in the agricultural improvement index slightly reduces the growth rate of gross production, but not yet has a significant impact on it due to the increase in water use efficiency and total power of agricultural machinery. The efficiency of water resource use was improved by expanding the water-efficient irrigation area, implementing water-saving irrigation technologies, and enacting relevant regulations. In addition, agricultural productivity has been significantly promoted by advanced technologies and equipment, mitigating reliance on agricultural resources. These results are consistent with recent studies on green productivity improvement in China (Ma et al., 2023; Shah et al., 2023). In brief, input reduction measures serve as catalysts propelling the synergy between ecological health and food productivity in the agricultural system, facilitating progress of green development toward more efficient ways.
According to the simulation results, the production and livelihood objectives are synergistically improved during the study period. The rural labor inputs decreased annually after 2012, while the gross agricultural output value increased steadily, indicating an improvement in China’s agricultural labor productivity. The application of mechanization for expanded production scale filled the gap in labor flow for food production. As mentioned in many studies, labor transfer is a concrete manifestation of agricultural economic growth in the new development stage. It does not have a substantial negative impact on China’s grain production, but rather promotes the structural transformation of agricultural production through intermediary measures (Giller et al., 2021; Lu and Huan, 2022). Furthermore, the financial support policy for agriculture is also an effective measure to promote agricultural economic growth. The pilot zones make great efforts to cultivate rural industries to broaden the source of farmers’ income, such as extending the agricultural industry chain, enhancing the value chain, and safeguarding the supply chain. This encourages farmers to obtain more value-added benefits of agricultural products and improve local farmers’ livelihoods.
In turn, higher incomes for farmers can drive improvements in ecological quality, which make farmers easier to accept advanced concepts, technologies, and measures for green production. As the important stakeholders, farmers have strong motivation to adopt sustainable practices (Piñeiro et al., 2020; Shen et al., 2024). In sum, the synergy of multi-objectives may drive a paradigm shift in agricultural green development.
The choice of strategic pathways should consider the evolutionary trajectories of different scenarios. Due to the high level of agricultural industrialization, mechanization, and production scale in Xuzhou, the trajectory of agricultural green development is on an upward trend, with a synergistic enhancement of multi-objectives in the short term. We can see that policy regulation and technological innovation contribute significantly to the efficiency of agricultural production and high-quality development of local economy, which in turn creates greater potential for agricultural green transformation. Therefore, economically developed pilot zones can strengthen the integrated application of green technologies such as input reduction, waste recycling, and conservation tillage. Furthermore, local governments should continue to explore new industrial models through eco-agriculture such as “rice-fish coculture” and “pig-biogas-fruit” etc.
Compared to Xuzhou, Nanping has distinct ecological endowments, while facing a more convoluted transition process. On the one hand, the agricultural green transformation is urgent to harmonize agricultural production with environmental carrying capacities. In fact, the local government has taken strong measures in recent years to accelerate green development of agriculture. On the other hand, Nanping is constrained by the trade-off between “green” and “development” due to its moderate level of economy. Therefore, maintaining the current intensity of transformation becomes a more appropriate strategic choice for the future. In general, economically less developed regions have a greater need for financial incentives or ecological compensation policies to support the green transformation of agriculture. Moreover, the local government should make an effort to convert the region’s ecological advantages into economic benefits, such as developing agricultural products with local characteristics and cultivating special agricultural brands etc.
In this study, the different pathways of green transformation observed in economically developed and less developed regions provide empirical validation for the environmental Kuznets curve. In line with the development trajectories of many industrialized nations, developed cities have a stronger foundation for investing in green technologies and institutional innovations, thereby promoting synergies between production and ecological sustainability through long-term practices. However, this process often entails irreversible ecological costs. For ecologically significant regions such as Nanping, it is crucial to avoid the “treatment after pollution” model while pursuing economic growth. In this context, China’s spatial-functional heterogeneity presents a unique advantage, as national or interregional ecological compensation mechanisms can mitigate economic constraints in ecologically sensitive areas while safeguarding farmers’ livelihoods without compromising existing ecological resources. By designing compensation frameworks based on regional resource endowments to “trade space for time,” China can facilitate large-scale sustainable transformation.
System dynamics models can be used to evaluate policy interventions in complex systems. In this study, the simulation model serves as a forecasting tool, identifying synergies and trade-offs among system elements, thereby facilitating the choice of future development trajectories. Certainly, it can also be used in other pilot zones to test the impact of strategic options in achieving long-term synergies. This study enhances the application of complex systems in sustainability research by incorporating causality and feedback mechanisms in agricultural systems. It establishes a replicable analytical framework for green development in diverse agricultural regions, enabling the prediction of policy impacts on system performance. Additionally, it provides policymakers with tools to strengthen the effectiveness of development strategies. Nevertheless, this study confronts certain limitations. For instance, the intricate interactions within agricultural systems pose substantial challenges in both qualitative and quantitative phases of modeling. First, despite drawing upon existing researches for model conceptualization, the selection of variables retains a certain subjectivity. Second, the number of green development pilot zones for modeling is insufficient, and the conclusions of this study are mainly based on the target pilot zones, thus further validation of our findings in various areas will be necessary for the future. Third, enhancing the understanding of agricultural systems through government surveys and farmer interviews would increase the simulation accuracy.
The system dynamics model can be considered as a promising tool for the strategic choice of agricultural green transformation. In the practices of the pilot zones, specific natural conditions, advanced technologies, policy-making, local economic levels, and system complexity pose significant challenges in identifying synergies and trade-offs. We developed a system dynamics model to examine relationships of multi-objectives for green development under different scenarios, which has performed very well in predicting pathway trends and strategy choices.
The agricultural system can achieve synergy by improving the ecological environment, ensuring sustainable productivity, and enhancing farmer welfare in the two target pilot zones. Over the study period, the agricultural transition shows a gradual improvement process, but it should be noted that there is a trade-off relationship between food production and environmental protection in the forecast period. Nevertheless, it cannot change the overall synergies of the green transformation in the target pilot zones by 2030.
Different types of pilot zones are expected to present diverse strategic trajectories in the future. Regions with developed economies and advanced technologies possess greater potential for green transformation and are capable of adopting more powerful measures for high-quality development. Meanwhile, less economically developed and ecologically oriented regions should adhere to a balance between environmental protection and economic development, maintain the current policy intensity, and promote agricultural transformation through the support of ecological compensation policies from the central government, other beneficiary regions and the cultivation of eco-friendly industries. These two types of pilot zones are emblematic of China’s agricultural production areas, and their development paths offer valuable insights for future strategies.
The original contributions presented in the study are included in the article/Supplementary material, further inquiries can be directed to the corresponding author.
MP: Conceptualization, Data curation, Formal analysis, Methodology, Visualization, Writing – original draft. GZ: Conceptualization, Funding acquisition, Methodology, Supervision, Validation, Writing – review & editing.
The author(s) declare that financial support was received for the research and/or publication of this article. This research was supported by the China Agricultural University under the “Implementation Plan for the Strategic Research on Building a Strong Agriculture with Chinese Characteristics.”
The authors declare that the research was conducted in the absence of any commercial or financial relationships that could be construed as a potential conflict of interest.
All claims expressed in this article are solely those of the authors and do not necessarily represent those of their affiliated organizations, or those of the publisher, the editors and the reviewers. Any product that may be evaluated in this article, or claim that may be made by its manufacturer, is not guaranteed or endorsed by the publisher.
The Supplementary material for this article can be found online at: https://www.frontiersin.org/articles/10.3389/fsufs.2025.1432501/full#supplementary-material
Ali, M. F., Sulong, S. H., Julius, K., Smith, C., and Aziz, A. A. (2022). Using a participatory system dynamics modelling approach to inform the management of Malaysian rubber production. Agric. Syst. 202:103491. doi: 10.1016/j.agsy.2022.103491
Arshad, F. M., Bala, B., Alias, E., and Abdulla, I. (2015). Modelling boom and bust of cocoa production systems in Malaysia. Ecol. Model. 310, 22–32. doi: 10.1016/j.ecolmodel.2015.03.020
Barbier, E. B. (2020). Is green rural transformation possible in developing countries? World Dev. 131:104955. doi: 10.1016/j.worlddev.2020.104955
Chen, P., and Li, J. (2023). Sustainable agricultural management: how to achieve carbon neutrality in agriculture-evidence from China agricultural sustainable development plan. Sustain. Dev. 32, 2846–2857. doi: 10.1002/sd.2821
Coyle, G. (2000). Qualitative and quantitative modelling in system dynamics: some research questions. Syst. Dyn. Rev. 16, 225–244. doi: 10.1002/1099-1727(200023)16:3<225::AID-SDR195>3.0.CO;2-D
Dardonville, M., Urruty, N., Bockstaller, C., and Therond, O. (2020). Influence of diversity and intensification level on vulnerability, resilience and robustness of agricultural systems. Agric. Syst. 184:102913. doi: 10.1016/j.agsy.2020.102913
Datola, G., Bottero, M., De Angelis, E., and Romagnoli, F. (2022). Operationalising resilience: a methodological framework for assessing urban resilience through system dynamics model. Ecol. Model. 465:109851. doi: 10.1016/j.ecolmodel.2021.109851
Deng, Y., and Zeng, F. (2022). Sustainable path of food security in China under the background of green agricultural development. Sustain. For. 15:2538. doi: 10.3390/su15032538
Ding, W., Chang, N., Zhang, G., Kang, J., Yi, X., Zhang, J., et al. (2023). Soil organic carbon changes in China's croplands: a newly estimation based on DNDC model. Sci. Total Environ. 905:167107. doi: 10.1016/j.scitotenv.2023.167107
Egerer, S., Cotera, R. V., Celliers, L., and Costa, M. M. (2021). A leverage points analysis of a qualitative system dynamics model for climate change adaptation in agriculture. Agric. Syst. 189:103052. doi: 10.1016/j.agsy.2021.103052
Elsawah, S., Pierce, S. A., Hamilton, S. H., Van Delden, H., Haase, D., Elmahdi, A., et al. (2017). An overview of the system dynamics process for integrated modelling of socio-ecological systems: lessons on good modelling practice from five case studies. Environ. Model Softw. 93, 127–145. doi: 10.1016/j.envsoft.2017.03.001
Fader, M., Cranmer, C., and Lawford, R. (2018). Toward an understanding of synergies and trade-offs between water, energy, and food SDG targets. Front. Environ. Sci. 6:410179. doi: 10.3389/fenvs.2018.00112
FAO, IFAD, UNICEF, WFP and WHO (2023). The state of food security and nutrition in the world 2023. Urbanization, agrifood systems transformation and healthy diets across the rural-urban continuum. Rome: FAO.
Forbus, K. D. (1984). Qualitative process theory. Artif. Intell. 24, 85–168. doi: 10.1016/0004-3702(84)90038-9
Forrester, J. W., and Senge, P. M. (1980). Tests for building confidence in system dynamics models. Inst. Manage. Sci. 14, 209–228.
Ghose, B. (2014). Food security and food self-sufficiency in China: from past to 2050. Food Energ. Secur. 3, 86–95. doi: 10.1002/fes3.48
Giller, K. E., Delaune, T., Silva, J. V., Descheemaeker, K., Ven, G. W. J., Schut, A. G. T., et al. (2021). The future of farming: who will produce our food? Food Secur. 13, 1073–1099. doi: 10.1007/s12571-021-01184-6
Guo, C., Bai, Z., Shi, X., Chen, X., Chadwick, D., Strokal, M., et al. (2021). Challenges and strategies for agricultural green development in the Yangtze River basin. J. Integr. Environ. Sci. 18, 37–54. doi: 10.1080/1943815X.2021.1883674
Hansen, A. R., Ingram, J. S., and Midgley, G. (2020). Negotiating food systems resilience. Nat. Food 1:519. doi: 10.1038/s43016-020-00147-y
Hosseinzadeh, M., Samadi Foroushani, M., and Sadraei, R. (2022). Dynamic performance development of entrepreneurial ecosystem in the agricultural sector. Br. Food J. 124, 2361–2395. doi: 10.1108/BFJ-08-2021-0909
IPCC (2023). “Summary for policymakers” in Climate change 2023: Synthesis report. Contribution of working groups I, II and III to the sixth assessment report of the intergovernmental panel on climate change. eds. H. Lee and J. Romero (Geneva: IPCC), 1–34.
Jagustović, R., Papachristos, G., Zougmoré, R. B., Kotir, J. H., Kessler, A., Ouédraogo, M., et al. (2021). Better before worse trajectories in food systems? An investigation of synergies and trade-offs through climate-smart agriculture and system dynamics. Agric. Syst. 190:103131. doi: 10.1016/j.agsy.2021.103131
Kumar, R., Singh, S., Bilga, P. S., Singh, J., Singh, S., Scutaru, M., et al. (2020). Revealing the benefits of entropy weights method for multi-objective optimization in machining operations: a critical review. J. Mater. Res. Technol. 10, 1471–1492. doi: 10.1016/j.jmrt.2020.12.114
Lankoski, J., and Lankoski, L. (2023). Environmental sustainability in agriculture: identification of bottlenecks. Ecol. Econ. 204:107656. doi: 10.1016/j.ecolecon.2022.107656
Li, W., Jiang, R., Wu, H., Xie, J., Zhao, Y., Song, Y., et al. (2023). A system dynamics model of urban rainstorm and flood resilience to achieve the sustainable development goals. Sustain. Cities Soc. 96:104631. doi: 10.1016/j.scs.2023.104631
Lin, G., Palopoli, M., and Dadwal, V. (2020). “From causal loop diagrams to system dynamics models in a data-rich ecosystem” in Leveraging Data Science for Global Health. eds. L. Celi, M. Majumder, P. Ordóñez, J. Osorio, K. Paik, and M. Somai (Cham: Springer).
Liu, J., Li, Y., and Wang, Z. (2023). The potential for carbon reduction in construction waste sorting: a dynamic simulation. Energy 275:127477. doi: 10.1016/j.energy.2023.127477
Liu, Y., Sun, D., Wang, H., Wang, X., Yu, G., and Zhao, X. (2020). An evaluation of China's agricultural green production: 1978-2017. J. Clean. Prod. 243:118483. doi: 10.1016/j.jclepro.2019.118483
Liu, J., Tan, X., Zheng, J., and Wang, Z. (2024). Quantification of carbon potential of construction waste treatment: a case study of Guangzhou, China. J. Green Building 19, 221–244. doi: 10.3992/jgb.19.3.221
Lu, H., and Huan, H. (2022). Does the transfer of agricultural labor reduce China's grain output? A substitution perspective of chemical fertilizer and agricultural machinery. Front. Environ. Sci. 10:961648. doi: 10.3389/fenvs.2022.961648
Ma, W., Liu, T., Li, W., and Yang, H. (2023). The role of agricultural machinery in improving green grain productivity in China: towards trans-regional operation and low-carbon practices. Heliyon 9:e20279. doi: 10.1016/j.heliyon.2023.e20279
Mbow, C. C., Rosenzweig, L. G., Barioni, T. G., Benton, M., Herrero, M., Krishnapillai, E., et al. (2019). “Food security” in Climate change and land: An IPCC special report on climate change, desertification, land degradation, sustainable land management, food security, and greenhouse gas fluxes in terrestrial ecosystems. eds. P. R. Shukla, J. Skea, E. C. Buendia, and V. Masson-Delmotte (Geneva: IPCC).
Ministry of Ecology and Environment of the People’s Republic of China (2023). Annual statistic report on ecology and environment in China 2021. Beijing: Ministry of Ecology and Environment of the People’s Republic of China.
Musvoto, C., Nahman, A., Stafford, W., and Nortje, K. (2018). “Agriculture in a green economy” in Green economy implementation in the agriculture sector. ed. C. Musvoto (Cham: Springer).
Nazir, N., Olabisi, L., and Ahmad, S. (2018). Forest wood consumption and wood shortage in Pakistan: estimation and projection through system dynamics. Pakistan Dev. Rev. 57, 73–98. doi: 10.30541/v57i1pp.73-98
Ostrom, E. (2009). A general framework for analyzing sustainability of social-ecological systems. Science 325, 419–422. doi: 10.1126/science.1172133
Piñeiro, V., Arias, J., Dürr, J., Elverdin, P., Ibáñez, A. M., Kinengyere, A., et al. (2020). A scoping review on incentives for adoption of sustainable agricultural practices and their outcomes. Nature Sust. 3, 809–820. doi: 10.1038/s41893-020-00617-y
Sarfraz, M., Iqbal, K., Wang, Y., Bhutta, M. S., and Jaffri, Z. U. A. (2022). Role of agricultural resource sector in environmental emissions and its explicit relationship with sustainable development: evidence from Agri-food system in China. Res.Policy 80:103191. doi: 10.1016/j.resourpol.2022.103191
Shah, W. U. H., Hao, G., Yasmeen, R., Yan, H., Shen, J., and Lu, Y. (2023). Role of China's agricultural water policy reforms and production technology heterogeneity on agriculture water usage efficiency and total factor productivity change. Agric. Water Manag. 287:108429. doi: 10.1016/j.agwat.2023.108429
Shen, Y., Shi, R., Yao, L., and Zhao, M. (2024). Perceived value, government regulations, and farmers’ agricultural green production technology adoption: evidence from China’s Yellow River Basin. Environ. Manag. 73, 509–531. doi: 10.1007/s00267-023-01893-y
Sterman, J. D. (2000). Business dynamics: Systems thinking and modeling for a complex world. New York, NY: Irwin McGraw-Hill.
Tao, T., Ma, L., Wang, X., Wu, S., Shi, Z., and Cui, X. (2023). Resilience or efficiency? Strategic options for sustainable development of agricultural systems in ecologically fragile areas of China. Sci. Total Environ. 881:163411. doi: 10.1016/j.scitotenv.2023.163411
Ten Broeke, G., and Tobi, H. (2021). Mapping validity and validation in modelling for interdisciplinary research. Qual. Quant. 55, 1613–1630. doi: 10.1007/s11135-020-01073-8
Tripathi, H. G., Kunin, W. E., Smith, H. E., Sallu, S. M., Maurice, S., Machera, S. D., et al. (2022). Climate-smart agriculture and trade-offs with biodiversity and crop yield. Front. Sust. Food Syst. 6:868870. doi: 10.3389/fsufs.2022.868870
Walters, J. P., Archer, D. W., Sassenrath, G. F., Hendrickson, J. R., Hanson, J. D., Halloran, J. M., et al. (2016). Exploring agricultural production systems and their fundamental components with system dynamics modelling. Ecol. Model. 333, 51–65. doi: 10.1016/j.ecolmodel.2016.04.015
Wang, J., Han, X., Liu, W., Ni, C., and Wu, S. (2023). Comprehensive assessment system and spatial difference analysis on development level of green sustainable agriculture based on life cycle and SA-PP model. J. Clean. Prod. 434:139724. doi: 10.1016/j.jclepro.2023.139724
Wang, W., Li, K., Liu, Y., Lian, J., and Hong, S. (2022). A system dynamics model analysis for policy impacts on green agriculture development: a case of the Sichuan Tibetan area. J. Clean. Prod. 371:133562. doi: 10.1016/j.jclepro.2022.133562
Wang, S., Zhu, J., Wang, L., and Zhong, S. (2022). The inhibitory effect of agricultural fiscal expenditure on agricultural green total factor productivity. Sci. Rep. 12, 20933–20918. doi: 10.1038/s41598-022-24225-2
Weiskopf, S. R., Rubenstein, M. A., Crozier, L. G., Gaichas, S., Griffis, R., Halofsky, J. E., et al. (2020). Climate change effects on biodiversity, ecosystems, ecosystem services, and natural resource management in the United States. Sci. Total Environ. 733:137782. doi: 10.1016/j.scitotenv.2020.137782
Xu, S., Zhao, Y., and Qian, J. (2024). Research on status, hotspots and developing trend of agricultural green development in China under the goal of high-quality development. Chinese J. Agric. Res. Reg. Plan. 45, 66–75. doi: 10.7621/cjarrp.1005-9121.20240706
Yu, C., Wenxin, L., Khan, S. U., Yu, C., Jun, Z., Yue, D., et al. (2020). Regional differential decomposition and convergence of rural green development efficiency: evidence from China. Environ. Sci. Pollut. Res. Int. 27, 22364–22379. doi: 10.1007/s11356-020-08805-1
Zhang, Q., Qu, Y., and Zhan, L. (2023). Great transition and new pattern: agriculture and rural area green development and its coordinated relationship with economic growth in China. J. Environ. Manag. 344:118563. doi: 10.1016/j.jenvman.2023.118563
Zhang, R., Yuan, Y., Li, H., and Hu, X. (2022). Improving the framework for analyzing community resilience to understand rural revitalization pathways in China. J. Rural. Stud. 94, 287–294. doi: 10.1016/j.jrurstud.2022.06.012
Zhang, H., Zhang, J., and Song, J. (2022). Analysis of the threshold effect of agricultural industrial agglomeration and industrial structure upgrading on sustainable agricultural development in China. J. Clean. Prod. 341:130818. doi: 10.1016/j.jclepro.2022.130818
Keywords: green transformation, system dynamics, synergy, trade-off, complexity
Citation: Pan M and Zhao G (2025) Strategy choice for agricultural green transformation in China from system dynamics perspective. Front. Sustain. Food Syst. 9:1432501. doi: 10.3389/fsufs.2025.1432501
Received: 14 May 2024; Accepted: 10 March 2025;
Published: 26 March 2025.
Edited by:
Sanzidur Rahman, University of Reading, United KingdomReviewed by:
Pablo Torres-Lima, Metropolitan Autonomous University, MexicoCopyright © 2025 Pan and Zhao. This is an open-access article distributed under the terms of the Creative Commons Attribution License (CC BY). The use, distribution or reproduction in other forums is permitted, provided the original author(s) and the copyright owner(s) are credited and that the original publication in this journal is cited, in accordance with accepted academic practice. No use, distribution or reproduction is permitted which does not comply with these terms.
*Correspondence: Guishen Zhao, emhnc2hAY2F1LmVkdS5jbg==
Disclaimer: All claims expressed in this article are solely those of the authors and do not necessarily represent those of their affiliated organizations, or those of the publisher, the editors and the reviewers. Any product that may be evaluated in this article or claim that may be made by its manufacturer is not guaranteed or endorsed by the publisher.
Research integrity at Frontiers
Learn more about the work of our research integrity team to safeguard the quality of each article we publish.