- 1School of Economics and Management, Jiangxi Agricultural University, Nanchang, China
- 2Accounting Institute, Wuxi Taihu University, Wuxi, China
Introduction: Leading the green transformation of agriculture through the digital economy is a crucial step toward high-quality agricultural growth. The role and mechanism of China's digital rural construction in promoting the green development of the whole process of agricultural production needs further analysis.
Methods: Based on the panel data of prefecture-level cities in Henan Province from 2013 to 2022, we used the entropy topsis model, the fixed effect model, the Tobit regression model, the intermediate effect model, and the threshold effect model, aiming to explore the effects of digital village construction on agricultural green development and their regional differences, impacts paths and non-linear effects.
Results: The study found the following: (1) The level of agricultural green development and the digital village construction have increased in Henan province, with the latter exhibiting a spatial clustering phenomena. (2) Digital village construction has a significant positive effect on green agricultural development, but this effect does not exist in mountainous areas. (3) The digital village construction can impact green agricultural development through the updating of agricultural structures. (4) The positive influence of digital village construction on green agricultural development is non-linear, with a single-threshold and increasing marginal effects.
Discussion: These findings provide references for other countries to use digital elements to promote green and sustainable development of agriculture. The main contribution of this study is to explore the role of agricultural industrial structure upgrading in the impact of digital rural construction on agricultural green development.
1 Introduction
Digital Village Construction (DVC) is the crucial content of digital construction, and the main representation of inclusive sharing of the digital economy, and an core method for implementing rural revitalization strategy. Countries around the world attach great importance to the application of digital elements in rural areas, and carry out a series of practices to promote the digital transformation of villages, such as the “Rural Broadband ReConnect” in the United States (Wang and Jayakar, 2024), the “Smart Villages” in the European Union (Adesipo et al., 2020), the “Countryside Online” in the Russia (Schmidt, 2011), and the “Smart Rural Communities” in the Colombia (Calzada, 2023). In 2018, China's No. 1 Central Document put forward the “digital village” strategy. Meanwhile, a series of policies such as the “Digital Village Development Action Plan (2022–2025),” and “Digital Village Construction Guide” were deployed to outline the blueprint for DVC.
The swift advancement of agriculture has resulted in the decline of the ecological environment. The imbalanced approach to agricultural development, primarily centered on economic interests, has intensified resource and environmental limitations. The adoption and application of essential production technologies constitute the core of green agricultural development. DVC serves as a novel catalyst for the modernization of agriculture and rural regions (Agusta, 2023), advocating for the incorporation of contemporary information technologies in agricultural practices and rural development (Li W. et al., 2023). The coordinated development between economic growth and ecological sustainability in China's agriculture urgently needs to promote the network, information and digital elements to empower agricultural production through relying on the DVC.
Digital village is a development form to realize the digitization of rural production, life and governance through information, network and intelligent elements (Li W. et al., 2023; Mei et al., 2022). The essence of DVC is to promote the construction of rural digital industry based on digital factor input, empower the transformation of agricultural industry with new productivity. Several studies have explored the correlation between DVC and agricultural advancement to assess the impact of digital elements on fostering high-quality agricultural growth (Ren et al., 2024). Scholars generally agree that DVC, supported by technological innovation and the flow of information, can reshape the agricultural supply chain and lead to the systematic and intelligent transformation of agriculture (Zhao et al., 2022). Meanwhile, the development of the rural digital economy can facilitate a reallocation of agricultural resources and an organizational division of labor, thus advancing agricultural modernization (Mei et al., 2022). Consequently, several studies have investigated the effects and mechanisms of DVC on the agricultural sector, discovering that DVC not only directly fosters the expansion of the agricultural economy (Wang et al., 2023), but also drives the development of the agricultural economy through technical advancement (Lu et al., 2024) and agricultural credit support (Cao et al., 2022). In addition, DVC can also reallocate agricultural resources such as labor, capital and land (Fu and Zhang, 2022), stimulate entrepreneurial activities of new agricultural formats (Lu et al., 2024b), and improve agricultural production efficiency through industrial agglomeration (Liu J. et al., 2024) and industrial integration (Zhang et al., 2023), thereby promoting agricultural development.
Current studies regarding the impact of DVC on green agricultural development mainly on macro and micro aspects. At the macro level, researchers primarily examine the impact of DVC on the agricultural green total factor productivity (AGTFP). For example, certain experts have determined that the digital transformation of villages can markedly enhance the green efficiency of land (Tang and Chen, 2022), and further enhance the AGTFP (Cai and Han, 2024). Moreover, DVC can enhance the AGTFP through numerous means, including the degree of human capital (Chen et al., 2023), agricultural technology progress (Lin and Li, 2023), agricultural informatization (Cai and Han, 2024) and mitigation of resource misallocation (Zhang et al., 2024). Some scholars also investigated the spatial spillover effect and non-linear effect of digital villages on the AGTFP (Lu et al., 2024b; Xu, 2024), but those studies of this non-linear effect have variously concluded that it is “increasing” (Hong et al., 2023; Liu and Liu, 2023) and decreasing (Jin et al., 2024), first increasing and then decreasing (Lu et al., 2024b). On the micro dimension, researchers mainly examined the influence of DVC on farmers' production methods (Lu et al., 2024a). Digital technology applications and digital literacy enhancement significantly reduce the farmers' use of chemical pesticides (Xie et al., 2024), and promote their adoption of green production technologies (Yang et al., 2024).
In summary, despite extensive research on the link between DVC and agricultural progress, particularly about green agricultural development, there remains considerable room for further exploration in this field. Firstly, the agricultural green development evaluation index system neglecting the influence of industrial production and residential activities on the agricultural production environment. In fact, pollutants in industrial emissions and domestic sewage will reduce the disease resistance of crops (Burney and Ramanathan, 2014; Liu R. et al., 2024), decreasing agricultural product yield (Bell et al., 2011). In addition, pollutants can cause harm to the human body through the enrichment of crops (Liu R. et al., 2024). Secondly, there are still differences in the non-linear relationship between DVC with agricultural green development. The marginal effect of 'increasing' or “decreasing” needs to be further verified. Furthermore, although current study mostly investigates the influence mechanism of DVC on agricultural green growth through production factors, overlooking the effect of agricultural structure. In fact, upgrading the agricultural structure exemplifies the enhancement of agricultural quality (Zhang H. et al., 2022), which facilitate the incorporation of digital technologies into agricultural and rural areas (Hua et al., 2024).
Therefore, this study has three innovations compared to the existing literature. First, enrich the evaluation system of agricultural green development. The existing research mainly focuses on agricultural non-point source pollution, ignoring the pollution of industrial production and residents' lives. In order to ensure that the evaluation system of agricultural green development is more comprehensive, relevant indicators are added to the evaluation system of agricultural green development to examine the negative externalities of industrial production and villagers' lives on agricultural green development. Second, explore the non-linear impact of DVC on agricultural green development. There are different conclusions on the marginal role of DVC in promoting agricultural green development. This study uses the threshold effect model to examine the non-linear impact of DVC on agricultural green development in order to solve the difference between the “increasing” and “decreasing” marginal effects in existing research. Thirdly, reveal the impact of upgrading agricultural structures in DVC and agricultural green development. At present, the research ignores the role of agricultural industrial structure. After clarifying the theoretical mechanism, this study empirically explores the intermediary role of upgrading agricultural structures between DVC and agricultural green development.
The structure of the rest of this study is as follows: The second part is a theoretical analysis, which describes the mechanism of DVC affecting the green development of agriculture. The third part is the scheme design, which introduces the analysis method and variable selection. The fourth part is the spatial and temporal evolution characteristics of DVC and agricultural green development. The fifth part is the quantitative analysis of the impact of DVC on agricultural green development, and it analyzes the intermediary role of upgrading agricultural industrial structures and the threshold role of DVC. The sixth part puts forward conclusions and suggestions.
2 Theoretical analysis and research hypothesis
2.1 The impact of DVC on agricultural green development
In the process before production, DVC can optimize the distribution of agricultural elements. First, DVC can optimize the land elements (Zhang F. et al., 2022). Land fragmentation limits the scale effects in land production, further limiting the efficiency of agriculture production (Knippenberg et al., 2020). DVC can generate non-agricultural employment opportunities and facilitate workforce transition from agricultural to non-agricultural sectors (Liu and Liu, 2024), thereby increasing the probability of land transfer, improving land use efficiency, and achieving large-scale operation of agriculture. Second, DVC can optimize the allocation of labor elements. DVC attracts skilled talent, which enhances the agricultural labor force and nurtures new agricultural management entities (Cai and Han, 2024). This process promotes the specialization and enhancement of agricultural productivity, ultimately altering the prior large farming model.
During the agricultural production process, DVC can enhance production efficiency and mitigate agricultural pollution. From one perspective, the DVC can eliminate information barriers (Leong et al., 2016) and alleviate market information asymmetry by transmitting effective supply and demand information in the agricultural market (Bhaskara and Bawa, 2021), thus assisting farmers in modifying their production structure and mitigating the opacity of production decisions to enhance production efficiency. From another perspective, DVC can integrate environmentally friendly technological elements into agricultural and rural development. Digital technologies, with their substitution effect on traditional agricultural production methods, can reduce over-reliance on material resources and fossil energy (Yang et al., 2024). Agricultural big data can effectively monitor the excessive use of agricultural chemicals, thereby alleviating surface source pollution in agriculture (Wu Y. et al., 2024).
In the process after production, DVC can enhance the efficiency of agriculture production and operations. Firstly, DVC empowers agricultural technology innovation with digital technology (Abiri et al., 2023). Technological developments have expanded the agricultural production curve, resulting in heightened crop yields (Deichmann et al., 2016). Meanwhile, technological innovation contributes to lower marginal production costs and higher profitability levels for farmers and agribusinesses. Secondly, the specialization of production brought by DVC is beneficial for the extension of agricultural industrial chain (Klingenberg et al., 2022). The intensive processing of agricultural products enhances the added value of agricultural products, thereby increasing the efficiency of agricultural output. Therefore, the following hypotheses are postulated:
Hypothesis 1: DVC has a positive impact on agricultural green development.
2.2 The mediating role of the upgrading for agricultural structure
The agricultural structure was defined the proportion of each subdivision industry, including the composition of agriculture, forestry, animal husbandry, fishery, and each service (Liu J. et al., 2024). Under urban-rural dual structure in China, the development of the primary service industry, such as rural agricultural and forest product processing, is lagging. The upgrading of agricultural structure facilitates the transition of rural primary industry to secondary and tertiary sectors while simultaneously elevating the quality of all three sectors (Wang et al., 2024). In our study, upgrading agricultural and industrial structures is defined as the optimal allocation of agricultural resources among various agricultural sub-areas, gradually transferred to agricultural product processing, agricultural socialization services, and agricultural variety cultivation.
The DVC offers a novel framework for enhancing the advancement of agricultural industrial frameworks (Wang et al., 2024). Firstly, DVC can optimize the agricultural product supply chain by introducing information and digital management tools for agricultural processing enterprises. For instance, food companies can reduce the spoilage of raw agricultural products during the transition from “field” to “workshop” by accurately controlling the storage environment in the circulation process (Luo et al., 2024). As a result, the stable assurance of both the quantity and quality of raw material supply ensures the economic benefits of agro-processing sector, thereby facilitating the upgrading of the agricultural structure. Secondly, DVC promotes agricultural information integration and data sharing, thereby supporting the development of agricultural service platforms (Bhaskara and Bawa, 2021). The DVC can integrate the data of the entire agricultural supply chain and then enhance socialized services such as technical guidance, financial support, supply and demand docking, and market forecasting for farmers relying on agricultural information service platforms (Mushi et al., 2024). Meanwhile, the application of remote sensing and IoT (Internet of Things) technologies can help farmers obtain personalized agricultural service plans (Zhang M. et al., 2022) based on their land endowment and crop conditions. This fosters the standardization and precision development of agricultural social service platforms, ultimately leading to the evolution of agricultural structure. In addition, DVC drives innovation in resource elements and creates new scenarios like intelligent agriculture and bio-agriculture (Misra et al., 2022). It not only helps overcome the limitations of traditional single-function agricultural production but also facilitates the integration of primary, secondary, and tertiary industries so as to promote the upgrading of agricultural structures (Yan and Cao, 2024).
The upgrading of the agricultural structure facilitates the green development of agriculture (Zhang H. et al., 2022). Initially, it promotes the growth of agro-processing sector. In the process of expansion, the imbalance in supply and demand for raw agricultural commodities prompts companies to use contract farming and similar methods to manage this challenge. The development of contract farming helps farmers optimize their cropping structure and avoid the risk of market uncertainty (Vamuloh et al., 2020). At the same time, the establishment of contractual relationships between enterprises and farmers can regulate farmers' production behaviors (Liu et al., 2018), such as eliminating the overreliance on conventional chemical fertilizers and insecticides. Secondly, agricultural social services can promote moderate-scale agricultural management through land trusteeship and substitute farming (Cai et al., 2022). By reducing the cost of applying digital and intelligent agricultural equipment, scale agricultural operations can improve production factor utilization efficiency (Wang et al., 2022), thereby driving agriculture's intensive and green development. Thirdly, the upgrading of agricultural structure exerts a siphon effect on social capital, which facilitates the development of rural agricultural machinery financial credit business (Li G. et al., 2023). The development of agricultural machinery financial credit business provides funding for the renewal of agricultural production equipment (Liu C. et al., 2023), which helps enhance farmers' capacity for large-scale operations. In addition, upgrading agricultural structures has the effect of technological progress. Agricultural technology research can overcome the constraints of agricultural resource endowment and facilitate the transition of agriculture from being resource dependent to technology-integrated (Hong et al., 2022). Therefore, the following hypotheses are postulated:
Hypothesis 2: DVC can affect the green development of agriculture by upgrading agricultural structure.
Based on the above analysis, we construct a theoretical model of DVC affecting agricultural green development. Figure 1 shows the theoretical model.
3 Methodology and materials
3.1 Variables selection
3.1.1 Dependent variable: agricultural green development
Agricultural green development is an innovative approach to agriculture that achieves the harmonious coexistence of humanity and nature. It is characterized by efficient use of resources and environmental friendliness. Agricultural green development is not only a change in production methods but also a path toward sustainable development under the guidance of green values. Green practices permeate the entire agricultural production process, from pre-production through production to post-production. In order to reveal the status of agricultural green development, this study is based on the definition of the concept and reference to previous literature, constructs a measurement system including 16 specific indicators from the four dimensions of green production environment (pre-production), agricultural production input (pre-production), green production mode (production), and agricultural green output (post-production).
Green production environment dimension. The green production environment reflects the point source pollution of the regional ecological environment. Pollutants in industrial emissions and domestic sewage will reduce the disease resistance of crops (Burney and Ramanathan, 2014; Liu R. et al., 2024), resulting in a decrease in the yield of agricultural products (Bell et al., 2011). Therefore, the ecological environment of agricultural production is measured by the emission of sulfur dioxide and industrial dust in industrial production, the treatment of domestic sewage by farmers and the greening degree of villages.
Agricultural production inputs dimension. Green development of agriculture depends on the effective input of production factors (Wu X. et al., 2024). This study examines the input of green agricultural production from the perspective of labor input, agricultural machinery input, and agricultural capital input.
Green production model dimension. Green production mode reflects the non-point source pollution caused by agricultural production to the ecological environment. The chemical elements such as nitrogen and phosphorus in fertilizers, pesticides, and livestock manure can cause pollution of underground and surface waters (Luo et al., 2024). In addition, the incomplete treatment of residual film will also lead to the decline of cultivated land quality and output level (Liu et al., 2014; Uzamurera et al., 2023). Therefore, the use intensity of pesticides, fertilizers, agricultural film and the comprehensive utilization rate of livestock and poultry manure are used to measure the green production mode of agriculture.
Agricultural green outputs dimension. Green output directly measures agricultural green development results and emphasizes agricultural output efficiency (Zhang F. et al., 2022). The output per unit of energy consumption, cultivated land area, agricultural practitioners, and agricultural water consumption reflect the output level of agricultural energy saving and high efficiency. Specific indicators are set as shown in Table 1. The entropy weight TOPSIS method is employed to calculate agricultural green development level.
3.1.2 Core independent variable: digital village construction
The digital rural strategy was first proposed by the central document No. 1 in 2018. The connotation was defined as empowering rural economic development through informatization, networking, and digitization, promoting the upgrading of rural infrastructure and public services, and achieving rural areas' modernization transformation and development. Referring to the official definition and existing researches (Jin et al., 2024; Mei et al., 2022), this paper holds that the essence of DVC is to promote the construction of rural digital industry based on digital factor input, empower the transformation of agricultural industry with new productivity. Based on the above definition and existing researches, this study assesses the level of DVC from across dimensions: digital industry foundation, agricultural industry digitization, and rural digital industrialization. The specific indicators are set as shown in Table 2, entropy weight topsis method is used to calculate the level of DVC.
Foundation of digital industry dimension. Digital technology and elements are the basis of digital rural construction (Agusta, 2023). Scientific and technical services provide technical support for the digital industry, while the transportation industry supports the logistics base and the information industry supplies the data elements. Therefore, the investment of these indicators is selected to reflect the basic situation of the digital industry. In addition, rural electricity consumption is added to the system as it reflects the number and intensity of use of rural appliances, which provides a facility base for the digital transformation of villages.
Digitalization of agricultural industry dimension (Lu et al., 2024b). The digitization of rural industry reflects the integration of modern information technology and rural industries. Water-saving irrigation and facility agriculture realize agricultural digitization through automation equipment. Taobao village construction reflects the development of rural e-commerce, which is the performance of the digitalization of rural commerce. In addition, digital inclusive finance represents the degree of rural financial digitization.
Agricultural digital industrialization dimension. Rural digital industrialization indicates the scale and industrialization of the rural digital industry. The scale of rural digital industries such as cable TV, Internet, mobile phones and modern logistics can reflect the level of digital rural construction (Wang and Tang, 2023).
3.1.3 Control variable
This study refers to previous literature (Wu X. et al., 2024; Zhang F. et al., 2022; Zhu et al., 2023) to include a range of control variables within the model to mitigate the endogeneity issue resulting from variable omission. The control variables of this study are as follows: (1) Financial support for agriculture (FSA), measured by the proportion of local government expenditure on agriculture, forestry, and water in fiscal expenditure. (2) Rural infrastructure, the rural road hardening rate measures the level of RI. (3) Opening up (OU), measured by per capita foreign actual investment. (4) Agricultural security (AS), quantified by the proportion of agricultural insurance premium revenue to the total value of agricultural output. (5) Agricultural organization is measured by the number of agricultural cooperatives per 10,000 people. (6) Technological progress (TP), is quantified by the proportion of research and technology expenditure to GDP in each city.
3.1.4 Mediator variable
According to the research hypothesis, the upgrading of agricultural structure (UAS) is selected as the intermediary variable. It is expressed by the proportion of the output value of the agricultural products processing sector to that of the primary sector. The natural logarithm was used for all relevant variables to address potential heteroskedasticity in the data.
3.2 Baseline regression model
In this study, a panel model is developed to evaluate the influence of DVC on agricultural green development:
Where i and t represent the city and year, respectively. Greenit is the dependent variable, which signifies the extent of agricultural green development of city in year t. As the core explanatory variable, Digitalit represents the level of DVC in the city i in t years. controlit denotes a series of control variables that influence the green development of agriculture, including the level of rural infrastructure, the level of technological progress, the level of financial support for agriculture and the level of openness to the outside world. β0, β1, and β2 indicate the parameters to be estimated. μi and μt denote regional fixed effect and time fixed effect, respectively. εit represents the random error term.
Additionally, considering that the level of DVC is a constrained dependent variable with values >0, using traditional OLS regression may lead to biased results. Therefore, the Tobit model is constructed to further investigate the impact of DVC on agricultural green development. The Tobit model is formulated as follows:
3.3 Mediation effects model
To investigate the contribution of agricultural structure upgrading in the DVC affecting the green development of agriculture, a mediating effect model is constructed. The model is constructed as follows:
3.4 Threshold regression model
A threshold model is constructed to test the non-linear effects of DVC. The levels of DVC were set as threshold variables. The threshold model is specified as follows:
In the threshold model, I(·) denotes the indicator function. θit is the threshold variable, and ω is the threshold value. Only single-threshold models are considered here.
3.5. Research area and data source
3.5.1. Research area
Henan Province is situated in the central and eastern regions of China (Figure 2), encompassing the middle and lower reaches of the Yellow. It has 17 prefecture-level cities and 1 provincial city (Figure 3). Most of Henan Province is located in the warm temperate zone, with its landscape composed of plains and basins, mountains, hills and water surface. Thus, it is the core area of China's grain production and an advantageous production area for cash crops. Meanwhile, Henan is an important communication hub because China's fiber optic cable backbone network, “eight vertical and eight horizontal” has “three vertical and three horizontal” through its jurisdiction. Therefore, in order to facilitate the comprehensive integration of information technology with agriculture and rural regions, the Henan Provincial Government proposed the “Implementing Opinions on Accelerating Agricultural Informatization and Digital Countryside Construction” in 2020. Therefore, the DVC in Henan Province has developed rapidly, with multiple digital rural demonstration areas established. Although the DVC can reshape the countryside with modern information technology (Dumitru et al., 2021), it remains to be explored whether it will advance the green transformation of agriculture and alleviate the pressure on environmental carrying capacity. The digital village strategy was officially proposed by the Chinese government in 2018. The data for the 5 years before 2018 and the 5 years after 2018 are representative. Therefore, the analysis is based on the data from 2013 to 2022.
The study area was Henan Province because of its representativeness in terms of geomorphic features, crop structure, economic situation and population size. Henan Province is located in the central parts of China, spanning the Yangtze River, Huaihe River, Yellow River, and Haihe River. Its landscape is rich and representative, including plains and basins, mountains, hills, and water. Meanwhile, its planting structure covers the main food crops in China, such as wheat, rice, corn, and soybean, which has a diversity of crop types. In addition, the economic development level of Henan Province is located in the middle reaches of China, and its rural population is 42.39 million, accounting for 8.6% of the national rural population.
3.5.2 Data source
This research employs panel data from 18 cities in Henan Province covering the period from 2013 to 2022. The data come from Henan Provincial Statistical Yearbook, China Urban Statistical Yearbook, Henan Provincial Water Resources Bulletin, China Economic Network Statistical Database County Annual Library, China Taobao Village Research Report, China County Statistical Yearbook, WIND database and Statistical Yearbooks, and Bulletins of each cities. Missing data are supplemented using the interpolation method. Table 3 shows the result of descriptive statistics of variables.
4 Spatial-temporal pattern analysis of digital village construction and agricultural green development
4.1 Time series evolution analysis of digital village construction and agricultural green development level
Figure 4 reports the score changes of DVC and agricultural green development measured by The CRITIC-entropy weight TOPSIS methods. For the DVC, the score of Henan Province rose from 0.532 in 2013 to 1.109 in 2022, which showed a steady growth trend. Meanwhile, all the first-level indicators have experienced a gradual upward evolution process, among which the digital industry foundation score has the fastest growth rate, increasing from 0.136 to 0.353; the scores of agricultural industry digitization and agricultural digital industrialization increased from 0.158 and 0.238 to 0.309 and 0.447, respectively. Overall, the DVC in Henan Province requires further improvement.
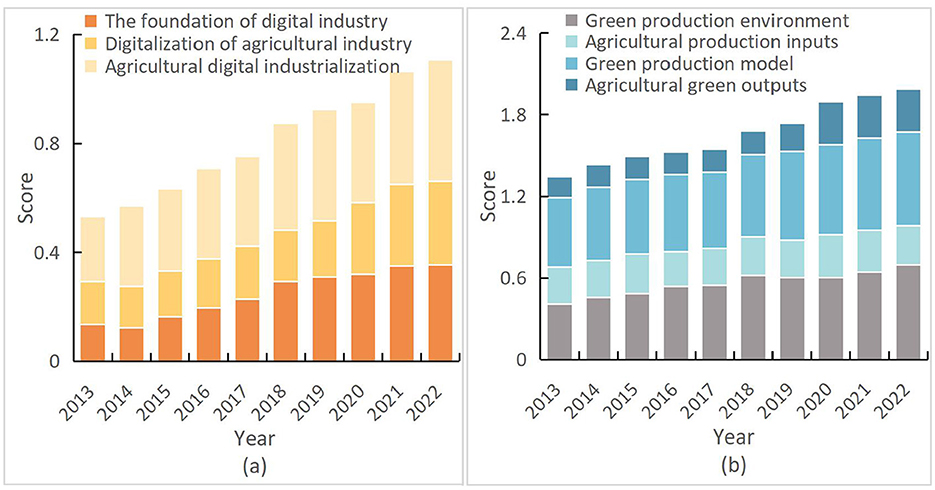
Figure 4. The temporal evolution characteristics of digital village construction (a) and agricultural green development (b) with their each dimension.
For the green development of agriculture, the score rose from 1.347 in 2013 to 1.991 in 2022. Meanwhile, green production environment and production method scores are high, showing an evolutionary pattern of increase yearly, from 0.409 and 0.509 to 0.697 and 0.687, respectively. The scores of agricultural production input and agricultural green output were low and showed the development characteristics of fluctuating rise, which increased from 0.273 and 0.156 to 0.286 and 0.321, respectively.
4.2 Spatial characteristics of digital village construction and agricultural green development level
In order to explore the spatial distribution of DVC and agricultural green development in Henan Province, Moran's index was used to analyze their spatial characteristics. Table 4 presents the Moran index of DVC and agricultural green development in Henan Province. The global Moran's index for DVC is significantly positive in most years. The results show a significant positive spatial correlation in DVC. Nevertheless, the spatial spillover effect is gradually reduced, indicating that the impact of DVC on the surrounding cities is gradually weakened. The possible reason is that with the implementation of the DVC strategy, the level and endogenous power of DVC in various regions have gradually increased. Therefore, the impact of DVC on other cities has gradually weakened.
In contrast, the Moran index of agricultural green development is significant in only a few years, indicating a weak spatial association in green development of agriculture. A possible reason is the significant differences in resource endowments and economic structures among cities in Henan Province, which result in varying economic contribution rates of the primary industry. Consequently, no spatial agglomeration effect of green agricultural development has been formed.
5 Analysis of empirical results
5.1 Stationarity tests
To ensure the reliability of pertinent variables in model estimate and to prevent spurious regression issues, it is essential to assess the stationarity of the data. The relevant data of this study are short panel data, so the HT test is used for the unit root test. The test results indicating that the data is a stationary sequence. Meanwhile, the VIF (variance inflation factor) is applied to test multicollinearity. The results show that the mean VIF of the relevant variables in this study is 1.86, demonstrating that multicollinearity is not present.
5.2 Benchmark regression
Table 5 displays the influence of DVC on agricultural green development. Model (1) presents the OLS regression results, while Models (2) and (3) shows the results of the fixed effects model and the random effects model, respectively. Meanwhile, the green development of agriculture is a limited dependent variable >0, and the estimation outcomes of the Tobit model are more reliable. Models (4, 5) presents the estimation outcomes of the fixed effect Tobit and random effect Tobit models. The influence coefficient of DVC on green development of agriculture is significant in each model, indicating that DVC can promote agricultural green development. DVC empowers the whole agricultural production process, improving the agricultural ecological environment, increasing input-output efficiency, and optimizing agricultural production methods. Meanwhile, the fixed-effects panel regression model exhibits the highest goodness of fit. Consequently, the fixed panel model is utilized in the subsequent analysis.
Table 5 also presents the regression results of control variables, indicating that the level of rural infrastructure, the degree of organization, and the level of technical advancement significantly influence the green growth of agriculture, corroborating previous study findings (Song et al., 2014; Yu et al., 2022). The impact of financial support for agriculture on agricultural green development is negative. The possible reason is that the efficiency of financial funds for supporting agriculture could be higher in the stage of production structure transformation of China's agriculture, which constrains the green transformation of agriculture. In addition, the level of external openness adversely affects the green development of agriculture. A possible reason is that the heightened demand for agricultural commodities compels farmers to augment their output by increasing the application of pesticides and fertilizers, hence resulting in agricultural non-point source pollution (Jalal Kazemi and Samouei, 2024).
5.3 Endogeneity test
5.3.1 System GMM model
Although more control variables are introduced and the fixed effects of year and region are controlled in the benchmark regression, endogeneity concerns may persist between DVC and green agricultural growth. Recognizing that agricultural green development is a protracted endeavor, a system GMM dynamic panel regression model is established to deal with endogenous bias.
Model 1 in Table 6 presents the regression outcomes of the system GMM model. The AR (1) test indicates the presence of first-order serial correlation in the differenced equation (P < 0.05). At the same time, the AR (2) results show no second-order serial correlation (P > 0.10), verifying that the model meets the autocorrelation test criteria. Meanwhile, the Hansen test findings demonstrate that instrumental variable was valid. Thus, the regression result of system GMM is consistent and reliable. In conclusion, the positive impact of DVC on green agricultural development remains significant after considering the dynamic effect of the model, supporting the reliability of the baseline regression results.
5.3.2 Instrumental variable method
DVC promote the green development of agriculture. However, the level of DVC in areas with higher levels of agricultural green development may also be higher, which leads to two-way causal problems. The DVC mean value of adjacent cities is used as an instrumental variable to solve the endogenous problem. On the one hand, the results of Table 4 show that there is a spatial spillover effect of DVC, which proves that the DVC of the adjacent area has an impact on the DVC of the region. DVC will have a demonstration effect on the adjacent areas, so that the variable correlation requirements are met. On the other hand, the DVC of adjacent cities will not have an impact on the green development of agriculture in the region. The impact of DVC on agriculture depends on the local resource endowment and policy environment, while the impact on the green development of agriculture in adjacent areas is weak, so the exogenous requirements are met.
Therefore, mean value of DVC in adjacent cities is used as instrumental variable and regressed using a two-stage 2SLS. The first stage estimation findings indicate that the coefficient of the instrumental variable's influence on the explanatory variable DVC is significant at the 1% level. Meanwhile, the statistics of F for the Kleibergen-PaapWaldrk test and the Cragg-DonaldWald test (624.10 and 99.50) are greater than the Stock-Yogo critical value of 16.38, which demonstrates that there is no under-identification problem with the instrumental variables and no weak instrumental variable problem. Model (2) in Table 6 demonstrates the results of the second estimation, and the influence of DVC on agricultural green development remains significant after correcting the endogeneity problem.
5.4 Robustness check
This study employs three methods for robustness testing to verify the accuracy of the benchmark regression results.
(1) Replace the measurement of the explanatory variable. The entropy weight method is used to re-measure the green development of agriculture. Model (3) in Table 5 presents the results of substituting this measure into the fixed effects model. The coefficient of DVC continues to be significant.
(2) Replace core explanatory variable. In recent years, China has built national and provincial DVC pilots, which have promoted regional rural digital transformation through experience sharing and demonstration promotion. Therefore, the core explanatory variable DVC is represented by whether there is a digital village pilot in the region. Table 5 Model (4) shows the regression results. The impact of DVC on agricultural green development is still significant after replacing the proxy variables of the core explanatory variables.
(3) Winsorization. The main variables are bilaterally winsorized at the 5% level to mitigate the impact of data outliers on the empirical model. Model (5) in Table 5 presents the regression results after winsorization. The influence of DVC on green agricultural development remains robust.
(4) Change the time window. The digital village strategy was officially proposed by the Chinese government in 2018. In order to remove the impact of policy inclination, the sample data in the interval of 2013–2017 are selected for regression. Model (6) in Table 5 shows the regression results, where the impact of DVC on the green development of agriculture is significantly positive.
5.5 Heterogeneity analysis
Based on “The Outline of the Plan for Comprehensively Building a Well-off Society in Henan Province,” Henan Province has been divided into four economic zones: Central Plains Urban Agglomeration, Northern Henan, Western and Southwest Henan, and Huanghuai Region. Different economic levels, industrial structures, and resource endowments in different economic zones lead to possible differences in the impact of DVC on green agricultural development. Models (1)–(4) in Table 7 show that DVC has a significant positive impact on agricultural green development in most economic zones in Henan Province. The influence coefficient of DVC shows the order of “Northern Henan > Huanghuai Region > Central Plains Urban Agglomeration,” while the coefficient of Western and Southwestern Henan is not significant.
Cities in Northern Henan are in the stage of industrial restructuring, and digital resource elements are tilted from industry to agriculture, which in turn promotes the innovative combination of agricultural production and digital elements and provides support for the green development of agriculture. The Huang-Huai Region is densely covered with river networks and the terrain is dominated by plains, which has superior agricultural development conditions. The advanced production and management mode caused by DVC can optimize the allocation of agricultural resources and reduce production costs. Therefore, the Huanghuai Region has fully utilized the role of digital elements in empowering the green development of agriculture. However, the promotion effect of DVC on the Central Plains Urban Agglomeration is lower than the average level. The reason may be that although the region is more developed, which makes the rural digital infrastructure perfect, the lower dependence on the agricultural industry makes the digital elements not yet fully integrated with the agricultural industry. Additionally, the landforms in Western and Southwestern Henan are dominated by mountains and hills, which leads to the high cost of digital rural infrastructure construction. Therefore, digital elements cannot drive the green transformation of agriculture.
5.6 Analysis of the mediation effect
Table 8 presents the outcomes of the three-step mediation effect analysis. The coefficient of DVC in the model (1) of Table 8 is positive and significant at the 1% level, indicating that the total effect of DVC on agricultural green development is positive. The coefficient of DVC in model (2) of Table 8 is significantly positive, proving that DVC positively affects the upgrading of agricultural structure. DVC provides advanced elements and digital technology for upgrading agricultural structure and promotes the transition of agricultural industry to technology-intensive industry (Hua et al., 2024). The impact of DVC on agricultural green development is robust positive after adding intermediary variables to the model (3), indicating that the indirect effect is significant. Besides, the upgrading of agricultural structure also has a obvious influence on the green development of agriculture, indicating that the direct effect is significant.
According to the results of Models (1)–(3) of Table 8, the coefficient of DVC in Models (2)–(3) and the coefficient of agricultural structure upgrading in Model (3) are significantly positive, indicating that some mediating effects exist. DVC can affect the green development of agriculture through the upgrading of agricultural structure. DVC can affect the green development of agriculture through the upgrading of agricultural structures. Hypothesis 3 supports that DVC can influence the green development of agriculture through upgrading of agricultural structure. Furthermore, the mediating effect of agricultural structure upgrading (0.164 * 0.310) accounted for 48% of the total effect (0.105). This result indicates that 40% of the impact of DVC on agricultural green development is mediated through the upgrading of the agricultural structure.
In addition, the Sobel test is carried out to ensure the robustness of the mediating effect test. The Sobel test method is especially suitable for the fixed effect model. Table 8 demonstrates the significant results of the Sobel test and the amount of mediating effect of structural upgrading of the agricultural industry was 48%. Therefore, hypothesis H2 is verified.
5.7 Threshold effect analysis
In order to explore the non-linear influence of DVC on agricultural green development, this study uses DVC as a threshold variable to test the threshold effect. The threshold effect test results are displayed in Table 9. DVC passed the double threshold test as a threshold variable, and the threshold values were 0.756 and 1.148, respectively. Thus, a double-threshold regression model is built in this work.
Table 8 model (4) shows the threshold regression results. The impact of DVC on farmers' green development is divided into two stages. The impact coefficient of DVC is 0.211 when its value is below the threshold of 0.756. However, when the value falls between the first threshold of 0.756 and the second threshold of 1.148, the impact coefficient increases to 0.325. Furthermore, when the value surpasses the second threshold of 1.148, the impact coefficient is further enhanced to 0.724.
6 Discussions and recommendations
6.1 Discussions
DVC and agricultural green development levels in Henan Province showed an upward trend. Consistent with the findings of Jin et al. (2024) and Mei et al. (2022), China's rural digitization level and AGTFP have gradually increased under the impetus of rural revitalization strategies. Furthermore, DVC exhibits a spatial spillover effect, where the DVC in a given region is positively influenced by the advancements in neighboring areas. In contrast, agricultural green development does not exhibit spatial spillover effects, which differs from the findings of Fu and Zhang (2022). The possible reason is that agriculture needs to rely on resource endowments such as land, which has spatially fixed attributes. The differences in regional resource endowments and development models will lead to uneven implementation of green development policies, thus weakening spatial correlation (Liu Y. et al., 2023).
In line with the study by Zhang et al. (2024), DVC significantly impacts agricultural green development. DVC empowers the whole process of agricultural production and promotes the improvement of the agricultural ecological environment (Ren et al., 2024), the improvement of the input-output efficiency (Zhang F. et al., 2022), and the optimization of production mode (Yang et al., 2024). The substitution of digital technology for traditional agricultural production technology can improve the efficiency of agricultural output and reduce the problem of agricultural non-point source pollution (Wu Y. et al., 2024). However, heterogeneity exists in the impact of DVC on agricultural green development. Specifically, DVC has less impact on green development of agriculture in economically developed areas, consistent with previous studies (Fu and Zhang, 2022). In areas with advantageous agricultural resource endowments (e.g., the plains), DVC can significantly promote the integration of digital elements with agricultural production, thereby realizing the green development of agriculture. In contract, the impact of DVC on the green development of agriculture in mountainous areas is not significant, which is inconsistent with the previous literature (Cai and Han, 2024). The construction cost of DVC-dependent digital infrastructure in mountainous areas is relatively high, and low-value-added agriculture cannot afford it. Therefore, the role of DVC in promoting agricultural greening has not yet appeared.
DVC can promote agricultural green development by upgrading the structure of the agricultural industry (Cai and Han, 2024; Lin and Li, 2023) on the mechanism of DVC promoting agricultural green development. The transformation of agricultural technology, factor innovation, and capital deepening caused by the DVC benefit farmers by optimizing agricultural structure (Wang et al., 2024). Farmers thus have the economic ability and green literacy to integrate digital elements into agricultural production (Abiri et al., 2023; Yang et al., 2024). Consequently, the goal of the digital village strategy of leading the green development of agriculture and rural areas with digital technology is realized.
With the improvement of the level of DVC, the diffusion effect on the green development of agriculture has gradually increased. This finding confirms the existing research conclusions (Hong et al., 2023; Liu and Liu, 2023). The possible reason is that technological progress changes the traditional mode of agricultural production with a time lag. Once the digital village has reached its threshold, the economies of scale of digital technology for greening agriculture have begun to be realized (Li W. et al., 2023). Digital and traditional agricultural elements such as talent, land, and capital are gradually approaching the optimal combination ratio, reinforcing the promotion of green agricultural development.
6.2 Policy recommendations
The spatial spillover effect of DVC, the green development of agriculture does not exhibit such effects. Therefore, the government should use the spatial spillover effect of DVC and avoid the widening gap of agricultural green development in various regions. On the one hand, the government should establish a regional collaboration mechanism to promote sharing resources and experience in digital infrastructure construction and information technology applications in neighboring areas. This would ultimately create a coordinated development framework for digital village construction, where advanced regions drive the progress of less developed ones. On the other hand, the government should formulate region-specific policies for the green development of agriculture in response to the resource endowment characteristics of different regions. For regions with weak resource endowments, the agricultural sector should provide financial support and promote measures such as water-saving irrigation, land improvement, and green agricultural technology to enhance their green production capacity.
In view of the heterogeneity of the impact of digital rural construction on agricultural green development, policy formulation should be adapted to the terrain conditions of each region. In plain areas, it is crucial to further deepen the integration of digital elements with green agricultural production. Policy incentives, such as tax reductions and targeted subsidies, should encourage enterprises to increase investment in DVC projects in plain areas to further enhance the efficiency of agricultural green development. For high construction costs in mountainous areas, scientific research departments should develop low-cost digital technologies suitable for terrain and economic conditions, such as UAV monitoring, small sensor networks, etc. In addition, mountain areas should develop characteristic agriculture (such as organic agriculture and high-value agricultural products) to enhance the carrying capacity of agriculture to digital technology.
Promote the application of digital technology in agricultural product processing and agricultural social services. Encourage local governments to cooperate with agricultural science and technology enterprises to establish industrial parks according to regional agricultural resource endowments and industrial structure. The agglomeration of agricultural industry in the industrial park can reduce the application cost of digital elements and expand the application scenarios of agricultural digitization for the upgrading of agricultural industrial structure.
After the construction of digital villages in various regions reaches a certain level, its spillover effect on agricultural development can be maximized. In areas with low levels of digital rural construction, the government should provide financial support and technical guidance to help the region break through the digital threshold and enter the stage of rapid development of green agriculture. Areas with a high level of DVC can learn from the coordinated development model of “digital village-sustainable agriculture” in foreign countries (Agusta, 2023) to encourage the deep integration of digital technology and agriculture. For example, rural areas can refer to the application experience of “intelligent agricultural management system” in other countries (Adesipo et al., 2020) and use the Internet of Things, big data and artificial intelligence to achieve precision agricultural management.
7 Conclusions and limitations
7.1 Conclusions
Based on data from prefecture-level cities in Henan Province from 2013 to 2022, this study constructs the evaluation index system of DVC and agricultural green development and uses the entropy weight topsis model to evaluate their levels. Then, the fixed effect model was used to analyze the impact of DVC on the green development of agriculture. Secondly, we use the intermediary model to examine the role of agricultural industrial structure upgrading in DVC and agricultural green development. In addition, the differences in DVC on agricultural green development among regions in Henan Province were examined. Finally, the threshold model is used to test the non-linear effect of DVC on agricultural green development. The logical framework and analysis method of the evaluation index system, driving mechanism, and policy design constructed in this study will help China's digital village empower agricultural development and provide a reference and basis for the United States, Russia, Africa, and other countries or regions.
In summary, this study draws the following conclusions. First, the level of DVC and agricultural green development in Henan Province is on the rise, and there is a significant spatial positive correlation in DVC. Second, DVC significantly impacts agricultural green development, and it is still significant after replacing the regression model and many robustness tests. Third, the impact of DVC on agricultural green development has heterogeneity in different terrains and different levels of economic development. Fourth, the upgrading of agricultural structure plays a partial intermediary role in the impact of DVC on agricultural green development. Moreover, the upgrading of agricultural structures has a significant double threshold effect on DVC and agricultural green development. With the improvement of the level of DVC, its impact on the green development of agriculture has gradually increased.
7.2 Limitations
There are still some limitations in the study. First of all, Henan Province has a representation of topography, crop planting structure, and economic development. However, there may still be a problem of sample selection bias. Meanwhile, it is more macroscopic to study the target of prefecture-level cities, and future studies will pay more attention to county data at the national level to make more targeted policy recommendations. Secondly, the research cycle is short due to the limited availability of data and the development stage. In fact, DVC is dynamic and diverse, and long-term effects will be studied in the future. In addition, the characteristics and stages of DVC in different regions will be considered in future research.
Data availability statement
The raw data supporting the conclusions of this article will be made available by the authors, without undue reservation.
Author contributions
ZL: Conceptualization, Data curation, Methodology, Software, Writing – original draft, Writing – review & editing. KH: Conceptualization, Formal analysis, Funding acquisition, Project administration, Writing – original draft, Writing – review & editing. QS: Conceptualization, Funding acquisition, Methodology, Project administration, Supervision, Writing – original draft, Writing – review & editing.
Funding
The author(s) declare financial support was received for the research, authorship, and/or publication of this article. This work was supported by the Jiangxi Management Science Decision Consulting Project (20244BAA10026); the Henan Province Philosophy and Social Science Planning Youth Project (Grant No. 2023CJJ181); the Key projects of social science planning in Jiangxi Province (Grant No. 22SQ06); the Jiangxi University Humanities and Social Science Research Project (Grant No. GL23207); and the Jiangxi Provincial Department of Education Science and Technology Research Project (Grant No. GJJ2200426).
Conflict of interest
The authors declare that the research was conducted in the absence of any commercial or financial relationships that could be construed as a potential conflict of interest.
Generative AI statement
The author(s) declare that no Gen AI was used in the creation of this manuscript.
Publisher's note
All claims expressed in this article are solely those of the authors and do not necessarily represent those of their affiliated organizations, or those of the publisher, the editors and the reviewers. Any product that may be evaluated in this article, or claim that may be made by its manufacturer, is not guaranteed or endorsed by the publisher.
References
Abiri, R., Rizan, N., Balasundram, S. K., Shahbazi, A. B., and Abdul-Hamid, H. (2023). Application of digital technologies for ensuring agricultural productivity. Heliyon 9:e22601. doi: 10.1016/j.heliyon.2023.e22601
Adesipo, A., Fadeyi, O., Kuca, K., Krejcar, O., Maresova, P., Selamat, A., et al. (2020). Smart and climate-smart agricultural trends as core aspects of smart village functions. Sensors 20:5977. doi: 10.3390/s20215977
Agusta, Y. (2023). Managing the development of a sustainable digital village. Sustainability 15:7575. doi: 10.3390/su15097575
Bell, J. N. B., Power, S. A., Jarraud, N., Agrawal, M., and Davies, C. (2011). The effects of air pollution on urban ecosystems and agriculture. Int. J. Sustain. Dev. World Ecol. 18, 226–235. doi: 10.1080/13504509.2011.570803
Bhaskara, S., and Bawa, K. S. (2021). Societal digital platforms for sustainability: agriculture. Sustainability 13:5048. doi: 10.3390/su13095048
Burney, J., and Ramanathan, V. (2014). Recent climate and air pollution impacts on Indian agriculture. Proc. Nat. Acad. Sci. 111, 16319–16324. doi: 10.1073/pnas.1317275111
Cai, B., Shi, F., Huang, Y., and Abatechanie, M. (2022). The impact of agricultural socialized services to promote the farmland scale management behavior of smallholder farmers: empirical evidence from the rice-growing region of southern China. Sustainability 14:316. doi: 10.3390/su14010316
Cai, Q., and Han, X. (2024). Impacts and mechanisms of digital village construction on agricultural green total factor productivity. Front. Sustain. Food Syst. 8:1431294. doi: 10.3389/fsufs.2024.1431294
Calzada, I. (2023). Smart rural communities: action research in colombia and mozambique. Sustainability 15:9521. doi: 10.3390/su15129521
Cao, L., Niu, H., and Wang, Y. (2022). Utility analysis of digital villages to empower balanced urban-rural development based on the three-stage DEA-Malmquist model. PLoS ONE 17:e0270952. doi: 10.1371/journal.pone.0270952
Chen, Y., Hu, S., and Wu, H. (2023). The digital economy, green technology innovation, and agricultural green total factor productivity. Agriculture 13:1961. doi: 10.3390/agriculture13101961
Deichmann, U., Goyal, A., and Mishra, D. (2016). Will digital technologies transform agriculture in developing countries? Agric. Econ. 47, 21–33. doi: 10.1111/agec.12300
Dumitru, E. A., Ursu, A., Tudor, V. C., and Micu, M. M. (2021). Sustainable development of the rural areas from romania: development of a digital tool to generate adapted solutions at local level. Sustainability 13:11921. doi: 10.3390/su132111921
Fu, W., and Zhang, R. (2022). Can digitalization levels affect agricultural total factor productivity? Evidence from China. Front. Sustain. Food Syst. 6:860780. doi: 10.3389/fsufs.2022.860780
Hong, M., Tian, M., and Wang, J. (2022). Digital inclusive finance, agricultural industrial structure optimization and agricultural green total factor productivity. Sustainability 14:11450. doi: 10.3390/su141811450
Hong, M., Tian, M., and Wang, J. (2023). The impact of digital economy on green development of agriculture and its spatial spillover effect. China Agric. Econ. Rev. 15, 708–726. doi: 10.1108/CAER-01-2023-0004
Hua, J., Yu, J., Song, Y., Xue, Q., and Zhou, Y. (2024). The enabling effect of digital economy on high-quality agricultural development-evidence from China. Sustainability 16:3859. doi: 10.3390/su16093859
Jalal Kazemi, M., and Samouei, P. (2024). A new bi-level mathematical model for government-farmer interaction regarding food security and environmental damages of pesticides and fertilizers: case study of rice supply chain in Iran. Comput. Electr. Agric. 219:108771. doi: 10.1016/j.compag.2024.108771
Jin, M., Feng, Y., Wang, S., Chen, N., and Cao, F. (2024). Can the development of the rural digital economy reduce agricultural carbon emissions? A spatiotemporal empirical study based on China's provinces. Sci. Total Environ. 939:173437. doi: 10.1016/j.scitotenv.2024.173437
Klingenberg, C. O., Valle Antunes Júnior, J. A., and Müller-Seitz, G. (2022). Impacts of digitalization on value creation and capture: evidence from the agricultural value chain. Agric. Syst. 201:103468. doi: 10.1016/j.agsy.2022.103468
Knippenberg, E., Jolliffe, D., and Hoddinott, J. (2020). Land fragmentation and food insecurity in ethiopia. Am. J. Agric. Econ. 102, 1557–1577. doi: 10.1002/ajae.12081
Leong, C., Pan, S. L., Newell, S., and Cui, L. (2016). The emergence of self-organizing E-commerce ecosystems in remote villages of China: a tale of digital empowerment for rural development. MIS Q. 40, 475–484. doi: 10.25300/MISQ/2016/40.2.11
Li, G., Jia, X., Khan, A. A., Khan, S. U., Ali, M. A. S., and Luo, J. (2023). Does green finance promote agricultural green total factor productivity? Considering green credit, green investment, green securities, and carbon finance in China. Environ. Sci. Pollut. Res. 30, 36663–36679. doi: 10.1007/s11356-022-24857-x
Li, H., Zhang, W., Xiao, X., Lun, F., Sun, Y., and Sun, N. (2024). Temporal and spatial changes of agriculture green development in beijing's ecological conservation developing areas from 2006 to 2016. Sustainability 16:219. doi: 10.3390/su16010219
Li, W., Zhang, P., Zhao, K., Chen, H., and Zhao, S. (2023). The evolution model of and factors influencing digital villages: evidence from guangxi, China. Agriculture 13:659. doi: 10.3390/agriculture13030659
Lin, Y., and Li, C. (2023). Towards sustainable development: research on the green growth effect of digital agriculture in China. Environ. Sci. Pollut. Res. doi: 10.1007/s11356-023-28367-2
Liu, C., Chen, L., Li, Z., and Wu, D. (2023). The impact of digital financial inclusion and urbanization on agricultural mechanization: Evidence from counties of China. PLoS ONE 18:e0293910. doi: 10.1371/journal.pone.0293910
Liu, E. K., He, W. Q., and Yan, C. R. (2014). ‘white revolution' to ‘white pollution'—Agricultural plastic film mulch in China. Environ. Res. Lett. 9:091001. doi: 10.1088/1748-9326/9/9/091001
Liu, J., Feng, X., Liu, J., and Yamaka, W. (2024). Digital economy and industrial structure transformation: mechanisms for high-quality development in China's agriculture and rural areas. Agriculture 14:1769. doi: 10.3390/agriculture14101769
Liu, L., and Liu, K. (2023). Can digital technology promote sustainable agriculture? Empirical evidence from urban China. Cogent Food Agric. 9:2282234. doi: 10.1080/23311932.2023.2282234
Liu, M., and Liu, H. (2024). The influence and mechanism of digital village construction on the urban–rural income gap under the goal of common prosperity. Agriculture 14:775. doi: 10.3390/agriculture14050775
Liu, R., Xie, X., Hou, Q., Han, D., Song, J., and Huang, G. (2024). Spatial distribution, sources, and human health risk assessment of elevated nitrate levels in groundwater of an agriculture-dominant coastal area in hainan island, China. J. Hydrol. 634:131088. doi: 10.1016/j.jhydrol.2024.131088
Liu, Y., Lu, C., and Chen, X. (2023). Dynamic analysis of agricultural green development efficiency in China: spatiotemporal evolution and influencing factors. J. Arid Land 15, 127–144. doi: 10.1007/s40333-023-0007-6
Liu, Z., Yang, D., and Wen, T. (2018). Agricultural production mode transformation and production efficiency: a labor division and cooperation lens. China Agric. Econ. Rev. 11, 160–179. doi: 10.1108/CAER-07-2017-0129
Lu, S., Sun, Z., and Huang, M. (2024a). The impact of digital literacy on farmers' pro-environmental behavior: an analysis with the Theory of Planned Behavior. Front. Sustain. Food Syst. 8:1432184. doi: 10.3389/fsufs.2024.1432184
Lu, S., Zhuang, J., Sun, Z., and Huang, M. (2024b). How can rural digitalization improve agricultural green total factor productivity: empirical evidence from counties in China. Heliyon 10:e35296. doi: 10.1016/j.heliyon.2024.e35296
Lu, Y., Meng, Y., Chen, L., and Zhang, Y. (2024). Can the digital economy contribute to rural revitalization? A case of from China? PLoS ONE 19:e0310313. doi: 10.1371/journal.pone.0310313
Luo, Q., Wang, Y., and Chen, B. (2024). Empirical study on the impact of the digital economy on the efficiency of agricultural product circulation under the dual carbon goals. Environ. Res. Commun. 6:085010. doi: 10.1088/2515-7620/ad6824
Mei, Y., Miao, J., and Lu, Y. (2022). Digital villages construction accelerates high-quality economic development in rural china through promoting digital entrepreneurship. Sustainability 14:14224. doi: 10.3390/su142114224
Misra, N. N., Dixit, Y., Al-Mallahi, A., Bhullar, M. S., Upadhyay, R., and Martynenko, A. (2022). IoT, big data, and artificial intelligence in agriculture and food industry. IEEE Internet Things J. 9, 6305–6324. doi: 10.1109/JIOT.2020.2998584
Mushi, G. E., Burgi, P.-Y., and Di Marzo Serugendo, G. (2024). State of agricultural e-government services to farmers in Tanzania: toward the participatory design of a farmers digital information system (FDIS). Agriculture 14:475. doi: 10.3390/agriculture14030475
Ren, J., Chen, X., Shi, L., Liu, P., and Tan, Z. (2024). Digital village construction: a multi-level governance approach to enhance agroecological efficiency. Agriculture 14:478. doi: 10.3390/agriculture14030478
Schmidt, H. (2011). Digital'nye derevenščiki/digital villagers: Russian online projects from the countryside. Stud. East Eur. Thought 63, 95–109. doi: 10.1007/s11212-011-9137-z
Song, Y., Qi, G., Zhang, Y., and Vernooy, R. (2014). Farmer cooperatives in China: diverse pathways to sustainable rural development. Int. J. Agric. Sustain. 12, 95–108. doi: 10.1080/14735903.2013.858443
Tang, Y., and Chen, M. (2022). The impact mechanism and spillover effect of digital rural construction on the efficiency of green transformation for cultivated land use in China. Int. J. Environ. Res. Public Health 19:16159. doi: 10.3390/ijerph192316159
Tian, J., Liu, C., and Ma, G. (2024). The role of the digital economy on the coordinated development of green agriculture and food security: evidence from China. Front. Sustain. Food Syst. 8:1446410. doi: 10.3389/fsufs.2024.1446410
Uzamurera, A. G., Wang, P.-Y., Zhao, Z.-Y., Tao, X.-P., Zhou, R., Wang, W.-Y., et al. (2023). Thickness-dependent release of microplastics and phthalic acid esters from polythene and biodegradable residual films in agricultural soils and its related productivity effects. J. Hazard. Mater. 448:130897. doi: 10.1016/j.jhazmat.2023.130897
Vamuloh, V. V., Kozak, R. A., and Panwar, R. (2020). Voices unheard: barriers to and opportunities for small farmers' participation in oil palm contract farming. J. Clean. Prod. 275:121955. doi: 10.1016/j.jclepro.2020.121955
Wang, F., Wang, H., and Xiong, L. (2024). Does the digital economy exhibit multiplier effects? A case study on the optimization of agricultural production structure in rural digital economy. Int. J. Agric. Sustain. 22:2386821. doi: 10.1080/14735903.2024.2386821
Wang, H., and Tang, Y. (2023). Spatiotemporal distribution and influencing factors of coupling coordination between digital village and green and high-quality agricultural development—evidence from China. Sustainability 15:8079. doi: 10.3390/su15108079
Wang, L., Tang, J., Tang, M., Su, M., and Guo, L. (2022). Scale of operation, financial support, and agricultural green total factor productivity: evidence from China. Int. J. Environ. Res. Public Health 19:9043. doi: 10.3390/ijerph19159043
Wang, P., Li, C., and Huang, C. (2023). The impact of digital village construction on county-level economic growth and its driving mechanisms: evidence from China. Agriculture 13:1917. doi: 10.3390/agriculture13101917
Wang, R. Y., and Jayakar, K. (2024). Learning from the neighbors: the diffusion of state broadband policies in the united states. Telecomm. Policy 48:102809. doi: 10.1016/j.telpol.2024.102809
Wang, S. (2022). The positive effect of green agriculture development on environmental optimization: measurement and impact mechanism. Front. Environ. Sci. 10:1035867. doi: 10.3389/fenvs.2022.1035867
Wu, X., Xie, M., Xu, S., Fei, R., and Pan, A. (2024). Does agricultural fiscal policy improve green development in China's agriculture sector? Evidence from energy and environmental perspectives. Environ. Dev. Sustain. doi: 10.1007/s10668-024-04770-8
Wu, Y., Du, H., Wei, X., and Li, H. (2024). Big data development and agricultural carbon emissions: Exacerbation or suppression? A quasi-natural experiment based on the establishment of the national big data comprehensive pilot zone. J. Environ. Manage. 368:122178. doi: 10.1016/j.jenvman.2024.122178
Xie, L., Qiu, Z., Chen, S., and Lei, X. (2024). Smartphone access, digital economy, and pesticide use intensity: evidence from China. Sci. Total Environ. 943:173867. doi: 10.1016/j.scitotenv.2024.173867
Xu, J. (2024). Spatial spillover and threshold effects of digital rural development on agricultural circular economy growth. Front. Sustain. Food Syst. 8:1337637. doi: 10.3389/fsufs.2024.1337637
Yan, M., and Cao, X. (2024). Digital economy development, rural land certification, and rural industrial integration. Sustainability 16:4640. doi: 10.3390/su16114640
Yang, C., Ji, X., Cheng, C., Liao, S., Obuobi, B., and Zhang, Y. (2024). Digital economy empowers sustainable agriculture: Implications for farmers' adoption of ecological agricultural technologies. Ecol. Indic. 159:111723. doi: 10.1016/j.ecolind.2024.111723
Yu, H., Bai, X., and Zhang, H. (2022). Strengthen or weaken? Research on the influence of internet use on agricultural green production efficiency. Front. Environ. Sci. 10:1018540. doi: 10.3389/fenvs.2022.1018540
Zhang, F., Wang, F., Hao, R., and Wu, L. (2022). Agricultural science and technology innovation, spatial spillover and agricultural green development—taking 30 provinces in China as the research object. Appl. Sci. 12:845. doi: 10.3390/app12020845
Zhang, H., Zhang, J., and Song, J. (2022). Analysis of the threshold effect of agricultural industrial agglomeration and industrial structure upgrading on sustainable agricultural development in China. J. Clean. Prod. 341:130818. doi: 10.1016/j.jclepro.2022.130818
Zhang, M., Zhang, Y., Weng, Z., and Chen, Z. (2022). Design and service effect evaluation of agricultural social service platform based on 5G and cloud computing. Wireless Commun. Mobile Comput. 2022:4949242. doi: 10.1155/2022/4949242
Zhang, Q., Yang, Y., Li, X., and Wang, P. (2024). Digitalization and agricultural green total factor productivity: evidence from China. Agriculture 14:1805. doi: 10.3390/agriculture14101805
Zhang, Z., Sun, C., and Wang, J. (2023). How can the digital economy promote the integration of rural industries—taking China as an example. Agriculture 13:2023. doi: 10.3390/agriculture13102023
Zhao, W., Liang, Z., and Li, B. (2022). Realizing a rural sustainable development through a digital village construction: experiences from China. Sustainability 14:14199. doi: 10.3390/su142114199
Keywords: digital village construction, green development of agriculture, industrial structure upgrading, threshold effect model, digital economy
Citation: Li Z, Hu K and Shi Q (2025) The influence of digital village construction on agricultural green development-based on the mediate role of industrial structure upgrading. Front. Sustain. Food Syst. 8:1538845. doi: 10.3389/fsufs.2024.1538845
Received: 03 December 2024; Accepted: 31 December 2024;
Published: 17 January 2025.
Edited by:
Chiedza Tsvakirai, University of South Africa, South AfricaReviewed by:
Jielun Yang, Northwest A&F University, ChinaWeiwei Li, Guangxi Agricultural Vocational and Technology University, China
Aoxiang Zhang, Xiamen University, China
Copyright © 2025 Li, Hu and Shi. This is an open-access article distributed under the terms of the Creative Commons Attribution License (CC BY). The use, distribution or reproduction in other forums is permitted, provided the original author(s) and the copyright owner(s) are credited and that the original publication in this journal is cited, in accordance with accepted academic practice. No use, distribution or reproduction is permitted which does not comply with these terms.
*Correspondence: Kai Hu, Y2FybC1odUAxNjMuY29t