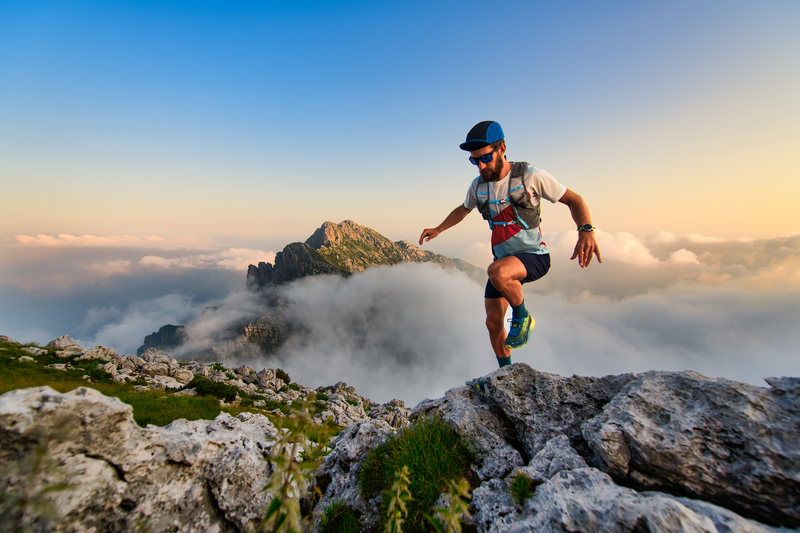
94% of researchers rate our articles as excellent or good
Learn more about the work of our research integrity team to safeguard the quality of each article we publish.
Find out more
SYSTEMATIC REVIEW article
Front. Sustain. Food Syst. , 06 January 2025
Sec. Agricultural and Food Economics
Volume 8 - 2024 | https://doi.org/10.3389/fsufs.2024.1514546
This article is part of the Research Topic Harnessing Digital Innovation for Sustainable Agricultural Development View all 9 articles
Mobile phone-mediated scaling of agricultural technologies (MPSATs) attracts attention as an effective approach for promoting agricultural development and sustainability. Despite the growing interest, a comprehensive understanding of drivers of MPSAT at the farm level and the evidence base of their impacts remains limited. To fill this gap, we conducted a systematic literature review and meta-analysis of 18 relevant empirical studies covering 10,757 farmers across 12 countries. Meta-analyses reveal that farmers’ innovativeness and full-time farming increase the odds of adopting agricultural technologies. Age, gender, digital skills, mobile phone device ownership, and membership in farmer groups also influence MPSAT but display heterogeneity. Moderation analysis reveals that the development status of countries plays a moderating role in variables such as asset ownership and farm size. Moreover, the results show that using mobile phones as a standalone method increases the odds of adopting agricultural technologies by 2%. In combination with traditional extension methods, this figure rises significantly to 17%. Additionally, MPSAT increases yields by 2%, and profits by 5%, and contributes to a 3% improvement in farmers’ learning outcomes. This study sheds light on the potential and multifaceted nature of MPSAT, providing insights for policymakers and practitioners promoting sustainable agriculture through digital technologies.
Digital agriculture is considered as a crucial component of sustainable agriculture and food systems transformation in a changing climate (Fabregas et al., 2019; Basso and Antle, 2020; Herrero et al., 2021; MacPherson et al., 2022; Rosenstock et al., 2024). In recent years, among digital tools, mobile phones have rapidly penetrated the developing world and emerged as powerful tools with the potential to revolutionize various sectors, including agriculture (Fabregas et al., 2019; Tsan et al., 2021). According to the International Telecommunication Union (ITU, 2020), nearly three out of four people aged 10 and above had a mobile phone in 2022. This widespread adoption of mobile phones brings promising prospects for digital development (Fabregas et al., 2019; Tsan et al., 2021). They have the potential to reduce information asymmetry and can play a role in facilitating technology adoption (Mittal and Hariharan, 2018; Yegbemey and Egah, 2021; Gouroubera et al., 2024). It enhances productivity, improves access to information, and fosters sustainable practices within the agricultural landscape (Fabregas et al., 2019; Quandt et al., 2020; Aparo et al., 2022).
The challenges facing agricultural extension services, particularly in developing countries, have created a strong case for using mobile phones as a transformative solution (Aker et al., 2016; Wyche and Steinfield, 2016; Klerkx et al., 2019; Cole and Fernando, 2021). Mobile phones offer a scalable, cost-effective means to overcome reach limitations and provide real-time, tailored support to farmers (Wyche and Steinfield, 2016; Cole and Fernando, 2021). Digitalizing extension services enhances farmers’ technical proficiency, tackles socioeconomic challenges, improves food traceability, and mitigates environmental impact (Balafoutis et al., 2017; Klerkx et al., 2019; MacPherson et al., 2022; Abdulai et al., 2023; Amoussohoui et al., 2024). Mobile phones can mediate agricultural technologies between farmers and agricultural advisory services. The emergence of digital extension technologies provides a unique opportunity to enhance agricultural output, strengthen agricultural value chains, and contribute to food security (Gow et al., 2020; Gouroubera et al., 2024). In light of their transformative potentials, there has been a growing interest in understanding how mobile phones can facilitate the scaling of agricultural technologies (MPSATs). Several studies on MPSATs highlighted the critical role of mobile phones in facilitating knowledge and information sharing and collaboration among farmers, thus fostering social connections and a sense of connectedness among farmers (Butt, 2015; van Baardewijk, 2017). Mobile phone technologies are valuable tools for overcoming barriers associated with traditional extension methods (Klerkx et al., 2019). While the potential benefits of mobile phones in enhancing social relationships are evident, understanding of how and to what extent they can facilitate or enable widespread adoption of agricultural technologies is limited and MPSATs are low. Identifying factors influencing this limited adoption is crucial for developing strategies to overcome barriers and fully leverage the benefits of MPSATs. The existing literature on factors influencing MPSATs is growing (Asif et al., 2017; Michels et al., 2020; Krell et al., 2021). While these studies provide valuable insights into diverse drivers, including gender, age, income, education, etc., they tend to be predominantly exploratory and conducted at the national to sub-national level, especially in developing countries, lacking robust empirical establishment.
Another crucial area of discussion is the impacts of MPSATs on farmers. While there is promising evidence regarding its impacts on market integration (Jensen, 2007; Aker, 2011), education (Aker et al., 2012), and access to finance (Karlan and Morduch, 2010; Jack et al., 2013), there is a notable gap in evidence of agricultural outcomes. Existing evidence is sparse and insufficient to allow an understanding of the impact of MPSAT on various farm-level outcomes. Effective MPSAT necessitates proving its impacts on farmers and identifying the influencing factors.
In this paper, we conducted a systematic literature review and a meta-analysis on MPSATs with three main objectives: (1) to synthesize the effect sizes of factors influencing MPSATs, (2) to assess the impacts of mobile phones as a standalone agricultural extension approach in comparison to mobile phones combined with traditional methods like farmer-to-farmer interactions, Farmers Field Schools, and demonstrations, and (3) to determine the effects of the technologies delivered via mobile phones on farmers’ yields, profit, and learning outcomes, including knowledge, skills, and understanding.
In this study, we used a meta-analytic approach, consisting of a systematic literature search followed by a meta-analysis to quantify the effect size of MPSAT determinants and their impacts. We adhered to the Preferred Reporting Items for Systematic Review and Meta-Analysis (PRISMA) Protocols (Moher et al., 2009) for a rigorous aggregation and synthesis of findings. This approach provides a more robust foundation for evidence-based decision-making.
We conducted a systematic literature search in Scopus and Web of Science, two academic databases commonly used for systematic review because of their ability to provide easy access to complex search terms and their extensive coverage both in terms of discipline and quality of publications (Totin et al., 2018; Segnon et al., 2024). The literature search covered publications available in the databases at the time of the search. Building on previous systematic reviews and protocols (Rosenstock et al., 2016; Totin et al., 2018; Arslan et al., 2022), we developed keywords and search terms to identify literature related to agricultural technologies (associated with the following themes: crop production, agroforestry, and livestock production) and MPSAT drivers and their impacts (See Supplementary material 1). These keywords and search terms were combined using Boolean operators and searched in Title, Abstract and Keywords. The publications resulting from the search were screened for their relevance using prior-defined inclusion and exclusion criteria (Table 1).
The search in Web of Science and Scopus resulted in 1005 publications, which were exported into the CADIMA platform (Kohl et al., 2018) for screening. After removing duplicate publications (45), the remaining 960 publications underwent a two-stage screening process. During the first stage, titles and abstracts were assessed against the inclusion criteria (Table 1), excluding 835 papers. The full-text screening of the remaining 125 publications resulted in 16 publications that met the inclusion criteria. Two co-authors performed the screening process with a Cohen’s kappa coefficient of 0.81. A backward reference search on Google Scholar yielded two more papers meeting the criteria. The final meta-database (Figure 1) of 18 relevant publications is presented in Supplementary material 2.
To ensure consistency and uniformity in the extraction process, we used a structured data extraction guide with predefined codes to extract data from the relevant publications (Stanley et al., 2013). Data extracted included study locations, sample sizes, agricultural technologies, econometric specifications, adoption determinants, results of randomized control data on adoption, yields, profit and learning outcomes, regression coefficients, and the significance levels of these coefficients. Two co-authors conducted the coding to ensure precision and consistency in the coded content. Intercoder reliability was assessed by cross-checking the data among the coders.
We used a comprehensive assessment framework to assess the quality of the publications included in the study. Building on the modified Newcastle-Ottawa Scale for nonrandomized studies (Stang, 2010) and the Cochrane Risk of Bias tool for randomized controlled trials (Higgins and Green, 2008), we established nine quality assessment criteria, including representativeness, interventions, agricultural technologies, control for factors, adoption measurement, statistical analysis, reporting quality, bias assessment, and external validity (See Supplementary material 3). We classified publications as high-quality (score above nine), as moderate (score between nine and seven), and those below 7 as low contributions. These criteria encompassed essential dimensions including. We ensured that only studies meeting quality standards were integrated into our meta-analysis, reinforcing the reliability and credibility of our findings. Supplementary material 4 presents the quality assessment outcome.
To quantify the effect sizes of factors influencing the adoption of MPSATs, we used the random effects model to account for the variability in study characteristics (Brockwell and Gordon, 2001). We used the R package Metafor (Viechtbauer, 2010) to perform the analysis. The effect sizes (Equation 1) were calculated based on the following equation (Veroniki et al., 2016):
where represents the observed effect in study , is the common true effect, follows a normal distribution with mean 0 and variance , and follows a normal distribution with mean 0 and variance , where is the between-study variance. The observed variation is a combination of within-study variance and between-study variance . The mean effect in the random-effects model (Equation 2) was computed following Borenstein et al. (2009):
where , is the weight related to study , and is the effect size from study . It is important to note that the presence of between-study variance results in lower weights for study and wider 95% confidence intervals (CI) (Borenstein et al., 2009).
We estimated the effect sizes of the impact of MPSAT adoption on various outcomes, including adoption rates, yields, profit, and farmers’ capacity building, using odds ratios (OR), the ratio of the odds of following recommendations in the treatment group to the odds in the control group (Fabregas et al., 2019). In this context, “odds” represent the likelihood of adopting technologies or following advice, leading to increased yields, profit, or learning outcomes, compared to those of not adopting technologies or following advice. The analysis employs weights derived from a random effects model, accounting for variability across studies in the meta-analysis.
To assess the heterogeneity of true effect sizes among studies, we used the p-value associated with the test of heterogeneity (Cochrane’s Q) and I2 values. Studies are heterogeneous when the p < α (α = 0.05). I2 represents the proportion of true variance, and is employed for the homogeneity test, which is independent of the number of studies or effect size (Borenstein et al., 2009). Following Higgins and Green (2008), I2 values were categorized as low (< 25%), moderate (25% ≤ I2 < 50%), high (50% ≤ I2 < 75%), or considerably high (I2 ≥ 75%). An I2 value close to zero indicates no heterogeneity between effect sizes (Gouroubera et al., 2023).
We performed a moderation analysis to address heterogeneity (Melsen et al., 2014), using country, continent, or the development status of the countries as a moderator to explore its impact on effect sizes. This approach allows us to understand how contextual variations specific to different geographical locations might influence the effectiveness of interventions. The moderation analysis enhances the robustness of our findings, providing insights into potential sources of heterogeneity for a more tailored interpretation of results.
To evaluate publication bias, we employed Rosenthal’s test (Pittelkow et al., 2015; Benítez-López et al., 2017). This test estimates the number of missing studies needed to negate the summary effect size. Additionally, we used funnel plots to assess potential publication bias. Less accurate estimates tend to cluster at the bottom of the graph, while more precise estimates are positioned toward the top of the funnel, aiding in identifying potential bias in the literature.
The meta-analysis encompasses diverse studies focused on understanding the impacts and factors influencing the MPSATs. Table 2 presents the characteristics of the 18 included studies in this meta-analysis. Of the 18 papers, 10 focused on MPSAT determinants, and 11 focused on MPSAT impacts.
The included papers were published from 2014 to 2023, with the majority (83.33%, n = 15) between 2019 and 2023. The studies were conducted in Asia (n = 8), Africa (n = 7), and Europe (n = 3) (Figure 2). Twelve studies focused on maize (n = 2), wheat (n = 2), cotton (n = 1), cowpea (n = 1), tomato (n = 1), sugarcane (n = 1), Yardlong bean (n = 1), litchi (n = 1) and rice (n = 1) production system. Six studies did not indicate the crops. The agricultural technologies delivered via mobile phones include crop management technologies (integrated pest and weed control, drought-tolerant variety, farm input, agricultural good practices, postharvest technologies, and nutrient management); livestock management technologies (herd management and data collection, animal healthcare, and animal feeding); soil and fertilizer management (fertilizer management practices, soil testing, and precision fertilization technology); information and decision support (market information, alerts on agricultural or livestock activities, livestock information, information related to seed, fertilizer, weather); specific technologies and practices (e.g., Bt cotton seed, use of pesticide, fertilizer).
Table 3 presents the synthesis of the drivers of MPSAT adoption and summarizes the overall effect size of the drivers, categorized into five groups comprising 15 variables: demographic and socioeconomic factors (six variables); digital-related factors (two variables); farm characteristics (three variables); risk and attitude (two variables); and information-related variables (two variables).
Farmers’ age, gender, and education level emerged as the most frequently examined factors (appearing in 10 studies). The effect sizes of age, gender (male), and full-time farming were statistically significant. Being an older farmer decreases the likelihood of MPSAT adoption by 0.0365 times. Male farmers are more likely to adopt technologies 0.103 times more than female farmers. Additionally, being a full-time farmer increases the chance of adopting MPSATs by 0.0197 times.
The effect sizes of digital skills and possession of IoT devices were statistically significant. Farmers with digital skills and IoT device possession were 1.15 and 0.497 times more likely to adopt MPSATs, respectively. Both the effect sizes of membership in farmer groups and innovativeness were statistically significant. Membership in farmer groups increases the chance of adoption by 0.321 times, while farmers with higher innovativeness were 0.299 times more likely to adopt MPSATs. However, none of the variables related to farm characteristics and information-related variables were statistically significant.
Cochran’s Q and the I2 index show that most of the variables (10 out of 15) had a significant Cochran’s Q at 1%. The I2 index of these variables was >74%, indicating significant heterogeneity. For example, even though the effect size of age was highly significant, it had a significant Cochran’s Q of 52.335 and an I2 index of 93.88%.
To address the heterogeneity among the studies, we conducted a moderation analysis using the development status of the countries as moderators (Table 4). The studies were grouped into developed countries and emerging economies (China, Germany, Switzerland), and developing countries (Kenya, Zambia, Burkina Faso, Pakistan). Only the effect sizes of two variables, namely assets ownership and farm size were statistically significant. In developed and emerging countries, asset ownership increases the adoption of MPSATs by 0.801 times. However, farm size has the opposite impact, with larger farms decreasing the likelihood of MPSATs adoption by 0.00921 times.
The aggregated findings reveal a range of positive impacts attributed to the MPSATs (Figures 3A,B). Adoption of agricultural technologies by farmers increases by ~2% when disseminated or delivered through mobile phones as a standalone approach (OR = 0.02 [95% CI: 0.01–0.02], p = 0.001). There was no evidence of heterogeneity within studies using mobile phones as a standalone approach (I2 = 3.37%, and Cochran’s Q test was not significant). Combining mobile phones with traditional extension approaches such as demonstrations, field visits, training, and video projections increases the adoption of agricultural technologies by 17% (OR = 0.17 [95% CI: 0.10–0.25], p = 0.001). The test for heterogeneity indicated a high level of heterogeneity within studies combining mobile phones with other approaches (I2 = 93.01%, and Cochran’s Q test was significant at 1%). The moderation analysis with geographical regions as a moderator was unsuccessful in reducing the presence of heterogeneity (I2 = 93.9% for country as a moderator, I2 = 93.88%, Cochran’s Q tests significant at 1% for both analyses).
Figure 3. Impact of MPSATs on technology adoption. (A) Impact of MPSAT as a standalone approach; (B) impacts of MPSAT in combination with traditional extension approaches.
Figure 4 reports a meta-analysis examining the impact of MPSATs on farm yields and profits (Figures 4A,B, respectively). This analysis integrates multiple studies, including the research conducted by Zheng and Ma (2023) in China, which investigated the effects of smartphone-based delivery of drought-tolerant wheat varieties on farmers’ yields and profits. Additionally, the study by Casaburi et al. (2019) contributed data on the impact of SMS-based content (covering fertilizer management practices, weeding, and farm management practices) on sugarcane yields in Kenya. Furthermore, the study by Cole and Fernando (2021) in India explored the impacts of phone-based voice messages and SMS-based content (focusing on improved cotton seed, pest management, and fertilizer information) on cotton yield and profit. Lastly, the research conducted in Nigeria by Arouna et al. (2021) investigated the impact of mobile phone apps on rice nutrient management and its effects on rice yield and profit. The analyses indicate that MPSATs result in an approximate increase of 2% in yields and 5% in profits.
Figure 4. Meta-analysis of impacts of MPSAT on (A) crop yields, (B) farming profit, and (C) farmers’ learning outcome.
Moreover, three studies (Figure 4C) experimentally investigated the effect of mobile phones on farmers’ learning outcomes. Among these, Giulivi et al. (2023) conducted a study in Nepal, revealing MPSATs impact on farmers’ agronomic literacy. Similarly, the research by Mwalupaso et al. (2019) in Zambia delved into the influence of MPSATs on farmers’ technical efficiency. Additionally, two trials in Burkina Faso investigated the impacts of phone-based messages on farmers’ skills and understanding of the technologies regarding cowpea technologies (Maredia et al., 2018). The meta-analysis results indicate a 3% increase in farmers’ overall learning outcomes.
The heterogeneity test reveals the presence of heterogeneity within the studies pooled to estimate the effect size of yields (I2 = 76.91%) and those combined to estimate the effect size of capacity building (I2 = 75.05%), with both Cochran’s Q tests being significant at 1%. However, there was no heterogeneity within the studies combined to estimate the effect size of profit (I2 = 0%, and Cochran’s Q test was not significant, p = 0.499). The moderation analysis was not applicable considering the geographical area as a moderator due to the uneven distribution of the studies.
This meta-analysis reveals a concentration of studies on MPSATs in Asian (n = 8) and African (n = 7) countries. This finding aligns with Aparo et al. (2022) systematic review, indicating a predominant focus on developing countries in studies exploring MPSATs. Similar patterns have been observed in research on agricultural technology adoption more broadly (Olum et al., 2020; Tey and Brindal, 2024). This focus on Asia and Africa may be related to the increasing demand for technology scaling initiatives ensuring food security and fostering sustainable development in these regions (Pangaribowo and Gerber, 2016). Moreover, most of these studies (15 out of 18) were published between 2019 and 2023, indicating the growing interest of MPSATs in the discourse on sustainable development and food security (Mugambiwa and Tirivangasi, 2017; Aparo et al., 2022).
The meta-analyses unveil several positive impacts of MPSAT. Our findings indicate that using mobile phones as a standalone method increases the adoption of agricultural technologies by ~2%. Given the challenges of reaching farmers through conventional means, such as the scarcity of extension workers, mobile phones offer a cost-effective solution to disseminate agricultural technologies. While there is diversity in the literature on the effectiveness of mobile phones as standalone methods, this study aligns with studies supporting this approach (Maredia et al., 2018; Fabregas et al., 2019; Cole and Fernando, 2021). Notably, the study reveals that combining mobile phones with traditional extension approaches increases the adoption to ~17%. This finding supports the view that mobile phones, while effective on their own, achieve optimal impact when integrated with traditional extension methods like demonstrations and training sessions (LaRochelle and Berkes, 2003; Silvestri et al., 2021; Mwambi et al., 2023).
The observed increase in yields by 2% and profits by 5% due to MPSAT underlines the effectiveness of these technologies, supporting previous studies (Duncombe, 2016; Quandt et al., 2020; Gupta et al., 2024). These outcomes highlight the practical advantages of integrating mobile technologies into agricultural practices. Enhanced access to information and targeted use of inputs can increase agricultural productivity while minimizing adverse environmental impacts (Jack et al., 2013; Cui et al., 2018). Moreover, MPSAT can indirectly reach more users, with farmers who receive digital agricultural extension information often sharing it with their peers, creating additional positive effects (Fabregas et al., 2019; Cole and Fernando, 2021).
The finding that MPSAT results in a 3% increase in farmers’ learning outcomes aligns with existing literature on technology-enhanced learning in agriculture (Maredia et al., 2018; Wang et al., 2020). The learning outcomes are essential for promoting sustainable farming practices (Maredia et al., 2018). Using mobile phones in delivering agricultural technologies and practices appears to contribute positively to farmers’ skills and understanding, potentially leading to more informed and sustainable farming decisions. This finding underscores the educational potential of MPSAT in empowering farmers with the knowledge and capabilities needed to navigate modern agricultural challenges. Furthermore, it reinforces the argument that leveraging technology in agricultural extension services can play a pivotal role in enhancing farmers’ capacity and, by extension, contributing to the overall sustainability of agriculture (Basso and Antle, 2020; Metta et al., 2022).
The meta-analysis shows 15 factors reported in the included studies. Among these factors, seven were statistically significant and influenced MPSAT. The nuanced insights into these factors influencing MPSAT contribute to the growing body of literature.
The study reveals the influence of risk and attitude-related factors on MPSAT. In this line, farmers’ innovativeness increases technology adoption. This finding is consistent with Aparo et al. (2022), who found a positive relationship between MPSAT adoption and farmers’ innovativeness. Spurk et al. (2023) also highlighted the influence of farmers’ innovativeness in technology adoption. These findings underscore the necessity to set programs or trainings that improve farmers’ innovativeness. A way to strengthen farmers’ innovativeness is by combining extension approaches (Dosso et al., 2024). Membership in farmers’ groups also positively influences MPSAT adoption. These results are consistent with those of Gouroubera et al. (2023), who show in their meta-analysis that group membership increases digitally mediated climate information adoption by farmers. Feyisa (2020), Muema et al. (2018), and Li et al. (2023) also reported similar findings in farmers’ agricultural technologies adoption.
The findings also underscore the significant influence of demographic and socioeconomic factors on MPSAT adoption. Notably, the meta-analysis unveils that age plays a crucial role, negatively influencing MPSAT adoption. This aligns with various studies such as Arslan et al. (2022), Feyisa (2020), Mozzato et al. (2018), Xie and Huang (2021), and Li et al. (2023). The implication is that younger farmers exhibit greater openness and motivation to utilize mobile phones in their farming practices, showcasing a propensity for innovation and technological adoption (Chellappan and Sudha, 2015). Kabirigi et al. (2023) advocate for youth involvement, emphasizing strategies that facilitate smartphone access and the potential transfer of digital skills from younger to older farmers for successful agricultural digitalization. Another noteworthy sociodemographic factor influencing MPSAT is gender, revealing that male farmers are more inclined to adopt MPSATs than their female counterparts. While this finding contrasts with Gouroubera et al. (2023), Guo et al. (2020), and Tey and Brindal (2024), it aligns with numerous studies on agricultural technology adoption, including Djokoto et al. (2016), Rola-Rubzen et al. (2020), and Xie and Huang (2021). The gender disparity in adoption rates may be attributed to inequalities in access to information, physical and financial resources, and societal norms (van Dijk and van Deursen, 2014; Theis et al., 2018). Furthermore, the study reveals the positive influence of full-time farming on MPSAT, supporting the findings of Jones-Garcia and Krishna (2021). It suggests that individuals engaged in full-time farming are more likely to embrace and adopt technologies, potentially due to their deeper involvement and reliance on agricultural practices, further emphasizing the importance of occupation in shaping technology adoption patterns in agriculture.
Regarding digital-related factors, the study reveals that farmers’ digital skills and IoT device possession influence MPSAT. The positive influence of digital skills and IoT device possession on MPSAT underscores the pivotal role of technological literacy and access to devices in shaping technology adoption. Highlighting the importance of digital skills in MPSAT, scholars, including van Deursen et al. (2021) and Scheerder et al. (2017) stress the role of digital literacy in effective technology engagement. The “digital divide” concept underscores disparities arising from a lack of digital skills in agriculture, where MPSATs play a vital role. Allmann and Blank (2021) and van Deursen et al. (2021) studies reveal that digital skills empower individuals to navigate online information and effectively use digital tools. So, in the context of MPSATs, farmers with higher digital skills are better positioned to understand and implement technological solutions, enhancing adoption and improving agricultural practices. Addressing the digital skills gap is crucial for successful MPSAT integration and impact on agriculture.
IoT device possession amplifies the influence of MPSAT by providing farmers with the necessary tools to integrate technology into their agricultural practices. Possessing IoT devices facilitates real-time data collection, monitoring, and decision-making, thereby enhancing the precision and effectiveness of MPSATs. These devices create a connected and smart agricultural ecosystem, enabling farmers to harness data-driven insights for improved productivity and sustainability. This finding is consistent with those of van Deursen et al. (2021), Chen and Li (2022), and Heponiemi et al. (2023). van Dijk (2013) draws attention to the fact that digital material possession is not always sufficient to induce usage in the context of MPSAT. Farmers’ motivation is a prerequisite for adopting mobile phones, and subsequently, their usage. Moreover, it is noteworthy that the positive correlation between digital skills, IoT device possession, and MPSAT may be influenced by various contextual factors, including infrastructure, affordability, and training opportunities (van Dijk, 2013; van Deursen et al., 2021; Chen and Li, 2022; Heponiemi et al., 2023). Addressing potential barriers and ensuring equitable access to digital resources is crucial in promoting widespread adoption among farmers.
The analysis indicates that the development status of countries plays a significant moderating role in MPSAT for the variables “Assets Ownership” and “Farm Size.” The finding indicated that in developed and emerging countries, there is a higher likelihood of adopting MPSATs when farmers own assets underscoring the importance of economic resources and ownership in driving MPSAT in more economically advanced settings. Several systematic reviews of technology adoption conducted in developing countries support the idea that socioeconomic factors are significant determinants (Olum et al., 2020; Aparo et al., 2022; Arslan et al., 2022; Li et al., 2023). These reviews emphasized the importance of socioeconomic factors, including income and education, as key influencers in technology adoption. However, Gouroubera et al. (2023) study showed the opposite result, emphasizing that information-related factors are crucial for digitally mediated technology adoption. Conversely, in developed and emerging countries, the effect size suggests a slight decrease in the likelihood of MPSAT adoption with an increase in farm size. It may be due to complexity or specific challenges associated with larger farms. In developing countries, the negative coefficient implies a different dynamic, potentially influenced by land distribution and resource constraints. These findings highlight the nuanced relationship between development status and MPSAT adoption, emphasizing the need for tailored strategies based on economic contexts. Policymakers and practitioners should consider these moderating effects when designing interventions to enhance MPSAT in diverse agricultural systems.
The MPSAT meta-analysis provides an in-depth examination of the current landscape. By examining multiple facets of MPSAT impacts, including adoption rates, yield and profit increases, and improvements in farmers’ learning outcomes, the study provides nuanced insights into digital agriculture. Conducting a moderation analysis based on countries’ development status adds depth to the results and highlights the contextual nuances influencing MPSAT. However, limitations, such as the small evidence base, geographical region gap, identified heterogeneity between studies, and a temporal concentration of publications (2019–2023), require careful consideration. These limitations highlight the need for cautious interpretation and suggest avenues for future research to fill gaps and improve the generalizability of findings. Overall, the meta-analysis contributes valuable knowledge to the discourse on the transformative potential of MPSATs in agriculture and emphasizes the importance of context-specific strategies for adopting sustainable technologies.
This meta-analysis provides valuable insights into the MPSATs, shedding light on their multifaceted impacts and influencing factors. The findings indicate that while using mobile phones as a standalone method increases the odds of adopting agricultural technologies, combining it with traditional extension approaches yielded significantly higher outcomes. Specifically, the findings reveal that such synergistic approaches lead to marked improvements in agricultural yields, profitability, and the overall learning experiences of farmers. This highlights the potential of MPSATs as a transformative tool in promoting sustainable agricultural development. Moreover, the study identifies key drivers of MPSATs, including farmers’ innovativeness, full-time engagement in farming, age, IoT device ownership, digital literacy, and membership in farmer groups. Understanding these factors is crucial for designing targeted interventions that can enhance the adoption and efficacy of MPSATs, particularly in diverse economic contexts. The nuanced relationship between development status and MPSATs adoption underscores the importance of tailoring interventions to local economic and cultural contexts. Additionally, the geographical focus on Asian and African countries may limit the generalizability of findings to other regions.
This study advances our understanding of the role of mobile phones in transforming agriculture and food systems, offering policymakers, researchers, and practitioners’ actionable information to promote sustainable farming practices through technology integration. Future studies should aim to broaden the geographical scope and deepen the investigation into the contextual factors that influence MPSAT adoption and effectiveness. By doing so, stakeholders can better harness the potential of mobile technology to foster sustainable agricultural practices and improve the livelihoods of farmers worldwide.
The original contributions presented in the study are included in the article/Supplementary material, further inquiries can be directed to the corresponding author.
MG: Conceptualization, Data curation, Formal analysis, Investigation, Methodology, Validation, Visualization, Writing – original draft, Writing – review & editing. SA: Conceptualization, Data curation, Methodology, Writing – original draft. AS: Conceptualization, Methodology, Validation, Formal analysis, Writing – review & editing. IM-M: Validation, Supervision, Writing – review & editing. RZ: Funding acquisition, Validation, Supervision, Writing – review & editing.
The author(s) declare that financial support was received for the research, authorship, and/or publication of this article. ACS and RBZ acknowledge the financial support of the Accelerating Impacts of CGIAR Climate Research for Africa (AICCRA) project, funded by the International Development Association (IDA) of the World Bank.
The authors declare that the research was conducted in the absence of any commercial or financial relationships that could be construed as a potential conflict of interest.
The author(s) declare that no Gen AI was used in the creation of this manuscript.
All claims expressed in this article are solely those of the authors and do not necessarily represent those of their affiliated organizations, or those of the publisher, the editors and the reviewers. Any product that may be evaluated in this article, or claim that may be made by its manufacturer, is not guaranteed or endorsed by the publisher.
The Supplementary material for this article can be found online at: https://www.frontiersin.org/articles/10.3389/fsufs.2024.1514546/full#supplementary-material
Abdulai, A.-R., Tetteh Quarshie, P., Duncan, E., and Fraser, E. (2023). Is agricultural digitization a reality among smallholder farmers in Africa? Unpacking farmers’ lived realities of engagement with digital tools and services in rural northern Ghana. Agric. Food Secur. 12:11. doi: 10.1186/s40066-023-00416-6
Aker, J. C. (2011). Dial “a” for agriculture: a review of information and communication technologies for agricultural extension in developing countries. Agric. Econ. 42, 631–647. doi: 10.1111/j.1574-0862.2011.00545.x
Aker, J. C., Ghosh, I., and Burrell, J. (2016). The promise (and pitfalls) of ICT for agriculture initiatives. Agric. Econ. 47, 35–48. doi: 10.1111/agec.12301
Aker, J. C., Ksoll, C., and Lybbert, T. J. (2012). Can Mobile phones improve learning? Evidence from a field experiment in Niger. Am. Econ. J. Appl. Econ. 4, 94–120. doi: 10.1257/app.4.4.94
Allmann, K., and Blank, G. (2021). Rethinking digital skills in the era of compulsory computing: methods, measurement, policy and theory. Inf. Commun. Soc. 24, 633–648. doi: 10.1080/1369118X.2021.1874475
Amoussohoui, R., Arouna, A., Bavorova, M., Verner, V., Yergo, W., and Banout, J. (2024). Analysis of the factors influencing the adoption of digital extension services: evidence from the RiceAdvice application in Nigeria. J. Agric. Educ. Ext. 30, 387–416. doi: 10.1080/1389224X.2023.2222109
Aparo, N. O., Odongo, W., and De Steur, H. (2022). Unraveling heterogeneity in farmer’s adoption of mobile phone technologies: a systematic review. Technol. Forecast. Soc. Chang. 185:122048. doi: 10.1016/j.techfore.2022.122048
Arouna, A., Michler, J. D., Yergo, W. G., and Saito, K. (2021). One size fits all? Experimental evidence on the digital delivery of personalized extension advice in Nigeria. Am. J. Agric. Econ. 103, 596–619. doi: 10.1111/ajae.12151
Arslan, A., Floress, K., Lamanna, C., Lipper, L., and Rosenstock, T. S. (2022). A meta-analysis of the adoption of agricultural technology in sub-Saharan Africa. PLOS Sust. Transf. 1:e0000018. doi: 10.1371/journal.pstr.0000018
Asif, A. S., Uddin, M. N., Dev, D. S., and Miah, M. A. M. (2017). Factors affecting mobile phone usage by the farmers in receiving information on vegetable cultivation in Bangladesh. JAI 8, 33–43. doi: 10.17700/jai.2017.8.2.376
Balafoutis, A., Beck, B., Fountas, S., Vangeyte, J., Wal, T. V., Soto, I., et al. (2017). Precision agriculture technologies positively contributing to GHG emissions mitigation, farm productivity and economics. Sustainability 9:1339. doi: 10.3390/su9081339
Basso, B., and Antle, J. (2020). Digital agriculture to design sustainable agricultural systems. Nat. Sust. 3, 254–256. doi: 10.1038/s41893-020-0510-0
Benítez-López, A., Alkemade, R., Schipper, A. M., Ingram, D. J., Verweij, P. A., Eikelboom, J. A. J., et al. (2017). The impact of hunting on tropical mammal and bird populations. Science 356, 180–183. doi: 10.1126/science.aaj1891
Borenstein, M., Hedges, L. V., Higgins, J. P., and Rothstein, H. R. (2009). Introduction to Meta-analysis. Chichester: John Wiley & Sons, Ltd.
Brockwell, S. E., and Gordon, I. R. (2001). A comparison of statistical methods for meta-analysis. Stat. Med. 20, 825–840. doi: 10.1002/sim.650
Butt, B. (2015). Herding by Mobile phone: technology, social networks and the “transformation” of pastoral herding in East Africa. Hum. Ecol. 43, 1–14. doi: 10.1007/s10745-014-9710-4
Cai, Y., Qi, W., and Yi, F. (2023). Smartphone use and willingness to adopt digital pest and disease management: Evidence from litchi growers in rural China. Agribusiness. 39, 131–47. doi: 10.1002/agr.21766
Casaburi, L., Kremer, M., Mullainathan, S., and Ramrattan, R. (2019). Harnessing ICT to increase agricultural production: Evidence from Kenya. Cambridge, MA: Harvard University.
Chellappan, S., and Sudha, R. (2015). Investment, adoption, attitude and extent of participation of farmers in soil conservation projects in the Western Ghats of India. Int. J. Soc. Econ. 42, 251–275. doi: 10.1108/IJSE-10-2013-0219
Chen, W., and Li, X. (2022). Digital inequalities in American disadvantaged urban communities: access, skills, and expectations for digital inclusion programs. Inf. Commun. Soc. 25, 1916–1933. doi: 10.1080/1369118X.2021.1907434
Cole, S. A., and Fernando, A. N. (2021). ‘Mobile’izing agricultural advice technology adoption diffusion and sustainability. Econ. J. 131, 192–219. doi: 10.1093/ej/ueaa084
Cui, Z., Zhang, H., Chen, X., Zhang, C., Ma, W., Huang, C., et al. (2018). Pursuing sustainable productivity with millions of smallholder farmers. Nature 555, 363–366. doi: 10.1038/nature25785
Djokoto, J. G., Owusu, V., and Awunyo-Vitor, D. (2016). Adoption of organic agriculture: evidence from cocoa farming in Ghana. Cogent Food Agric. 2:1242181. doi: 10.1080/23311932.2016.1242181
Dosso, F., Gouroubera, M. W., Idrissou, L., and Moumouni-Mousa, I. (2024). The combination of extension approaches strengthens farmers’ innovativeness in sustainable land management. Environ. Dev. Sustain. 26, 10043–10062. doi: 10.1007/s10668-023-03134-y
Duncombe, R. (2016). Mobile phones for agricultural and rural development: a literature review and suggestions for future research. Eur. J. Dev. Res. 28, 213–235. doi: 10.1057/ejdr.2014.60
Fabregas, R., Kremer, M., and Schilbach, F. (2019). Realizing the potential of digital development: The case of agricultural advice. Science 366:eaay3038. doi: 10.1126/science.aay3038
Feyisa, B. W. (2020). Determinants of agricultural technology adoption in Ethiopia: a meta-analysis. Cogent Food Agric. 6:1855817. doi: 10.1080/23311932.2020.1855817
Giulivi, N., Harou, A. P., Gautam, S., and Guereña, D. (2023). Getting the message out: information and communication technologies and agricultural extension. Am. J. Agric. Econ. 105, 1011–1045. doi: 10.1111/ajae.12348
Gouroubera, M. W., Kora Sabi, A., Bio Comada, T. K., Dosso, F., Fatondji, S. A., Gouthon, M. B., et al. (2023). Designing effective digital-based delivery of climate information for smallholder farmers: a mini meta-analysis on drivers and barriers. Clim. Pol. 24, 1443–1456. doi: 10.1080/14693062.2023.2266475
Gouroubera, M. W., Moumouni, I. M., Okry, F., and Idrissou, L. (2024). A holistic approach to understanding ICT implementation challenges in rural advisory services: lessons from using farmer learning videos. J. Agric. Educ. Ext. 30, 213–232. doi: 10.1080/1389224X.2023.2171077
Gow, G., Chowdhury, A., Ramjattan, J., and Ganpat, W. (2020). Fostering effective use of ICT in agricultural extension: participant responses to an inaugural technology stewardship training program in Trinidad. J. Agric. Educ. Ext. 26, 335–350. doi: 10.1080/1389224X.2020.1718720
Groher, T., Heitkämper, K., and Umstätter, C. (2020). Digital technology adoption in livestock production with a special focus on ruminant farming. Animal. 14, 2404–13. doi: 10.1017/S1751731120001391
Guo, Q., Ola, O., and Benjamin, E. O. (2020). Determinants of the adoption of sustainable intensification in southern African farming systems: a meta-analysis. Sustain. For. 12:3276. doi: 10.3390/su12083276
Gupta, A., Ponticelli, J., and Tesei, A. (2024). Language barriers, technology adoption and productivity: evidence from agriculture in India. Rev. Econ. Stat. 22, 1–28. doi: 10.1162/rest_a_01501
Heponiemi, T., Gluschkoff, K., Leemann, L., Manderbacka, K., Aalto, A.-M., and Hyppönen, H. (2023). Digital inequality in Finland: access, skills and attitudes as social impact mediators. New Media Soc. 25, 2475–2491. doi: 10.1177/14614448211023007
Herrero, M., Thornton, P. K., Mason-D’Croz, D., Palmer, J., Bodirsky, B. L., Pradhan, P., et al. (2021). Articulating the effect of food systems innovation on the sustainable development goals. Lancet Planetar. Health 5, e50–e62. doi: 10.1016/S2542-5196(20)30277-1
Higgins, J., and Green, S. (2008). Cochrane handbook for systematic reviews of interventions. Chichester: John Wiley & Sons, Ltd.
ITU (2020). Facts and figures 2022: Mobile phone ownership. Geneva: International Telecommunication Union (ITU).
Jack, W., Ray, A., and Suri, T. (2013). Transaction networks: evidence from Mobile money in Kenya. Am. Econ. Rev. 103, 356–361. doi: 10.1257/aer.103.3.356
Jensen, R. (2007). The digital provide: information (technology), market performance, and welfare in the south Indian fisheries sector*. Q. J. Econ. 122, 879–924. doi: 10.1162/qjec.122.3.879
Jones-Garcia, E., and Krishna, V. V. (2021). Farmer adoption of sustainable intensification technologies in the maize systems of the global south. A review. Agron. Sust. Dev. 41:8. doi: 10.1007/s13593-020-00658-9
Kabirigi, M., Sekabira, H., Sun, Z., and Hermans, F. (2023). The use of mobile phones and the heterogeneity of banana farmers in Rwanda. Environ. Dev. Sustain. 25, 5315–5335. doi: 10.1007/s10668-022-02268-9
Karlan, D., and Morduch, J. (2010). “Chapter 71 - access to finance” in Handbook of development economics. eds. D. Rodrik and M. Rosenzweig (North-Holland: Elsevier), 4703–4784.
Khan, N. A., Qijie, G., Sertse, S. F., and Nabi, M. N. P. (2019). Farmers’ use of mobile phone-based farm advisory services in Punjab Pakistan, Inf. Dev. 36, 390–402. doi: 10.1177/0266666919864126
Khan, N., Ray, R. L., Kassem, H. S., and Zhang, S. (2022). Mobile Internet Technology Adoption for Sustainable Agriculture: Evidence from Wheat Farmers. Appl. Sci. 12, 4902. doi: 10.3390/app12104902
Klerkx, L., Jakku, E., and Labarthe, P. (2019). A review of social science on digital agriculture, smart farming and agriculture 4.0: new contributions and a future research agenda. NJAS Wageningen J. Life Sci. 91, 1–16. doi: 10.1016/j.njas.2019.100315
Kohl, C., McIntosh, E. J., Unger, S., Haddaway, N. R., Kecke, S., Schiemann, J., et al. (2018). Online tools supporting the conduct and reporting of systematic reviews and systematic maps: a case study on CADIMA and review of existing tools. Environ. Evid. 7:8. doi: 10.1186/s13750-018-0115-5
Krell, N. T., Giroux, S. A., Guido, Z., Hannah, C., Lopus, S. E., Caylor, K. K., et al. (2021). Smallholder farmers’ use of mobile phone services in Central Kenya. Clim. Dev. 13, 215–227. doi: 10.1080/17565529.2020.1748847
LaRochelle, S., and Berkes, F. (2003). Traditional ecological knowledge and practice for edible wild plants: biodiversity use by the Rarámuri, in the Sirerra Tarahumara, Mexico. Int. J. Sustain. Dev. World Ecol. 10, 361–375. doi: 10.1080/13504500309470112
Li, J., Ma, W., and Zhu, H. (2023). A systematic literature review of factors influencing the adoption of climate-smart agricultural practices. Mitig. Adapt. Strateg. Glob. Change 29:2. doi: 10.1007/s11027-023-10098-x
MacPherson, J., Voglhuber-Slavinsky, A., Olbrisch, M., Schöbel, P., Dönitz, E., Mouratiadou, I., et al. (2022). Future agricultural systems and the role of digitalization for achieving sustainability goals. A review. Agron. Sust. Dev. 42:70. doi: 10.1007/s13593-022-00792-6
Maredia, M. K., Reyes, B., Ba, M. N., Dabire, C. L., Pittendrigh, B., and Bello-Bravo, J. (2018). Can mobile phone-based animated videos induce learning and technology adoption among low-literate farmers? A field experiment in Burkina Faso. Inf. Technol. Dev. 24, 429–460. doi: 10.1080/02681102.2017.1312245
Melsen, W. G., Bootsma, M. C. J., Rovers, M. M., and Bonten, M. J. M. (2014). The effects of clinical and statistical heterogeneity on the predictive values of results from meta-analyses. Clin. Microbiol. Infect. 20, 123–129. doi: 10.1111/1469-0691.12494
Metta, M., Ciliberti, S., Obi, C., Bartolini, F., Klerkx, L., and Brunori, G. (2022). An integrated socio-cyber-physical system framework to assess responsible digitalisation in agriculture: a first application with living labs in Europe. Agric. Syst. 203:103533. doi: 10.1016/j.agsy.2022.103533
Michels, M., von Hobe, C.-F., and Musshoff, O. (2020). A trans-theoretical model for the adoption of drones by large-scale German farmers. J. Rural. Stud. 75, 80–88. doi: 10.1016/j.jrurstud.2020.01.005
Mittal, S., and Hariharan, V. K. (2018). Mobile-based climate services impact on farmers risk management ability in India. Clim. Risk Manag. 22, 42–51. doi: 10.1016/j.crm.2018.08.003
Moher, D., Liberati, A., Tetzlaff, J., Altman, D. G., and The, P. G. (2009). Preferred reporting items for systematic reviews and Meta-analyses: The PRISMA statement. PLoS Med. 6:e1000097. doi: 10.1371/journal.pmed.1000097
Mozzato, D., Gatto, P., Defrancesco, E., Bortolini, L., Pirotti, F., Pisani, E., et al. (2018). The role of factors affecting the adoption of environmentally friendly farming practices: can geographical context and time explain the differences emerging from literature? Sustain. For. 10:3101. doi: 10.3390/su10093101
Muema, E., Mburu, J., Coulibaly, J., and Mutune, J. (2018). Determinants of access and utilisation of seasonal climate information services among smallholder farmers in Makueni County, Kenya. Heliyon 4::e00889. doi: 10.1016/j.heliyon.2018.e00889
Mugambiwa, S. S., and Tirivangasi, H. M. (2017). Climate change: a threat towards achieving ‘sustainable development goal number two’ (end hunger, achieve food security and improved nutrition and promote sustainable agriculture) in South Africa. Jàmbá J. Disaster Risk Stu. 9:350. doi: 10.4102/jamba.v9i1.350
Mwalupaso, G. E., Wang, S., Xu, Z., and Tian, X. (2019). Towards auspicious agricultural Informatization—implication of farmers’ behavioral intention apropos of Mobile phone use in agriculture. Sustain. For. 11:6282. doi: 10.3390/su11226282
Mwambi, M., Depenbusch, L., Bonnarith, U., Sotelo-Cardona, P., Kieu, K., di Tada, N., et al. (2023). Can phone text messages promote the use of integrated pest management? A study of vegetable farmers in Cambodia. Ecol. Econ. 204:107650. doi: 10.1016/j.ecolecon.2022.107650
Mwambi, M., Depenbusch, L., Bonnarith, U., Sotelo-Cardona, P., Kieu, K., di Tada, N, et al. (2022). Can phone text messages promote the use of integrated pest management? A study of vegetable farmers in Cambodia. Ecological Economics. 204:107650.
Olum, S., Gellynck, X., Juvinal, J., Ongeng, D., and De Steur, H. (2020). Farmers’ adoption of agricultural innovations: a systematic review on willingness to pay studies. Outlook Agric. 49, 187–203. doi: 10.1177/0030727019879453
Pangaribowo, E., and Gerber, N. (2016). “Innovations for food and nutrition security: impacts and trends,” in Technological and institutional innovations for marginalized smallholders in agricultural development, eds. F. W. Gatzweiler and J. Braunvon (Cham: Springer International Publishing), 41–64
Pittelkow, C. M., Linquist, B. A., Lundy, M. E., Liang, X., van Groenigen, K. J., Lee, J., et al. (2015). When does no-till yield more? A global meta-analysis. Field Crop Res. 183, 156–168. doi: 10.1016/j.fcr.2015.07.020
Quandt, A., Salerno, J. D., Neff, J. C., Baird, T. D., Herrick, J. E., McCabe, J. T., et al. (2020). Mobile phone use is associated with higher smallholder agricultural productivity in Tanzania, East Africa. PLOS ONE 15:e0237337. doi: 10.1371/journal.pone.0237337
Rola-Rubzen, M. F., Paris, T., Hawkins, J., and Sapkota, B. (2020). Improving gender participation in agricultural technology adoption in Asia: from rhetoric to practical action. Appl. Econ. Perspect. Policy 42, 113–125. doi: 10.1002/aepp.13011
Rosenstock, T. S., Joshi, N., Segnon, A. C., Cramer, L., Mwongera, C., Nowak, A. C., et al. (2024). Decision support tools for agricultural adaptation in Africa. Nat. Food 5, 186–188. doi: 10.1038/s43016-024-00936-9
Rosenstock, T. S., Lamanna, C., Chesterman, S., Bell, P., Arslan, A., Richards, M., et al. (2016). The scientific basis of climate-smart agriculture: A systematic review protocol. Copenhagen: CGIAR.
Scheerder, A., van Deursen, A., and van Dijk, J. (2017). Determinants of internet skills, uses and outcomes. A systematic review of the second- and third-level digital divide. Telematics Inform. 34, 1607–1624. doi: 10.1016/j.tele.2017.07.007
Segnon, A. C., Magassa, M., Obossou, E. A., Partey, S. T., Houessionon, P., and Zougmoré, R. B. (2024). Gender vulnerability assessment to inform gender-sensitive adaptation action: a case study in semi-arid areas of Mali. Front. Clim. 6:1418015. doi: 10.3389/fclim.2024.1418015
Silvestri, S., Richard, M., Edward, B., Dharmesh, G., and Dannie, R. (2021). Going digital in agriculture: how radio and SMS can scale-up smallholder participation in legume-based sustainable agricultural intensification practices and technologies in Tanzania. Int. J. Agric. Sustain. 19, 583–594. doi: 10.1080/14735903.2020.1750796
Spurk, C., Koch, C., Bürgin, R., Chikopela, L., Konaté, F., Nyabuga, G., et al. (2023). Farmers’ innovativeness and positive affirmation as main drivers of adoption of soil fertility management practices – evidence across sites in Africa. J. Agric. Educ. Ext. 18, 1–25. doi: 10.1080/1389224X.2023.2281909
Stang, A. (2010). Critical evaluation of the Newcastle-Ottawa scale for the assessment of the quality of nonrandomized studies in meta-analyses. Eur. J. Epidemiol. 25, 603–605. doi: 10.1007/s10654-010-9491-z
Stanley, T. D., Doucouliagos, H., Giles, M., Heckemeyer, J. H., Johnston, R. J., Laroche, P., et al. (2013). Meta-analysis of economics research reporting guidelines. J. Econ. Surv. 27, 390–394. doi: 10.1111/joes.12008
Tey, Y. S., and Brindal, M. (2024). A meta-analysis of factors speeding the adoption of agricultural intensification methods in Africa. J. Agric. Educ. Ext. 30, 521–534. doi: 10.1080/1389224X.2023.2240758
Theis, S., Lefore, N., Meinzen-Dick, R., and Bryan, E. (2018). What happens after technology adoption? Gendered aspects of small-scale irrigation technologies in Ethiopia, Ghana, and Tanzania. Agric. Hum. Values 35, 671–684. doi: 10.1007/s10460-018-9862-8
Totin, E., Segnon, A. C., Schut, M., Affognon, H., Zougmoré, R. B., Rosenstock, T., et al. (2018). Institutional perspectives of climate-smart agriculture: a systematic literature review. Sustain. For. 10:1990. doi: 10.3390/su10061990
Tsan, M., Totapally, S., Hailu, M., and Addom, B. K. (2021). The digitalisation of African agriculture report, 2018–2019. Wageningen: Technical Centre for Agricultural and Rural Cooperation (CTA).
van Baardewijk, M. (2017). The impact of Mobile phone use and IKSL’s audio messages on the Asset Base of poor farmers in Lucknow, India. Electr. J. Inf. Syst. Dev. Countr. 79, 1–17. doi: 10.1002/j.1681-4835.2017.tb00584.x
Van Campenhout, B., Spielman, D. J., and Lecoutere, E. (2021). Information and Communication Technologies to Provide Agricultural Advice to Smallholder Farmers: Experimental Evidence from Uganda. Am J Agric Econ. 103, 317–37. doi: 10.1002/ajae.12089
van Deursen, A. J. A. M., van der Zeeuw, A., de Boer, P., Jansen, G., and van Rompay, T. (2021). Digital inequalities in the internet of things: differences in attitudes, material access, skills, and usage. Inf. Commun. Soc. 24, 258–276. doi: 10.1080/1369118X.2019.1646777
van Dijk, J. A. G. M. (2013). “A theory of the digital divide” in The digital divide: The internet and social inequality in international perspective. eds. M. Regnedda and G. W. Muschert (London: Routledge), 29–51.
van Dijk, J. A. G. M., and van Deursen, A. J. A. M. (2014). Digital skills: Unlocking the information society. New York, NY: Palgrave Macmillan US.
Veroniki, A. A., Jackson, D., Viechtbauer, W., Bender, R., Bowden, J., Knapp, G., et al. (2016). Methods to estimate the between-study variance and its uncertainty in meta-analysis. Res. Synth. Methods 7, 55–79. doi: 10.1002/jrsm.1164
Viechtbauer, W. (2010). Conducting Meta-analyses in R with the metafor package. J. Stat. Soft. 36, 1–48. doi: 10.18637/jss.v036.i03
Wang, X., Tan, S. C., and Li, L. (2020). Technostress in university students’ technology-enhanced learning: an investigation from multidimensional person-environment misfit. Comput. Hum. Behav. 105:106208. doi: 10.1016/j.chb.2019.106208
Wyche, S., and Steinfield, C. (2016). Why Don’t farmers use cell phones to access market prices? Technology affordances and barriers to market information services adoption in rural Kenya. Inf. Technol. Dev. 22, 320–333. doi: 10.1080/02681102.2015.1048184
Xie, H., and Huang, Y. (2021). Influencing factors of farmers’ adoption of pro-environmental agricultural technologies in China: Meta-analysis. Land Use Policy 109:105622. doi: 10.1016/j.landusepol.2021.105622
Yegbemey, R. N., and Egah, J. (2021). Reaching out to smallholder farmers in developing countries with climate services: a literature review of current information delivery channels. Clim. Serv. 23:100253. doi: 10.1016/j.cliser.2021.100253
Keywords: mobile phone, technology adoption, impact assessment, scaling, meta-analysis, digital agriculture, ICT
Citation: Gouroubera MW, Adechian SA, Segnon AC, Moumouni-Moussa I and Zougmoré RB (2025) Drivers and impacts of mobile phone-mediated scaling of agricultural technologies: a meta-analysis. Front. Sustain. Food Syst. 8:1514546. doi: 10.3389/fsufs.2024.1514546
Received: 21 October 2024; Accepted: 13 December 2024;
Published: 06 January 2025.
Edited by:
Siphe Zantsi, Agricultural Research Council of South Africa (ARC-SA), South AfricaReviewed by:
Nasiphi Bontsa, University of Fort Hare, South AfricaCopyright © 2025 Gouroubera, Adechian, Segnon, Moumouni-Moussa and Zougmoré. This is an open-access article distributed under the terms of the Creative Commons Attribution License (CC BY). The use, distribution or reproduction in other forums is permitted, provided the original author(s) and the copyright owner(s) are credited and that the original publication in this journal is cited, in accordance with accepted academic practice. No use, distribution or reproduction is permitted which does not comply with these terms.
*Correspondence: Alcade C. Segnon, YWxjYWRlc2VAZ21haWwuY29t
Disclaimer: All claims expressed in this article are solely those of the authors and do not necessarily represent those of their affiliated organizations, or those of the publisher, the editors and the reviewers. Any product that may be evaluated in this article or claim that may be made by its manufacturer is not guaranteed or endorsed by the publisher.
Research integrity at Frontiers
Learn more about the work of our research integrity team to safeguard the quality of each article we publish.