- 1Agrosystems Research Group, Wageningen University and Research, Wageningen, Netherlands
- 2Plant Production Systems Group, Wageningen University and Research, Wageningen, Netherlands
- 3Biomass Research, Wageningen, Netherlands
- 4Graduate School of Horticulture, Chiba University, Chiba, Japan
Introduction: Kakamega Forest, Kenya's last tropical rainforest, faces threats from escalating demands for firewood, charcoal, and agricultural expansion driven by population growth. Sustainable resource management is critical, with maize stover—a common lignocellulosic crop residue—proposed as a source for biogas and bioslurry production. However, its removal raises concerns about soil fertility and erosion control. This study evaluates the trade-off between maize stover's use for energy generation and its role in soil erosion mitigation in Western Kenya, considering biophysical conditions and households' socio-economic status.
Methods: Socio-economic data were collected from 91 households to assess energy usage. Soil erosion was quantified using the Revised Universal Soil Loss Equation (RUSLE). High-resolution remote sensing, self-organizing maps (SOM), and Hidden Markov Models (HMM) were integrated for annual field condition monitoring. A decision-tree machine learning model identified farm characteristics favorable for maize stover use in biogas production.
Results: Larger households were found to consume more energy per capita, while proximity to forests did not significantly influence firewood or charcoal consumption. Maize yields were significantly associated with land preparation methods (tractor, oxcart, or manual plowing; p < 0.001) and field size (p < 0.05). Remote-sensing data indicated that the distance between homesteads and fields impacted crop growth status. RUSLE analysis revealed that soil erosion was more strongly influenced by landscape features than by soil properties or farming practices.
Discussion: The integration of SOM-HMM and microsatellite data improved field monitoring and data accuracy, providing valuable insights for sustainable agricultural practices in Kakamega. These findings highlight the complex trade-offs between maize stover utilization for energy production and its role in maintaining soil health, emphasizing the need for balanced resource management strategies.
1 Introduction
Western Kenya, home to the Kakamega Forest—the nation's last tropical rainforest covering 238 square kilometers—faces significant environmental challenges due to rapid population growth and urbanization (Obonyo et al., 2023; Mitchell et al., 2009). Sustainable resource utilization is critical to balance conservation efforts and livelihood needs (Reetsch et al., 2020). Maize stover, the residue from maize harvesting, offers a dual benefit: it can serve as a renewable energy source through biogas production and as crop residue mulch to reduce soil erosion and enhance soil fertility. These practices are particularly vital in fragile ecosystems like Kakamega Forest and Mt. Elgon, where traditional fuel demands drive deforestation (Tumwesige et al., 2011; Smith et al., 2015).
Anaerobic digesters using maize stover for biogas production not only provide energy but also generate nutrient-rich effluent that improves soil fertility, reducing the need for chemical fertilizers (Kinyua et al., 2016). However, maize stover collection presents trade-offs. It may mitigate nitrate leaching but increase sediment runoff or greenhouse gas emissions due to additional fertilizer use (Gramig et al., 2013; Mann et al., 2002). Studies suggest that sustainable management practices, such as no-till farming, can minimize these adverse effects (Moebius-Clune et al., 2011, 2008).
In Sub-Saharan Africa (SSA), competing demands for maize stover—primarily as livestock feed—limit its use for soil health management (Hellin et al., 2013; Erenstein et al., 2011). Addressing these challenges requires context-specific strategies incorporating socio-economic and environmental factors (Jaleta et al., 2015; Orskov et al., 2014). Promoting anaerobic digesters, advancing maize stover research, and aligning practices with local farmer needs are essential steps forward.
Kenya has initiated significant efforts to reduce greenhouse gas (GHG) emissions by 30% (Dalla Longa and van der Zwaan, 2017), prioritizing expanded access to renewable energy. The Draft Strategy and Action Plan for Bioenergy and LPG Development in Kenya (2015–2020) highlights advancements in modern bioenergy technologies (Ministry of Energy Pe, 2015) and the sustainable utilization of agricultural crops and residues for biogas and biomass fuel production. Progress in renewable energy adoption is evident, with the number of installed biogas systems increasing from 6,749 in 2012 (Patinvoh and Taherzadeh, 2019) to ~20,000 by 2015 (Ministry of Energy Pe, 2015).
This study investigates maize stover utilization in Western Kenya, examining its dual potential for energy generation and soil erosion mitigation. Using the Revised Universal Soil Loss Equation (RUSLE) model, we estimate soil loss at the farm level while machine learning techniques evaluate the feasibility of maize stover-based biogas production. To further refine these analyses, we integrate high-resolution remote sensing data with hybrid classification methods, offering a comprehensive perspective on year-round farm conditions (Kim et al., 2012). This approach aims to contribute to sustainable resource management and energy solutions.
2 Material and methods
This section outlines the research area (Section 2.1), data collection of socio-economic information (Section 2.2), soil loss estimation using RUSLE (Section 2.3), application of remote sensing (Section 2.4), and machine learning-based estimation of partial energy replacement by maize stover-based methane production (Section 2.5). Statistical methods are described in Section 2.6.
2.1 Study area
The study included 96.7% of farmers from Busia, Bungoma, Kakamega, and Vihiga counties (Western Kenya) and 3.3% from Siaya County (Nyanza Province). The region experiences long and short rainy seasons, with an average maize yield of 1.9 tons/ha (van Ittersum et al., 2016). Fertilization practices include DAP at planting and CAN for top-dressing, with a density of 53,333 plants/ha. Detailed descriptions of soil types, agro-climatic zones, and farm systems are in Jindo et al. (2020). Farmers were supported by Agrics, a Kakamega-based social enterprise owned by ICS, which provides input packages and services, as previously detailed (Duflo et al., 2008).
2.2 Socio-economic information
Socio-economic data were collected from 91 farmers in 2016 using 33-question digital surveys via ODK on Android devices (FAO, 1983). Interviews lasted ~30 min per farmer and captured household status, agronomic practices, and energy use. GPS coordinates of farmer fields and nearby forests were recorded (Kenya Forest Service), and maize yield (reported as 90-kg bags) was converted to dry weight using a 0.9 ratio. Distance to forests was measured using QGIS. Data were validated with local collaborators.
2.3 Soil loss estimation using Revised Universal Soil Loss Equation (RUSLE)
We employed the Revised Universal Soil Loss Equation (RUSLE), a widely used empirical model, to assess soil erosion risks in maize fields under varying stover management practices (Benavidez et al., 2018). RUSLE estimates soil loss (A, t ha−1 year−1) as the product of five factors:
where A is the annual soil loss (t ha−1 year−1), R is the rainfall erosivity (MJ mm t ha−1 h−1 year−1), K is the soil erodibility (t ha−1 MJ mm−1), LS is the length-slope factor, C is the cover management factor, and P is the conservation practice factor (Wischmeier and Smith, 1978). Data sources for RUSLE factors are detailed in Supplementary Table 1. The R factor was derived using WorldClim's precipitation data (http://www.worldclim.org/current). For the K factor, soil properties (texture, organic carbon, bulk density) were extracted from SoilGrids ISRIC (250 m resolution) and calculated using Williams's (1995) approach, commonly applied in models like EPIC and SWAT (Arnold et al., 1998). The LS factor was computed using Moore and Burch's (1986) method with a 30 m resolution DEM from the Shuttle Radar Topography Mission (SRTM). Flow accumulation and slope data were processed in ArcGIS, combining unit stream length (L) and slope (S) values (details in Supplemental material and Figure 1). The CCC factor, based on vegetation cover, was estimated using biannual NDVI data from PlanetScope (Karmage et al., 2017). Finally, the P factor was calculated using slope data, following Kogo et al. (2020).
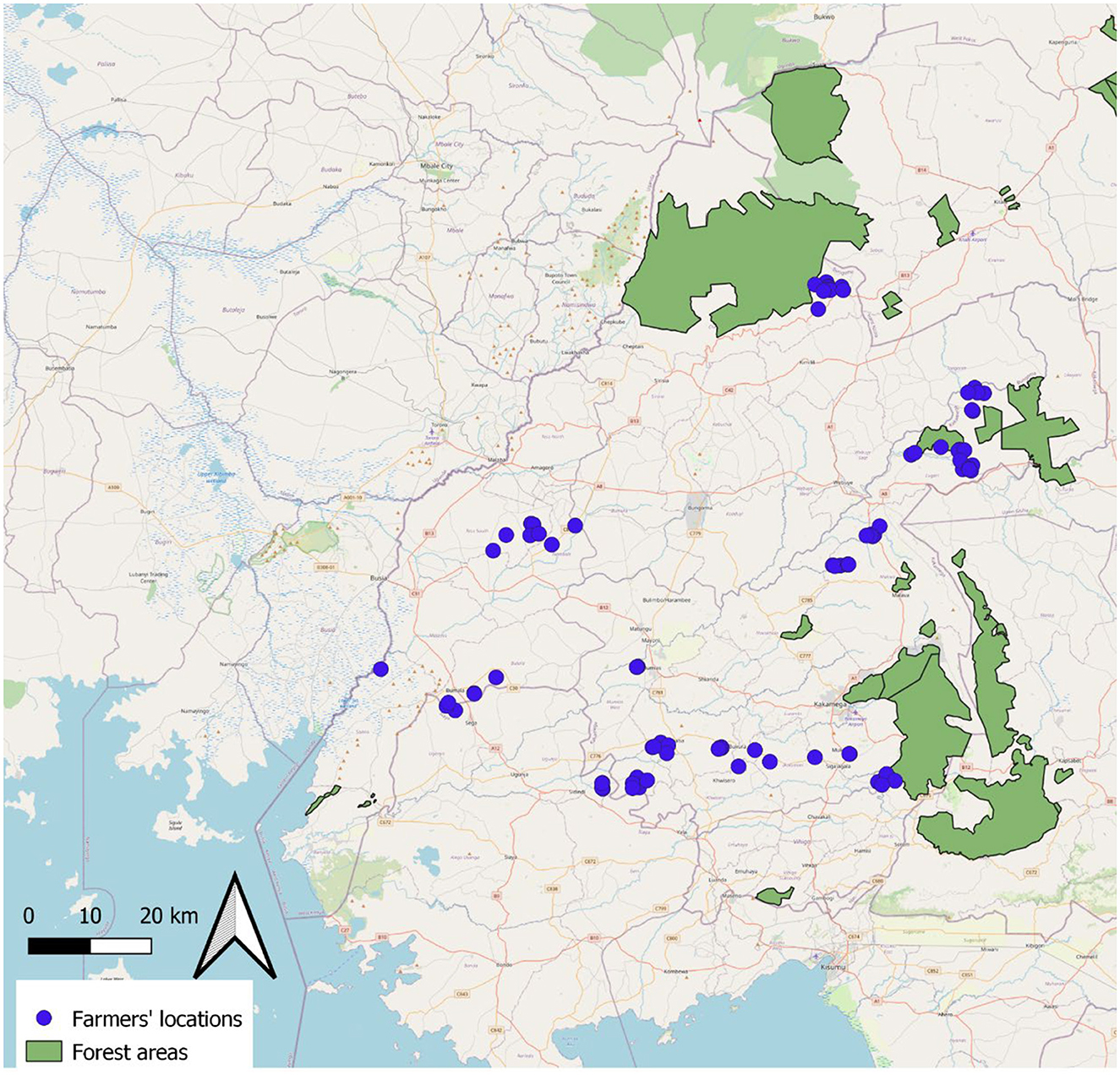
Figure 1. Location of farmers' fields (blue circle) and Kakamega forest areas (green polygons) in western Kenya.
This approach integrates terrain, soil, and vegetation dynamics to assess erosion risks comprehensively.
2.4 Remote-sensing data and the Self-Organizing Maps–Hidden Markov Models (SOM-HMM) approach
The PlanetScope constellation comprises ~130 CubeSats, offering near-daily global coverage at a 3-meter resolution across four spectral bands (red, green, blue, and near-infrared) (Planet, 2020). This high-resolution imagery supports applications such as disaster monitoring (Ganci et al., 2020), detecting fine-scale land cover changes (Halls and Magolan, 2019), precision agriculture (Breunig et al., 2020), and tree-crown phenology analysis (Wu et al., 2021). However, like other satellite data, PlanetScope imagery is prone to artifacts from BRDF effects and cloud contamination, necessitating preprocessing to reduce these errors (Wang et al., 2021; Valman et al., 2024).
Despite selecting cloud-free images, some contamination remained in our dataset (Figure 2). To address this, we applied a time-series modeling approach using SOM-HMM (Sawada, 2010). Self-Organizing Maps (SOM) cluster and visualize high-dimensional data, while Hidden Markov Models (HMM) identify sequences of underlying states, enabling monthly and annual field state classifications based on spectral analysis (Figure 3).
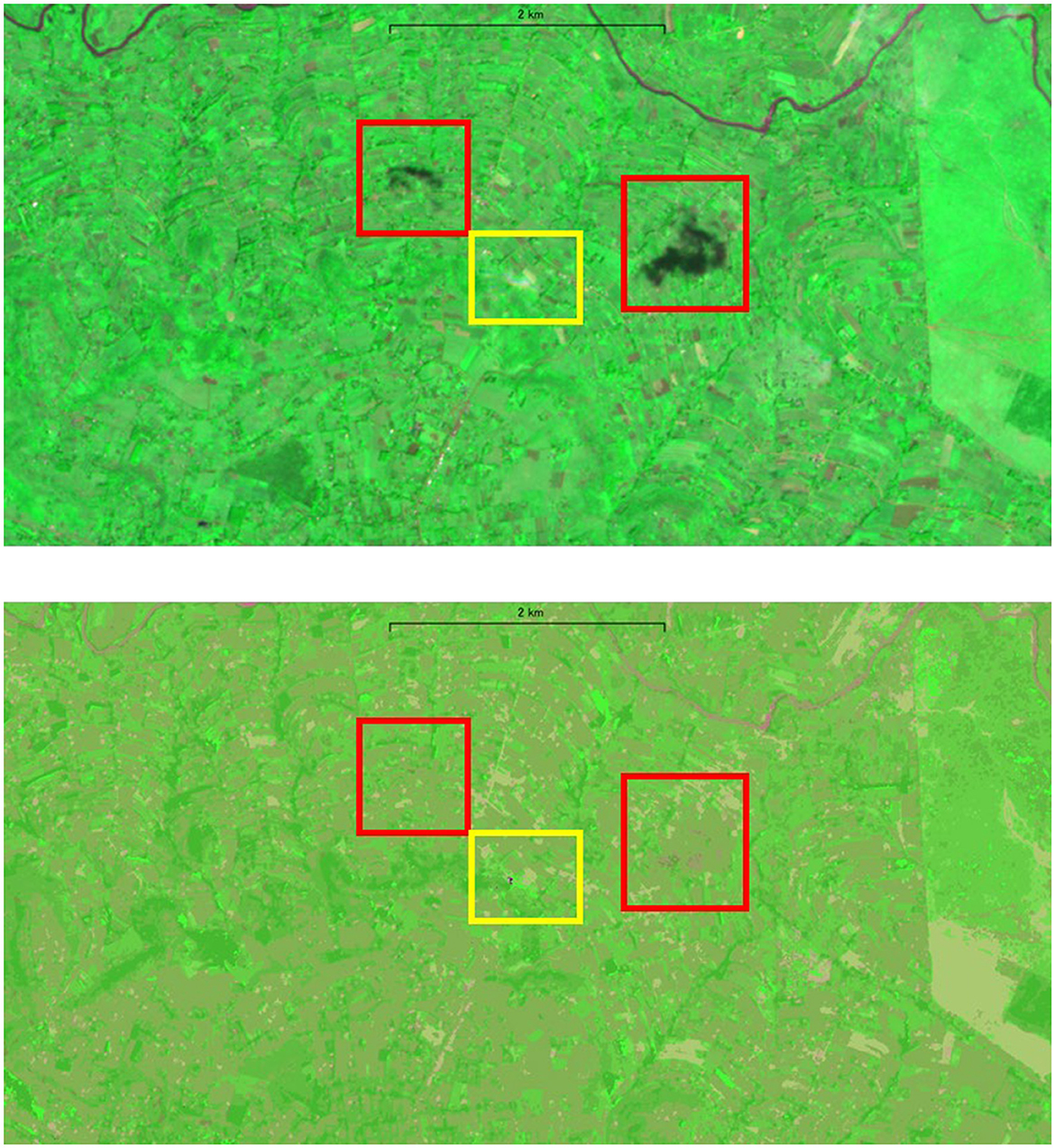
Figure 2. Change in the PlanetScope basemap satellite imagery (monthly, Nov. 2020). (Upper image) Original imagery before processing; (lower image) processed using the Hidden Markov Models (spectral cluster). Red boxes indicate cloud shadows, while the yellow box highlights the clouds.
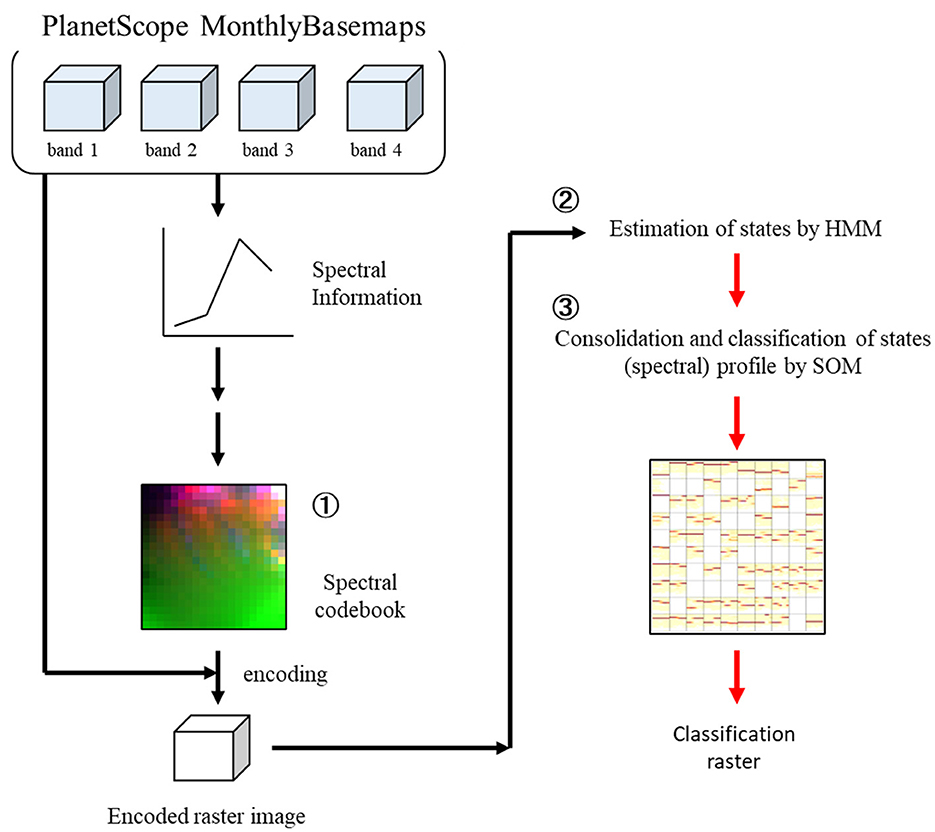
Figure 3. Flowchart illustrating the analysis of PlanetScope high-resolution imagery using self-organizing maps (SOM) and Hidden Markov Models (HMMs) with four spectral bands. The discrete time-series model includes three modules: (1) spectral codebook generation and encoding via SOM, (2) time-series modeling with HMMs, and (3) seosanal change profile classification using SOM.
In addition to optical data, radar remote sensing data from PALSAR (Phased Array type L-band Synthetic Aperture Radar) was used to monitor field and soil conditions. As an active microwave sensor, PALSAR provides cloud-free, day-and-night observations (JAXA). Backscatter coefficients (HV and HH) were retrieved from the “Global PALSAR-2/PALSAR Yearly Mosaic” on Google Earth Engine, and additional metrics, such as HV/HH and HH+VV, were derived (Shimada et al., 2014). The Radar Vegetative Index (RVI) was calculated as per Yadav et al. (2022).
2.5 Learning for estimating partial energy replacement via maize stover-based methane production
Decision tree learning uses a structured model to analyze observations and predict target variables, applied widely in statistics, data mining, and machine learning. Classification trees predict discrete variables, with class labels at the leaves and feature combinations on branches, while regression trees estimate continuous variables. Decision trees aid decision analysis and summarize complex datasets for actionable insights. Random forests extend decision trees by constructing multiple trees during training. For classification, they select the majority class; for regression, they compute the average prediction (Breiman, 2001). The predictor variable in this study is the “replacement of energy consumption by maize stover-based biogas,” derived from three variables: (1) household energy consumption per capita, (2) household size, and (3) maize stover yield (calculated using a harvest index ratio of 0.5 based on other works (Lal, 2004; Karlen et al., 2015; Obrycki et al., 2018; Paul et al., 2019). Methane yield from maize stover is estimated at 288 m3 CH4/ton (Total Solid) based on Langeveld and Peterson (2018), and converted to calorific value (MJ) following Suhartini et al. (2019). Potential methane energy is then compared to household energy needs. The households currently derive energy from some combination of firewood, charcoal and/or LPG gas, so conversion efficiency of methane to electricity is not considered. Farmers experiencing soil erosion (RUSLE > 0) are assumed to retain maize stover in the field, prioritizing soil conservation.
2.6 Statistical analysis
Statistical analyses included t-tests, Mann–Whitney tests, one-way ANOVA, Kruskal–Wallis tests, and correlation analyses. Post-hoc Tukey and Games-Howell tests were applied for group comparisons. Normality was assessed using Anderson-Darling tests, with p ≤ 0.05 considered significant. R packages such as “agricolae” and “randomForest” were utilized (R Core Team, 2020). ChatGPT (OpenAI model GPT-4) was utilized to assist in the correction of our R script, ensuring accuracy and optimization for the analyses conducted in this study. The input prompts and outputs related to this use are provided in the Supplementary material for transparency.
3 Results
3.1 Household dataset (Linear regression and t-test)
Field management practices, such as type of land preparation, significantly impact maize yield (p < 0.001; Figure 4A). The average yields in maize fields prepared by tractor, oxcart, and farmer's hand are 317.0, 251.3, and 152.8 kg/ha respectively (Figure 4A). Maize yield was notably influenced by field size (p < 0.01; Figure 4B) and mechanization level. Farmers with larger fields tend to utilize tractors for land preparation, whereas those with smaller fields cultivate manually. The amount of weeding and manure applications also weakly affects maize yield (0.1 < p < 0.05). The number of hired laborers had no statistically significant effect on yield. Duration of familiy food self-sufficiency is notably influenced by the household size (p < 0.05) and, to some extent, field size (0.1 < p < 0.05). Interestingly, farmers without off-farm income exhibit a superior duration of self-sufficiency (7.9 months compared to 6.6 months, p < 0.05). Energy consumption expressed per individual increases with family size (p < 0.05). While not achieving statistical significance, farmers with off-farm income allocate a greater proportion of maize for sale compared to those without such income (0.1 < p < 0.05). No gender disparities were found in maize yield, land size, or livestock stock units (LSU). Widows and single mothers do not exhibit inferior performance or asset levels in relation to crop yield, land size, or livestock. No significant difference was shown in the relationship between distance to the homestead and maize yield. The mean maize stover value is 540.4 kg per household, with a standard deviation of 430.66 kg per household, indicating considerable diversity among the smallholder farmers in our dataset.
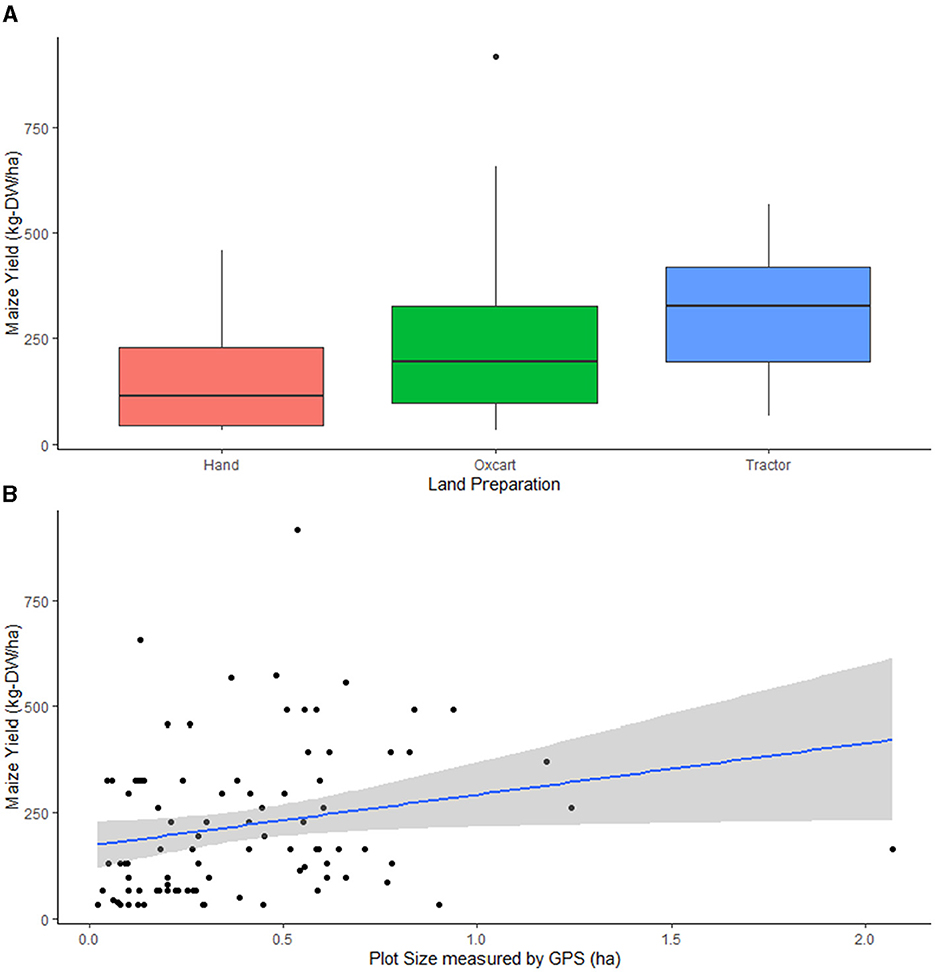
Figure 4. (A) Maize yield (kg dry weight/ha) based on the land preparation methods: Hand (red), Oxcart (green), tractor (blue). (B) Maize yield (kg dry weight/ha) in relation to plot size (ha), measured using GPS. The blue line represents the linear regression line.
3.2 Soil erosion
The mean value of the potential soil erosion rate of the farmers' fields is 0.01 ton/ha per year. The maximum and minimum values of the potential soil erosion rate are 0.19 and 0.003 ton/ha per year, respectively, but 80.3% of farmers show no soil loss according to RUSLE (A = 0) as is explained by their fields' low LS factors. The mean values of factors K, R, LS, C, and P are 0.0267, 6,529.4, 0.028, 0.0184 and 0.1063, respectively.
3.3 Self-Organizing Maps–Hidden Markov Models (SOM-HMM)
A spectral codebook for PlanetScope's multi-band images was created using SOM, and it contains 400 codes. Next, images of the target area were encoded using the obtained spectral codebook. The time series modeling software using SOM-HMM includes a feature for automatically determining the number of HMM states, and the optimal number of states was found to be 20. The mean spectral reflectance spectra of the obtained states corresponded to “water,” “bare soil/artificial features,” and “vegetation” land cover categories for each of the 20 states. States 1 to 6 represent “water bodies,” state 7 corresponds to “bare soil,” and states 8 to 20 correspond to “vegetation.” Additionally, the temporal changes in states at the pixel level were found to represent the phenology of that location. The time series profiles of states for each pixel were classified into 67 categories. As seen in Figure 2, the noise of the cloud and cloud shadow in optical remote-sensing data is removed by this approach.
In our study, there are substantial relationships between the field states variables from SOM-HMM and other variables from the field survey. The distance to the homestead field significantly affected the monthly state of May and September in the SOM-HMM (p < 0.05). The monthly state of March and April was weakly affected to a lesser degree (0.05 < p < 0.1). The distance to the nearest forest significantly affected the annual Cluster ID of SOM-HMM (p < 0.05). The distance to the nearest forest from the farmer's field significantly affected the monthly state of May, June, October and November in the SOM-HMM (p < 0.05), and these months were at the peak of the two cropping seasons (long-rain and short-rain seasons) where plant growth increases strongly. Other months, such as July, August, September and December, which were toward the end of the cropping seasons, have been weakly affected (0.05 < p < 0.1). No significant difference is shown in the relationship between annual cluster-ID and maize yield.
3.4 Synthetic Aperture Radar (SAR) remote sensing data
Linear regression analysis was conducted to find relationship with SAR derived variables and others. HH has significantly relationship with monthly state over the year, especially, dry season such as November (p < 0.05), December (p < 0.05), January (p < 0.05), and February (p < 0.01), respectively. RVI also has significantly relationship with the monthly state of November (p < 0.05) and February (p < 0.05). No significant relationship is seen between maize yield and the all SAR-derived variables. Interestingly, manure amount has significant relationship with HV (p < 0.05) and HH+HV (p < 0.05).
3.5 Correlation matrix
Figure 5 represents the correlation matrix between variables. The order of the variables in the correlation matrix is arranged according to the outcomes of the hierarchical clustering conducted to identify cluster groups across different variables. The correlation analysis shows that elevation positively correlates with distance to the forest, crop yield, land preparation type, field size, and monthly field states from optical remote-sensing data (May, June, Augustus, September, and October). At the same time, it negatively correlates with manure application levels. No clear link was found between the proximity to the nearest forest and energy consumption. Per capita energy consumption was found to have a slightly positive correlation with the number of household members but a negative correlation with self-sufficiency duration. The proportion of the maize that is sold was correlated with plot size. The level of energy consumed per person is positively correlated with household size and negatively with the duration of self-sufficiency. Maize field states derived from remote sensing during the May 2021 to October 2021 period have a significant negative correlation with the distance to the field. Further, annual Cluster ID has a weak negative correlation with distance to the nearest forest, household number and soil loss rate. Maize yield has negatively correlated with the nearest forest, positively with a type of preparation, field size, and elevation. Daily income is highly correlated with the number of hired laborers, the number of household members, and LSU. Variables from SAR-remote sensing (e.g. RVI, HH/HV and HH+HV) have positive correlations with monthly field states measured by optical imagery from October to February. Interestingly, the variables of HH+HV and HV are positively correlated with the amount of manure application.
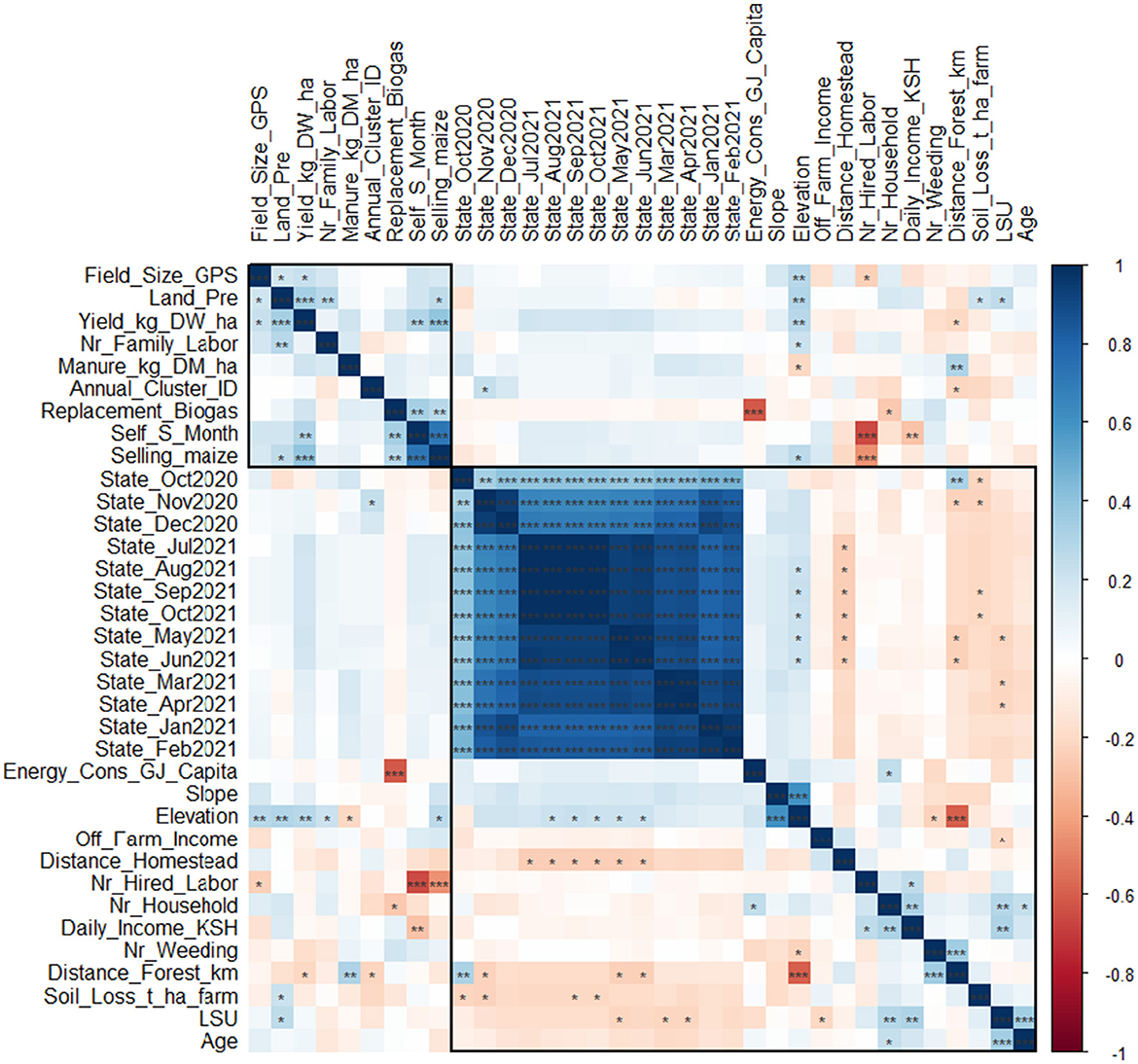
Figure 5. Correlation matrix plots using varialbes from field surveys and remote-sensing data. The correlations are represented with a color scale: red indicates a negative correlation, and blue indicates a positive correlation. Asterisks denotes statistically significant correlations, highlighting stronger relationships.
Based on the result of the clustering method, the number of clusters was chosen to 2, as seen in the Supplementary Figure 2. Ten variables are selected in the small clustering group, including LSU, Age of the household-head, Off-farm income, and Daily income. Other large cluster group covers other variables.
3.6 Decision tree random forest
Figure 6 represents the result of the decision tree, which is one of machine learning methods. The different ratios (0.7, 0.75, and 0.8) between the train data and the test data were tested. And 0.75:0.25 was chosen due to the lowest value of RMSE. The number of splits is two according to the “Complexity Parameter Method” result for the decision tree machine learning method (Venkatasubramaniam et al., 2017). The mean value of the percentage of replacing the energy consumption per household by the maize stover-based biogas generation is 11% of the total energy composition per household (Figure 6). The largest group with 63% of the households in this study (n = 57), could replace 3.4% of the energy consumption by the maize stover biogas. They are categorized as the group which has higher energy consumption than 5.7 GJ/capita and lower maize yield than 279 kg-DW/ha. The second and the third largest groups, including 18% and 13% of the households in our study, respectively, could replace 7.3 and 20% of the energy by the maize stover. Conversely, the maximum benefit is achieved in households with fewer members, primarily relying on LPG gas and producing maize at a rate of 229.46 kg per hectare. This household, representing 1% of our dataset, can make 245% of the replacement. Secondly, farmers who produce higher yields than 197 kg-DW/ha, representing 3% of our dataset, can replace 52% of the energy consumption with maize stover-based biogas.
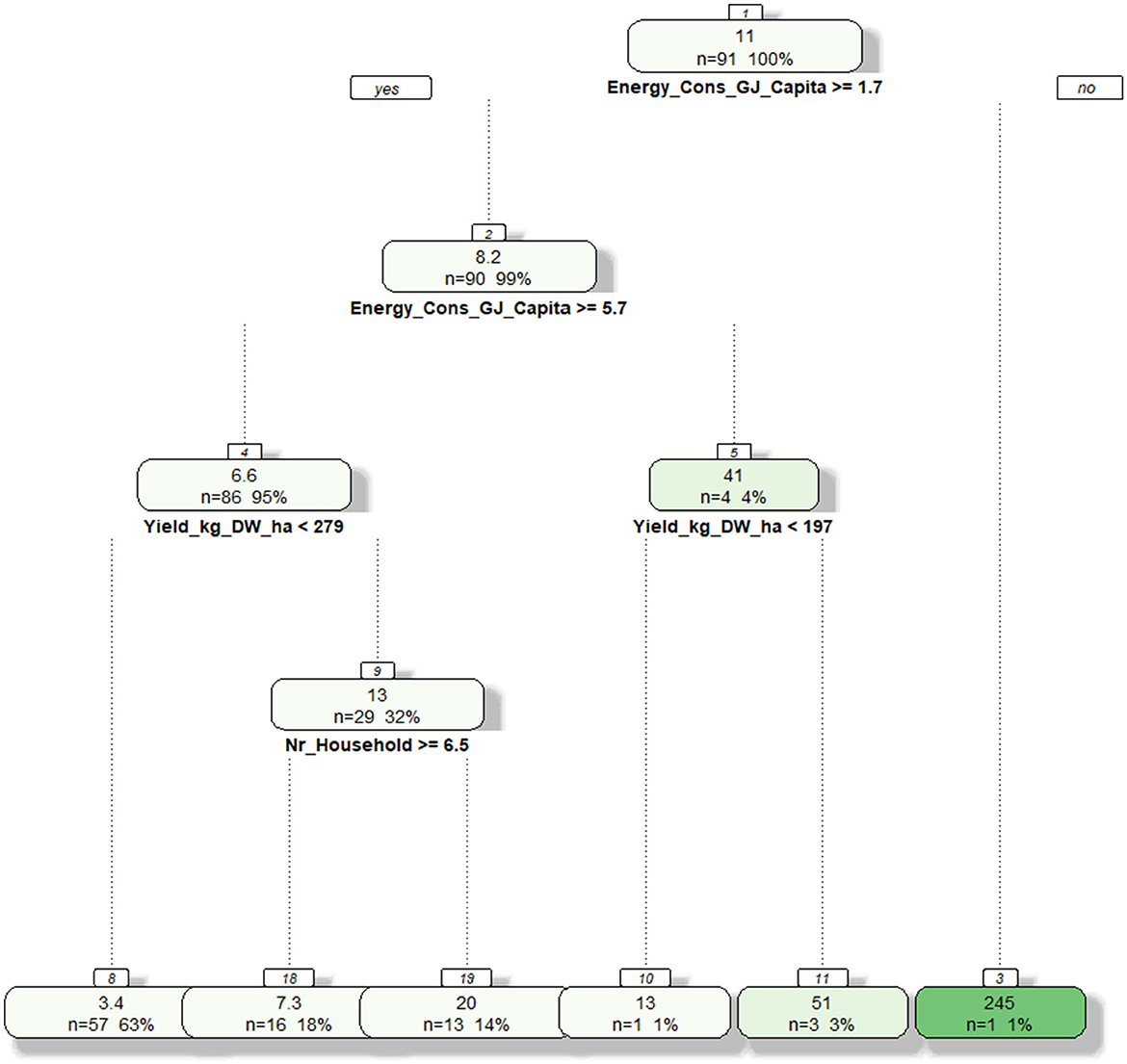
Figure 6. Decission tree chart from machne learning analysis for substituting energy consuption with maize stover-based methane genaration. The key variables in this models are energy consuption per capita (“Energy_Cons_GJ_Capita”), maize yield (“Yield_kg_DW_ha”), and numbe of household members (“Nr_Household”).
Energy consumption associated with maize stover-based biogas production is examined in our study. Key variables for assessing this method include energy consumption per capita, household size, crop yield, field size, and duration of self-sufficiency. Additionally, variables such as “Annual cluster ID” and “Distance to the nearest forest,” while not directly linked to household energy consumption or maize yield, are deemed relatively important (Supplementary Figure 3). The diversity among farming households is evident in our dataset, as seen in the variability of these variables, thereby resulting in different groups for energy substitution with maize stover.
4 Discussion
Biogas production in Sub-Saharan Africa (SSA) has considerable potential due to favorable climate, feedstock availability and a large part of the workforce involved in agriculture (Timothy et al., 2022). Despite this potential, the uptake of biogas systems remains slow and sporadic, primarily due to high installation costs and limited awareness (Rupf et al., 2015). Biogas also comes with barriers to electrification, as many available conversion technologies are expensive, inefficient, and may require costly intermediate steps (Kabeyi and Olanrewaju, 2022). Collaboration among various stakeholders, including government, financial institutions, NGOs, research entities, and entrepreneurs, is deemed essential for successful implementation (Roopnarain et al., 2021). Socio-economic constraints hindering biogas adoption need to be addressed, with proposed solutions emphasizing standardization, quality control, and integrated farming (Mwirigi et al., 2014).
Maize stover, residual biomass left in the field after maize grain harvest, holds promise as a feedstock for biogas production (Mazurkiewicz et al., 2019). Maize stover is more abudant feedstock in smallholder households in Western Kenya than other feedstocks (Torres-Rojas et al., 2011). This carbon rich material, holds cellulose, hemicellulose and lignin with the proportion of 34:19:19 (Langeveld and Peterson, 2018), and contains the range of 0.6%−0.75% of Nitrogen (Karlen et al., 2015; Ludemann et al., 2022; Meya et al., 2023) and 1.2%−1.7% of Potassium (Islam et al., 2018; Meya et al., 2023). Its high methane yield potential, valuable digestate (suited for nutrient recovery), and low hydrogen levels exceed those of alternative feedstocks such as coffee pulp, cotton waste, sugarcane leaves, and banana stalks (Nzila et al., 2015). Converting maize stover to biogas circumvents the conflict between food and biofuel production, and it contributes to sustainable agricultural practices by returning nutrients to the soil through digestate application (Mazurkiewicz et al., 2019). However, in practice, variations in lignin content across maize stover fractions can impact methane yield, which necessitates further research (Wozniak et al., 2021). Also, harvesting corn stover for energy production can lead to increased erosion, reduced soil quality, and impacts on soil organic carbon dynamics (Mann et al., 2002).
In practice, most farmers included in our dataset had an extremely low risk of soil erosion, confirming other soil erosion estimates in Western Kenya (Kogo et al., 2020). The average loss is 0.4 t/ha/y. The study area's most dominant severity class has low erosion rates (< 5 ton/ha/y), which are explained by low LS factors as supported by other studies (Kogo et al., 2020; Schürz et al., 2020). Future studies could address this limitation by incorporating field measurements of erosion rates under varying crop residue and soil management practices, providing empirical evidence to complement the modeling results presented in this study.
Several agro-ecological zones exist in Western Kenya, given its topographic diversity. The positive correlations between elevation and monthly field states during the cropping seasons could reflect this difference (Figure 5). As an example, the fields located at higher elevations close to Mt. Elgon encounter favorable climate and soil conditions for maize growth which allow higher yield levels. On the contrary, fields located in lowland areas require higher manure applications, as reflected in the negative correlation between elevation and manure application.
Apart from the varied landscape and agro-ecological zones, the location of the farmer's field in relation to the farmer's home is important, given that different field management (e.g. fertilizer, manure, weeding, pest management) depending on the distance to the home creates a fertility gradient within the farm (Vanlauwe et al., 2006; Tittonell et al., 2007; Kihara et al., 2015; Assefa et al., 2020). Generally, soils located close to homesteads show higher fertility including soil organic carbon and cation exchange capacity due to organic amendments of crop residues and animal manure compared to those located far from the home (Yakob et al., 2023). Farmers utilize decomposed plant material, kitchen refuse, and household wastes, often leaving them in heaps around their households for decomposition (Nkamleu, 2007). The decomposed material is applied differentially across fields, with homestead fields receiving higher application rates.
Our study found that the SOM-HMM approach, utilizing remote-sensing data, correlates significantly with the distance between homes and homestead fields. Specifically, we observed significantly higher above-biomass status during the cropping season, as reflected by the variables of remote-sensing data, in fields closer to the home. High-resolution remote sensing data has been instrumental in monitoring smallholder farmers' fields globally (Burke and Lobell, 2017). Additionally, innovative approaches such as the insurance index and platform-based advice to farmers have been developed based on vegetative indices like NDVI. Furthermore, radar sensor measurements can provide supplementary monitoring of soil moisture and vegetative water content (Narvekar et al., 2015), as demonstrated in our study, where positive correlations were observed with variables of the SOM-HMM during the dry season (January and February).
The effectiveness of these technologies is further enhanced when combined with freely available soil digital maps, providing insights into various soil properties. While the use of soil digital maps at the regional level is often criticized for lacking precision in capturing spatial heterogeneity at the smallholder farming level (Jemo et al., 2014; Maynard et al., 2023), the HMM-SOM model integrated with microsatellite data serves as a valuable complementary tool for enhancing the monitoring of field status over the years and improving data quality.
It should be noted that in our study, the variables derived from the SOM-HMM model have no significant correlation with farmer self-report yield. The mean value of maize yield self-reported by farmers, 0.22 ton/ha, is much lower than values reported elsewhere for small-scale farms in Kenya in the same cropping season (2016 long-rain; around 0.80–1.0 ton/ha; Marinus et al., 2021; Kätterer et al., 2022). The difference is probably attributed to the different measurement methods between self-report and physical crop-cutting. Earlier studies found the inaccuracy of farmer self-reported yields being a strong constraint for the understanding of farming systems in SSA (Carletto et al., 2015; Burke and Lobell, 2017) while the gaps with field monitoring based on remote sensing approach have been often reported (Burke and Lobell, 2017; Paliwal and Jain, 2020). It is important for future work to identify ways to increase the accuracy of self-reported yield estimates at the plot-level and find an alternative method (e.g. integration between self-reported yield and physical crop-cutting measurements).
In Kenya, unsustainable logging for wood fuel, particularly charcoal production, is a primary cause of deforestation (Watson and Diaz-Chavez, 2011), while also causing land degradation and destruction of water catchment areas (Nunes et al., 2021). Promoting biogas usage can reduce fuelwood and charcoal demand, contributing to deforestation mitigation efforts. By shifting from biomass to biogas as an energy source, the demand for non-renewable biomass decreases, addressing a major driver of deforestation (Piadeh et al., 2024). In addition, it should be noted that biogas systems constitute important mid-term household cooking transition alternatives not only for energy savings but also for atmospheric emission reductions (e.g. PM2.5, NOx, and NMVOCs) from firewood fuel, which is a major social issue in Western Kenya (Carvalho et al., 2019, 2020). Furthermore, biodigestate from biogas production, known as “bioslurry,” enhances soil fertility, particularly over the long term, and helps reduce the need for harmful land-use practices while supporting forest conservation efforts (Piadeh et al., 2024). Other studies (Musse et al., 2020; Yadav et al., 2023) have reported that liquid bioslurry contains 1.5%−2% total nitrogen, 300 mg/kg of available phosphorus, and 715 mg/kg of available potassium. This nutrient management cycle, which includes the application of bioslurry, not only helps maintain soil fertility but also has the potential to reduce dependence on chemical fertilizers, thus benefiting smallholder farmers by lowering input costs.
Replacing traditional energy sources and chemical fertilizers with biogas in Kenya is estimated to save households between $21 and $25 per month (Kimutai et al., 2024). However, the high cost of installing biogas systems continues to pose a significant challenge, particularly in resource-limited settings (Naik et al., 2014). The cost of a small-scale plant in Kenya was estimated at $1,500 due to high construction material costs (Roopnarain et al., 2021). For example, construction materials in Kenya are not only expensive but also difficult to access, as transportation to and from villages adds to the expense. The Kenyan government has facilitated the implementation of ~20,000 biogas units nationwide (Kimutai et al., 2024). Also, it is reported that there exist several company in Kenya that has reduced construction cost up to 20% by modifying design and materials (Clemens et al., 2018). Despite this effort, these systems account for only 11.4%−14.6% of the household energy mix in peri-urban areas, reflecting a limited adoption rate (Kimutai et al., 2024). While these figures show progress, they highlight barriers to wider adoption. Financial, cultural, and technical factors likely limit uptake. Future research should address these to promote biogas use among smallholder farmers.
Our study revealed significant variability in the potential for maize stover-based biogas to substitute household energy consumption (Figure 5). This variation reflects the heterogeneous nature of smallholder farming in Western Kenya, influenced by factors such as family size, energy consumption levels, and maize yields. Farms with fewer household members and higher maize yields are particularly well-positioned for maize stover biogas generation. Notably, one subgroup, which accounted for 14% of our observations, demonstrated the capacity to replace up to 20% of their energy consumption with biogas. However, the economic feasibility of this solution hinges on addressing the high installation costs of anaerobic digestion systems. These findings suggest that while biogas from maize stover offers promising potential for partial energy substitution in certain contexts, its broader adoption will require targeted interventions to make the technology economically viable and accessible for smallholder farmers.
5 Conclusion
While there is significant potential for biogas production from crop residues in Sub-Saharan Africa (SSA), including maize stover, the economic feasibility of adopting anaerobic digestion systems remains a key challenge. The high installation costs of efficient biogas systems may limit their accessibility, particularly for smallholder farmers. Addressing this economic barrier is crucial for realizing the full potential of biogas as a sustainable energy source in the region.
This study highlights the dual challenges of utilizing maize stover for biogas production and managing its removal to mitigate soil erosion risks. By analyzing geography, household characteristics, and field management practices, we propose strategies that support sustainable development, productivity, and resource conservation for smallholder farmers in Western Kenya. Our findings reveal that biogas production from maize stover can partially substitute conventional energy sources for specific household profiles, particularly larger families with higher per capita energy demands. However, this potential remains constrained by the economic cost of biogas systems and the variability in maize stover availability across farms.
Integrating bioslurry, a by-product of biogas production, as an organic fertilizer further underscores the importance of a holistic approach to stover management. Practices such as proper land preparation, controlled manure application, and timely weeding are essential for enhancing maize yields and stover availability. Additionally, advanced monitoring techniques like the integration of SOM-HMM and remote-sensing data enable more precise field-level assessments, improving our understanding of household energy needs, crop yields, and soil erosion risks. Further research is needed to explore the financial and technical constraints on biogas adoption, including the economic viability of bioslurry as a partial substitute for chemical fertilizers. Future studies should also integrate farm-level soil loss assessments and refine yield estimates using physical crop-cutting data combined with remote-sensing technology.
In conclusion, this study provides valuable insights into the trade-offs associated with maize stover use for biogas production in Western Kenya. By addressing both the technical and economic dimensions of biogas adoption, it contributes to the broader discourse on sustainable agriculture and energy production in SSA. These findings emphasize the importance of targeted interventions to reduce the cost of biogas systems, making this technology more accessible to smallholder farmers and enhancing its role in improving livelihoods and promoting environmental sustainability.
Data availability statement
The original contributions presented in the study are included in the article/Supplementary material, further inquiries can be directed to the corresponding author.
Author contributions
KJ: Conceptualization, Data curation, Formal analysis, Funding acquisition, Investigation, Methodology, Project administration, Software, Supervision, Validation, Visualization, Writing – original draft, Writing – review & editing. GG: Data curation, Writing – review & editing. ML: Data curation, Formal analysis, Visualization, Writing – review & editing. AL: Writing – review & editing. YS: Data curation, Formal analysis, Visualization, Writing – review & editing. HL: Writing – review & editing.
Funding
The author(s) declare financial support was received for the research, authorship, and/or publication of this article. The authors thank “MINERVA: Dutch network on MIcrowaves for a New Era of Remote sensing of Vegetation for Agricultural monitoring” by NWO (NSOKNW.2019.001), “Geodata for Agriculture and Water (G4AW) project” financed by space agency of the Dutch government, Organic Fertiliser Valorisation Implementer (OFVI) African Biodigester Component (ABC), and the Agrosystems Research Group of Wageningen University and Research (3710473400).
Acknowledgments
Keiji Jindo thanks the Plant Production System Group for hosting him and providing his postdoc position. The authors also acknowledge the farmers whose activities were the basis and inspiration of this research. ChatGPT (OpenAI model GPT-4) was utilized to assist in the correction of our R script, ensuring accuracy and optimization for the analyses conducted in this study. The input prompts and outputs related to this use are provided in the Supplementary material for transparency.
Conflict of interest
The authors declare that the research was conducted in the absence of any commercial or financial relationships that could be construed as a potential conflict of interest.
Generative AI statement
The author(s) declare Gen AI was used in the creation of this manuscript. ChatGPT (OpenAI model GPT-4) was utilized to assist in the correction of our R script, ensuring accuracy and optimization for the analyses conducted in this study. The input prompts and outputs related to this use are provided in the Supplementary material for transparency.
Publisher's note
All claims expressed in this article are solely those of the authors and do not necessarily represent those of their affiliated organizations, or those of the publisher, the editors and the reviewers. Any product that may be evaluated in this article, or claim that may be made by its manufacturer, is not guaranteed or endorsed by the publisher.
Supplementary material
The Supplementary Material for this article can be found online at: https://www.frontiersin.org/articles/10.3389/fsufs.2025.1409457/full#supplementary-material
References
Arnold, J. G., Srinivasin, R., Muttiah, R. S., and Williams, J. R. (1998). Large srea hydrologic modeling and assessment: Part I. Model development. JAWRA 34, 73–89. doi: 10.1111/j.1752-1688.1998.tb05961.x
Assefa, B. T., Chamberlin, J., Reidsma, P., Silva, J. V., and van Ittersum, M. K. (2020). Unravelling the variability and causes of smallholder maize yield gaps in Ethiopia. Food Secur. 12, 83–103. doi: 10.1007/s12571-019-00981-4
Benavidez, R., Jackson, B., Maxwell, D., and Norton, K. (2018). A review of the (Revised) Universal Soil Loss Equation ((R)USLE): with a view to increasing its global applicability and improving soil loss estimates. Hydrol. Earth Syst. Sci. 22, 6059–6086. doi: 10.5194/hess-22-6059-2018
Breunig, F. M., Galvao, L. S., Dalagnol, R., Dauve, C. E., Parraga, A., Santi, A. L., et al. (2020). Delineation of management zones in agricultural fields using cover crop biomass estimates from PlanetScope data. Int. J. Appl. Earth Obs. Geoinf. 85:102004. doi: 10.1016/j.jag.2019.102004
Burke, M., and Lobell, D. B. (2017). Satellite-based assessment of yield variation and its determinants in smallholder African systems. Proc. Nat. Acad. Sci. 114, 2189–2194. doi: 10.1073/pnas.1616919114
Carletto, C., Gourlay, S., and Winters, P. (2015). From guesstimates to GPStimates: land area measurement and implications for agricultural analysis. J. Afr. Econ. 24, 593–628. doi: 10.1093/jae/ejv011
Carvalho, R. L., Lindgren, R., García-López, N., Nyambane, A., Nyberg, G., Diaz-Chavez, R., et al. (2019). Household air pollution mitigation with integrated biomass/cookstove strategies in Western Kenya. Energy Policy, 131, 168–186. doi: 10.1016/j.enpol.2019.04.026
Carvalho, R. L., Yadav, P., García-López, N., Lindgren, R., Nyberg, G., Diaz-Chavez, R., et al. (2020). Environmental sustainability of bioenergy strategies in Western Kenya to address household air pollution. Energies 13:719. doi: 10.3390/en13030719
Clemens, H., Bailis, R., Nyambane, A., and Ndung'u, V. (2018). Africa biogas partnership program: a review of clean cooking implementation through market development in East Africa. Energy Sustain. Dev. 46, 23–31. doi: 10.1016/j.esd.2018.05.012
Dalla Longa, F., and van der Zwaan, B. (2017). Do Kenya's climate change mitigation ambitions necessitate large-scale renewable energy deployment and dedicated low-carbon energy policy? Renew. Energy 113, 1559–1568. doi: 10.1016/j.renene.2017.06.026
Duflo, E., Kremer, M., and Robinson, J. (2008). How high are rates of return to fertilizer? Evidence from field experiments in Kenya. Am. Econ. Rev. 98, 482–488. doi: 10.1257/aer.98.2.482
Erenstein, O., Samaddar, A., Teufel, N., and Blummel, M. (2011). The paradox of limitedmaize stover use in India's smallholder crop–livestock systems. Exp. Agric. 47, 677–704. doi: 10.1017/S0014479711000433
FAO (1983). Wood Fuel Surveys. Available at: https://www.fao.org/4/q1085e/q1085e00.htm#Contents
Ganci, G., Cappello, A., Bilotta, G., and Del Negro, C. (2020). How the variety of satellite remote sensing data over volcanoes can assist hazard monitoring efforts: the 2011 eruption of Nabro volcano. Remote Sens. Environ. 236:111426. doi: 10.1016/j.rse.2019.111426
Gramig, B. M., Reeling, C. J., Cibin, R., and Chaubey, I. (2013). Environmental and economic trade-offs in a watershed when using corn stover for bioenergy. Environ. Sci. Technol. 47, 1784–1791. doi: 10.1021/es303459h
Halls, J. N., and Magolan, J. L. (2019). A methodology to assess land use development flooding, and wetland change as indicators of coastal vulnerability. Remote Sens. 11:2260. doi: 10.3390/rs11192260
Hellin, J. J., Erenstein, O., Beuchelt, T. D., Camacho Villa, T. C., and Flores, D. (2013). Maize stover use and sustainable crop production in mixedcrop–livestock systems in Mexico. Feild Crops Res. 153, 12–21. doi: 10.1016/j.fcr.2013.05.014
Islam, S., Timsina, J., Salim, M., Majumdar, K., and Gathala, M. K. (2018). Potassium supplying capacity of diverse soils and K-use efficiency of maize in South Asia. Agronomy 8:121. doi: 10.3390/agronomy8070121
Jaleta, M., Kassie, M., and Erenstein, O. (2015). Determinants of maize stover utilization as feed, fuel and soil amendment in mixed crop-livestock systems, Ethiopia. Agric. Syst. 134, 17–23. doi: 10.1016/j.agsy.2014.08.010
Jemo, M., Jayeoba, O. J., Alabi, T., and Montes, A. L. (2014). Geostatistical mapping of soil fertility constraints for yam based cropping systems of North-central and Southeast Nigeria. Geoderma Reg. 2–3, 102–109. doi: 10.1016/j.geodrs.2014.10.001
Jindo, K., Schut, A. G. T., and Langeveld, J. W. A. (2020). Sustainable intensification in Western Kenya: who will benefit? Agric. Syst. 182:102831. doi: 10.1016/j.agsy.2020.102831
Kabeyi, M. J. B., and Olanrewaju, O. A. (2022). Sustainable energy transition for renewable and low carbon grid electricity generation and supply. Front. Energy Res. 9:743114. doi: 10.3389/fenrg.2021.743114
Karlen, D. L., Kovar, J. L., and Birrell, S. J. (2015). Corn stover nutrient removal estimates for Central Iowa, USA. Sustainability 7, 8621–8634. doi: 10.3390/su7078621
Karmage, F., Zhang, C., Liu, T., Maganda, A., and Isabwe, A. (2017). Soil erosion risk assessment in Uganda. Forests 8:52. doi: 10.3390/f8020052
Kätterer, T., Roobroeck, D., Kimutai, G., Karltun, E., Nyberg, G., Sundberg, C., et al. (2022). Maize grain yield responses to realistic biochar application rates on smallholder farms in Kenya. Agron. Sustain. Dev. 42:63. doi: 10.1007/s13593-022-00793-5
Kihara, J., Tamene, L. D., Massawe, P., and Bekunda, M. (2015). Agronomic survey to assess crop yield, controlling factors and management implications: a case-study of Babati in northern Tanzania. Nutr. Cycl. Agroecosyst. 102, 5–16. doi: 10.1007/s10705-014-9648-3
Kim, Y., Jackson, T., Bindlish, R., Lee, H., and Hong, S. (2012). Radar vegetation index for estimating the vegetation water content of rice and soybean. IEEE Geosci. Remote Sens. Lett. 9, 564–568. doi: 10.1109/LGRS.2011.2174772
Kimutai, S. K., Kimutai, I. K., and Manirambona, E. (2024). Impact of biogas adoption on household energy use and livelihood improvement in Kenya: an overview on a roadmap toward sustainability. Int. J. Energy Sector Manag. doi: 10.1108/IJESM-07-2024-0053
Kinyua, M. N., Rowse, L. E., and Ergas, S. J. (2016). Review of small-scale tubular anaerobic digesters treating livestock waste in the developing world. Renew. Sustain. Energy Rev. 58, 896–910. doi: 10.1016/j.rser.2015.12.324
Kogo, B. K., Kumar, L., and Koech, R. (2020). Impact of land use/cover changes on soil erosion in western kenya. Sustainability 12, 1–17. doi: 10.3390/su12229740
Langeveld, J. W. A., and Peterson, E. C. (2018). “Feedstocks for biogas production: biogas and electricity generation potentials,” in Biogas. Biofuel and Biorefinery Technologies, Vol. 6, eds. M. Tabatabaei, and H. Ghanavati (Cham: Springer). doi: 10.1007/978-3-319-77335-3_2
Ludemann, C. I., Hijbeek, R., van Loon, M. P., Murrell, S., Dobermann, A., van Ittersum, M. K., et al. (2022). Estimating maize harvest index and nitrogen concentrations in grain and residue using globally available data. Field Crops Res. 2:108578. doi: 10.1016/j.fcr.2022.108578
Mann, L., Tolbert, V., and Cushman, J. (2002). Potential environmental effects of corn (Zea mays L.) stover removal with emphasis on soil organic matter and erosion. Agric. Ecosyst. Environ. 89, 149–166. doi: 10.1016/S0167-8809(01)00166-9
Marinus, W., Descheemaeker, K. K. E., van de Ven, G. W. J., Waswa, W., Mukalama, J., Vanlauwe, B., et al. (2021). “That is my farm” – an integrated co-learning approach for whole-farm sustainable intensification in smallholder farming. Agric. Syst. 188:103041. doi: 10.1016/j.agsy.2020.103041
Maynard, J. J., Yeboah, E., Owusu, S., Buenemann, M., Neff, J. C., Herrick, J. E., et al. (2023). Accuracy of regional-to-global soil maps for on-farm decision-making: are soil maps “good enough”? Soil 9, 277–300. doi: 10.5194/soil-9-277-2023
Mazurkiewicz, J., Marczuk, A., Pochwatka, P., and Kujawa, S. (2019). Maize straw as a valuable energetic material for biogas plant feeding. Materials 12:3848. doi: 10.3390/ma12233848
Meya, A. I., Swennen, R., Ndakidemi, P. A., Mtei, K. M., and Merckx, R. (2023). Maize stover transfers from maize fields to banana-based agroforestry homegardens and the corresponding nutrient flows in central-northern Tanzania. Front. For. Glob. Change 6:1131328. doi: 10.3389/ffgc.2023.1131328
Ministry of Energy and Pe (2015). Ministry of Energy and Petroleum Draft Strategy and Action Plan for Bioenergy and LPG Development in Kenya. Kenya.
Mitchell, N., Schaab, G., and Wägele, J. W. (2009). Kakamega forest ecosystem: an introduction to the natural history and the human context. Karlsruher Geowiss. Schriften (A) 17, 1–58.
Moebius-Clune, B. N., van Es, H. M., Idowu, O. J., Schindelbeck, R. R., Kimetu, J. M., Ngoze, S., et al. (2011). Long-term soil quality degradation along a cultivation chronosequence in Western Kenya. Agric. Ecosyst. Environ. 141, 86–99. doi: 10.1016/j.agee.2011.02.018
Moebius-Clune, B. N., Van Es, H. M., Idowu, O. J., Schindelbeck, R. R., Moebius-Clune, D. J., Wolfe, D. W., et al. (2008). Long-term effects of harvesting maize stover and tillage on soil quality, Soil Sci. Soc. Am. J. 72, 4, 960–969. doi: 10.2136/sssaj2007.0248
Moore, I. D., and Burch, G. J. (1986). Modeling erosion and deposition. Topographic effects. Trans. Am. Soc. Agric. Eng. 29, 1624–1630. doi: 10.13031/2013.30363
Musse, Z. A., Yoseph Samago, T., and Beshir, H. M. (2020). Effect of liquid bio-slurry and nitrogen rates on soil physico-chemical properties and quality of green bean (Phaseolus vulgaris L.) at Hawassa Southern Ethiopia. J. Plant Interact. 15, 207–212. doi: 10.1080/17429145.2020.1781270
Mwirigi, J., Balana, B. B., Mugisha, J., Walekhwa, P., Melamu, R., Nakami, S., et al. (2014). Socio-economic hurdles to widespread adoption of small-scale biogas digesters in Sub-Saharan Africa: a review. Biomass Bioenergy 70, 17–25. doi: 10.1016/j.biombioe.2014.02.018
Naik, L., Gebreegziabher, Z., Tumwesige, V., Balana, B. B., Mwirigi, J., Austin, G., et al. (2014). Factors determining the stability and productivity of small scale anaerobic digesters. Biomass Bioenergy 70, 51–57. doi: 10.1016/j.biombioe.2014.01.055
Narvekar, P. S., Entekhabi, D., Kim, S. B., and Njoku, E. G. (2015). Soil moisture retrieval using L-band radar observations. IEEE Trans. Geosci. Remote Sens. 53, 3492–3506. doi: 10.1109/TGRS.2014.2377714
Nkamleu, G. B. (2007). “Modeling farmers' decisions on integrated soil nutrient management in sub-Saharan Africa: a multinomial logit analysis in Cameroon,” in Advances in Integrated Soil Fertility Management in sub-Saharan Africa: Challenges and Opportunities, eds. A. Bationo, B. Waswa, J. Kihara, and J. Kimetu (Dordrecht: Springer). doi: 10.1007/978-1-4020-5760-1_85
Nunes, M. R., De, M., McDaniel, M. D., Kovar, J. L., Birrell, S., Karlen, D. L., et al. (2021). Science-based maize stover removal can be sustainable. Agron. J. 113, 3178–3192. doi: 10.1002/agj2.20724
Nzila, C., Njuguna, D., Madara, D., Githaiga, J., Muasya, R., Muumbo, A., et al. (2015). Characterization of agro-residues for biogas production and nutrient recovery in Kenya. J. Emerg. Trends Eng. Appl. Sci. 6, 327–334.
Obonyo, O. A., Agevi, H., and Tsingalia, M. H. (2023). Above-ground carbon stocks and its functional relationship with tree species diversity: the case of Kakamega and North Nandi Forests, Kenya. Sci. Rep. 13:20921. doi: 10.1038/s41598-023-47871-6
Obrycki, J. F., Karlen, D. L., Cambardella, C. A., Kovar, J. L., and Birrell, S. J. (2018). Corn stover harvest, tillage, and cover crop effects on soil health indicators. Soil Sci. Soc. Am. J. 82, 910–918. doi: 10.2136/sssaj2017.12.0415
Orskov, E. R., Yongabi Anchang, K., Subedi, M., and Smith, J. (2014). Overview of holistic application of biogas for small scale farmers in Sub-Saharan Africa. Biomass Bioenergy 70, 4–16. doi: 10.1016/j.biombioe.2014.02.028
Paliwal, A., and Jain, M. (2020). The accuracy of self-reported crop yield estimates and their ability to train remote sensing algorithms. Front. Sustain. Food Syst. 4:25. doi: 10.3389/fsufs.2020.00025
Patinvoh, R. J., and Taherzadeh, M. J. (2019). Challenges of biogas implementation in developing countries. Curr. Opin. Environ. Sci. Health 12, 30–37. doi: 10.1016/j.coesh.2019.09.006
Paul, S., Dutta, A., Thimmanagari, M., and Defersha, F. (2019). Techno-economic assessment of corn stover for hybrid bioenergy production: a sustainable approach. Case Stud. Thermal Eng. 13:100408. doi: 10.1016/j.csite.2019.100408
Piadeh, F., Offie, I., Behzadian, K., Rizzuto, J. P., Bywater, A., Córdoba-Pachón, J. R., et al. (2024). A critical review for the impact of anaerobic digestion on the sustainable development goals. J. Environ. Manage. 349:119458. doi: 10.1016/j.jenvman.2023.119458
Planet (2020). Planet Imagery Product Specification. Available at: https://developers.planet.com/docs/apis/data/ (accessed December 20, 2023).
R Core Team (2020). R: A Language and Environment for Statistical Computing. Vienna: R Foundation for Statistical Computing. Available at: http://www.R-project.org/ (accessed January 22, 2023).
Reetsch, A., Kimaro, D., Feger, K. H., and Schwärzel, K. (2020). “Traditional and adapted composting practices applied in smallholder banana-coffee-based farming systems: case studies from Kagera and Morogoro Regions, Tanzania,” in Organic Waste Composting through Nexus Thinking, eds. H. Hettiarachchi, S. Caucci, and K. Schwärzel (Cham: Springer). doi: 10.1007/978-3-030-36283-6_8
Roopnarain, A., Rama, H., Ndaba, B., Bello-Akinosho, M., Bamuza-Pemu, E., Adeleke, R., et al. (2021). Unravelling the anaerobic digestion ‘black box’: biotechnological approaches for process optimization. Renew. Sustain. Energy Rev. 152:111717. doi: 10.1016/j.rser.2021.111717
Rupf, G. V., Bahri, P. A., de Boer, K., and McHenry, M. P. (2015) Barriers opportunities of biogas dissemination in Sub-Saharan Africa lessons learned from Rwanda, Tanzania, China, India, Nepal. Renew. Sustain. Energy Rev. 52, 468–476. doi: 10.1016/j.rser.2015.07.107
Sawada, Y. (2010). Development of a discrete time-series model for generating snow products. J. Jpn. Soc. Photogramm. Remote Sens. 48, 15–31. doi: 10.4287/jsprs.48.15
Schürz, C., Mehdi, B., Kiesel, J., Schulz, K., and Herrnegger, M. (2020). A systematic assessment of uncertainties in large-scale soil loss estimation from different representations of USLE input factors-a case study for Kenya and Uganda. Hydrol. Earth Syst. Sci. 24, 4463–4489. doi: 10.5194/hess-24-4463-2020
Shimada, M., Itoh, T., Motooka, T., Watanabe, M., Tomohiro, S., Thapa, R., et al. (2014). New global forest/non-forest maps from ALOS PALSAR data (2007-2010). Remote Sens. Environ. 155, 13–31. doi: 10.1016/j.rse.2014.04.014
Smith, J. U., Fischer, A., Hallett, P. D., Homans, H. Y., Smith, P., Abdul-Salam, Y., et al. (2015). Sustainable use of organic resources for bioenergy, food and water provision in rural Sub-Saharan Africa. Renew. Sustain. Energy Rev. 50, 903–917. doi: 10.1016/j.rser.2015.04.071
Suhartini, S., Lestari, Y. P., and Nurika, I. (2019). Estimation of methane and electricity potential from canteen food waste. IOP Conf. Ser.: Earth Environ. Sci. 230:012075. doi: 10.1088/1755-1315/230/1/012075
Timothy, N., Joseph, G., Nellie, O., and Emily, K. (2022). Sustainable biomass energy production and utilization in sub-Saharan Africa: a case study of Kenya. J. Horticult. Forest. 14, 56–67. doi: 10.5897/JHF2022.0689
Tittonell, P., Vanlauwe, B., de Ridder, N., and Giller, K. (2007). Heterogeneity of crop productivity and resource use efficiency within smallholder Kenyan farms: soil fertility gradients or management intensity gradients. Agric. Syst. 94, 376–390. doi: 10.1016/j.agsy.2006.10.012
Torres-Rojas, D., Lehmann, J., Hobbs, P. R., Joseph, S., and Neufeldt, H. (2011). Biomass availability, energy consumption and biochar production in rural households of Western Kenya. Biomass Bioenergy 35, 3537–3546. doi: 10.1016/j.biombioe.2011.05.002
Tumwesige, V., Lisa, M.A., Austin, G, Balana, B.B., Bechtel, K., Casson, E., et al. (2011). Small-scale biogas digester for sustainable energy production in Sub-Saharan Africa.
Valman, S. J., Boyd, D. S., Carbonneau, P. E., Johnson, M. F., and Dugdale, S. J. (2024). (2024). An AI approach to operationalise global daily PlanetScope satellite imagery for river water masking. Remote Sens. Environ. 301:113932. doi: 10.1016/j.rse.2023.113932
van Ittersum, M. K., van Bussel, L. G. J., Wolf, J., Grassini, P., van Wart, J., Guilpart, N., et al. (2016). Can sub-Saharan Africa feed itself? Proc. Natl. Acad. Sci. U.S.A. 113, 14964–14969. doi: 10.1073/pnas.1610359113
Vanlauwe, B., Tittonell, P., and Mukalama, J. (2006). Within-farm soil fertility gradients affect response of maize to fertilizer application in western Kenya. Nutr. Cycl. Agroecosyst. 76, 171–182. doi: 10.1007/s10705-005-8314-1
Venkatasubramaniam, A., Wolfson, J., Mitchell, N., Barnes, T., JaKa, M., and French, S. (2017). Decision trees in epidemiological research. Emerg. Themes Epidemiol. 14:11. doi: 10.1186/s12982-017-0064-4
Wang, J., Yang, D., Chen, S., Zhu, X., Wu, S., Bogonovich, M., et al. (2021). Automatic cloud and cloud shadow detection in tropical areas for PlanetScope satellite images. Remote Sens. Environ. 264:112604. doi: 10.1016/j.rse.2021.112604
Watson, H. S., and Diaz-Chavez, R. A. (2011). An assessment of the potential of drylands in eight sub-Saharan African countries to produce bioenergy feedstocks. Interface Focus 1, 263–270. doi: 10.1098/rsfs.2010.0022
Williams, J. R. (1995). “The EPIC model,” in Computer Models of Watershed Hydrology, Chapter 25, ed. V. P. Singh (Highlands Ranch, CO: Water Resources Publications), 909–1000.
Wischmeier, W. H., and Smith, D. D. (1978). Predicting Rainfall Erosion Losses: A Guide to Conservation Planning. Washington, DC: Department of Agriculture, Science and Education Administration.
Wozniak, M., Ratajczak, I., Wojcieszak, D., Waśkiewicz, A., Szentner, K., Przybył, J., et al. (2021). Chemical and structural characterization of maize stover fractions in aspect of its possible applications. Materials 14:1527. doi: 10.3390/ma14061527
Wu, S., Wang, J., Yan, Z., Song, G., Chen, Y., Ma, Q., et al. (2021). Monitoring tree-crown scale autumn leaf phenology in a temperate forest with an integration of PlanetScope and drone remote sensing observations. ISPRS J. Photogramm. Remote Sens. 171, 36–48. doi: 10.1016/j.isprsjprs.2020.10.017
Yadav, R., Sudhishri, S., Khanna, M., Lal, K., Dass, A., Kushwaha, H. L., et al. (2023). Temporal characterization of biogas slurry: a pre-requisite to sustainable nutrigation in crop production. Front. Sustain. Food Syst. 7:1234472. doi: 10.3389/fsufs.2023.1234472
Yadav, V. P., Prasad, R., Bala, R., Srivastava, P. K., and Vanama, V. S. K. (2022). Appraisal of dual polarimetric radar vegetation index in first order microwave scattering algorithm using sentinel – 1A (C - band) and ALOS - 2 (L - band) SAR data. Geocarto Int. 37, 6232–6250. doi: 10.1080/10106049.2021.1933209
Yakob, G., Habte, M., Smith, J. U., Hallett, P. D., Phimister, E., Rivington, M., et al. (2023). Changes in soil properties with long-term organic inputs due to distance from homestead and farm characteristics in southern Ethiopian farmlands. Geoderma Reg. 35:e00710. doi: 10.1016/j.geodrs.2023.e00710
Keywords: bioslurry, crop residues, deforestation, energy, remote-sensing, smallholder farmers
Citation: Jindo K, Ghaffari G, Lamichhane M, Lazarus A, Sawada Y and Langeveld H (2025) Assessment of trade-off balance of maize stover use for bioenergy and soil erosion mitigation in Western Kenya. Front. Sustain. Food Syst. 9:1409457. doi: 10.3389/fsufs.2025.1409457
Received: 30 March 2024; Accepted: 13 January 2025;
Published: 07 February 2025.
Edited by:
Maria Pilar Bernal, Spanish National Research Council (CSIC), SpainReviewed by:
Marco E. Mng'ong'o, Mbeya University of Science and Technology, TanzaniaEric C. Peterson, INRS Eau Terre Environnement, Canada
Copyright © 2025 Jindo, Ghaffari, Lamichhane, Lazarus, Sawada and Langeveld. This is an open-access article distributed under the terms of the Creative Commons Attribution License (CC BY). The use, distribution or reproduction in other forums is permitted, provided the original author(s) and the copyright owner(s) are credited and that the original publication in this journal is cited, in accordance with accepted academic practice. No use, distribution or reproduction is permitted which does not comply with these terms.
*Correspondence: Keiji Jindo, a2VpamkuamluZG9Ad3VyLm5s